1. Introduction
The commons is a general term used to refer to a shared resource in which each competing stakeholder has an equal interest in a given resource (Hardin, Reference Hardin1968). Because the term is often conflated with ‘open access commons’, researchers in this area typically refer to common pool resource (CPR) systems that can have a variety of access permissions (Frischmann et al., Reference Frischmann, Marciano and Ramello2019). CPRs are natural or man-made resources shared among different users. These resources are defined by two main features: (i) they are sufficiently large so that it is costly to exclude potential beneficiaries from using them, and (ii) they are characterized by a high degree of subtractability, or rivalry of consumption (Ostrom, Reference Ostrom1990, Reference Ostrom2009). We can find a wide range of examples of goods defined as commons, which have been widely explored in the CPR literature: fisheries, forests, innovations, online communities, hacker communities, etc. (Cox et al., Reference Cox, Arnold and Tomás2010; Dietz et al., Reference Dietz, Ostrom and Stern2003; Harris, Reference Harris2018; Potts, Reference Potts2018; Safner, Reference Safner2016; Williams and Hall, Reference Williams and Hall2015). A less widely known example of a CPR system is the exploitation of electromagnetic spectrum bands for wireless communications.
As Staple and Werbach (Reference Staple and Werbach2004) explain, radio waves do not pass through an ethereal medium called ‘spectrum’. Rather, these radio waves are the medium. Placing spectrum within the CPR context, spectrum bands have a subtractability feature, given that if a user transmits using an allocated band, its transmissions add to the noise level for all other users in the same band. These increases in noise decrease the available channel capacity, acting as a ‘foe’ to other signals transmitted in the same band (Dytso et al., Reference Dytso, Tuninetti and Devroye2016; Lee Reference Lee1990). Inevitably, the band may reach a point in which it becomes unsuitable for any additional wireless communications in the same frequency, space, and/or time (i.e. a spectrum band). As to the excludability characteristic of CPRs, it is relatively difficult to exclude an arbitrary user from most regions of the radio-electric spectrum. Technologies that exploit spectrum bands have made it difficult, complex, and costly to do so. For example, it would be a very complex and costly task to exclude any given user from transmitting and receiving wireless signals using a Bluetooth and/or Wi-Fi transceiver (Punnoose et al., Reference Punnoose, Tseng and Stancil2001). Consequently, based on the common good features defined by Elinor Ostrom (Reference Ostrom1997, Reference Ostrom2009, Reference Ostrom2010), we concur with the previous research (Bustamante et al., Reference Bustamante, Gomez, Weiss and Rose2018; Henrich-Franke, Reference Henrich-Franke2011; Herter, Reference Herter1985; Weiss et al., Reference Weiss, Lehr, Acker and Gomez2015) that the exploitation of radio-electric spectrum bands for wireless transmissions is consistent with the definition of a CPR.
In contrast to the case of CPRs, which situates enforcement as part of the governance structure and incorporates it into the definition of rules, the most common governance mechanism for regulating the exploitation of spectrum bands in the United States has been centralized specification and enforcement of property rights. Usually, a government agency such as the Federal Communications Commission (FCC) or the National Telecommunications and Information Agency (NTIA) requires or prohibits specific actions or technologies. Rule-breakers are subject to fines, sanctions, and/or imprisonment, depending on the seriousness of the infraction. This system has been the de facto approach for spectrum allocation and enforcement in the USA since the Radio Act of 1927, which determined the creation of the FCC to administer spectrum allocation according to ‘public convenience, interest, or necessity’ (Dietz et al., Reference Dietz, Ostrom and Stern2003). The emergence of the FCC addresses, to an extent, open access conflicts arising with spectrum usage (Hazlett, Reference Hazlett2017). Initially, spectrum was allocated through bureaucratic priorities in a typical command-and-control fashion. Inspired by the property rights approach of Ronald Coase, the FCC instituted markets to allocate spectrum (Coase, Reference Coase1966; Hazlett et al., Reference Hazlett, Porter and Smith2011). The main mechanism for spectrum assignment and allocation used by the FCC (and most regulators internationally) has been spectrum licensing. Licenses provide incumbents with exclusive property rights to use the corresponding frequency bands, if they remain consistent with the underlying license conditions (Bazelon, Reference Bazelon1975).
The licensing system is a property rights approach. Similar to command and control, the creation of property rights overcomes commons dilemmas (Alchian, Reference Alchian1965; Demsetz, Reference Demsetz1967). The difference between market and government allocations is that once the government establishes private property rights, the market will allocate them to their highest value uses, subject to the transaction costs of reallocating them (Allen, Reference Allen2015; Coase, Reference Coase1960).
Self-governance may be a more appropriate institutional arrangement to allocate spectrum even though the licensing system is considered an improvement over bureaucratic allocation of spectrum. This is particularly true in cases where more than one user is concurrently accessing the bands, as is the case of spectrum-sharing scenarios. First, although the property rights approach recognizes the possibility of the emergence of rights in government-less environments (Anderson and Hill, Reference Anderson and Hill2004; Umbeck, Reference Umbeck1977), the assignment of rights in the Coasean setting comes from government. Leeson and Harris (Reference Leeson and Harris2018) and Leeson et al. (Reference Leeson, Harris and Myers2020) explain some of the challenges with government-created property rights. In many instances, rent-seeking contributes to government failure, but this is not always the case. If those in charge of creating and assigning rights are not residual claimants, then the rights created may not reflect an efficient property rights arrangement. In such situations, self-governing communities cannot push the costs of definition and enforcement of rights to the state, the ultimate third-party enforcer. When self-governing communities bear the full costs and reap the full rewards from definition and enforcement of rights, they have strong incentives to get the property rights ‘right’. Thus, if self-governance is possible for spectrum, it may be an improvement over government-enforced private property rights.
Second, all private property rights involve some extent of monopoly power that can reduce innovation (Posner and Weyl, Reference Posner and Weyl2018). One way to address this is by more continuous use of markets to allocate rights, or what Posner and Weyl (Reference Posner and Weyl2018) call ‘radical markets’. Through technology, self-governance can enable a more continuous reallocation of rights in response to local conditions.
In recent years, telecommunications regulators in the USA (i.e. the FCC and NTIA) have been working toward shifting from an exclusive licensing scheme to more technically and economically efficient methods for the use and allocation of spectrum bands. One of the most recent approaches has been spectrum sharing between Federal and Commercial entities (Matinmikko et al., Reference Matinmikko, Mustonen, Roberson and Röning2014). This ‘non-traditional’ allocation approach aims to change the current exclusive licensing methods to allow for more flexible resource allocation that addresses many of the challenges stemming from centralized, property-rights approaches.
The non-traditional approach for resource allocation still falls short of self-governance. Our comparative institutional analysis considers both governance structures and enforcement systems that range from formal institutions in command-and-control to self-policing frameworks. In the case of the latter, government controllers or community structures (e.g. third-party agencies) are not required (at least as principal actors). This government-less environment constitutes a distributed enforcement approach. It is an ‘anarchy’, which is defined by a lack of formal government intervention, where norms, rules, and enforcement mechanisms are solely the product of repeated interactions among the intervening agents in a given environment (Leeson, Reference Leeson2006, Reference Leeson2007).
In this work, we propose a CPR-based governance model for self-governing and self-monitoring in Federal–Commercial spectrum-sharing scenarios. For our analysis, we have selected a well-defined and widely known sharing framework: The Advanced Wireless Services (AWS-3) band in the 1,695 and 1,710 MHz frequency range (i.e. the 1,695–1,710 MHz band). This environment is characterized by its simplicity and well-defined rules, which allow us to illustrate how self-governance would look like in a spectrum-sharing scenario.
We use agent-based modeling (ABM) to study self-governance in spectrum sharing. By designing and developing an ABM for this specific sharing scheme, we are able to analyze the suitability of the proposed self-governance mechanism in greater detail. ABM simulations allow us to observe how macro-phenomena can emerge from micro-level interactions among independent agents. In this regard, this approach provides insight into the emergence of what Hayek referred to as spontaneous order: order which arises without a conscious design of enforcement (Boettke and Coyne, Reference Boettke and Coyne2005; Hayek, Reference Hayek2012). Our central finding, to paraphrase Leeson (Reference Leeson2014a, Reference Leeson2014b), is that spectrum self-governance is a real possibility and works better than we think, under the right circumstances.
This paper is organized as follows: in Section 2, we present an overview of the main characteristics of the sharing framework and self-governance. Then, in Section 3, we describe the ABM created for this work. Finally, in Section 4, we discuss our ABM-based experiment and the main results of our analysis. Section 5 concludes the paper.
2. Sharing Framework and Self-governance Systems
We focus on the scheme defined for the 1,695–1,710 MHz band as our base spectrum-sharing model. This frequency band is part of the Advanced Wireless Services (AWS-3) defined by the FCC and the NTIA (Commission et al., 2014). We have selected this framework for its simplicity (e.g. there is only one primary user (PU) or incumbent, which is physically stationary) and the advantages of working with an existing, widely known, and well-defined sharing scheme.
In this Federal–Commercial sharing framework, the participants have been previously defined by the FCC and NTIA in a ‘license-like’ manner. The incumbents or PUs in this sharing scheme are the meteorological satellites of the National Oceanic and Atmospheric Administration (NOAA). The secondary users (SUs) or new entrants are mobile phones (i.e. LTE mobile stations) (Commission et al., 2014; Weiss et al., Reference Weiss, Lehr, Acker and Gomez2015). Note that, even though the number of PUs is predefined in the band, the number of allowed SUs might vary according to different locations of the incumbents across the USA, and transmission opportunities which depend on traffic and congestion in the radio spectrum.
One key aspect in spectrum-sharing scenarios is that incumbents or PUs should be protected against ‘harmful interference’, which can be defined as external wireless transmissions that impact the normal operations of a given station. This interference is usually the product of the operations of other users in the band (e.g. new entrants) (Saruthirathanaworakun and Peha, Reference Saruthirathanaworakun and Peha2010). For the 1,695–1,710 MHz band, the FCC and NTIA proposed two static protection zones in order to mitigate possible interference events: (1) an exclusion zone (EZ), which is a restricted area where no new entrants are allowed to operate, and (2) a coordination zone (CZ), which extends beyond the EZ boundaries, and allows for new entrants' operations under predefined circumstances (Commission et al., 2014). The size (i.e. boundary) of these protection areas is based on several technical factors, including transmit power, time variations, receiver susceptibility to interference, propagation effects of radio waves, among others (Bhattarai et al., Reference Bhattarai, Ullah, Park, Reed, Gurney and Gao2015). Note that, even though the boundaries of these ‘protection zones’ have been revised on multiple occasions by the FCC and NTIA, they are still static (Altamimi et al., Reference Altamimi, Weiss and McHenry2013), hence representing a centralized governance approach. This centralized approach has the potential of reducing the value and incentives for new entrants, and it disregards important factors that impact local-sharing conditions.
Based on the definitions of EZs and CZs, several authors (Altamimi et al., Reference Altamimi, Weiss and McHenry2013; Bhattarai et al., Reference Bhattarai, Ullah, Park, Reed, Gurney and Gao2015, Reference Bhattarai, Park, Lehr and Gao2017) have designed methods to specify the characteristics of this Federal–Commercial sharing environment. In particular, authors have sought to develop methods for creating and sizing both restriction zones. Available approaches propose a more flexible scheme than the one suggested by the FCC/NTIA, as their main objective is to reduce the size of both zones to increase the value and incentives for new entrants. In this paper, we use the notation introduced by Bhattarai et al. (Reference Bhattarai, Ullah, Park, Reed, Gurney and Gao2015) to define the ‘Multi-Tiered Incumbent Protection Zones (MIPZ)’. This framework allows the PU to adjust the size of the CZs and EZs ‘on the fly’. As a result, three zones (or areas) are defined around the PUs' transmitters (see Figure 1):
• No access zone (NAZ): Spatial area in the immediate vicinity of the PU, where transmission privileges are limited to licensed incumbents.
• Limited access zone (LAZ): Spatial area surrounding the NAZ. In this region, a limited number of new entrants are allowed to transmit simultaneously. The limit in the number of simultaneous transmissions is determined by the PU.
• Unlimited access zone (UAZ): The region that lies outside the outer boundary of the LAZ. Unlimited transmission privileges are granted to the SUs in this area.

Figure 1. Definitions of the 1,695–1,710 MHz band environment.
A common, ‘alternative’ governance system that has emerged from the study of CPRs is self-governance (Ostrom, Reference Ostrom2010). In such systems, all appropriators of CPRs repeatedly show their capacity to organize themselves, create rules, monitor others and themselves, and successfully enforce the agreed-upon norms. Hence, these self-governed organizations are able to create self-organized and self-controlled institutions without the need to fully rely on central authorities (e.g. governmental entities). As explained in Ostrom (Reference Ostrom1997), the self-governance institutions that have emerged have been sustained over long periods without the participation (at least as principal actors) of any external agency. Thus, self-governance has become a viable mechanism to govern complex CPRs.
Note that self-governance (i.e. government-less, private-governing, or self-enforcement) does not refer to the complete absence of law; instead, it refers to the lack of a formal government or state dictating and enforcing the law. The main idea here is that agents who find themselves in government-less situations (or choose to eschew government) develop their own, privately created law (Leeson, Reference Leeson2014b). A common assumption in centralized approaches is the presence of an ‘authority’ figure (e.g. law enforcers). This figure is deemed as a necessary mechanism to guarantee that agents are going to ‘behave’ and not break the agreements (ex-ante) in future interactions (ex-post). Consequently, the natural question in private-governing arrangements is: how is law enforced? The short answer to this question comes from the ‘discipline of continuous dealing’ (Bernstein, Reference Bernstein1992; Leeson, Reference Leeson2014b; Stringham, Reference Stringham2002). The idea behind this principle is simple, ‘if you do not behave today, I will take repressive actions’. These actions include stopping the interaction with you tomorrow, telling others not to interact with you, reduce your future privileges, etc. Consequently, if you value future interactions with a given user and their social network (e.g. neighbors), you will not break the ex-ante agreements. In this type of system, the continuous interactions usually lead to a stable state. In other words, a point in the dealing process where the agents agree to a given set of system parameters that benefit all parties.
This principle lies at the heart of contractual theories of governance. Alchian and Demsetz (Reference Alchian and Demsetz1972) argued that discipline within firms is like a market: managers in the firm ‘punish’ only by withholding future business or by use of courts to honor exchange. An employer ‘can fire or sue, just as I can fire my grocer for stopping purchases from him or sue him for delivering faulty projects’ (Alchian and Demsetz, Reference Alchian and Demsetz1972: 777). Managers continuously renegotiate contracts in ways that are acceptable to both parties. The difference is team use of inputs: the firm is a centralized contractual agent in a cooperative team production process. The contracts themselves, as Macaulay (Reference Macaulay1963) understood, are open ended.
Continuous dealing applies to government and self-government. Governments certainly rely more on coercion, though what Somin (Reference Somin2020) calls ‘foot voting’ is similar to a market, provided individuals can move jurisdictions. For self-governance, law is enforced the same way it is with a grocer: when your neighbor does not do what you agree to, in a sense, you ‘fire’ them, though if something changes, the relationship can be resumed in the future. Thus, our expectation is that the extent to which self-governance works will reflect the ability of the users of spectrum to enforce their rules along the lines suggested by Macaulay, Alchian and Demsetz, and of course, Elinor Ostrom.
It is worth mentioning that contrary to the centralized approach, self-enforcement is not a one-size-fits-all solution. Different self-enforcement contexts come with different problems of property protection and conflict resolution. Therefore, one particular model of a private-governing institution will not necessarily be successful in a different context. More importantly, these emerging institutions are fitted to maximize the well-being of the members of a specific community by continuously adapting their rules, norms, and practices.
A key aspect in government-less scenarios is the difference in the cost of government (Leeson, Reference Leeson2006). In the case of the spectrum-sharing scheme in the 1,695–1,710 MHz band, the cost of ‘creating a state’ can be neglected (the FCC and NTIA are fully working entities). Nonetheless, we still find organizational costs of government in this scheme. As an example, consider the decision-making costs of defining the set of rules and their enforcement. The FCC and NTIA have defined static-shielding areas to protect the incumbent of the band. These definitions are more or less uniform across all 10 locations selected for the sharing scheme of the 1,695–1,710 MHz band (Force, Reference Force2002). This approach adds little value and incentives for new entrants, since it does not consider spectrum usage dynamics. For instance, the antennas in the satellite earth station are not stationary.Footnote 1 Instead, they ‘follow’ the satellite as it moves around the earth, changing the shape of the area to be shielded. Furthermore, decisions made by centralized entities (in this case, the FCC and NTIA) may result in solutions that disregard interests that are particular to local environments. For instance, some NOAA satellites are in densely populated areas with a higher traffic exchange.
Self-governance can help us overcome many of the challenges stemming from decisions made by centralized government entities. For instance, since the decision-making process would not be centralized anymore, decisions could now be dynamic. A more flexible or dynamic scheme could reduce the size of the restricted areas, which would increase the value of spectrum and provide incentives for new entrants. Besides, all decisions would be made at a local level, taking into account local interests. Such an approach would help reduce possible externalities (and negative effects on other, coexisting, sharing schemes), and would address the actual needs of local communities.
3. The agent-based model
Agent-based models consist of individual and independent agents (e.g. persons, households, firms, technological equipment, etc.) that are represented as software objects with multiple characteristics (e.g. gender, age, utility functions, perception functions, etc.). All these agents interact with each other and the environment where they are placed. This allows us to study potential emergent macro-phenomena (Badham, Reference Badham2015). Many authors studying CPRs have successfully explored CPR management and governance problems through the implementation of agent-based models (Deadman et al., Reference Deadman, Schlager and Gimblett2000; Ghorbani and Bravo, Reference Ghorbani and Bravo2016; Jager and Janssen, Reference Jager and Janssen2002; Janssen and Ostrom, Reference Janssen and Ostrom2006a).
Our model simulates the interaction of two main types of agents: (1) a single PU or incumbent (e.g. meteorological satellite), and (2) several SUs or new entrants (i.e. LTE handsets).Footnote 2 A third type of agent represents the base stations or eNodeBs that serve as coordination and communication points for the PU and SUs. All these agents are placed in a simulation environment that captures the transmission zones (i.e. NAZ, LAZ, and UAZ) defined for the sharing scheme in the 1,695–1,710 MHz band. The main premise of the model is that the size or boundaries of the restricted zones are not static, as previously defined by centralized authorities (e.g. the FCC). Instead, zone boundaries are the result of the continuous interactions and the communication efforts among the PU and SUs. The main intent of this negotiation process is for the agents, and only the agents, to agree on optimal boundaries for the restricted zones (LAZ and NAZ) that protect the incumbent and provide enough incentives for the new entrants. This captures a key aspect of self-governance, the discipline of continuous dealing.
The model considers that conflict situations may arise, and these represent circumstances where the normal operations of the incumbent are impacted by unauthorized actions by one or more new entrants (or SUs). These conflict situations (i.e. interference or enforceable events) arise in the restricted areas of the sharing scheme (i.e. LAZ and NAZ).Footnote 3 To avoid future conflict situations, the PU increases the size of the restricted areas to obtain additional interference protection against unauthorized SUs' transmissions. Nonetheless, an increase in the size of the restricted areas reduces the available spectrum space for new entrants. In the absence of conflict (i.e. when SUs are complying with the transmission requirements in the band), the PU reduces the size of its protection zones, hence increasing participation incentives and resource value for the SUs. The ideal scenario in this continuous dealing framework is to find the ‘optimal’ boundaries for the different sharing zones, which would lead to a scenario where the system is in a ‘stable’ state. In the context of our work, stable means that there are no future drastic changes in the size of the restricted zones. In other words, a stable system would represent a well self-governed band where agents agree on a restricted zone size that guarantees that conflict situations would not impact the normal operations of the PU, while giving enough incentives to the SUs (i.e. higher opportunities to transmit) to use the band. In the sections that follow, we present additional details of the different components of our agent-based model.
3.1. The agents
The spectrum-sharing framework of the 1,695–1,710 MHz band comprises three types of equipment utilized by the PUs and SUs: meteorological stations, mobile handsets, and base stations. These entities are represented as independent agents in our model (see Figure 2), and they have the following characteristics:
• NOAA Meteorological Satellite (MetSat Station): A single, static agent located in the middle of the protection zones.
• LTE mobile stations (LTE handsets): Multiple agents that move around the zones while communicating to their corresponding eNodeBs.
• Base stations (eNodeB): Four static agents that only serve as coordination and communication points between the PU and SUs.

Figure 2. Agents and the environment of the agent-based model.
3.2. Agent's behavior
The PUs and SUs, through the agents that represent them, are assigned a pre-defined set of functions or responsibilities at the beginning of our model simulation. These functions account for the behavior of each entity. Note that even though we have defined three types of agents in our model, only the incumbent or PU (MetSat station) and the new entrants or SUs (LTE mobile stations) of the band are continuously interacting. The eNodeBs only serve as communication points between these agents.
• PU's behavior: The PU defines the initial boundary of the restricted zones, as well as the maximum number of simultaneous transmissions that can take place in the LAZ.
• SUs' behavior: The mobile stations are randomly moving around the environment. At each time period (i.e. simulation time step), each mobile station determines whether it has data to transmit. If a transmission is required, the handset makes a decision whether to start a communication based on its independent perception of the environment.
3.3. Rules, norms, and strategies
Once the main functionalities of the agents are assigned, the next step is to define the rules, norms, and individual strategies that the agents will follow throughout the simulation. As defined by North (Reference North1991), institutions are ‘[t]he set of rules actually used by a set of individuals to organize repetitive activities that produce outcomes affecting those individuals and potentially affecting others’. Based on this definition, we can see that the 1,695–1,710 MHz sharing framework can be categorized as an institution: the actions of the incumbents have an impact on the new entrants and vice versa. This new definition is key to leverage the benefits of the ADICO Grammar of Institutions, which is a model that permits the definition of shared strategies, norms, and rules as simple statements using five components (Crawford and Ostrom, Reference Crawford and Ostrom1995; Ghorbani and Bravo, Reference Ghorbani and Bravo2016):
• Attributes: The participants to whom the institutional statement applies (i.e. the agents of the system).
• Deontic: The deontic operators dictate the actions allowed to the agents: obligated, permitted, and forbidden.
• aIm: Describes the action or outcomes to which the institutional statement applies (i.e. the actions related to the deontic operator for each agent).
• Condition: The set of parameters that define when and where a statement (i.e. rule, norm, or strategy) applies.
• Sanction (or else): Is the consequence of non-compliance with an assigned institutional assignment.
We leverage the simplicity of the ADICO model to define the rules for both, the PUs and SUs in our system as explained in what follows.
3.3.1. Definitions for the primary user
The main responsibility of the PU in the system is to update the boundaries of its surrounding protection zones and communicate them to the eNodeBs in the band. This update process is based on the behavior of the SU agents surrounding the PU, in particular, the presence of conflict (i.e. interference) situations (see Table 1). The MetSat can reduce the size of the LAZ and NAZ areas if it receives a ‘good’ signal from the SUs (i.e. no interference has occurred). It can also increase the size of both zones to achieve greater protection against interference events. In any case, the variation in the size of these zones has a direct impact on the ability to detect enforceable events (i.e. the detection rate decreases when the monitoring area increases). For our model, we have selected a linear relationship to capture this problem, which is described in expression (1):

Table 1. ADICO-defined rules for the PUs

Expression (1) captures the relationship between increasing the size of the protection zones, S, and the ability of the system to detect interference situations, d. This detection rate of interference events is also the product of M, which represents the minimum size of the zone to avoid interference, and E, which is the detection effectiveness of the equipment being used (i.e. a probabilistic variable of whether an interfering agent was ‘caught’). Although the size of the restricted areas is dynamically adjusted in the system, the PU still has to decide the initial boundary of the restricted areas. In self-enforcement, this is considered as an ‘initial gesture of trust’ to start a dealing process (Leeson, Reference Leeson2014b). Whether an interference event is detected or not by the system in place, the PU is responsible for updating the size of the restricted zones. This is given by the strategy for the PU to modify the boundaries defined according to expression (2). Once the PU has defined the size of the restricted zones, it communicates this information to the band coordination points (i.e. LTE eNodeBs):

3.3.2. Definitions for the secondary users
The new entrants in the band start by obtaining information on the size of restriction zones from the LTE eNodeBs. At the same time, SUs are moving around the environment while transmitting using the available spectrum space. To model the behavioral strategies of SUs, we have relied on tax evasion literature, particularly on the studies by Bloomquist (Reference Bloomquist2004), Mittone and Patelli (Reference Mittone and Patelli2000), and Davis et al. (Reference Davis, Hecht and Perkins2003). Such well-known modeling strategy allows us to capture user perception of enforcement when complying with the assigned rules. In this manner, although all SU agents have a set of rules to follow (see Table 2), they might break them from time to time, based on their own enforcement perception and associated risk profiles. In other words, they might choose to transmit in the NAZ or the LAZ (when the maximum threshold has already been reached), even though this would cause an interference event.
Table 2. ADICO-defined rules for the SUs

To account for this perception-based decision-making process, our model is based on the standard microeconomic theory of Allingham and Sandmo (Reference Allingham and Sandmo1972). This economics theorem states that a given user will break the rules whenever the perceived caught rate, p, and penalty rate (i.e. sanction), f (where f ≥ 0), take on values that make expression (3) true:

The problem with equation (3) is that it does not capture other factors that affect the decision-making process of a given agent. Bloomquist (Reference Bloomquist2004) argues that rule-breakers with high compliance opportunity costs (i.e. high discount rates) are more likely to break the rules than other agents. Nonetheless, this is not the only factor that influences the decisions of a given agent. For instance, the time lag between breaking-the-rule and the sanction, or the perceived detection ability of the system should also be taken into account. Consequently, we can use the alternative decision-making expression shown in equation (4):


With our new parameters, a given user will break the rules if, and only if, expression (4) is true. The cr factor is the product of the interaction of the most important factors affecting the decisions of a given agent, and it is defined by equation (5). In equation (5), t is the average number of time periods between the infraction and the detection; d is the detection rate of the enforcer, where 0 ≤ d ≤ 1; and r i is the discount rate for the agent i (Bloomquist, Reference Bloomquist2004). Based on expressions (4) and (5), an SU agent will break the rules whenever the perceived caught rate, p, and the agent perception, cr, take on values that make expression (6) true:

The factors described in expressions (4), (5), and (6) can take on multiple levels. Furthermore, different combinations of these factors can result in distinct decision-making processes for the agents, as depicted in Figure 3. For example, if the detection is immediate, the decision to transmit depends only on the detection rate, d. If only one time period passes between the infraction and the sanction, an agent's transmission decision is based only on its discount rate, r i. We also observe that the discount rate, detection time, and detection rate of the system affect the different features of the decision-making process, hence providing different outcomes. This shows that the Bloomquist expression captures all the factors involved in the decisions of an independent agent, in a very concrete manner. In our agent-based model, we capture all the aforementioned parameters (see Table 3).

Figure 3. Effects of the different parameters on the decision-making process of a given SU agent.
Table 3. Independent variables and levels included in the ABM simulations
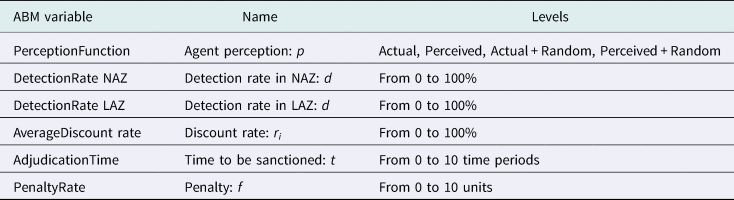
Different perceptions: Our model is based on the perception of the users about not only the probability of being caught but also, on the status of the neighbors (i.e. a ‘social network’). The individual perception of each agent can take four types of functions:
(1) Actual: The agents know the exact detection rate, d. All agents have the same perception with no distinctions.
(2) Actual + Random: The agents know the exact detection rate, d. Nevertheless, they have different perceptions based on their risk profiles.
(3) Perceived: The agents do not know the exact detection rate, d. A random perceived rate is assigned to each agent. These rates are based on their risk profiles. It is important to note that these agents' perception is updated according to their own experiences and those of their neighbors. Hence, when a certain agent of one of its closest ‘friends’ is sanctioned (i.e. ‘caught’), it changes its perception and corresponding future behavior according to the aforementioned expressions.
(4) Perceived + Random: The agents do not know the exact detection rate, d. A random perceived rate is assigned to each agent. These rates vary according to the agents' risk profiles. This perception is dynamically updated based on the experiences of an agent's and its neighbors.
The social network of an agent refers to the ‘status’ of the neighbors of a given agent. In other words, neighbor's sanctions have an impact on an agent's perception regarding future interactions. Consequently, the social network of an agent modifies its behavior and strategies. This characteristic was included to capture the effect of ‘social pressure’ in the agents' decision-making process. This allows us to simulate what happens when communication and information exchange between agents is added to the governance process (Ostrom, Reference Ostrom2010).
3.4. Implementation
Our ABM was implemented in the NetLogo platform. This platform allows for multi-agent programing and a modeling environment for simulating natural, artificial, and social phenomena. One of the main characteristics of NetLogo is that it is particularly well suited for modeling complex systems evolving over time. This tool also facilitates the exploration of connections between micro-level behaviors of independent individuals (i.e. agents) and macro-level patterns that emerge from their interactions (Tisue and Wilensky, Reference Tisue and Wilensky2004). The resulting model is the product of the agents and their corresponding rules, norms, strategies, and interactions as shown through the model screenshot included in Figure 4.

Figure 4. Screenshot of the user interface of the ABM implemented in NetLogo.
4. Experiments and results
4.1. Experiments
To capture all the possible combinations of the factors and variables implemented in the ABM, we utilize full factorial experimental design: all combinations of levels, assuming k factors, every ith factor with n levels and r repetitions for each level being tested (see expression (7)). For our setup, we have chosen a total of 10 replications for each experiment to guarantee that the variance in the model is captured. It is necessary to point out that in the results highlighted in the following section all figures but Figure 9 capture the average behavior of the different factors, levels and repetitions:

In a self-enforcement governance mechanism, the different factors of the system are coordinated between the agents representing the PUs and SUs. However, as an initial setup of the system, the model requires the input of two key factors: initial zone size (LAZ and NAZ) and detection effectiveness (see Table 4). To simulate the different interactions, we also need to define some basic characteristics about the agents and the environment, which are detailed in Table 5.
Table 4. Self-enforcement independent variables, factors, and levels

Table 5. General environment independent variables, factors, and levels
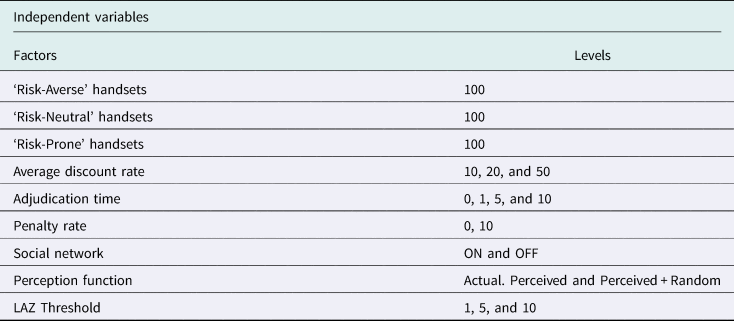
4.2. The results
The main goal of the experiments presented in this work is to observe the viability of having a self-governing scheme in spectrum-sharing scenarios and the conditions leading to it.
The first step in validating our spectrum self-enforcement scenario is to verify that the model is, indeed, capturing the basic components of detection and size conditions. In this regard, we first need to verify that a change in the boundaries of the restricted zones is changing the transmission conditions for the new entrants in the band. From the previous sections, we know that the boundaries of the restricted zones, LAZ and NAZ, result only from the interactions between the incumbent and the new entrants. Similarly, the ability to detect interference (i.e. enforceable event) is based on the size of the area to monitor and the effectiveness of the method used to detect these potential events. Let's first consider the results in Figure 5. In the graph on the top right corner, we observe the impact of the size of the NAZ on the average number of possible enforceable events. We can observe that the mean number of events increases as the size of the NAZ increases. The graph on the bottom left corner shows the relationship between the detection effectiveness in the NAZ and the number of interference events. The resulting detection rate in the zone has a negative impact on the average number of interference events as it increases from 0 to 100%. The same phenomenon is described for the case of LAZ, in the top and bottom right graphs of Figure 5. These results concur with the initial design of the model in terms of the global behavior of agents, the detection system in place, and the boundaries of the restricted zones.

Figure 5. Number of interference (conflict) events in the restricted areas of the sharing scheme (NAZ and LAZ).
Another key characteristic of our model is that the SU's behavior is based on their perception of the environment. This includes the enforcement and detection perception and the status of their ‘social network’. An essential part of these agents' perception is access to information. We capture this through agents' knowledge of the enforcement rate of the system: a given agent can learn the actual enforcement (i.e. detection) rate or have its own perception about it. We observe that this characteristic has a significant impact on the number of events in the system. This means that knowing the actual detection rate avoids some unauthorized transmissions, which would occur were agents to rely only on their rate perception (see Figure 5). Our results show that, in cases where the detection rate is low, full knowledge of this characteristic results in a maximum peak in the number of events in the system. This is consistent with the behavior of users and their perceptions of enforcement and auditing described in the tax literature (see e.g. Bloomquist, Reference Bloomquist2004).
While negotiations of the different enforcement parameters take place dynamically, two initial parameters are still necessary for our simulation: the boundaries of the restricted areas and the detection effectiveness of the enforcement system (i.e. the amount of policing elements). These parameters are key due to the significance of initial signaling or initial gestures in a self-governing arrangement. These gestures, by one or more of the intervening agents, are defined as initial tokens of trust when starting a dealing relationship (Leeson, Reference Leeson2006, Reference Leeson2007, Reference Leeson2014b). In Figure 6, we highlight the influence of the initial size of both areas, NAZ (top graph) and LAZ (bottom graph), on the average number of interference events in the system. When considering the smallest restricted zone size around the PU, which represents its highest trust signaling gesture, we find that, on average, 25% of the time there are no, or a very small number, of interference events in the restricted zones. On the other hand, for larger initial area sizes (e.g. 75 and 100%), we see a higher average number of interference events. This is more evident when the initial boundary is at its maximum possible size. In this case, the detection rate causes an immediate peak in the number of events from the first time period.Footnote 4

Figure 6. Effects of the initial size.
Another initial signaling element in our model is the detection effectiveness (i.e. the number of policing elements) of the system. In Figure 7, we can observe how this factor shapes the environment, where additional efficacy implies a bigger number of sensing and detecting elements in the system. As depicted, the effectiveness factor alone does have an impact on the average number of events in both the NAZ (top graph) and LAZ (bottom graph) restriction areas. Nonetheless, it is not as clear as the impact of the size of the restricted zones. In this case, all the scenarios have at least some interference events. Furthermore, as expected, the number of events is inversely proportional to effectiveness (i.e. as effectiveness increases there are fewer interference events). This behavior agrees with other self-governing signaling examples in the literature (see, e.g. Leeson Reference Leeson2014b).

Figure 7. Effects of the detection effectiveness.
In our model, a well self-governed 1,695–1,710 MHz band is one where the system reaches a ‘stable’ state. Stability represents a condition in which the incumbent and the new entrants of the band reach an agreement on the size of the restricted zones around the PU without a government, in any form, intervening in the negotiation process. Furthermore, when the system is in a stable state, the number of conflict situations (i.e. interference events) due to SUs' unauthorized transmissions is minimal, hence limiting the impact on the normal operations of the PU.Footnote 5 In this regard, we can observe in Figure 8 that the proposed negotiation for the size of restricted areas takes place in almost all scenarios regardless of their initial configurations. All simulations representing a case where there is a change in the initial boundaries of the restricted zones (left graph) converge to a stable state in which we reach an agreement on a proper area size. Additionally, we notice that when initial sizes are over 50% of the maximum allowed, they are reduced to more manageable boundaries. When analyzing the detection effectiveness of the system (right graph), we observe that this factor has an impact on the negotiation process. This is due to the fact that when a higher number of agents are caught or their neighbors have been sanctioned, their perception of the enforcement mechanisms changes. Consequently, the number of interference events is reduced, and negotiations take place to adjust the size of the restricted areas. In the particular case of effectiveness, when it is very low, we can expect only an increase in the LAZ and NAZ. However, for values over 50%, we can see a reduction in the areas, which is even more evident at very high effectiveness rates. When considering the effectiveness of the system alone (i.e. the equipment capabilities to detect interference events), we can observe that the entire system also reaches a stable state. In other words, there are no further changes in the boundaries of the restricted zones.

Figure 8. Evolution of the SU/PU negotiation process of updating the LAZ and NAZ size.
As previously mentioned, another key element when evaluating the stability of the system is the number of conflict events occurring in the system. In this context, it is important to observe how the amount of interference events (i.e. enforceable events) correlates with factors such as the initial signals provided by the PU and SUs. In Figure 9, we describe the relationship between the initial gestures and the total number of events in the system. In this figure, the x-axis represents the size of the restricted zones, the y-axis shows the effectiveness of the detection method, and the proportion and color of the ‘bubbles’ represent the total number of events in the simulation. These results show that the combination of a very high detection rate and the smallest initial size results in the lowest total number of enforceable events in the system. Furthermore, we find the lowest total number of events in all cases representing smaller restriction areas. For larger area sizes, we observe an interesting phenomenon: even when the detection effectiveness increases, the number of events is not reduced in the same proportion. This demonstrates again that in self-enforcement scenarios, signaling between users has a greater impact than the effectiveness to catch ‘bad’ agents.

Figure 9. Initial size versus detection rate and their impact in the number of interference (conflict) events.
These results lead us to conclude that a self-enforcement mechanism could be a successful alternative to govern and enforce the spectrum-sharing framework of the 1,695–1,710 MHz band. Nevertheless, it is necessary to point out some of the caveats of the system. First of all, the band has well defined and identifiable participants, which makes it easier to assign the norms and rules for each participant. Second, as in many other self-governing scenarios, the system reaches a stable state where the PUs and SUs can agree on the parameters of the system; however, this requires a continuous process where enforceable events are still happening in many situations. Finally, the outcome of the system is highly correlated with the initial signaling process. In light of this, gestures of higher trust generate better scenarios for future dealings. This is especially true in cases where the initial size of the CZs and EZs are smaller. Furthermore, initial gestures in self-governing scenarios were a more successful path to reduce the number of enforceable events than increasing the system's ability to catch bad agents (i.e. detection effectiveness, E), which is usually the premise of private property rights schemes.
5. Conclusions
In this work, we explored the spectrum as a CPR, using the ABM to examine alternative governance structures. ABM has been widely used in other CPR settings, including models of fisheries, forests, and water systems (Janssen and Ostrom, Reference Janssen and Ostrom2006a, Reference Janssen and Ostrom2006b). Nevertheless, little work has been carried out to explore spectrum sharing using ABM, especially self-governance of the spectrum commons. Our approach complements the existing studies of enforcement in the law and economics literature (e.g. Polinsky and Shavell, Reference Polinsky and Shavell1998, Reference Polinsky and Shavell2000) by conceptualizing users' perceptions rather than utility, which allows us to capture how characteristics, such as future value of transmissions and the delay between infractions and sanctions introduced by the enforcer, influence spectrum governance. Also, this approach allows us to analyze the consequences of differences in the knowledge of the enforcement system. The most important aspect of self-enforcing governing is the successful interaction of PUs and SUs. We showed that the size of the boundaries around the incumbent users, and hence the ability to detect ‘bad guys’ within the system, stems only from the negotiation process of independent agents. Furthermore, the system successfully allocates the shared resources according to the band's predefined set of rules irrespective of the initial conditions, such as initial signaling. Thus, spectrum sharing through a self-governing arrangement is possible under a wide variety of realistic circumstances.
Regarding the process of self-governance, we showed that once the initial boundaries assigned into the categories of limited and unlimited use, the trust signal of reducing the size for the starting point has the biggest impact on governance of spectrum. When starting with the smallest size, we can expect little or no interference with the system, which is consistent with the continuous dealing principle: good gestures by PUs are ‘paid’ by the SUs, and vice versa. Our analysis also shows that perception characteristics, as represented by differences in perception functions of the SUs, have a great impact on self-governance. When users know the rate of detection, more ‘infractions’ are committed when the detection rate is relatively low. On the other hand, when the agents only have a perception of this rate, the number of events is considerably reduced. Nonetheless, the sole perception of a rate leads to the occurrence of interference events whereas in full knowledge scenarios, especially with higher detection rates, this is not the case. In this regard, one of the main benefits of adopting self-governance frameworks is that sharing schemes can switch from static and centralized definitions to local and dynamic agreements. Such agreements would reflect the local conditions of the sharing process, provide enough protection to the incumbent, and add significant value and incentives to the new entrants.
These results show that a self-governance structure is possible in spectrum-sharing scenarios under the right circumstances. For the band of our analysis these circumstances include a set of well-defined participants, communication channels, sharing conditions, and, most importantly, a common goal of defining optimal protection zones (i.e. avoid conflict situations for the PU while providing incentives and value for the SUs). Additionally, the band provides a clear definition for the different interactions between agents and the associated rewards for a ‘good’ behavior. As aforementioned, self-governance is not a ‘one-fits-all’ solution. In light of this, other spectrum-sharing scenarios might not benefit from a self-governing approach. For instance, if there is no common incentive between the agents to reach a continuous and stable dealing process, there is a lack of clear definitions for the different agents, or there is an absence of clear communication channels between agents that lead to ‘unstable’ dealings and a high number of conflict situations.
There are several avenues for future research. This includes exploring additional rules of association and negotiation in the self-enforcement approach, including the initial assignment of property rights, as well to study additional strategies and rules in the agents' behavioral space that may influence the stability of the system. Along these lines, we also seek to explore the efficiency of the ‘stable’ state of the system by incorporating a marginal analysis, on behalf of the PU, of costs of detection and benefits of less ‘harmful interference’. Another area for future research is exploring additional options for the governance framework, such as the collaborative systems appearing in the literature of CPRs. One example of this type of enforcement is the polycentric approach studied by Ostrom (Reference Ostrom2010). Moreover, an important avenue to consider is community enforcement systems (Leeson, Reference Leeson2008), where the task of ‘catching bad agents’ is a collaborative effort of multiple agents at the same level of hierarchy.
More research is also necessary regarding the top-down aspects of self-governance. Ostrom et al. (Reference Ostrom, Agrawal, Blomquist, Schlager and Tang1989) emphasized that the success of self-governance depends in part on the extent that higher-level authorities recognize the rights to self-governance. Pennington (Reference Pennington2013) extends Ostrom's perspective by showing that to understand why top-down government works better than bottom-up governance, it is necessary to explicitly consider the information and incentives problems confronting government. Research on commons also emphasized the meta-political challenge that the government requires incentives to provide communities with autonomy to self-govern (Agrawal and Ostrom, Reference Agrawal and Ostrom2001), as well as the analysis of the relationships between collective action and governance of commons (Poteete and Ostrom, Reference Poteete and Ostrom2004). When combined with the idea that change in institutions depends on the cost and benefits to the privatizers (Allen, Reference Allen2015; Leeson, Reference Leeson2019), the analysis of the incentives of the FCC/NTIA, along with coalitions that may oppose self-governance, is an important future area of research.
Acknowledgements
This work was sponsored in part by the National Science Foundation through grants 1265886, 1547241,1563832, 1642949, and 1642928. We thank the editor, Geoffrey Hodgson, and the referees for constructive feedback. We thank participants in the Policy and Political Economy Workshop hosted by the Center for Governance and Markets at the University of Pittsburgh for useful suggestions on some of the ideas presented in this paper.