Introduction
Knowledge exploration refers to the nonlocal search of new knowledge across technological or organisational boundaries beyond the current expertise of an organisation (Rosenkopf & Nerkar, Reference Rosenkopf and Nerkar2001; Bierly, Damanpour, & Santoro, Reference Bierly, Damanpour and Santoro2009). It represents a process of knowledge transferred from one external source to the organisation. The role played by knowledge exploration in innovation has received considerable attention in research (e.g., Ahuja & Lampert, Reference Ahuja and Lampert2001; Gupta, Smith, & Shalley, Reference Gupta, Smith and Shalley2006; Harryson, Kliknaite, & Dudkowski, Reference Harryson, Kliknaite and Dudkowski2008; Bojica, del Mar Fuentes, & Gómez-Gras, Reference Bojica, del Mar Fuentes and Gómez-Gras2011; Choi, Lee, & Kim, Reference Choi, Lee and Kim2012; Piezunka & Dahlander, Reference Piezunka and Dahlander2015; Lopez-Vega, Tell, & Vanhaverbeke, Reference Lopez-Vega, Tell and Vanhaverbeke2016). Since innovation broadly denotes the generation and adoption of something novel and useful (Scott & Bruce, Reference Scott and Bruce1994; Crossan & Apaydin, Reference Crossan and Apaydin2010), unfamiliar knowledge obtained from exploration provides key inputs to innovation (Bojica, del Mar Fuentes, & Gómez-Gras, Reference Bojica, del Mar Fuentes and Gómez-Gras2011; Li, Maggitti, Smith, Tesluk, & Katila, Reference Li, Maggitti, Smith, Tesluk and Katila2013; Love, Roper, & Vahter, Reference Love, Roper and Vahter2014; Nicholas, Ledwith, Aloini, Martini, & Nosellas, Reference Nicholas, Ledwith, Aloini, Martini and Nosella2015). However, too much exploration could produce a diminishing return on innovation, because of the excessive effort expended to search for, acquire, and integrate unfamiliar knowledge into the firm (Ahuja & Lampert, Reference Ahuja and Lampert2001), as well as the saturation and redundancy of the potential innovations. Building on this logic, researchers have proposed and empirically verified a curvilinear (inverted U) relationship between knowledge exploration and innovation (Katila & Ahuja, Reference Katila and Ahuja2002; Nerkar, Reference Nerkar2003; Wu & Shanley, Reference Wu and Shanley2009).
To advance the understanding of the curvilinear relationship between exploration and innovation, it is necessary to study how the curve changes (i.e., the inflection point on the curve). While the exact position of an inflection point will be context-specific (Pierce & Aguinis, Reference Pierce and Aguinis2013), developing a general theory of how this is determined can help to explain how exploration evolves and provide a guideline for managers to avoid wasting their efforts on exploration. Moreover, the various costs and benefits of exploration at different levels identified by different theories need to be pulled together to examine the origin of changes that cause a curvilinear relationship. Displaying the costs alongside the benefits forces a critical articulation of the theory that underpins the way in which costs and benefits change as the exploration advances.
These issues are studied by drawing on a recombinatory search framework that investigates organisational learning as a result of combining the new and old knowledge elements possessed by the firm (Ahuja, Lampert, & Tandon, Reference Ahuja, Lampert and Tandon2008). Since the framework focusses on knowledge elements and their combination, it is possible to study the inflection points and changes in costs and benefits of exploration at an elementary level. The logic of the recombinatory search framework enables the production of an inverse sigmoid (inverse-S) curve that is different from the current empirical results of an inverted U relationship between exploration and innovation. The more refined picture obtained by studying the exploration of knowledge at an elementary level contributes to the literature by highlighting the changes in the benefits and costs of exploration as the exploration increases, as well as providing a revised proposition of the relationship between exploration and innovation.
A Review On Research On Exploration And Innovation
According to the seminal work of March, exploration refers to ‘things captured by terms such as search, variation, risk taking, experimentation, play, flexibility, discovery, innovation’ (Reference March1991: 71). This is in contrast to exploitation, which corresponds to ‘such things as refinement, choice, production, efficiency, selection, implementation, execution’ (Reference March1991: 71). Exploration and exploitation have since then been studied as two ends of a uni-dimensional scale (e.g., Benner & Tushman, Reference Benner and Tushman2003; Sidhu, Commandeur, & Volberda, Reference Sidhu, Commandeur and Volberda2007; Raisch, Birkinshaw, Probst, & Tushman, Reference Raisch, Birkinshaw, Probst and Tushman2009). However, in the knowledge-based view and innovation literature, exploration and exploitation are often studied as orthogonal constructs that vary independently of each other (e.g., Katila & Ahuja, Reference Katila and Ahuja2002; He & Wong, Reference He and Wong2004; Gupta, Smith, & Shalley, Reference Gupta, Smith and Shalley2006; Soosay & Hyland, Reference Soosay and Hyland2008; Andriopoulos & Lewis, Reference Andriopoulos and Lewis2010) and they are differentiated by the boundaries of the knowledge search conducted by a firm. Knowledge exploitation is the search at the local area and within certain boundaries, whereas knowledge exploration refers to search activities that span distant organisational, technological (Rosenkopf & Nerkar, Reference Rosenkopf and Nerkar2001), and geographical boundaries (Hansen, Reference Hansen1999; Ahuja & Katila, Reference Ahuja and Katila2004), or a combination of some of those boundaries (Rosenkopf & Nerkar, Reference Rosenkopf and Nerkar2001). As such, a firm that undertakes a high level of exploration can engage in either high or low exploitation. This paper adopts the orthogonal approach and chooses to focus on exploration.
Table 1 summarises 21 selected empirical studies that examine the relationship between exploration and innovation published in key management journals (including Academy of Management Journal, Journal of Business Research, Journal of Management & Organization, Management Science, Organization Science, Research Policy, and Strategic Management Journal) since March (Reference March1991). The definition of knowledge exploration and innovation is diverse in the literature of innovation and knowledge management (e.g., Ahuja, Lampert, & Tandon, Reference Ahuja, Lampert and Tandon2008; Choi, Lee, & Kim, Reference Choi, Lee and Kim2012; Aloini & Martini, Reference Aloini and Martini2013; Shafique, Reference Shafique2013). Obviously, individual authors define and measure particular aspects of exploration and innovation to suit their own research questions and empirical settings. Papers that fit the broad definition of knowledge exploration and innovation were included in the review. Although this present review is not exhaustive, it provides a good coverage of the research on exploration and innovation.
Table 1 Literature review of empirical studies on the knowledge exploration–innovation relationships (in chronological order)

The majority of the 21 empirical studies listed in Table 1 focus on the benefits of exploration (e.g., Nerkar, Reference Nerkar2003; Sidhu, Commandeur, & Volberda, Reference Sidhu, Commandeur and Volberda2007; Fu, Diez, & Schiller, Reference Fu, Diez and Schiller2013). Knowledge exploration has been found to have a positive effect on both the output (Katila & Ahuja, Reference Katila and Ahuja2002; Wu & Shanley, Reference Wu and Shanley2009; Agarwal & Selen, Reference Agarwal and Selen2013; Fu, Diez, & Schiller, Reference Fu, Diez and Schiller2013; Li et al., Reference Li, Maggitti, Smith, Tesluk and Katila2013; Kim & Lui, Reference Kim and Lui2015) and performance (Ahuja & Lampert, Reference Ahuja and Lampert2001; Phene, Fladmoe-Lindquist, & Marsh, Reference Phene, Fladmoe-Lindquist and Marsh2006; Sidhu, Commandeur, & Volberda, Reference Sidhu, Commandeur and Volberda2007; Choi, Lee, & Kim, Reference Choi, Lee and Kim2012; Love, Roper, & Vahter, Reference Love, Roper and Vahter2014) of innovation. The knowledge-based view (Grant, Reference Grant1996) and organisational learning theory (March, Reference March1991) are often used to explain the relationship between the exploration and innovation of knowledge. The knowledge-based view suggests that knowledge exploration is the search for new knowledge that is distant from existing knowledge across various organisational, technological, and spatial boundaries (Rosenkopf & Nerkar, Reference Rosenkopf and Nerkar2001; Sidhu, Commandeur, & Volberda, Reference Sidhu, Commandeur and Volberda2007; Bojica, del Mar Fuentes, & Gómez-Gras, Reference Bojica, del Mar Fuentes and Gómez-Gras2011; Li et al., Reference Li, Maggitti, Smith, Tesluk and Katila2013), while the organisational learning theory perceives exploratory knowledge to be learning behaviour (Wadhwa & Kotha, Reference Wadhwa and Kotha2006). Learning takes place when knowledge external to an organisation is assimilated and internalised for use (Argote & Ingram, Reference Argote and Ingram2000). When integrating these two theories, knowledge exploration involves both a search and transfer process (Hansen, Reference Hansen1999). Innovation increases as a result of learning activities generated from knowledge exploration.
Building upon the generally positive relationship between exploration and innovation, some studies have identified several moderators that could potentially modify the learning process between exploration and innovation (e.g., McGrath, Reference McGrath2001; Laursen & Salter, Reference Laursen and Salter2006; Sidhu, Commandeur, & Volberda, Reference Sidhu, Commandeur and Volberda2007; Laursen, Masciarelli, & Prencipe, Reference Laursen, Masciarelli and Prencipe2012; Zhou & Li, Reference Zhou and Li2012; Li et al., Reference Li, Maggitti, Smith, Tesluk and Katila2013; Huang, Rice, & Martin, Reference Huang, Rice and Martin2015; Frankort, Reference Frankort2016; Isaksson, Simeth, & Seifert, Reference Isaksson, Simeth and Seifert2016). For instance, McGrath (Reference McGrath2001) focussed on the role of managerial oversight in supporting variance-enhancing learning during exploration, and found that goal autonomy and supervision autonomy enhance the positive relationship between exploration and innovation in new business development projects. Besides, Laursen and Salter (Reference Laursen and Salter2006) revealed that intensive Research and Development (R&D) substitutes the effect of a broad and in-depth exploration on innovation because intensive R&D represents an internal research effort that can compete with an external exploration for resources and managerial attention. In addition, Sidhu, Commandeur, and Volberda (Reference Sidhu, Commandeur and Volberda2007) suggested that the outcome of exploration is contingent on the nature of the environment, since this dictates the nature of the innovation and search process. They found that a dynamic environment enhances the influence of a supply-side exploratory search on innovation, but reduces the influence of a demand-side exploratory search. Laursen, Masciarelli, and Prencipe (Reference Laursen, Masciarelli and Prencipe2012) also showed that social capital at the regional level moderates the impact of external knowledge acquisition on innovation of the firms in the region; a region of high level of social capital tends to enhance the contributions of externally acquired R&D to the likelihood of the firms to innovate. Furthermore, Zhou and Li (Reference Zhou and Li2012) proposed that exploration as knowledge acquisition interacts with the depth of a firm’s knowledge base to increase radical innovation. This is because exploration expands the potential of the rich experience and know-how that resides within a firm’s knowledge base. Additionally, Li et al. (Reference Li, Maggitti, Smith, Tesluk and Katila2013) revealed that the intensity of the search moderates the impact of search selection for the introduction of new products. This is because the effort expended on the search negatively moderates the relationship between the distant search selection and the new product introduction, and the effort expended on the search positively moderates the relationship between diverse search selection and new product introduction, while persistence has a negative effect. Moreover, Huang, Rice, and Martin (Reference Huang, Rice and Martin2015) found that the impact of interfirm networking on innovation performance is negatively moderated by firm size, in that only small and medium-sized enterprises (rather than large firms) benefit from interfirm networking in innovation. In the buyer–supplier partnership, Isaksson, Simeth, and Seifert (Reference Isaksson, Simeth and Seifert2016) also showed that relationship duration positively moderates the effect of knowledge exploration from buyer technological innovation on supplier technological innovation. Finally, Frankort (Reference Frankort2016) uncovered that the positive influence of the knowledge acquisition from alliances on new product development is moderated positively by technological relatedness and negatively by product-market competition.
Yet, other studies have found a nonlinear relationship between exploration and innovation by examining the costs of knowledge exploration (Katila & Ahuja, Reference Katila and Ahuja2002; Nerkar, Reference Nerkar2003; Laursen & Salter, Reference Laursen and Salter2006; Phene, Fladmoe-Lindquist, & Marsh, Reference Phene, Fladmoe-Lindquist and Marsh2006; Wu & Shanley, Reference Wu and Shanley2009; Roper & Hewitt-Dundas, Reference Roper and Hewitt-Dundas2016). It has been argued that high levels of exploration will be negatively related to innovation due to the associated costs of searching, communication, integration, and coordination (e.g., Ahuja & Lampert, Reference Ahuja and Lampert2001; Ahuja & Katila, Reference Ahuja and Katila2004; Phene, Fladmoe-Lindquist, & Marsh, Reference Phene, Fladmoe-Lindquist and Marsh2006). Considering both the benefits and costs of exploration, this line of research suggests an inverted U relationship (e.g., Laursen & Salter, Reference Laursen and Salter2006; Roper & Hewitt-Dundas, Reference Roper and Hewitt-Dundas2016) in which the costs of exploration will become significant when the benefits of exploration on innovation reach a maximum level and innovation will be reduced.
There are also some studies that adopt the complexity theory (Waldrop, Reference Waldrop1992; Kauffman, Reference Kauffman1995; Levinthal, Reference Levinthal1997; Anderson, Reference Anderson1999) to analyze the relationship between knowledge exploration and innovation. For instance, Fleming and Sorenson (Reference Fleming and Sorenson2001) built on complex adaptive systems theory and applied Kauffman’s (Reference Kauffman1993) NK model to explore how the number of knowledge acquired (N) and the interdependence among them (K) affect the usefulness of the invention. Their major findings include a positive effect (yet at a diminishing rate) of the number of knowledge exploration on invention usefulness, and an inverted U-shaped relationship between the interdependence and the invention impact partially due to the complexity catastrophe.
In sum, the above review shows that empirical evidence has so far concluded that there is a generally positive relationship between knowledge exploration and innovation, with research on moderators and nonlinearity providing some qualifications to the relationship. Moreover, researchers have often adopted a costs and benefits framework, either implicitly or explicitly, to formulate their predictions. Research that focusses on the benefits of exploration concludes that exploration has a positive effect on innovation and moderators are tested to determine how they may reduce the benefits. Finally, research that focusses on the cost of exploration points to an inverted U-shaped effect of exploration, as costs often creep up with higher levels of exploration. The various costs and benefits of exploration identified in past research need to be simultaneously considered in order to provide a conclusive answer to an important question: How much exploration is most conducive to firm innovation?
Theoretical Framework
An integrated recombinatory search framework
This paper adopts a recombinatory search framework of innovation as the analytical framework to examine the underlying processes that link knowledge exploration and innovation. A recombinatory search framework was an implicit premise for a large volume of innovation research (e.g., Henderson & Clark, Reference Henderson and Clark1990; Nerkar, Reference Nerkar2003; Yayavaram & Ahuja, Reference Yayavaram and Ahuja2008; Cecere, Reference Cecere2015; see a review in Ahuja, Lampert, & Tandon, Reference Ahuja, Lampert and Tandon2008), and is consistent with complexity theory of organisations (Anderson, Reference Anderson1999; Fleming & Sorenson, Reference Fleming and Sorenson2001). The two core propositions of this framework are (i) new invention is a function of the recombination of (old and/or new) knowledge elements, and (ii) more valuable recombinations of knowledge elements lead to a higher level of innovation (Schumpeter, Reference Schumpeter1950; Nelson & Winter, Reference Nelson and Winter1982; Kogut & Zander, Reference Kogut and Zander1992; Nahapiet & Ghoshal, Reference Nahapiet and Ghoshal1998; Fleming & Sorenson, Reference Fleming and Sorenson2001).
Some core micro-level concepts of the framework, including knowledge elements, knowledge combination, and knowledge recombining, delineate the underlying process of macro-level learning and innovation. Empirical studies often operationalise knowledge elements as patents; thus, the number of knowledge elements of an organisation is the total number of patents it uses to develop new patents (e.g., Rosenkopf & Nerkar, Reference Rosenkopf and Nerkar2001; Ahuja & Katila, Reference Ahuja and Katila2004; Chatterji & Fabrizio, Reference Chatterji and Fabrizio2014; Wang, Rodan, Fruin, & Xu, Reference Wang, Rodan, Fruin and Xu2014; Isaksson, Simeth, & Seifert, Reference Isaksson, Simeth and Seifert2016). Knowledge elements can be external to the organisation (Ahuja & Lampert, Reference Ahuja and Lampert2001; Laursen & Salter, Reference Laursen and Salter2014; Operti & Carnabuci, Reference Operti and Carnabuci2014), and in this sense, its knowledge repertoire can be expanded by the incorporation of new knowledge elements from external sources, that is, knowledge exploration. Either all or part of these new elements can be linked and used to form various combinations of knowledge to produce innovation.
An example of innovation as a recombinatory search of knowledge elements is innovative cuisine (as an innovation of a restaurant) as a consequence of the knowledge of food ingredients (as knowledge elements) being linked or recombined by cooking techniques and methods (as new combination of knowledge elements) (Messeni & Savino, Reference Messeni and Savino2014). Another example is a nuclear-powered ship, which can be viewed as an invention integrating knowledge of boats and nuclear power engineering (cf., Fleming & Sorenson, Reference Fleming and Sorenson2001). A final example is new patent application by a firm, which is usually results of synergising multiple old and new patents and other scientific knowledge, some of which can be obtained from external sources (Ahuja & Katila, Reference Ahuja and Katila2004; Yayavaram & Chen, Reference Yayavaram and Chen2015).
The way of combining or recombining constituent knowledge elements also plays a critical role in the recombinatory search framework. Using the same set of core knowledge elements, a new architecture or novel way of recombining can produce an architectural innovation (Henderson & Clark, Reference Henderson and Clark1990).
Based on the recombinatory search framework of innovation, two benefits of knowledge exploration are articulated below, namely knowledge repertoire enlargement and knowledge variety enhancement, as well as two costs of knowledge exploration, namely knowledge acquisition costs and knowledge integration costs. The respective changes of these benefits and costs are then highlighted across various levels of exploration. Integrating the change patterns of benefits and costs produces a proposition of the inverse S-curve relationship between knowledge exploration and innovation. The core of the argument is summarised in Figure 1.
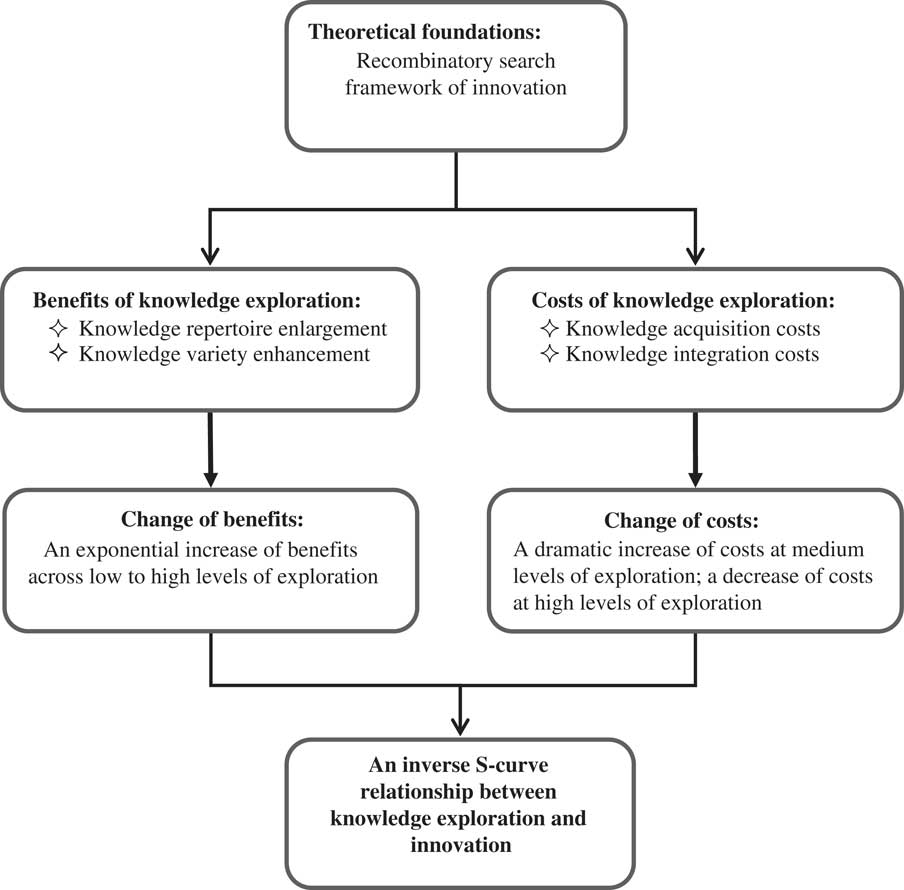
Figure 1 Core arguments for an inverse S-curve relationship between knowledge exploration and innovation
Benefits of knowledge exploration
According to the recombinatory search framework, knowledge exploration introduces new and nonlocal knowledge elements into the organisation’s existing knowledge repertoire. Based on existing research, knowledge exploration provides two major benefits to innovation, namely: knowledge repertoire enlargement (Ahuja & Katila, Reference Ahuja and Katila2004; Wu & Shanley, Reference Wu and Shanley2009) and knowledge variety enhancement (Ahuja & Lampert, Reference Ahuja and Lampert2001; Sidhu, Commandeur, & Volberda, Reference Sidhu, Commandeur and Volberda2007; Wu & Shanley, Reference Wu and Shanley2009).
Knowledge repertoire enlargement refers to an increase in the absolute size of the knowledge stock (Ahuja & Katila, Reference Ahuja and Katila2001). Knowledge exploration imports new knowledge elements into an organisation, which enlarges its knowledge repertoire (Huang, Rice, & Martin, Reference Huang, Rice and Martin2015; Frankort, Reference Frankort2016). A larger knowledge repertoire enables the organisation to have a better overview of the knowledge elements for innovation purposes and makes it more capable of identifying innovation opportunities. For instance, the technological capability is strengthened by accessing to more external knowledge sources (Zheng, Li, & Wu, Reference Zheng, Li and Wu2013). Thus, it leads to a greater likelihood of innovation.
Knowledge variety enhancement relates to an increase in the heterogeneity of knowledge elements. The introduction of new knowledge by exploring knowledge distant from the organisation’s current knowledge domain imports knowledge that is heterogeneous from the existing knowledge base. A variety of knowledge is vital for innovation because it enables a greater possibility of discovering new products or services suitable for the changing environment (McGrath, Reference McGrath2001; Shafique, Reference Shafique2013). Thus, an organisation that possesses a great variety of knowledge is more capable of adapting to the environment (McGrath, Reference McGrath2001; Bojica, del Mar Fuentes, & Gómez-Gras, Reference Bojica, del Mar Fuentes and Gómez-Gras2011; Rice, Liao, Martin, & Galvin, Reference Rice, Liao, Martin and Galvin2012). Although environmental change implies threats and risks, it also provides opportunities for innovation, and a great variety of knowledge enables organisations to capture these opportunities.
Moreover, nonfamiliarity in distant knowledge enables more promising and valuable combinations of knowledge elements than local knowledge (Sidhu, Commandeur, & Volberda, Reference Sidhu, Commandeur and Volberda2007). For example, new knowledge may be the solution to a problem that is hindering product development, thus, the new product can emerge when this new knowledge is combined with existing knowledge. Hence, the amount of potential innovation increases as the level of knowledge exploration increases (Ahuja & Katila, Reference Ahuja and Katila2001).
The two benefits mentioned above are well documented in research. In a departure from existing research, the change of benefits will be examined in this paper. It is posited that the benefit of knowledge exploration for innovation increases exponentially because the possible number of valuable combinations increases at an accelerating rate as knowledge exploration increases. In other words, the possible combinations of knowledge elements increase exponentially as the number of knowledge elements increase algebraically (cf., Fleming & Sorenson, Reference Fleming and Sorenson2001; Yayavaram & Ahuja, Reference Yayavaram and Ahuja2008), and this can be illustrated by the mathematical combination in the example below (a similar but more simple example can be found in Ahuja & Katila, Reference Ahuja and Katila2001: 200). Assuming that an organisation initially possesses four knowledge elements, the maximum number of knowledge combinations with different orders of elements is 15 (including four single elements, six combinations of two elements, four combinations of three elements, and one combination of four elements). If it engages in exploration and adds two external knowledge elements to those it already possesses, it will increase the number of knowledge elements to six; however, the maximum knowledge combinations will increase to 63, more than four times the original combinations. Furthermore, by acquiring two more knowledge elements, the number of knowledge elements will increase to eight, and the maximum combinations will be 255, 17 times the 15 combinations of the original four knowledge elements. In this particular example of four knowledge elements, one unit increase in knowledge elements by exploration leads to a 16-fold increase in knowledge combinations. Although not all possible knowledge combinations will be useful for innovation (cf., Ahuja & Lampert, Reference Ahuja and Lampert2001; Yayavaram & Ahuja, Reference Yayavaram and Ahuja2008), the exponential nature of the benefits of exploration to innovation is evident.
Proposition 1: The benefit of knowledge exploration on innovation increases gradually at low-to-medium levels of exploration, and exponentially at medium-to-high levels of exploration.
Costs of knowledge exploration
Knowledge exploration requires organisations to provide extra resources and supporting mechanisms (March, Reference March1991; Katila & Ahuja, Reference Katila and Ahuja2002). Based on the recombinatory search framework, the transformation of exploration into innovation involves two steps, namely knowledge acquisition and knowledge integration (Hansen, Reference Hansen1999). Existing research suggests that this process will involve two major types of costs, namely knowledge acquisition costs and knowledge integration costs (Sidhu, Commandeur, & Volberda, Reference Sidhu, Commandeur and Volberda2007). Contrary to previous research that assumes that these costs will generally increase as knowledge exploration rises, their various levels are examined in this paper as the amount of knowledge exploration increases.
Knowledge acquisition costs include the financial expenses and cognitive attention spent in searching and acquiring new knowledge beyond the boundary of the organisation (e.g., Laursen & Salter, Reference Laursen and Salter2006; Sidhu, Commandeur, & Volberda, Reference Sidhu, Commandeur and Volberda2007; Wu & Shanley, Reference Wu and Shanley2009; Piezunka & Dahlander, Reference Piezunka and Dahlander2015). For instance, Nicholas et al. (Reference Nicholas, Ledwith, Aloini, Martini and Nosella2015) proposed a measure of idea acquisition that includes five search activities: market awareness, idea management, customer involvement, open environment, and internal networking. Previous research assumes that acquisition costs increase linearly with exploration (e.g., Sidhu, Commandeur, & Volberda, Reference Sidhu, Commandeur and Volberda2007; Wu & Shanley, Reference Wu and Shanley2009). However, as indicated by a recombinatory search framework, this paper argues that this cost will increase initially, but decrease with high levels of exploration for two reasons. First, after accumulating experience during a learning curve (Epple, Argote, & Devadas, Reference Epple, Argote and Devadas1991), an organisation knows what to look for so that the amount of trial and error is reduced with high levels of exploration, and this reduces the cost of cognitive attention (Choi, Lee, & Kim, Reference Choi, Lee and Kim2012). Second, at high levels of exploration, the most suitable knowledge would have already been acquired and the pool of potentially useful new knowledge will be reduced. Paradoxically, an organisation has fewer external knowledge elements to choose from at high levels of exploration than at medium levels; therefore, the confusion and information overload of managers (Ahuja & Lampert, Reference Ahuja and Lampert2001) will be relieved. This leads to the following proposition:
Proposition 2: The cost of knowledge acquisition increases at low-to-medium levels of exploration, but decreases at high levels of exploration.
Knowledge integration costs are organisational expenses and efforts to integrate knowledge elements in order to yield new inventions (e.g., Ahuja & Katila, Reference Ahuja and Katila2001; Katila & Ahuja, Reference Katila and Ahuja2002; Sidhu, Commandeur, & Volberda, Reference Sidhu, Commandeur and Volberda2007). New knowledge acquired from exploration needs to be first integrated with existing knowledge or organised in a proper way prior to producing a new knowledge combination that is commercially valuable, that is, new innovation (Henderson & Clark, Reference Henderson and Clark1990; Fleming & Sorenson, Reference Fleming and Sorenson2001). For instance, the knowledge integration process will require complex headquarters and subsidiary coordination in multinational firms (Lin & Chen, Reference Lin and Chen2015). It is complex and costly and different integration costs may be generated at different levels of exploration.
When the number of new knowledge elements first begins to increase because of exploration activities, the complexity of an organisation’s knowledge base also increases, and this complexity raises the integration costs; thus, knowledge exploration increases integration costs at the beginning. Katila and Ahuja (Reference Katila and Ahuja2002) suggest that the growing complexity of knowledge stock increases the cost of integrating new knowledge into the firm’s original knowledge base. Theoretically, the number of all possible combinations of knowledge elements in the organisation’s knowledge repertoire for experimentation will rise dramatically even if the new knowledge elements only grow gradually (Fleming & Sorenson, Reference Fleming and Sorenson2001; Yayavaram & Ahuja, Reference Yayavaram and Ahuja2008). Therefore, knowledge integration costs tend to increase proportionally as knowledge exploration increases, as reported in the literature (e.g., Ahuja & Lampert, Reference Ahuja and Lampert2001; Katila & Ahuja, Reference Katila and Ahuja2002; Ahuja & Katila, Reference Ahuja and Katila2004; Phene, Fladmoe-Lindquist, & Marsh, Reference Phene, Fladmoe-Lindquist and Marsh2006; Sidhu, Commandeur, & Volberda, Reference Sidhu, Commandeur and Volberda2007; Wu & Shanley, Reference Wu and Shanley2009).
Contrary to existing research, this paper argues that integration costs will decline when knowledge exploration reaches a high level because of the increase in knowledge relatedness (Ahuja & Katila, Reference Ahuja and Katila2001) among knowledge elements and a new knowledge integration pattern. First, when knowledge exploration increases to a high level, the relatedness among existing and new knowledge elements tends to rise. While new knowledge elements may often be disparate from each other when the exploration is at a moderate level, more newly acquired knowledge as the exploration increases to a high level can amplify the chance of linking previously unconnected knowledge elements in the expanded knowledge repertoire (Fleming & Sorenson, Reference Fleming and Sorenson2001; Breschi, Lissoni, & Malerba, Reference Breschi, Lissoni and Malerba2003). The search for recombinant inventions, partially based on these interdependent knowledge elements, narrows the range of knowledge integration (Yayavaram & Ahuja, Reference Yayavaram and Ahuja2008) and facilitates sense-making, subsequently lowering the total integration costs (Kogut & Zander, Reference Kogut and Zander1992; Grant, Reference Grant1996; Ahuja & Katila, Reference Ahuja and Katila2001).
Second, a new knowledge integration pattern occurs at high levels of exploration that tends to reduce integration costs. When an organisation engages in an extensive knowledge exploration, its new knowledge elements will form a rich pool. The external knowledge elements can reach such a high level that they can generate innovation on their own without the need to integrate with old knowledge elements. These new innovations, which are mainly constituted of newly gained knowledge elements, are consistent with the exploratory innovation engendered by an extensive exploratory, nonlocal knowledge search, or a ‘long jump’ of organisational learning onto a distant technological trajectory (Levinthal, Reference Levinthal1997; Fleming & Sorenson, Reference Fleming and Sorenson2001). In this sense, the knowledge integration of these innovations occurs most often within the pool of new knowledge elements which, when recombined, represent only a portion of the organisation’s total knowledge stock. Therefore, there is less need for the organisation to evaluate every possible combination of existing and new knowledge to identify innovative configurations, reducing the total integration cost. This leads to the following proposition:
Proposition 3: The cost of knowledge integration increase at low-to-medium levels of exploration, but decrease at high levels of exploration.
An inverse S-curve proposition
The net effect of exploration on innovation is its benefits minus associated costs. Figure 2 shows how the difference between the benefits and costs discussed above result in an inverse S-shaped relationship between knowledge exploration and innovation. The lines of total benefit, knowledge integration cost, and knowledge acquisition cost in Figure 2 represent the arguments in the preceding section graphically. Each point on the total benefit line corresponds to the level of the total, rather than marginal, benefit of a specific level of knowledge exploration. The same applies to the lines of knowledge integration costs and knowledge acquisition costs. The net effect on innovation is the difference between the benefits and costs at corresponding levels of exploration.

Figure 2 Knowledge exploration and innovation: an inverse S-curve relationship
Corresponding to the theoretical arguments in the above sections, the dotted dashed line in Figure 2 illustrates the total recombinant benefits of exploration, which increase exponentially as exploration increases from low to high levels. Similarly, consistent with the conceptual articulation in this study, the smooth dotted line represents the total cost of exploration, which is the sum of knowledge acquisition costs and knowledge integration costs. As discussed in the last section, both knowledge acquisition costs and knowledge integration costs initially increase at an accelerating rate, and then decline when the exploration level is high. Taken together, the total cost of exploration initially rises dramatically and sequentially drops.
Based upon the lines of total benefits and total costs, it is possible to draw the bold solid line that represents the net gain from knowledge exploration in terms of innovation. The innovation outcome is the result of the total costs subtracted from the total benefits (cf., Lu & Beamish, Reference Lu and Beamish2004). Graphically, the net contribution of knowledge exploration to innovation is the distance between the lines of total benefits and total costs. The interaction between these two lines generates an overall inverse S curve that represents the relationship between exploration and innovation.
Summarising, at low levels of exploration, the increase in the recombinant benefit exceeds the increase in the total cost of knowledge acquisition and integration. This is because, on the one hand, the benefit rises dramatically to a high level even with a small increase in exploration due to the exponential increase in the benefit of exploration. On the other hand, knowledge acquisition and integration costs tend to be low at this stage because the amount of new knowledge is limited and is thus less expensive for the firm. Taken together, exploration at low levels tends to be positively associated with innovation.
In contrast, at medium levels of exploration, the increase in the total cost of exploration outstrips the increase in its benefit. The increasing acquisition costs and dramatically soaring integration costs together outweigh the increase in the recombinant benefits of knowledge exploration. Therefore, the medium levels of knowledge exploration have a negative effect on innovation overall.
Nevertheless, at high levels of exploration, a recombinatory search framework suggests that the difference between the benefits and costs of exploration will be enlarged because, in contrast to the benefit of exploration, which increases exponentially, the cost slows down or even declines. Therefore, the relationship between exploration and innovation will become positive again at high levels of exploration. This is similar to the findings of Brown and Eisenhardt’s (Reference Brown and Eisenhardt1997) case study that extreme experimentation (and thus knowledge gain) contributes to continuous product innovation in the computer industry.
The above inverse S-curve relationship can be succinctly illustrated with the three knowledge combination boxes at the bottom of Figure 2. According to current research, which proposes an inverted U relationship, initial exploration will be able to produce a few useful combinations (i.e., innovation) (five in the diagram), but innovation will decline to only a few when the level is medium (three in the second diagram). However, according to the recombinatory search framework adopted in this paper, there is a third stage: when exploration is high, the number of innovations might rise again (11 in the third diagram). Based on the above discussion, it is proposed that there is an inverse S-shaped relationship between organisational knowledge exploration and innovation, which leads to the following proposition:
Proposition 4: The relationship between knowledge exploration and innovation is an inverse S shape, with the slope positive at low levels of exploration, negative at medium levels of exploration, and becoming positive again at high levels of exploration.
Company Cases
As this study is exploratory and the above conceptual arguments are somewhat abstract, two company cases are now presented to illustrate these theoretical elaborations. These company cases are not meant to verify the theoretical propositions developed earlier. Instead, they serve an illustrative role in explaining the inverse S-curve relationship between knowledge exploration and innovation. Concrete examples make the abstract concepts and the relationships between them more accessible (Siggelkow, Reference Siggelkow2007). Hence, we follow the common practice of existing studies to offer real business case (e.g., Casadesus-Masanell & Ricart, Reference Casadesus-Masanell and Ricart2010; Demil & Lecocq, Reference Demil and Lecocq2010; Rosenkopf & McGrath, Reference Rosenkopf and McGrath2011).
Two innovative companies, IBM and Procter & Gamble, both have experience of conducting a wide range of knowledge exploration and can serve to provide an appropriate illustration of how innovation can change across a large spectrum of exploration. While study cases may contain selection bias, the real life scenarios of these two companies are expected to illustrate the relevance of the theoretical framework adopted by this study and provide some preliminary evidence.
IBM
In the early 1990s, IBM experienced a decline in its market share and financial performance and also struggled with new innovation. The largest annual loss ($5 billion) in its corporate history was disclosed in 1992 (Bhaskarabhatla & Hegde, Reference Bhaskarabhatla and Hegde2014). The company was labelled as ‘conservative’ and ‘regimented’ rather than innovative at that time Gerstner (Reference Gerstner2002: 181). Responding to these threats, IBM conducted a series of strategic changes under the leadership of Louis Gerstner since 1993, and its strategic renewal included some smart moves, such as withdrawing from nonpromising business, focussing on IT integration services, and rebuilding a culture that encouraged innovation (Gerstner, Reference Gerstner2002).
This phase can be seen as the first stage of exploration of the inverse S curve (see Figure 3). IBM reached out to its customers to understand their needs, especially new market trends. It not only gained market information from marketing consultancy agencies, but also communicated directly with current and potential customers (Meyer, Anzani, & Walsh, Reference Meyer, Anzani and Walsh2005a). Moreover, IBM also undertook knowledge exploration through its alliance networks (Dittrich, Duysters, & de Man, Reference Dittrich, Duysters and de Man2007). An increasing number of new exploratory alliances, such as those with Oracle and Microsoft, were added in IBM’s network to explore new technological fields, such as those related to the internet and middleware.

Figure 3 Case of IBM
Despite extensive knowledge exploration, IBM soon suffered losses in its innovation. For instance, the G1 mainframe created in 1994 was supposed to be a radical technological innovation, relative to IBM’s previous H6 mainframe. Despite its advantage of a small shape and other attributes, the G1 version only had less than a quarter of its predecessor’s processing power, a critical technical criterion. It took IBM 3 more years (i.e., until 1997) to further improve this G series to a G4 version to catch up with the processing power (Meyer, Anzani, & Walsh, Reference Meyer, Anzani and Walsh2005b). This period of decline in innovation can be viewed as the second stage of the inverse S curve.
However, IBM did not stop or slow down its knowledge exploration, but continued to invest in exploring new knowledge domains. This further exploration proved to be successful, especially in the strategic renewal of the information technology service business. This helped IBM to become a dominant player in the software and service industry and thus, regain its reputation as a leader in innovation. The strategic regeneration of IBM was largely attributable to its intensive and deliberate exploration, which illustrates the third stage of the inverse S curve.
The case of IBM’s strategic transformation to a leader in a new business field shows how continuous, extensive knowledge exploration may cause a loss of innovation at some point in time, but it can later contribute to a fundamental organisational adaptation and fruitful innovation. This demonstrates the evolution of the inverse S-curve relationship.
Procter & Gamble
The inverse S curve is also evident in the innovation practices of Procter & Gamble, one of the leading companies in the global consumer goods industry (see Figure 4). Procter & Gamble is well known for its continuous product innovation. Traditionally, its innovation projects were mainly conducted within the company by its R&D department, and this closed innovation model was seen to be successful. However, in the late 1990s, this method of developing new products gradually led to a low success rate of innovation. Its overall financial performance also suffered and was below the satisfactory level in 2000 (Drake, Sakkab, & Jonash, Reference Drake, Sakkab and Jonash2006). The company entered the second stage of exploration of the inverse S-curve relationship, where a medium level of exploration is negatively related to innovation.

Figure 4 Case of Procter & Gamble
Procter & Gamble strategically moved towards a new open innovation model in 2000 to overcome the decline in its innovation performance (Dodgson, Gann, & Salter, Reference Dodgson, Gann and Salter2006). It increasingly leveraged external knowledge and expertise in the process of developing new products (Huston & Sakkab, Reference Huston and Sakkab2006). The third stage of exploration was particularly evident in a new strategy, Connect and Develop, which involved extensive knowledge exploration. Connect and Develop was different from the traditional R&D in the sense that it endeavoured to develop new products and initiatives based on connecting the company with individuals and organisations that possessed valuable knowledge. Although internal resources and knowledge were still used, the majority of the weight was shifted to experts and knowledge outside Procter & Gamble (Huston & Sakkab, Reference Huston and Sakkab2006). For instance, in order to establish connections with a massive number of external experts, Procter & Gamble contacted more than two million individuals worldwide via email (Drake, Sakkab, & Jonash, Reference Drake, Sakkab and Jonash2006). In addition, the company also actively engaged with and absorbed new knowledge from a large number of external and formal institutions and networks. According to Huston and Sakkab, two vice-presidents of Procter & Gamble, ‘we look for ideas in government and private labs, as well as academic and other research institutions; we tap suppliers, retailers, competitors, development and trade partners, VC firms, and individual entrepreneurs’ Huston and Sakkab (Reference Huston and Sakkab2006: 63). The open external networks included NineSigma, InnoCentive, YourEncore, and Yet2.com, and the resources embedded in these large-scaled networks were well leveraged by Proctor & Gamble (Huston & Sakkab, Reference Huston and Sakkab2006). According to Huston and Sakkab (Reference Huston and Sakkab2006), the technological brief of the company had been circulated to >700,000 receivers by 2006 in order to obtain possible proposals and solutions. Besides, the company also sought technical solutions among 75,000 scientists and 800 retired scientists and engineers.
The extensive exploration at Procter & Gamble has increased innovative output. Procter & Gamble was able to double its innovation success rate in a more cost-effective manner. The proportion of new products with external originality increased from only 10% in 2000 (Drake, Sakkab, & Jonash, Reference Drake, Sakkab and Jonash2006) to over 35% in 2006 (Huston & Sakkab, Reference Huston and Sakkab2006). The Connect and Develop program of Procter & Gamble, with exponential knowledge elements and reduced cost, amply illustrates the third stage of the inverse S-curve proposition.
Discussion and Conclusion
In summary, this paper has explored how innovation varies as a function of knowledge exploration through the lens of a recombinatory search model. By examining the changes of costs and benefits as knowledge elements are acquired and combined to create innovation during exploration, an inverse S-curve relationship between knowledge exploration and innovation is tentatively proposed.
This paper contributes to research on knowledge exploration in three ways. First, by examining the inflection points along the curvilinear relationship between exploration and innovation, the inverted U curve is extended to an inverse S-curve proposition, which proposes a third stage of exploration, that is, innovation increases again when a firm engages in high levels of knowledge exploration. It is believed that the reasons research has so far only reported an inverted U curve rather than an inverse S relationship between exploration and innovation could be theoretical rather than empirical. As Kuhn (Reference Kuhn1970) explained in his classic text, scientific activities are often confined by and conducted within the prevailing theoretical paradigm that governs the scientific community (Pfeffer, Reference Pfeffer1993; Starbuck, Reference Starbuck2006). Researchers look for and stop at an inverted U hypothesis. An alternative three-sequential-stage proposition of knowledge exploration is proposed in this paper as an alternative to the well-received inverted U curve hypothesis by articulating the theory that underpins exploration.
Second, knowledge elements are identified as the fundamental building blocks underlying the various costs and benefits of knowledge exploration. The recombination of knowledge elements highlights the effect of various costs and benefits on innovation. The analysis at the knowledge element level is different from the existing innovation research, which attempts to identify the higher level industries, firms, and managerial determinants of innovation (e.g., Hauser, Tellis, & Griffin, Reference Hauser, Tellis and Griffin2006; Ahuja, Lampert, & Tandon, Reference Ahuja, Lampert and Tandon2008; Crossan & Apaydin, Reference Crossan and Apaydin2010). The focus on knowledge elements provides a novel analysis to explain the causal mechanism between exploration and innovation (Ahuja, Lampert, & Tandon, Reference Ahuja, Lampert and Tandon2008) and could be a useful lens through which to examine relationships related to knowledge in general.
Third, by focussing only on exploration but not exploitation, this paper adds to the body of research that studies knowledge exploration on its own (e.g., Katila & Ahuja, Reference Katila and Ahuja2002; Wu & Shanley, Reference Wu and Shanley2009; Rosenkopf & McGrath, Reference Rosenkopf and McGrath2011). An orthogonal approach towards exploration and exploitation suggests that these two concepts are not necessarily related to each other and should be studied independently. This approach is distinctive from the original uni-dimensional approach that March (Reference March1991) proposed more than 20 years ago. Based on an orthogonal approach, exploration has been studied in its own right in this paper in order to better understand its role in innovation.
This paper also offers some practical advice to managers. It challenges the widely held wisdom of an inverted U curve relationship between knowledge exploration and innovation, which suggests that a firm should conduct medium levels of knowledge exploration and not explore new knowledge beyond that. In contrast, the inverse S-curve relationship found in this paper implies that firms can actually gain more innovation from continuously seeking to explore more knowledge. The third stage yields the highest return of knowledge exploration. Provided the inverse S-curve hypothesis gains empirical support, the revised advice to managers would be as follows: after gaining some benefit from knowledge exploration in Stage 1, quickly get through the net negative effects of Stage 2 and move into the net positive zone of Stage 3. The last stage is the final target for companies that are built on knowledge. In a similar vein, a case study of a highly successful Korean internet company by Lee, Rho, Kim, & Jun (Reference Lee, Rho, Kim and Jun2007) illustrates how the zealous pursuit of knowledge exploration could lead to frequent and important innovation. Aloini and Martini (Reference Aloini and Martini2013) adopted a how-to-search approach to investigate the various search practices that enable exploration of Italian Hi-tech firms.
In addition, the results of this paper suggest that a unique knowledge exploration strategy should be developed to suit various levels of knowledge exploration. When exploration activities are at both low and high levels, managers can be more aggressive in their exploration activities, since the benefits of exploration outweigh the costs. However, when exploration is at a medium level, managers should cautiously control the costs, which are highest in the process, and proceed to the third stage of exploration as quickly as possible.
Some guidelines for empirical testing
To empirically verify the inverse S-curve proposition put forward in this paper, empirical researchers should take note of some methodological issues in order to ensure the rigour of the research design. An appropriate measure of key variables, including innovation, knowledge exploration, benefits, and costs, should be designed. Innovation may be measured as the number of patents a firm applies or is issued (e.g., Ahuja & Katila, Reference Ahuja and Katila2001; Berry, Reference Berry2014; Bhaskarabhatla & Hegde, Reference Bhaskarabhatla and Hegde2014), and exploration as the number of external patents cited by the firm’s applied patents (e.g., Phene, Fladmoe-Lindquist & Marsh, Reference Phene, Fladmoe-Lindquist and Marsh2006; Choi, Lee, & Kim, Reference Choi, Lee and Kim2012; Kim, Arthurs, Sahaym & Cullen, Reference Kim, Arthurs, Sahaym and Cullen2013; Yoon, Lee, & Song, Reference Yoon, Lee and Song2015). Acquisition and integration costs can be measured in terms of the organisational resources spent and attention paid to acquiring and integrating knowledge elements (e.g., Aloini & Martini, Reference Aloini and Martini2013; Nicholas et al., Reference Nicholas, Ledwith, Aloini, Martini and Nosella2015). Moreover, the empirical testing should include a cubic term of exploration in the estimation model of innovation as the dependent variable (cf., Contractor, Kundu, & Hsu, Reference Contractor, Kundu and Hsu2003; Lu & Beamish, Reference Lu and Beamish2004; Hashai, Reference Hashai2014). The coefficient of this cubic term would be expected to be statistically significant and positive to find evidence of an inverse S-curve relationship. Furthermore, a large sample is preferred when testing a complex relationship such as the inverse S-curve model. Since the effect of the size of the curvilinear term tends to be small, the power of empirical testing should be high to detect such a relatively small effect (Pierce & Aguinis, Reference Pierce and Aguinis2013). Finally, the sample needs to cover a wide range of knowledge exploration. The theoretical proposition of this paper is concerned with the implication of knowledge exploration on innovation across a wide range, from low, medium, to high; hence, the empirical data of knowledge exploration should cover a broad range to capture the complete relationship (cf., Pierce & Aguinis, Reference Pierce and Aguinis2013).
Limitations
One limitation of this paper is that it has not differentiated the type and radicalness of the innovation (Cabello-Medina, Carmona-Lavado, & Valle-Cabrera, Reference Cabello-Medina, Carmona-Lavado and Valle-Cabrera2006; Garriga, von Krogh, & Spaeth, Reference Garriga, von Krogh and Spaeth2013; Cheng & Shiu, Reference Cheng and Shiu2015), which may mitigate the relationship between knowledge exploration and innovation. For instance, an increase in innovation at a high level of knowledge exploration may be more likely to apply to radical than incremental innovation. Therefore, the examination of the boundary conditions of the theoretical arguments may further advance an understanding of the relationship between exploration and innovation. Another limitation of this paper is that knowledge exploitation is not discussed. We argue that knowledge exploitation and exploration are two orthogonal concepts for innovation and should be separately studied, and exploration is more important than exploitation for innovation. The ambidexterity concept of simultaneously managing exploration and exploitation (Soosay & Hyland, Reference Soosay and Hyland2008; Lin & Chen, Reference Lin and Chen2015) would point to an important qualifier to the inverse S proposition. Finally, future research may further take complexity theory as (one of) the theoretical lens to examine the role of knowledge exploration in innovation. Recombination as the theoretical foundation of this paper is also a major element of complexity theory (Anderson, Reference Anderson1999). Existing literature (e.g., Fleming & Sorenson, Reference Fleming and Sorenson2001) has also applied the idea of interdependence among elements into the exploration–innovation discussion. It may also be fruitful to integrate other key theoretical building blocks of complexity theory such as agents, equifinality, self-organisation, and edge of chaos.
Nevertheless, based on a recombinatory search framework, this paper discusses how the costs and benefits of knowledge exploration are arrayed systematically along different levels of exploration and how macro-level outcomes can be explained by micro-level activities. Thus, this paper adds to the current understanding of organisational learning and innovation. Although the S-curve proposition is conceptual and exploratory in nature, it is hoped that this paper can serve as a starting point for a more detailed investigation of the relationship between knowledge exploration and innovation.
Acknowledgements
The authors would like to thank the Associate Editor David Tappin and two anonymous reviewers for their valuable comments. This research was supported under the Australian Research Council’s Discovery Project Funding Scheme (#DP110100880) awarded to the second author, and the start-up research fund of School of Labor and Human Resources, Renmin University of China.