Introduction
Rice is one of the most important staple foods in the world and is grown mainly in South and Southeast Asia. Diseases and abiotic stresses coupled with climate change are major constraints to increasing rice production. Farmers lose an estimated average of 0.37 of rice crop due to major pests and diseases every year. Among these pests, rice tungro disease (RTD) can cause total crop failure, especially in several countries in South and Southeast Asia (Bajet et al., Reference Bajet, Aguiero, Daquioag, Jonson, Cabunagan, Mesina and Hibino1986; Hibino, Reference Hibino1996). This disease is caused by rice tungro spherical virus (RTSV), which acts as a helper virus for the transmission of rice tungro bacilliform virus. Rice tungro spherical virus has a polyadenylated single-stranded RNA genome of about 12 kb that is encapsidated within isometric particles. The genome encodes a single large open reading frame of 3473 amino acids, and although RTSV strains cannot be distinguished serologically, they do differ significantly at the molecular level (Azzam and Chancellor, Reference Azzam and Chancellor2002).
There are several approaches and methods employed in addressing these challenges: use of resistant or tolerant varieties, adjustment of planting strategies and changes in area planted, etc. Development of resistant varieties is one of the top approaches in coping with various stresses (Gregorio et al., Reference Gregorio, Senahidra, Mendoza, Manigbas, Roxas and Guerta2002; Das and Rao, Reference Das and Rao2015; Ali et al., Reference Ali, Xu, Gao, Ma, Meng, Wang, Pang, Guan, Xu, Revilleza, Franje, Zhou and Li2017; Kumar et al., Reference Kumar, Devi, Sharma, Ansari, Phurailatpam, Ng, Singh, Prakash, Kumar, Kumawat, Mandal and Kumar2017; Shamim and Singh, Reference Shamim and Singh2017). This involves backcrossing the source parent to susceptible varieties (recurrent parent) that have desirable properties and selecting resistant plants. These donor parents could come from existing sources or could be from the vast germplasm being stored in genebanks, which depends on the availability of information. This information could be the basis for breeders to determine the possible source of resistant traits in the next breeding programme. Hence, it is important to evaluate genetic resources using faster and more reliable preliminary processes. Molecular marker technology has been developed to study the molecular characterization of a range of crops (Das and Rao, Reference Das and Rao2015; Bhagwat et al., Reference Bhagwat, Gokhale, Sawardekar, Kelkar, Kambale and Kunkerker2017; Shamim and Singh, Reference Shamim and Singh2017).
Morphological markers were used first to study the diversity of species and then isozyme markers were used. However, with the development of modern biotechnology in recent years, DNA molecular markers have gradually become the main tool for studying genetic diversity (Yun et al., Reference Yun, Zhong, Wang, Wei, Hu and Qian1998; Ravi et al., Reference Ravi, Geethanjali, Sameeyafarheen and Maheswaran2003; Nybom, Reference Nybom2004; Li et al., Reference Li, Zhang, Gao, Smulders, Ma, Liu, Nan, Chang and Qiu2009; Huang et al., Reference Huang, Wu, Tao, Liu, Yang and Chen2015; Chen et al., Reference Chen, He, Nassirou, Nsabiyumva, Dong, Adedze and Jin2017). A range of molecular approaches is used to study the diversity in conserved germplasm (Ashraf et al., Reference Ashraf, Husaini, Ashraf Bhat, Parray, Khan and Ganai2016). Molecular markers are also important for the characterization of germplasm and offer opportunities to delineate genetic differences at the molecular level among the available diversity. Among the molecular markers, microsatellite markers, also known as simple sequence repeats (SSR), are most useful in the characterization of cultivars due to their reproducibility, multi-allelism, co-dominant inheritance and good genome coverage, allowing genetic discrimination even in related cultivars (Guichoux et al., Reference Guichoux, Lagache, Wagner, Chaumeil, Léger, Lepais, Lepoittevin, Malausa, Revardel, Salin and Petit2011). These markers have demonstrated a potential to detect genetic diversity, management of plant genetic resources and molecular screening of germplasm against biotic stresses (Virk et al., Reference Virk, Zhu, Newbury, Bryan, Jackson and Ford-Lloyd2000; Song et al., Reference Song, Liu, Tolia, Schneiderman, Smith, Martienssen, Hannon and Joshua-Tor2003; Sabar et al., Reference Sabar, Bibi, Farooq, Haider, Naseem, Mahmood and Akhter2016). They have also used successfully in the selection of distinct genotypes for breeding through marker-assisted selection, in order to increase the number of recombination events, resulting in new allelic combinations and a greater chance of obtaining superior genotypes (Sabar et al., Reference Sabar, Bibi, Farooq, Haider, Naseem, Mahmood and Akhter2016). The current study presented a fast-molecular screening approach using gene-specific SSR markers linked to the tsv1 gene, which confers resistance to RTSV and is one of the viruses that cause RTD. The study aimed to evaluate the use of tsv1 resistance linked SSR for the preliminary screening of Philippine traditional rice germplasm against RTSV.
Materials and methods
Plant materials
Accessions (103) were chosen randomly from the traditional rice varieties (TRVs) in the PhilRice-Genebank (Table 1). Leaf samples were collected in all accessions that were grown in the field in 2015 dry season in 1 × 1.5 m2 without replication at the Philippine Rice Research Institute, Maligaya, Muñoz, Nueva Ecija, the Philippines (N,15°40′E, 120°53′, 64.5 m a.s.l.).
Table 1. List of rice accessions used in the current study

Fresh leaf samples were used to extract the genomic DNA with the use of the modified Cetyl Tri-methyl Ammonium bromide (CTAB) method (Murray and Thompson, Reference Murray and Thompson1980). The quality and concentration of DNA were estimated by using Nanodrop spectrophotometer (Thermo Scientific, Inc., Waltham, MA, USA) and DNA was diluted in Tris-ethylenediaminetetraacetic acid (Tris-EDTA) buffer and stored at −4 °C prior to polymerase chain reaction (PCR) analysis.
Polymerase chain reaction analysis
Eleven SSR primers, linked with the tsv1 gene that confers resistance to RTSV (Lee et al., Reference Lee, Muhsin, Atienza, Kwak, Kim, De Leon, Angeles, Coloquio, Kondoh, Satoh, Cabunagan, Cabauatan, Kikuchi, Leung and Choi2010), were used from chromosome number 7. All primers were synthesized by 1st Base (Singapore) according to sequence information obtained from Gramene (http://www.gramene.org, Table 2). The PCR amplifying procedure used the protocol of McCouch Laboratory (Cornell University, Ithaca, NY, USA) with slight modifications: (i) initial denaturation at 94 °C for 5 min; (ii) denaturation at 94 °C for 1 min; (iii) annealing at temperature from 55 to 60 °C (based on specified annealing temperature) for 1 min; (iv) extension at 72 °C for 2 min; (v) repeat steps (ii) to (iv) 34 times; and (vi) then final extension at 72 °C for 7 min. The amplified products were stored at 4 °C. The DNA amplification was carried out with a total of 4.1 µl reaction containing 0.2 µm of each primer, 20 µm of deoxyribonucleotide triphosphate (dNTPs), 1.5 µ 10X PCR buffer, 1.5 mm magnesium chloride (MgCl2), 50 ng of DNA template and 0.5 unit of i-Taq DNA polymerase (Intron Biotechnology, Inc., South Korea). The amplified products and standard molecular weight markers 1Kb Plus (ThermoFisher Scientific, Massachusetts, US) were run on 8% non-denaturing polyacrylamide gel at 100 V, 500 mA for 75 min. The gel was stained with GelRed™ according to the manufacturer's protocol (Biotium, Inc., California, USA) and then observed under an ultraviolet transilluminator (Bio-Rad Laboratories, UK).
Table 2. Primers used in the current study linked to tsv1 genes in rice
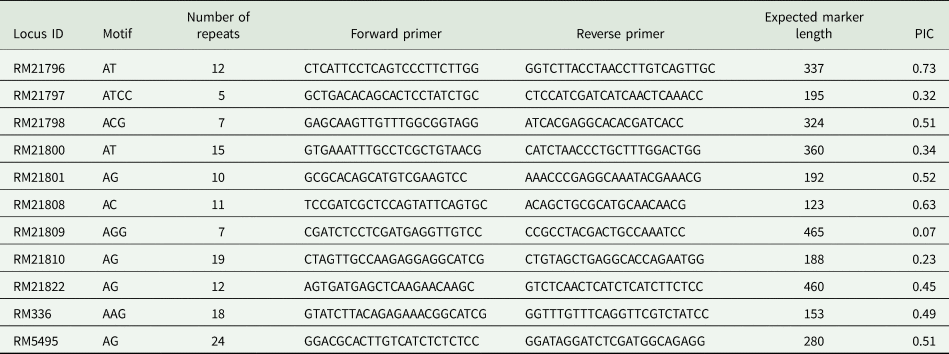
PIC, polymorphism information content.
Data analysis
The polymorphic SSR bands for each accession were scored individually for the presence or absence of the expected bands. This resulted in binary data of 1s and 0s. The polymorphism information content (PIC) was determined for each SSR locus using GenAlEx 6.5 (Peakall and Smouse, Reference Peakall and Smouse2012). Both matrices were then analysed using the Numerical Taxonomy and Multivariate Analysis in the statistical package NTSYSpc version 2.2 (Rohlf, Reference Rohlf2005). The data matrices were used to calculate genetic diversity based on Nei's coefficients, and one dendrogram displaying relationships among the 103 rice accessions was constructed using the Unweighted Pair Group Method with Arithmetic Mean (UPGMA) (Sokal and Michener, Reference Sokal and Michener1958). Principal coordinate analysis (PCA) was done to visualize individual and/or group differences using GenAlEx 6.5 (Peakall and Smouse, Reference Peakall and Smouse2012).
Results
Markers used in the study consisted of two-, three- and four-nucleotide repeat motifs ranging from 5 to 24 repeats (Table 2). Allele sizes ranged from 123 to 465 with PIC values ranging from 0.07 to 0.73 for the 11 SSR markers. The lowest PIC value was observed for RM21809 (0.07) while the highest was recorded for RM21796 (0.73). The average PIC value was 0.43. Informative markers with PIC values >0.5 were RM21796, RM21798, RM21801 and RM5495, with a range of 0.51–0.73 (Table 2).
All 11 markers showed polymorphism between 103 TRVs. A total of 30 bands were scored. The study revealed that primers RM336 and RM337 had four alleles each compared with the rest of the markers used (Fig. 1). Cluster analysis was used to group the varieties and construct a dendrogram. The Nei's coefficient from the similarity matrix was based on the UPGMA algorithm. A total of six major clusters at a 50% genetic distance coefficient were generated. Three clusters (Clusters D, E and F) had individual accessions, one (Cluster C) had three accessions while another cluster (Cluster B) had 56 accessions and lastly, one cluster (Cluster A) had 41 accessions (Fig. 2). There is no conclusive revelation among the germplasm in this cluster. The clustering revealed variation among the accessions in terms of the presence and absence of alleles based on the 11 tsv1-linked loci. From six major clusters, the PCA showed only three major clusters which could mean that these TRVs could be resistant, moderately resistant and susceptible (Fig. 3). The highest similarity was revealed between Buwa and Binakayo (100%) and the least between Binorsige and Binaka (<10%).

Fig. 1. Representative picture of a non-denaturing 8% polyacrylamide gel with RM336 showing four alleles with the 1 kb+ ladder as control, with some accessions showing similar banding patterns such as 1, 2 and 8 (253 bp); 3 and 4 (550 bp/201 bp); 5, 6, 9 and 10 (253 bp/201 bp). The bands represent the alleles with the corresponding sizes.

Fig. 2. Simplified UPGMA clustering of 103 O. sativa TRVs based on 11 tsv1-linked SSR markers.

Fig. 3. Principal coordinate analysis of 103 TRVs based on 11 tsv1-linked SSR markers showing distinct groupings.
Discussion
High similarity was revealed between Buwa and Binakayo (100%), which could be a possible duplicate, but since low-coverage SSR markers were used, based only in the 200 kb region, no conclusions about their relationship can be drawn. However, it could be stated from the current molecular analysis that these two TRVs are similar in their resistance to RTSV.
Lee et al. (Reference Lee, Muhsin, Atienza, Kwak, Kim, De Leon, Angeles, Coloquio, Kondoh, Satoh, Cabunagan, Cabauatan, Kikuchi, Leung and Choi2010) observed RM336 is expected to have five alleles based on the recombination that occurred at that locus. However, the current study found four alleles only for RM336 and RM337, which could be due to differences in the accessions used. Lee et al. (Reference Lee, Muhsin, Atienza, Kwak, Kim, De Leon, Angeles, Coloquio, Kondoh, Satoh, Cabunagan, Cabauatan, Kikuchi, Leung and Choi2010) used TN1 and Utri Merah crosses while the current study selected Philippine TRVs, which revealed more alleles than the TN1 × Utri Merah crosses. According to Schiff et al. (Reference Schiff, Wilson and Somerville2001), Lindhout (Reference Lindhout2002) and Ghosh et al. (Reference Ghosh, Sharmin, Islam, Pahloan, Islam and Khan2010), most disease resistance traits are controlled by multiple genes. These traits could involve many genes and environmental factors. Resistance indicated by molecular analysis still needs to be correlated with phenotypic evaluation (Keka et al., Reference Keka, Shamsuzzaman, Pahloan, Pervin, Rhaman and Khan2008).
In order to dissect the different genes, it is imperative that there should be a preliminary screening of germplasm in genebanks that is fast enough to support breeding new varieties with improved traits (2016 National Rice R&D Highlights). Various studies on genetic diversity in rice have found medium to high diversity in genebanks (Fuentes et al., Reference Fuentes, Escobar, Alvarez, Gallego, Duque, Ferrer, Deus and Tohme1999; Chakravarthi and Naravaneni, Reference Chakravarthi and Naravaneni2006). In the current paper, information from the analysis will be used to provide relevant information on the resistant germplasm stored in the genebank and its use in breeding for RTSV-resistant varieties. There have been several studies that have used molecular markers with emphasis on determining genetic diversity (Ni et al., Reference Ni, Colowit and Mackill2002; Ravi et al., Reference Ravi, Geethanjali, Sameeyafarheen and Maheswaran2003; Chakravarthi and Naravaneni, Reference Chakravarthi and Naravaneni2006; Thomson, et al., Reference Thomson, Septiningsih, Suwardjo, Santoso, Silitonga and McCouch2007; Rahman et al., Reference Rahman, Sohag and Rahman2010; Sajib et al., Reference Sajib, Hossain, Mosnaz, Hossain, Islam, Ali and Prodhan2012; Ashraf et al., Reference Ashraf, Husaini, Ashraf Bhat, Parray, Khan and Ganai2016). On one hand, there are studies that have looked into genetic diversity in terms of responses of different rice cultivars to abiotic stress such as salinity (Kanawapee et al., Reference Kanawapee, Sanitchon, Srihaban and Theerakulpisut2011), iron toxicity (Onaga et al., Reference Onaga, Egdane, Edema and Abdelbagi2013), drought (Anupam et al., Reference Anupam, Imam, Quatadah, Siddaiah, Das, Variar and Mandal2017) and aerobic condition tolerance (Singh and Sengar, Reference Singh and Sengar2015). On the other hand, very few studies have utilized molecular markers to showcase diversity such as bacterial leaf blight (Sabar et al., Reference Sabar, Bibi, Farooq, Haider, Naseem, Mahmood and Akhter2016) and blast-linked markers (Anupam et al., Reference Anupam, Imam, Quatadah, Siddaiah, Das, Variar and Mandal2017). The current study looked into the diversity using tsv1-linked markers that are involved with resistance to RTSV.
Ravi et al. (Reference Ravi, Geethanjali, Sameeyafarheen and Maheswaran2003) reported that SSR analysis resulted in a more definitive separation of clusters of genotypes, indicating a higher level of efficiency of SSR markers for the accurate determination of relationships between accessions. Chakravarthi and Naravaneni (Reference Chakravarthi and Naravaneni2006) supported this finding in their paper on genetic diversity and DNA fingerprinting of 15 elite rice genotypes using 30 SSR primers: distinct polymorphism was found among the cultivars studied, indicating the robust nature of microsatellites in revealing polymorphism. This polymorphism gives precise information about the extent of genetic diversity which helps in the development of an appropriate breeding programme. Sajib et al. (Reference Sajib, Hossain, Mosnaz, Hossain, Islam, Ali and Prodhan2012) found PIC values ranging from 0.14 (RM510) to 0.71 (RM163) in all loci, with an average of 0.43, similar to the findings of the current study (0.70–0.73). Rice genetic improvement depends mainly on the extent of variability present in the population. Molecular markers are useful tools in the assessment of genetic variation in terms of non-genic and genic markers. The current study presented the ability to use molecular markers linked to a specific gene of interest. The information could provide a fast and robust preliminary evaluation of rice germplasm using tsv1-linked SSR markers. The availability of these data will provide guidance to future breeding activities for RTSV resistance varieties. The study explored and was successful in the application of SSR markers for screening of the presence and diversity of resistance genes present in the germplasm. Such information will also provide a faster data turn-over to the database containing relevant information on the germplasm kept in genebanks.
Author ORCIDs
X. G. I. Caguiat, 0000-0002-5020-5704.
Acknowledgements
The author is grateful to the help of Mr Jelvince Patricio, the people of the Molecular Genetics Laboratory for the efficient laboratory activities and the contributor of the TRVs being kept at PhilRice Genebank.
Financial support
The author thanks the Philippine Rice Research Institute under the Research Fellowship grant as the funding source.
Conflict of interest
None.
Ethical standards
Not applicable.