1. INTRODUCTION
Vessel traffic service (VTS) refers to an integrated shore-side service provided to vessels navigating in territorial waters (Hughes, Reference Hughes1998). As early as 1948, the first radar chain, the predecessor of VTS, was established to minimise the delay of vessels at the port of Douglas (Isle of Man, UK) (Praetorius, Reference Praetorius2012). It was found that VTS can promote efficient, safe navigation in areas with high traffic density. Hence, more VTS centres have been established with advanced techniques, such as automatic identification system (AIS), marine geographic information system, meteorological/hydrological system, and closed-circuit television (Xu et al., Reference Xu, Li, Chen, Lee and Lee2015; Li et al., Reference Li, Chen and Khoo2016). Nowadays, there are more than 500 VTS centres around the world. According to the International Convention for the Safety of Life at Sea (SOLAS), Chapter V, Regulation 12, there are two types of VTS operations: port and coastal operations. Port operations focus on providing services to vessels entering or leaving ports, while coastal operations mainly provide services to vessels passing through a sea area. For both port and coastal operations, VTS operators (VTSOs) monitor the traffic situation through VTS consoles and directly communicate with vessels to provide three types of service: information, navigational assistance, and traffic organisation, through broadcasts on VHF radio.
VTS operations are complex and dynamic in nature thereby resulting in a high degree of task-related human fatigue (Akhtar and Utne, Reference Akhtar and Utne2014; Michalaki et al., Reference Michalaki, Quddus, Pitfield and Huetson2015; Li et al., Reference Li, Lee, Chen and Khoo2019), which causes an increase in response time and undermines the capacity of human operators. Thus, human fatigue is increasingly being considered as the primary cause of most traffic accidents (Bye and Aalberg, Reference Bye and Aalberg2018; Renata et al., Reference Renata, Li, Lee and Chen2018; Herlambang et al., Reference Herlambang, Taatgen and Cnossen2019). More recently, several maritime casualties have been caused by watchkeepers falling asleep while on duty (Jepsen et al., Reference Jepsen, Zhao, Pekcan, Barnett and Van Leeuwen2017). Hence, it is imperative and critical to manage task-related human fatigue in VTS.
Recognising causal factors and fatigue symptoms is generally considered as the first step in managing human fatigue (Ji et al., Reference Ji, Lan and Looney2006). It helps in understanding human fatigue as well as in developing fatigue countermeasures (May and Baldwin, Reference May and Baldwin2009). Ji et al. (Reference Ji, Lan and Looney2006) found that human fatigue could be modelled based on a set of fatigue-inducing factors. Thus, causal factors analysis has received interest from various fields, such as road transportation (Sallinen and Hublin, Reference Sallinen and Hublin2015), hospital (Paterson et al., Reference Paterson, Sofianopoulos and Williams2014), and maritime transportation (Bal et al., Reference Bal, Arslan and Tavacioglu2015).
Owing to a similar target of maintaining safety and smooth traffic, VTS operations are often compared with air traffic control (ATC) (Praetorius et al., Reference Praetorius, Van Westrenen, Mitchell and Hollnagel2012). Recent studies indicated that human fatigue of ATC operators is mainly caused by inadequate sleep, 24 h operations, the length of the duty period, and the pattern of workload (Gander, Reference Gander2001). Though there are some similarities between VTS operations and air traffic management, the pattern of workload is greatly different. Specifically, VTSOs currently only provide information and advisory services instead of instructions to pilots, while ATCs provide instructions to aircraft pilots (Praetorius, Reference Praetorius2012; Chang et al., Reference Chang, Yang and Hsu2019; Chen et al., Reference Chen, Lu and Mao2019). Moreover, VTSOs monitor two-dimensional traffic space, while ATCs monitor three-dimensional traffic space (Liu et al., Reference Liu, Trapsilawati, Lan, Sourina, Johan, Li, Chen and Mueller-Wittig2019). Due to these significant differences, the causal factors of human fatigue in VTS might be different from air traffic management and therefore are worthy of investigation.
The specific aim of this work is to determine the key causal factors and symptoms of human fatigue with a focus on VTS operations and the workspace environment. Several challenges are waiting to be addressed to achieve this aim: first, there is no clear and widely acknowledged definition of human fatigue (Trejo et al., Reference Trejo, Kubitz, Rosipal, Kochavi and Montgomery2015). It is always used as a synonym for tiredness and drowsiness (Trejo et al., Reference Trejo, Kubitz, Rosipal, Kochavi and Montgomery2015). Nevertheless, some researchers have indicated that there are significant differences between drowsiness and human fatigue (Phillips, Reference Phillips2015; Wolf et al., Reference Wolf, Perhats, Delao and Martinovich2017). Hence, it is necessary to define human fatigue clearly before identifying causal factors. Second, human fatigue is usually underestimated and not reported in maritime accidents (Alonso et al., Reference Alonso, Esteban, Useche and López De Cózar2016). Objectively assessing the importance of causal factors of human fatigue is difficult due to the limited accident reports related to human fatigue in VTS. Third, there are too many interacting factors that induce human fatigue (Sallinen and Hublin, Reference Sallinen and Hublin2015). Considering the complex inter-relations, it is very challenging to determine the key causal factors.
To reach the target of identifying the critical causal factors of human fatigue in VTS and addressing the abovementioned challenges, in this paper the authors propose a novel definition of human fatigue, develop a task-driven approach for causal factor identification and present a fatigue causal network for evaluation. The remainder of this paper is organised as follows. Section 2 provides a review of definitions of human fatigue and the methods of evaluating fatigue-inducing factors. Based on the analysis of existing studies, a novel definition of human fatigue is provided. Sections 3 and 4, respectively, describe the research methodology, including task-driven identification and causal network-based evaluation, and discuss the results of the vital causal factors and symptoms of human fatigue in VTS. Section 5 summarises the main conclusions reached in this work.
2. LITERATURE REVIEW
Defining human fatigue can help to determine the scope of this work and reduce the misunderstandings inherent in subjectively identifying causal factors (Phillips, Reference Phillips2015). Hence, definitions of human fatigue are reviewed below. In addition, the commonly used approaches to evaluate the importance of fatigue-inducing factors are analysed.
2.1. Definitions of human fatigue
Human fatigue is normally defined as a suboptimal state caused by exertion/inadequate rest. For the suboptimal state, several definitions describe human fatigue, especially mental fatigue, as a feeling (Phillips, Reference Phillips2015; Trejo et al., Reference Trejo, Kubitz, Rosipal, Kochavi and Montgomery2015). For example, fatigue was defined as an overwhelming sense of tiredness, a lack of energy, and a feeling of exhaustion. These experiential definitions of human fatigue heavily depend on subjective sense and individual understanding. Besides, the links of perceived fatigue to human performance are not straightforward (Phillips, Reference Phillips2015). Hence, other researchers define human fatigue from the perspective of objective measurements, including psychophysiological depletion and performance decrement (Alonso et al., Reference Alonso, Esteban, Useche and López De Cózar2016).
The causes of human fatigue are normally varied with different definitions. Some researchers suggested that fatigue is caused by inadequate rest (Gander, Reference Gander2001; Job and Dalziel, Reference Job, Dalziel, Hancock and Desmond2001). However, this kind of definition is used in a limited manner because it can easily be confused with drowsiness. Most studies agree that fatigue is caused by demanding physical or mental activities (Charbonnier et al., Reference Charbonnier, Roy, Bonnet and Campagne2016; Van Cutsem et al., Reference Van Cutsem, Marcora, De Pauw, Bailey, Meeusen and Roelands2017). Nevertheless, it has been found that both high-demand and low-demand work can induce human fatigue (Desmond and Hancock, Reference Desmond, Hancock, Hancock and Desmond2001). In addition, the workload, emotions and motivations of people are always linked to human fatigue (Montgomery et al., Reference Montgomery, Montgomery and Guisado1995). Hockey (Reference Hockey2013) defined mental fatigue as a lack of motivation, which was caused by decreased rewards of task engagement over time. As shown in Table 1, most researchers suggested that human fatigue is a psychophysiological condition caused by exertion, and there is still no definition that considers the specific consequences of fatigue and the effects of both exertion and subjective feelings. Phillips (Reference Phillips2015) claimed that the definition of human fatigue should include experiential fatigue as a central aspect. Hence, a novel definition that accounts for the interactions between subjective feelings and exertion concerning psychophysiological states is proposed in this study.
Table 1. Summary of definitions of human fatigue.

2.2. Identification of key fatigue-inducing factors
Researchers have investigated the causal factors of human fatigue through questionnaires, interviews, task analyses, and literature reviews (Bal et al., Reference Bal, Arslan and Tavacioglu2015; Sallinen and Hublin, Reference Sallinen and Hublin2015). More specifically, Sallinen and Hublin (Reference Sallinen and Hublin2015) identified the causal factors of human fatigue in road transportation by conducting a literature review. Paterson et al. (Reference Paterson, Sofianopoulos and Williams2014) conducted a survey to understand the causal factors contributing to human fatigue, and identified three leading causes, namely: sleep history, circadian factors and task-related factors. Bal et al. (Reference Bal, Arslan and Tavacioglu2015) analysed causal factors of human fatigue in seafarers using task analyses and interviews. Through interviewing seafarers and thoroughly examining their daily operations, Bal and colleagues identified the potential causal factors of seafarers' human fatigue.
To select key causal factors of human fatigue quantitatively, Bal et al. (Reference Bal, Arslan and Tavacioglu2015) adopted the analytic hierarchy process method, which utilises pair-wise comparisons to identify critical factors. However, it is computationally intensive and not suitable for situations where a vast number of causal factors are involved (Bal et al., Reference Bal, Arslan and Tavacioglu2015). To the best of the authors' knowledge, quantitative evaluations of causal factors of human fatigue are still lacking. Moreover, the complex inter-relationships between these causal factors remain unclear (Bal et al., Reference Bal, Arslan and Tavacioglu2015). To deal effectively with the complex inter-relationships, the authors postulate that causal networks can be used to perform causal factors evaluation.
Causal networks have been used to deal with problems in different domains, such as philosophy, health and environment, and tourism (Hollnagel, Reference Hollnagel1998; De Ambroggi and Trucco, Reference De Ambroggi and Trucco2011). Essentially, a causal network can be used to express the inter-relationships between causal factors visually and effectively (Hollnagel, Reference Hollnagel1998). De Ambroggi and Trucco (Reference De Ambroggi and Trucco2011) established a causal network of performance shaping factors to analyse their effect on human errors. Although causal networks have been widely used in various problem contexts, they have never been utilised to deal with human fatigue issues.
2.3. A novel definition of human fatigue
In this study, a novel definition was proposed to achieve the following improvements. (1) to focus on task-related human fatigue, the definition indicates that the causes of human fatigue are work-related. (2) The definition indicates the multidimensional consequences of human fatigue. Some researchers have divided fatigue into mental and physical fatigue (Trejo et al., Reference Trejo, Kubitz, Rosipal, Kochavi and Montgomery2015) based on physical or mental exertion. Nevertheless, this study proposes to combine them, as physical fatigue interacts with mental fatigue (Van Cutsem et al., Reference Van Cutsem, Marcora, De Pauw, Bailey, Meeusen and Roelands2017). (3) The definition optimises face validity by using an experiential and operational definition. To enable causal factors to be identified via subjective methods, such as surveys and interviews, everyday language should be used to define human fatigue (Phillips, Reference Phillips2015). In addition, the consequences of fatigue can be assessed by subjective feelings. According to Table 1, the existing definitions basically fail to satisfy these aspects. Based on the understanding above and the current definitions, the following novel definition is proposed:
Task-related human fatigue is a suboptimal physical, emotional, motivational, cognitive condition caused by a prolonged period of exposure to task-related stimuli.
Instead of using a phrase such as ‘psychophysiological’, the authors explicitly indicate the multiple dimensions of fatigue using everyday language. Fatigue is normally considered a decrement in physical and mental condition. Nevertheless, in some studies, the mental condition is always confused with cognitive states (Van Cutsem et al., Reference Van Cutsem, Marcora, De Pauw, Bailey, Meeusen and Roelands2017). Cognitive fatigue is sometimes used instead of mental fatigue, as ‘mental fatigue’ was found to be induced by typical cognitive tasks. Several multi-dimension fatigue scales (Ahsberg et al., Reference Ahsberg, Gamberale and Gustafsson2000; Zuraida and Iridiastadi, Reference Zuraida and Iridiastadi2015) show that fatigue includes various aspects, such as physical, cognitive, emotional and motivational aspects. Therefore, the definition proposed in this study implies that people under fatigue may experience performance decrement, a lack of motivation, a low mood and even impaired cognitive capacity, which is in line with the ideas of Zuraida and Iridiastadi (Reference Zuraida and Iridiastadi2015) and Van Cutsem et al. (Reference Van Cutsem, Marcora, De Pauw, Bailey, Meeusen and Roelands2017).
The definition implies that fatigue is a matter of degree relative to a specific optimal condition, where the optimal state is the average health condition after the individual is fully recovered and rested (Phillips, Reference Phillips2015). Defining fatigue as a relative condition enables people to recognise fatigue states based on experience. Additionally, it is in line with the idea that fatigue is a decrement in psychophysiological state (Phillips, Reference Phillips2015).
The reasons why ‘a prolonged period of exposure to task-related stimuli’ is used as the cause of human fatigue can be explained from three aspects. First, fatigue is a gradual and cumulative phenomenon (Charbonnier et al., Reference Charbonnier, Roy, Bonnet and Campagne2016); hence, the definition highlights the time effects of task-related factors. Second, instead of ‘exertion’, ‘task-related stimuli’ are used as the cause. The term ‘stimuli’ is in line with the definitions of Dodge (Reference Dodge1982) and Hirshkowitz (Reference Hirshkowitz2013). According to the definition of Phillips (Reference Phillips2015), the term ‘exertion’ emphasises that people have to put forth efforts. Nevertheless, people may experience fatigue under low-demand work conditions because such conditions are boring and monotonous. Also, people may have emotional fatigue due to psychological factors, such as feeling decreased rewards of task engagement over time or even feeling unwillingness to engage in some tasks (Hockey, Reference Hockey2013). Hence, the term ‘stimuli’ is used.
3. METHODOLOGY
Since causal factors of human fatigue are task specific, it is necessary to elicit factors with a focus on VTS operations. Hence, the authors propose a task-driven approach for elicitation of factors and a causal network-based approach for selecting causal factors.
3.1. Participants
This study involved a sample of 68 VTSOs, recruited from a VTS centre in Singapore. Specifically, eight VTSOs (male = 7; $\hbox{female}=1$) were recruited for the unstructured interview. They had an average age of 35 years (standard deviation (SD) = 5 years), with an average experience of eight years. The other 60 VTSOs participated in the questionnaire-based fatigue survey, where VTSOs reported the importance of causal factors and how often they suffered from the fatigue symptoms (
$1=\hbox{never}$,
$5=\hbox{always}$). In total, questionnaires from 48 licensed VTSOs (
$\hbox{male}=40$,
$\hbox{female}=8$), with an average age of 41 years and an average experience of 11 years, were collected. The response rate of 80% is acceptable (Liu et al., Reference Liu, Lyu, Qiu, He, Tong, Zhao and Li2017). The participants were informed in advance of the objectives of this study and of the definition of task-related human fatigue. They were required to sign informed consent for participating in this study. This research complied with the American Psychological Association Code of Ethics (Stricker, Reference Stricker2010) and was approved by the institutional review board. Informed consent was obtained from each participant.
3.2. Task-driven identification of causal factors
Figure 1 shows the framework of the proposed task-driven causal

Figure 1. Framework of the identification of task-driven causal factors.
factor identification method. A two-month field observation was conducted in VTS centres in Singapore to understand the daily tasks of VTSOs. Field observations were carried out in a naturalistic and passive manner, i.e., with the researcher standing beside an operator without interrupting his or her work.
Hierarchy task analysis is employed to decompose the main task hierarchically into subtasks to facilitate a better understanding of an operational process. Edwards (Reference Edwards1981) indicated that humans should interact with several aspects, when performing any task, and proposed the SHELL model, which is named after the initial letters of its components: software, hardware, environment and liveware. Based on this theory, SHELL-based interaction analysis is conducted to elicit causal factors from expert knowledge, as shown in Figure 1. Any subtask T n could be represented as $T_{n} \to [ S_{n}, \; H_{n}, \; E_{n}, \; L_{n}, \; CL_{n}] $, as shown in Figure 2. In the present study, experts analysed each subtask and determined how VTSOs interact with the system, including software, hardware, environment and other liveware. Specifically, S refers to non-physical and intangible aspects of a target industry that govern how the industry operates and how the information within the industry is organised. Software factors such as safety procedures, regulations and work sector characteristics have an influence on workload and may cause human fatigue. H refers to physical elements such as operating consoles, seats and computers. E refers to the context in which tasks are executed. L deals with the human elements of the target industry. CL means a central human element, the one who conducts the task.

Figure 2. Illustration of (a) SHELL-based interaction analysis and (b) SCM-based analysis.
With an understanding of VTS operations and interacting factors, an unstructured interview with eight VTSOs was conducted. The unstructured interviews consist of three crucial stages: asking open-ended questions, probing for details, and seeking to verify unclear points. The operators were asked to recall the challenges or problems they face in performing each subtask or interacting with each element. Probing questions were generated when an operator mentioned any fatigue patterns in order to examine it in more detail regarding the fatigue aspect. According to the novel definition, fatigue patterns can include physical, emotional, motivational and cognitive decrements. Finally, the interviewer repeated what she/he heard so that the interviewee could confirm the information summarised. To encourage operators to explore their knowledge and provide more information about fatigue, their identities were kept anonymous throughout the study.
The core concept of the Swiss cheese model (SCM) postulates that both direct and indirect factors, including preconditions, unsafe supervision, and organisational influences, cause accidents (Reason, Reference Reason1990). For the factors belonging to preconditions, their corresponding indirect factors fall under unsafe supervision and organisational influences. Figure 2(b) illustrates the process for SCM-enabled causal factor analysis. Based on the framework of the Human Factors Analysis and Classification System for Maritime Accidents (HFACS-MA), unsafe supervision includes inappropriately planned operations, the failure to rectify known problems, inadequate supervision, and violations by supervisors (Chen et al., Reference Chen, Wall, Davies, Yang, Wang and Chou2013). Organisational influences consist of the organisational climate, resource management and regulatory processes (Chen et al., Reference Chen, Wall, Davies, Yang, Wang and Chou2013).
3.3. Network-based evaluation
A causal network of human fatigue was established to evaluate the causal factors and their complex inter-relationships. The specific steps for realising the causal network are summarised as follows:
1. Analyse the inter-relationships between any two causal factors;
2. Add the necessary virtual nodes;
3. Rate the strength of each node; and
4. Establish a matrix to model the causal network.
There are two types of influence that one causal factor can have on another, that is, direct and indirect. Figure 3 shows the inter-relationships among the causal factors. For the direct influence (A), CF j interacts with CF k by directly affecting CF i. Regarding the indirect influence (B), CF j interacts with CF k by affecting the interaction between CF i and CF k. In this study, a matrix is established to present the inter-relationships among the causal factors. In general, direct influences can be represented by the entries of the matrix itself; however, representing indirect influences is more challenging (De Ambroggi and Trucco, Reference De Ambroggi and Trucco2011). To address this issue, that is, representing indirect influence, a virtual node (V) is introduced to convert an indirect influence into a direct influence. By using the virtual node, the indirect influence can be treated as that CF j influences CF k indirectly through the virtual element, V j. In this manner, the introduction of virtual node V j enables the indirect influence of CF i on CF k to be more clearly illustrated.

Figure 3. Illustration of the inter-relations among causal factors (CF).
Basically, the causal network utilises all causal factors, virtual nodes and fatigue symptoms (SF) as elements. To rate the importance of each element, an ordinal scale of low, moderate and high (Miles and Huberman, Reference Miles and Huberman1984), is adopted. The ordinal scale is subsequently converted into a comparative format where ‘1’ denotes ‘low’, ‘3’ represents ‘moderate’ and ‘5’ indicates ‘high’. The following matrix represents the inter-relations of any two elements:

Each column of C is a principal eigenvector of the effects of the jth element on the ith element. For i = j, c ij refers to the strength of the ith node. Table 2 illustrates the item format of the questionnaires.
Table 2. Demonstration of item format in the questionnaires.

CF = causal factor; SF = fatigue symptoms.
For each factor involved in the causal network, its importance can be determined by out-degree $( k_{{\rm out}}) $ and in-degree (k in), which refers to the number of adjacent nodes and jointly considers the direction and strength of the inter-relations with adjacent nodes (Freeman, Reference Freeman1977). The out-degree and in-degree are calculated by Equations (2) and (3):


where $k_{{\rm{out}}_{i}}$ refers to the out-degree of the ith node, and
$k_{{\rm{in}}_{i}}$ denotes the in-degree of ith node, and c ij is the inter-relation strength between factor i and factor j.

where T is the ratio of out-degree and in-degree. For a causal factor, whose T is larger than the threshold α, the causal factor can be classified as indirect factor (Niemeijer and de Groot, Reference Niemeijer and de Groot2008). α is set to be 10 in this study. Hence, indirect factors have a larger number of out-degree than in-degree (Niemeijer and de Groot, Reference Niemeijer and de Groot2008). For a causal factor, whose T is smaller than the threshold β, the causal factor can be classified as individual resilience. β is normally set to be smaller than 0·5. Hence, individual resilience has a larger amount of in-degree and a smaller amount of out-degree. The remaining causal factors, whose out-degree is roughly equal to the in-degree are state factors, named as task-related stimuli in this study.
Based on the out-degree and in-degree, the influence power of the ith node, I i, can be represented by Equation (5) as follows.

Basically, the influence of power indicates the impact of a node on its adjacent nodes. To determine the importance of a node, both its influence on its adjacent nodes and the range of influence need to be assessed. By considering the general properties of the causal network, the number of paths from the root nodes to the end of the chain node that passes through the ith node is defined as its influence range (Np i). Np i, which is a classical problem in network analysis, can be determined by using a depth-first search algorithm.
4. RESULTS AND DISCUSSION
4.1. Task analysis and identification of task-related factors
The VTS of Singapore provides only an information service to vessels. Figure 4 depicts the hierarchical analysis of the information service. First, VTSOs identify potential unsafe events by monitoring the traffic conditions of vessels and communicating with the masters or pilots. Second, once a possible hazardous event is spotted, VTSOs will gather the basic information and refresh the port traffic management system to check vessel movements. Finally, based on the information gathered, VTSOs will provide corresponding advice to the vessels, such as the intentions of other vessels, the number of vessels in the vicinity, and the type of vessels in the vicinity. At times, VTSOs may broadcast temporary notices to all vessels under special conditions, such as poor visibility.

Figure 4. Hierarchical analysis of information service.
The task analysis identified 23 task-related factors, as listed in Table 3. Besides these task-related factors, 24 factors were collected by SCM-based analysis, as listed in Table 4.
Table 3. Task-related factors.
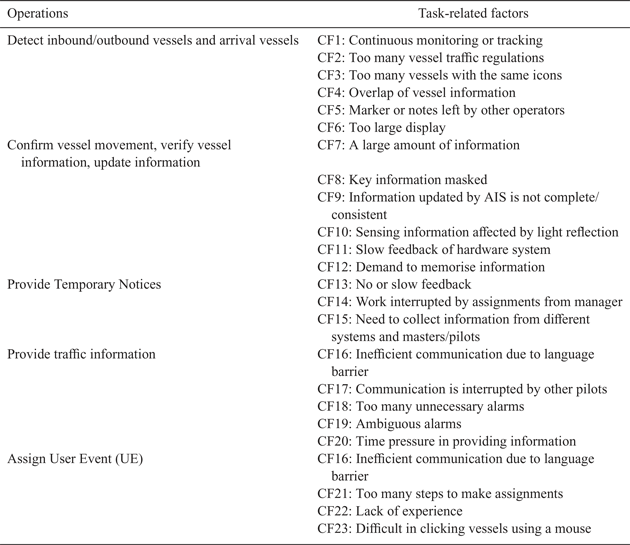
CF = causal factor.
Table 4. The causal factors (CF) identified by SCM-based analysis.

4.2. Symptoms of human fatigue in VTS
Table 5 shows the results related to the top ten fatigue symptoms in VTS. The mean value listed in the third column indicates the impact of fatigue symptoms. VTSOs suffer from multidimensional fatigue, and physical fatigue seems to be more serious than cognitive fatigue in VTS. There are six physical symptoms ranked in the top ten, while there are only two cognitive symptoms in Table 5. Owing to the dynamic workload and high intensity of information, cognitive fatigue has normally received more attention in VTS studies (Li et al., Reference Li, Lee, Chen and Khoo2019). This study indicates, however, that VTSOs suffer from a high possibility of physical fatigue. Accordingly, the countermeasure technologies should focus more on physical fatigue (May and Baldwin, Reference May and Baldwin2009).
Table 5. Fatigue symptoms in VTS.

SF = fatigue symptom.
In addition to physical and cognitive symptoms, symptoms related to motivation and emotion can be found in Table 5. The top-ranking symptom of human fatigue is ‘eyes feel strained’; also, its SD is smaller than most other symptoms. The decrement in cognitive states does not seem to be serious, as cognitive symptoms have a low ranking. Moreover, the symptom of ‘cannot sense information’ has the largest SD. It can be deduced that some operators suffer from impaired cognitive capacities, while others can maintain their information-processing capacity.
4.3. Key causal factors of human fatigue in VTS
In this study, the factors ranked in the top 25% were identified as key causal factors and are analysed below. Table 6 shows the key causal factors and their rankings in three dimensions: (1) a1 is the rank of the influence power (I) of each causal factor; (2) a2 is the rank of the influence range (Np) of each causal factor; and (3) a3 is the rank of influence power.
Table 6. Key causal factors of human fatigue in VTS.

Following the division method described in Section 3.3, ‘safety culture’ and ‘organisational rules’ were classified as indirect causal factors, as their T values are larger than threshold α; they have high rankings in both influence power and influence range. As previously mentioned, influence power indicates the influence of a node on adjacent nodes; the influence range indicates the ranges of its impact. Hence, the results indicate that ‘safety culture’ and ‘organisational rules’ have great effects on the entire fatigue causal network.
‘Rest/recovery’, ‘workload’, ‘subjective feelings’ and ‘health and wellness
condition’ are aspects of individual resilience that can be considered as preconditions of human fatigue, as their T values are smaller than threshold β. Table 6 shows that all of them except ‘subjective feelings’ are highly ranked in the three dimensions. Considering the three dimensions, ‘rest’ was ranked first. Hence, the ‘work/rest arrangement’ of VTS requires more development. VTSOs work in shift work; hence, they always suffer from the problem of rest and recovery. ‘Workload’ was ranked second; its a3 ranking is much lower than that of ‘rest’. a3 shows the subjective feelings of VTSOs. It can be concluded that operators felt the problem of ‘rest’ is more serious than ‘workload’. VTSOs did not work close to the limits of their capacity. Nevertheless, they felt a lack of motivation to continue their work.
Other key factors include ‘language barrier’, ‘amount of information’, ‘marker or notes’, ‘unnecessary alarms’, ‘overlap of vessel information’ and ‘continuous monitoring’. These are task-related stimuli and direct causal factors of human fatigue in VTS, as they have a high ranking in a3. A factor with a high ranking in a3 means that operators encountered the factor very frequently and felt that the factor is critical. These task-related stimuli are discussed below.
4.3.1. Language barrier
From the task decomposition, it appears that VTSOs frequently communicate with the pilots of vessels coming from different countries. This communication can be easily interrupted by the ‘language barrier’, as the crews of vessels maybe not proficient in English. During the unstructured interview, the VTSOs mentioned that pilots may ignore advice or even insist on speaking their own language during the communication.
4.3.2. Amount of information
From the task analysis of VTS operations, VTSOs frequently worked close to the limits of their information-processing capacity. They have to handle numerous warnings, calls from vessels, traffic information and so on. The effort required to process high-density information and make adequate decisions simultaneously can be daunting and may potentially induce a high workload. Due to its direct effect on workload, this factor ranks high in both a1 and a2.
4.3.3. Overlap of vessel information
The identification information of a vessel, including its name, call number and type, is displayed next to its icon. Nevertheless, when a vessel is close to another vessel, their information always overlaps, as shown in Figure 5. In such a case, VTSOs have to drag the information labels manually. According to the field observation, VTSOs spent most of their time dealing with the overlapping information.

Figure 5. Information overlap.
4.3.4. Markers or notes
VTSOs used ‘markers or notes’ to mark the vessels that require their attention, as shown in Figure 6. However, there is no standard in making markers. As a result, operators make markers according to their preferences and working habits. They may be confused by markers or notes left by other operators.

Figure 6. Markers and notes used by VTSOs.
4.3.5. Unnecessary alarms
The integrated VTS alarm system was developed to assist in identifying potentially unsafe issues. However, the VTSOs mentioned that most of these alarms are unnecessary. These unnecessary alarms induce distrust in systems, resulting in a slow response to the alarm system.
4.3.6 Continuous monitoring
With the increase in automation technology in VTS, the role of a VTSO tends to change from a controller to a monitor, whose main task is monitoring tasks. Thousands of laboratory experiments have demonstrated that human vigilance will easily be interrupted when people are working on monitoring tasks (Masoudian and Razavi, Reference Masoudian and Razavi2018). Also, VTSOs mentioned that their eyes felt strained after continuous monitoring. This factor was ranked just after ‘rest’ and ‘amount of information’ in a3.
In summary, the causal network-based evaluation provides a comprehensive assessment of three aspects: the influence range, influence impact, and subjective rate of importance. In general, operators could identify direct task-related stimuli according to their experience. Nevertheless, the importance of indirect causal factors could not be determined by subjective feelings. The proposed network can well fill this gap (Liu et al., Reference Liu, Lyu, Qiu, He, Tong, Zhao and Li2017).
4.4. Recommendations for recruiting and training VTS personnel
Some recommendations can be generated for recruiting and training suitable candidates to reduce the risks of human fatigue. According to the IALA ‘Recommendation (V-103) Standards for Training and Certification of VTS Personnel’ (Hughes, Reference Hughes2009; IALA, 2009), there are five tests in selecting VTS personnel, namely: entry requirements, aptitude assessment, assessment of prior learning, medical/physical requirements and personal attributes assessment.
For entry requirements, V103 states that the candidate should be fluent in English. However, in this study, the ‘language barrier’ was still identified as a critical causal factor, though Singapore VTSOs are proficient in English. This study revealed that the language problem was caused by masters/pilots instead of VTSOs, since some masters/pilots cannot understand English well. Hence, language courses might be given to VTSOs to help them better understand the languages normally used by masters/pilots.
Consistent with V103, this study indicated the importance of information processing and pointed out the specific tasks that require information capacity. For example, it is reported that too much information is required in verifying vessel identity, as shown in Table 3. Based on this, a test for verifying vessel identity can be developed to select or train candidates. Moreover, the participants in this study, who are experienced VTSOs, pointed out that information overload was still serious in VTS (as shown in Table 6). As discussed in Section 4.3, rearranging vessel information to reduce information overlap and redesigning the vessel alarm system to manage the amount of warnings might be helpful.
The medical/physical requirement is a critical part of selecting VTS personnel. According to the analysis in Section 4.2, VTS personnel have a high chance of suffering from physical fatigue, such as strained eyes and stiff neck. Hence, candidates should not have optical problems and spine-related problems. Moreover, some training courses on how to maintain the body and restore these physical problems might be conducted.
Personal attributes assessment is the last, but important, step in selecting VTS personnel. Normally, a sense of responsibility and willingness to cooperate with others is considered in existing recruiting procedures. Besides these teamwork-related attributes, this study pointed out the importance of subjective feelings, which induce motivational and emotional fatigue, as shown in \tref{Tables}{tab5} and \tref{\!}{tab6}. Based on the fatigue symptoms and causal factors identified, a person who can endure monotonous tasks and prolonged monitoring might be more suitable for VTS operation.
There are strict procedures worked out for recruiting and training VTS personnel (IALA, 2009). Nevertheless, some specific problems would occur in particular situations. Therefore, this study provides a task-related method to identify and evaluate the specific causal factors of human fatigue in VTS. The results are promising and valuable to help with the improvements in recruiting and training VTS personnel in real practice.
5. CONCLUSION
It is desirable to identify the task-related causal factors of human fatigue and to design corresponding preventive interventions to manage human fatigue subsequently. Based on this understanding, the authors conducted this study to identify the key causal factors of human fatigue in VTS. This work has the following contributions:
• A novel definition of human fatigue. The definition points out the multidimensional consequences of human fatigue and defines the scope of causes.
• A task-driven approach was proposed to systematically elicit causal factors in the human–system interactions and the underlying organisational factors. The proposed causal network-based evaluation method integrates three dimensions of judgements, and is feasible to be extended.
• Several key causal factors and symptoms were identified in VTS operations. It is found that VTSOs suffered from more serious physical fatigue than cognitive fatigue. Moreover, the factors and symptoms identified can provide references and guidelines for recruiting VTS personnel and properly designing required training and rest activities.
This study provides insightful guidance for mitigating human fatigue to safety managers and lays the foundation for further explorations of context-aware safety management. Due to the limited data, this study mainly identifies key factors from the empirical view of VTSOs, while more precise objective measurements will be considered, and the fatigue model will be generated correspondingly in the future as well. Hence, the authors would like to recommend two potential future research directions: (1) Evaluate the importance of a factor by the frequency of its corresponding task. If an operator always conducts a task, he/she may be frequently stimulated by a specific factor. (2) Evaluate the importance of a factor by its consequences. The symptoms of human fatigue heavily depend on their causes, so it is worthwhile to consider the results of causal factors in evaluation.
ACKNOWLEDGEMENTS
This work was supported by Singapore Maritime Institute Research Project (SMI-2014-MA-06). The authors would like to thank all VTSOs who participated in this study.