Choice and experiments
Social movements build power through coalitions. Their messages and messengers must mobilize supporters and persuade the unconvinced while also trying to minimize opposition to their cause (Benford and Snow, Reference Benford and Snow2000). A one-size-fits-all approach may not accomplish both of these goals, because the people social movements hope to reach often differ from those who actually receive their message.
Similarly, a one-size-fits-all experimental approach fails to capture the real world choices that determine who receives a social movement message. While standard survey experiments provide unbiased estimates of average treatment effects (ATE), scholars and practitioners often want to understand the effect of treatment on the people who will actually receive it (Gaines and Kuklinski, Reference Gaines and Kuklinski2011). Experimental designs that allow some subjects to choose to receive a treatment provide a means of estimating these effects (Knox et al., Reference Knox, Yamamoto, Baum and Berinsky2019). Despite the appeal of enhanced ecological validity, such designs remain rare in political science.
By incorporating choice, this study makes contributions to both experimental political science research and to research on social movement messaging. First, like previous studies incorporating choice, our design estimates the effects of treatment on those likely and unlikely to receive it. The former speaks to the potential real-world impact of a social movement’s message, while the latter offers insights into the persuasive potential of a message on a hard-to-reach and potentially resistant audience. Second, we show how randomizing conditional on subjects’ choices can allow researchers to address additional questions of interest while preserving statistical power. Specifically, we use our design to assess whether different messengers are more or less persuasive for these hard-to-reach subjects, but we believe the benefits of randomizing conditional on choice are broader. Experiments incorporating choice are costly, and this innovation offers researchers a way to get more bang for their experimental buck.
Using data from both convenience and nationally representative quota-based samples, we examine how the gender of messengers advocating for the #MeToo movement shapes who receives this message and how they process it. Support for gender equality and the #MeToo movement varies by partisanship and gender (Barnes and Cassese, Reference Barnes and Cassese2017; Deckman, Reference Deckman2018). But, polling on these issues also reveals that experience matters. Those who talk about sexual harassment with women and those with prior experiences with sexual assault and harassment are more supportive of the #MeToo movement. Footnote 1 Women have been the face of the #MeToo movement, and the gender of the movement’s messengers likely shapes attitudes in two ways. First, research on source cues clearly demonstrates that the source of a message matters, and that the effects of source cues are often heterogenous (Arceneaux and Kolodny, Reference Arceneaux and Kolodny2009; Goren, Federico and Kittilson, Reference Goren, Federico and Kittilson2009; Kam, Reference Kam2005; Nicholson, Reference Nicholson2012). Gender, in particular, has been found to shape perceptions of a speaker’s credibility, knowledge, and partisanship (Mendez and Osborn, Reference Mendez and Osborn2010; McDermott, Reference McDermott1998; Winter, Reference Winter2010). But, the gender of a message’s source can also matter in a second, more subtle way. Patterns of political discussion differ markedly by gender (Atkeson and Rapoport, Reference Atkeson and Rapoport2003; Hansen, Reference Hansen1997; Huckfeldt and Sprague, Reference Huckfeldt and Sprague1995). Men are less likely to encounter women in political conversation and vice versa (Karpowitz and Mendelberg, Reference Karpowitz and Mendelberg2014; Mendez and Osborn, Reference Mendez and Osborn2010), and individuals may be more likely to seek out information that conforms to their prior beliefs (Iyengar et al., Reference Iyengar, Hahn, Krosnick and Walker2008; Stroud, Reference Stroud2008, Reference Stroud2010). More concretely, someone may find a female speaker less persuasive for the same reasons they might avoid hearing the woman’s views. By allowing some subjects a choice in whether to encounter our treatment, we provide a more complete picture of who the #MeToo movement is likely to reach, how these individuals will respond, and how to persuade those who are less likely to encounter these messages. The results from this choice-based experimental approach can inform both the current efforts of social movements and expand the scope of future studies of framing, persuasion, and political communication.
Research design
To assess the effects of the #MeToo movement’s message, we build on experimental designs that provide some subjects with the opportunity to choose whether they receive the treatment – in this case, a persuasive argument about the importance of the #MeToo movement and the need for gender equality. Such designs are commonly known in public health as “patient preference trials” (Long, Little and Lin, Reference Long, Little and Lin2008; Rücker, Reference Rücker1989; Torgerson, Klaber-Moffett and Russell, Reference Torgerson, Klaber-Moffett and Russell1996). Similar designs are less common in political science but have been used to assess the effects of negative campaigns and partisan media (Arceneaux, Johnson and Murphy, Reference Arceneaux, Johnson and Murphy2012; de Benedictis-Kessner et al., Reference de Benedictis-Kessner, Baum, Berinsky and Yamamoto2019; Gaines and Kuklinski, Reference Gaines and Kuklinski2011) as well as preferences about policies (Leeper, Reference Leeper2017).
The stages of our design are presented in Figure 1. First, we told subjects that we were interested in their opinions about the #MeToo movement. After reporting their familiarity with the movement, subjects were randomly assigned to one of two design conditions (Stage 1). Approximately 40% of subjects were assigned to the experimental design in the top branch of Figure 1 where they were then randomly assigned to treatment or control (Stage 2a). Treated subjects read a brief persuasive argument attributed to the pictured “Joan” designed to convey the importance of the #MeToo movement and the broader need for gender equality in society. Footnote 2 Control subjects received no additional information.

Figure 1 Triply Randomized Parallel Design.
NOTES: It outlines the three stages of our design. In the first stage, subjects are randomly assigned to either an experimental condition, in which treatment is then randomly assigned (Stage 2a), or a choice condition in which subjects have the opportunity to select into or out of treatment (Stage 2b). Subjects opting to avoid treatment are then randomly assigned either to receive no further information or to receive the same information attributed to either a different woman or a man (Stage 3).
Subjects randomly assigned to the bottom branch of Figure 1 (Stage 2b) were first asked: before providing your opinions, would you like to hear what “Joan” (pictured) had to say on this issue? Subjects who said yes received the same information described above. Subjects who said no were randomly assigned to one of three conditions (Stage 3): they could receive no further information and proceed directly to answering our outcome measures, or they could be randomly assigned to receive the same information attributed to a different woman (“Jane”) or a different man (“John”). The images associated with Joan, Jane, and John come from the Chicago Face Database (Ma, Correll and Wittenbrink, Reference Ma, Joshua and Wittenbrink2015) and were chosen to have similar facial features.
We adapt the notation of Knox et al. (Reference Knox, Yamamoto, Baum and Berinsky2019) to describe our design: Let
${D_i} \in \{ 0,1\} $
denote whether a subject is assigned to the experiment (
${D_i}=0$
) or choice conditions (
${D_i}=1$
). Let
${C_i} \in \{ 0,1\} $
denote whether subjects in the choice condition choose to receive the treatment (
${C_i}=1$
) or avoid it (
${C_i}=0$
). Finally, let
${T_i} \in \{ {T_{{\rm{Joan}}}},{T_{{\rm{Jane}}}},{T_{{\rm{John}}}},{T_{{\rm{Control}}}}\} $
denote the treatment a subject actually receives, and let
${Y_i}(t)$
correspond to subjects’ potential outcomes.
Following Knox et al. (Reference Knox, Yamamoto, Baum and Berinsky2019), the random assignment of design conditions (
${Y_i}(t),{C_i}, \Bot {D_i}$
) and treatment conditions in the experiment (
$Yi(t),{C_i} \Bot {T_i}|Di=0$
) and the third stage of our design (
$Yi(t), \Bot {T_i}|Di=1,{C_i}=0$
) allow us to identify five causal estimands.
Footnote 3
First, from Stage 2a of Figure 1, we estimate the average treatment effect of a traditional experiment (
$ATE = E(Y|D=0,T = {T_{{\rm{Joan}}}}) - E(Y|D=0,T = {T_{{\rm{Control}}}})$
). Second, as Gaines and Kuklinski (Reference Gaines and Kuklinski2011) show, the ATE reflects the effect of treatment were everyone to receive it and can be thought of as a weighted average of the treatment effects among the proportion,
$\alpha $
, of respondents likely to seek out treatment and those likely to avoid it (
$1 - \alpha $
). Random assignment of design conditions coupled with the random assignment of treatments allows us to estimate what Knox et al. (Reference Knox, Yamamoto, Baum and Berinsky2019) refer to as Average Choice-Specific Treatment Effects (
$ACTE$
). Specifically, by taking the difference between a weighted average of the outcome in the selection condition (
$E(Y|D=1)$
)
Footnote 4
and the average of those in the experimental control (
$E(Y|D=0,T = {T_{{\rm{Control}}}})$
), and dividing this estimate by the proportion of people selecting treatment (
$\alpha $
), we can isolate the effect of the treatment on those likely to seek it out (
$ACT{E_{{\rm{Select}}}}$
).

Similarly, as Gaines and Kuklinski (Reference Gaines and Kuklinski2011) show, we can estimate the treatment’s effect on those likely to avoid it (
$ACT{E_{{\rm{Avoid}}}}$
) as follows:
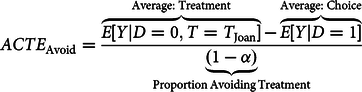
Standard errors for a ratio of estimates are constructed via the delta method (Cameron and Trivedi, Reference Cameron and Trivedi2005).
Finally, using only the responses from Stage 3 of Figure 1, we can estimate two conditional ACTEs (
$CACTE$
) by simply taking the difference of means between subjects assigned to receive the message from either Jane or John and subjects assigned to receive no additional information. The CACTE for the treatment attributed to Jane (
$CACT{E_{{\rm{Female}}}} = E[Y|D=1,C=0,T = {T_{{\rm{Jane}}}}] - E[Y|D=1,C=0,T = {T_{{\rm{Control}}}}]$
) offers a related but distinct estimate to the
$ACT{E_{{\rm{Avoid}}}}$
. It provides insights into the effects of encountering the messages of #MeToo movement from a typical source after explicitly trying to avoid this information. Similarly, the CACTE for the treatment attributed to John (
$CACT{E_{{\rm{Male}}}} = E[Y|D=1,C=0,T = {T_{{\rm{John}}}}] - E[Y|D=1,C=0,T = {T_{{\rm{Control}}}}]$
) assesses the effect of encountering the same message but from an unexpected source, in this case, a man. As we discuss below, the
$CACT{E_{{\rm{Female}}}}$
provides a stronger test of the possibility that the messages of #MeToo could backfire, while the
$CACT{E_{{\rm{Male}}}}$
offers insights into whether an unexpected messenger might be more effective for this particular audience.
Footnote 5
While the added insights of these
$CACTE$
s are appealing, researchers might balk at adding another layer of randomization to an already complicated design. Figure 2 illustrates some of the statistical benefits of randomizing conditional on choice using a series of power simulations discussed in further detail in the appendix. The simulations presented here assume that the effects of treatment are of equal size but opposite signs for those likely and unlikely to receive the treatment, that more people choose to receive the treatment than avoid it, and, as seems likely, that this choice is at least somewhat correlated with attitudes about the movement. While the
$ACTE$
among those avoiding treatment is underpowered (because fewer people in this scenario avoid treatment), the
$CACTE$
s have similar statistical power to the
$ACTE$
among those selecting treatment. While the
$CACTE$
s are estimated off of smaller samples, they gain precision from not having to estimate the variance of a ratio and decreased variance in the outcome due to the correlation between treatment choices and attitudes about #MeToo. In essence, randomizing conditional on choice provides similar benefits to conditioning on covariates or matching on a propensity score, but does so non-parametrically. By leveraging subjects’ observed choices, our design avoids issues that may arise from misspecification and omitted variable bias in more parametric approaches.
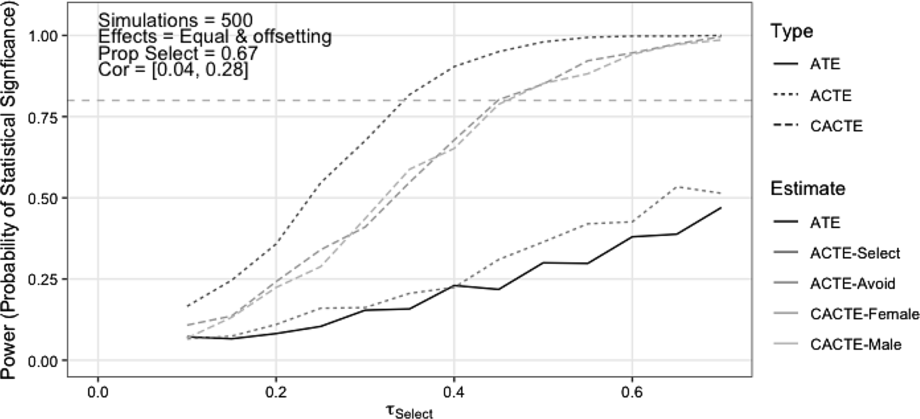
Figure 2 Statistical Power with More Selectors than Avoiders, Equal, and Offsetting Effects.
NOTES: It shows the probability of correctly rejecting a null hypothesis at p<0.05 for each of our estimands, assuming there are more people selecting treatment than avoiding it and that the effects of treatment (τ) are equal in size and opposite in direction.
Expectations
We consider three possible patterns of results.
Footnote 6
First, the baseline scenario is one of the homogeneous effects where the
$ATE$
,
$ACTE$
s, and
$CACTE$
s are all similar. In short, a standard survey experiment would have sufficed. Second, we consider patterns of heterogeneous and offsetting effects. Here, treatment effects are conditional on a subject’s willingness to receive the message. A positive
$ACTE_{} {_{{\rm{Select}}}} $
suggests that the message has its intended effect on its likely audience. Similarly, we interpret a negative or null
$ACT{E_{{\rm{Avoid}}}}$
as evidence of counter-arguing or resistance among those who would avoid #MeToo’s message if given the chance. Depending on the distribution and the size of the treatment effects for these two “types,” the overall ATE may be positive, negative, or non-significant. We expect the
$ACT{E_{{\rm{Avoid}}}}$
to be similar in sign to the
$CACT{E_{{\rm{Female}}}}$
since both receive the same message attributed to a woman, although it is possible that receiving this message after trying to avoid it may produce more backlash or resistance. For the
$CACT{E_{{\rm{Male}}}}$
, it is possible that hearing the same argument from an unexpected source has a more positive (or at least less negative) effect (Berinsky, Reference Berinsky2017). Finally, we assess whether these heterogeneous results depend on characteristics of the subjects themselves. Specifically, we examine whether the
$ATE$
s,
$ACTE$
s, and
$CACTE$
s vary by gender and partisanship.
Footnote 7
Data and measurement
The data for our study come from two samples. First, we conducted a study in September 2018 using Amazon’s Mechanical Turk (MTurk) to recruit 1,137 respondents. Berinsky, Huber and Lenz (Reference Berinsky, Huber and Lenz2012) note that MTurk samples tend to be less representative than national probability samples (though more representative than other convenience samples), because they tend to be younger and more liberal (Huff and Tingley, Reference Huff and Tingley2015). To address these limitations, we fielded a second study in January 2019, with 1,000 respondents from Qualtrics’s online panel recruited via quota-based sampling to be nationally representative by gender, age, education, and race. Footnote 8 Overall, the MTurk sample tends to be younger, more liberal, more Democratic, and more likely to be familiar with the #MeToo movement. The Qualtrics sample is more racially and economically diverse and has more respondents who, when given the choice, opted out of receiving treatment. The primary outcomes for our analysis consisted of a scale constructed from a principal components analysis of three items measured on a 100-point scale tapping specific support for the #MeToo movement. Footnote 9
Study 1: MTurk sample
Given a choice, 81% (N = 573) of our respondents chose to hear what “Joan” had to say about the #MeToo movement while 19% (N = 131) did not. Figure 3 shows how those who selected treatment differ from those who avoided it for the full sample and by gender and partisanship. As we will see below, gender strongly conditions the sign and size of our effects, but is unrelated to exposure. Other factors like partisanship and prior familiarity with #MeToo predict exposure, and, as we show in the appendix, condition the responses of men and women differently. In short, many factors may contribute to heterogeneous patterns of exposure and response. Rather than trying to detect individual sources of heterogeneity from post-hoc subgroup analysis, experiments with choice reveal potential heterogeneity through subjects’ observed choices.

Figure 3 Who is Likely to Seek Out or Avoid the Message of the #MeToo Movement?.
NOTES: It shows the difference in means between subjects who opted into and out of receiving treatment overall (left panel) and separately (right panel) for men (triangles) and women (squares).
Figure 4 presents the results from our design with 90% and 95% confidence intervals from the full sample and then separately for men and women and Republicans and Democrats. Point estimates and confidence intervals are provided in Table 1. Looking at the full sample, the
$ATE$
of reading Joan’s account appears to increase support for the #MeToo movement by 0.22 points (p < 0.05). Among those who would choose to receive this information when given the chance, the effect is also positive (0.19 points) and marginally significant (p < 0.10). Among those who would likely avoid this information, the
$ACTE$
is also positive but with a wide 95% confidence interval that spans a range of values from −0.47 to 1.20. Similarly, the effects of hearing this information from a different woman or a man are also positive, but not statistically significant, although the standard errors of these
$CACTE$
s are about two-thirds the size of the standard error for the
$ACTE$
for those avoiding treatment.

Figure 4 Heterogeneous Effects in the #MeToo MTurk Study.
NOTES: It compares the ATE of our message to the ACTEs of those likely and unlikely to receive it and the CACTEs of avoiding the treatment and then receiving the same information attributed to a different woman or a man.
Table 1 Treatment Effect Estimates on Specific Support for #MeToo (MTurk Sample)
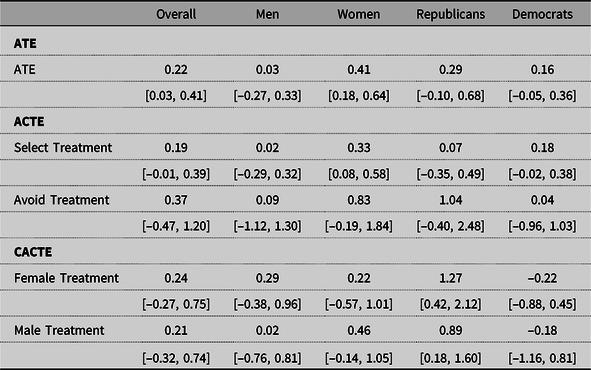
Note: The table provides point estimates and 95% confidence intervals for treatment effect estimated from the full sample and separately by gender and partisanship.
Turning to the results by gender in the center panel of Figure 4, we see that the effects are more evident among women. The
$ATE$
among women is 0.41 (p < 0.05), while among men it is 0.03 (p = 0.83). Among those women who would choose to hear another woman’s opinions, the
$ACTE$
is 0.33 (p < 0.05), while among men, the effect is 0.02 (p = 0.92). The
$ACTE$
among women who would opt out of receiving the message is substantively large (0.83 points) but imprecisely estimated (p = 0.11). Among those who tried to avoid the treatment but received an alternative version, neither male nor female versions of this alternative appear to have much of an effect for men or women. However, when we turn to the
$CACTE$
s estimated separately by partisanship, we see large, positive effects among Republicans who initially opted to avoid the treatment but subsequently received the same information from a man or a different woman. The
$ACTE$
among Republicans avoiding the treatment is similar in sign and magnitude, but less precisely estimated.
Had we only conducted a standard survey experiment, we would conclude that the treatment increased support for #MeToo and that these effects were most evident in the responses of women. Including choice in our design, we learn that most respondents are open to hearing a woman’s perspective on #MeToo when given the choice, and this information appears more resonant for women than men. Randomizing conditional on choice, we see that our treatment appears effective among Republicans who might, otherwise, avoid this information.
Study 2: Qualtrics sample
In our Qualtrics sample, two-thirds of respondents (N = 397) opted to hear what “Joan” had to say, while the other third chose to avoid the message (N = 197). Overall, people open to hearing a woman’s view about the #MeToo movement in this sample had higher levels of income, education, and familiarity with the movement, particularly among men. As with our first study, the results in Figure 5 suggest no single factor explains who is likely to encounter this message and how they will respond.

Figure 5 Who Seeks Out or Avoids the Message of the #MeToo Movement in a More Nationally Representative Sample?.
NOTES: It shows the difference in means between subjects who opted to receive treatment (N = 397) or chose to avoid this information (N = 197) overall (top panel) and for men and women separately (bottom panel).
Figure 6 and Table 2 presents the
$ATE$
,
$ACTE$
s, and
$CACTE$
s of our treatment on specific support for the #MeToo movement again for the full sample and then by gender and partisanship. The
$ATE$
is positive (0.29, p < 0.05). The
$ACTE$
s suggest this effect is most evident for those likely to encounter the treatment (0.24, p < 0.10). The estimates for those unlikely to receive the treatment are similar in sign and magnitude but estimated with less precision. The
$CACTE$
s for the full sample are also non-significant, but suggestive of polarizing heterogeneity. The effect of receiving the treatment after initially opting out has a positive effect when that message is attributed to a man (0.32, p < 0.10), but a negative, non-significant effect when the message is attributed to a different woman (−0.23, p = 0.22). This polarized response becomes more evident once we estimate the separate effects by gender. Again, looking just at the responses of those individuals who opted to avoid treatment but received a similar version, we see that men appear to respond favorably to this information when it is delivered by “John” (0.62, p < 0.05) but have roughly the opposite response when that information comes from “Jane” (−0.48, p < 0.10). We see a similar but more muted pattern of responses in the
$CACTE$
s for Republicans and show in the appendix that this polarized pattern of response is most evident among Republican men.
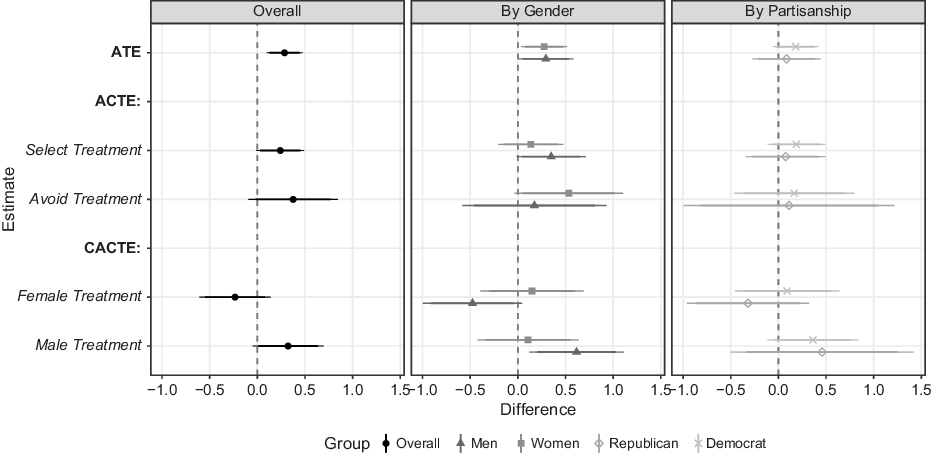
Figure 6 Heterogeneous Effects in the #MeToo Qualtrics Study.
NOTES: It provides the ATE, ACTEs, and CACTEs overall and separately for men and women and Republicans and Democrats in our national sample.
Table 2 Treatment Effect Estimates on Specific Support for #MeToo (Qualtrics Sample)

Note: The table provides point estimates and 95% confidence intervals for treatment effect estimated from the full sample and separately by gender and partisanship.
Discussion
Are movements like #MeToo preaching to the choir or changing hearts and minds? The answer depends both on who receives the message and how they respond to it. Our results suggest that these movements may reach a relatively large audience: when given a choice, two-thirds to four-fifths of subjects chose to receive our treatment. How such audiences respond to a movement’s messages is a more complicated question. Our two studies provide somewhat conflicting results. In our convenience sample, we see evidence of persuasion, particularly among women likely to encounter this message, but also among Republicans who initially avoided the treatment but were subsequently exposed to the same information attributed to a different source. In our more nationally representative sample, the treatment again appears to have the expected effect on its likely audience. However, for men in this sample who chose to avoid our treatment but received a similar message from a different source, the effects appear to depend on the gender of the source. A male increases support for #MeToo while a female source decreases support. Differences in the composition of each sample likely account for some of these divergent results: Subjects in our MTurk study were younger, more educated, and more familiar with the #MeToo movement than respondents to our Qualtrics study, and these differences were larger among men than women in the two studies. Another possible interpretation is that the treatment effects depend on people’s prior information about the #MeToo movement, which varies within groups and across samples. In the appendix, we find that the effects tend to be larger for those who report lower levels of familiarity with the movement.
The takeaway for #MeToo proponents is that both the content and source of their message are likely to matter. While our design focused on the role of source cues in this process, how social movements frame their messages clearly shapes their success (Johnston and Noakes, Reference Johnston and Noakes2005). Our study framed its #MeToo message around facts and statistics related to sexual harassment. Undoubtedly other frames, such as a message about personal experiences with sexual harassment, could have different effects and would likely reach different audiences. While this is a limitation of our experiment, it is an opportunity for future research to incorporate multiple messages into this expanded choice framework.
More broadly, we hope this paper encourages scholars to bring choice and self-selection into the design and analysis of their experiments. As the opportunity to choose what political messages or information we receive increases, researchers must account for this process. While fielding experiments incorporating choice can seem daunting, these designs are more powerful and readily adaptable to the questions scholars care about. Knox et al. (Reference Knox, Yamamoto, Baum and Berinsky2019), for example, show how scholars can estimate effects when subjects are presented with multiple treatment options. Our own work highlights the potential of randomizing conditional on subjects’ choices. Doing so allows scholars to answer more nuanced questions while preserving statistical power. Our design is but one of many ways scholars could accomplish this, and we urge future work to focus on relative tradeoffs between different approaches. Choice is an inherent feature of politics. We hope it becomes a prominent feature of more experimental designs.
Supplementary material
To view supplementary material for this article, please visit https://doi.org/10.1017/XPS.2020.24