Introduction
The explanatory standing of Standard Qualitative Comparative Analysis (hereafter, QCA: Ragin, Reference Ragin2014, Reference Ragin2000, Reference Ragin2008; Rihoux and Ragin, Reference Berg-Schlosser, De Meur, Rihoux and Ragin2009) seldom proves plain. Recently, Møller and Skaanig (Reference Møller and Skaaning2019: 81) maintained that QCA and set-theoretic methods ‘provide little in the way of distinguishing between logical and causal relationships’. Munck (Reference Munck2016: 777) clarified that ‘causation is not a logical relation but, rather, a relation between events or, more precisely, between changes in the properties of things’ and, as such, ‘must be understood ontologically’ – which he deemed beyond QCA. Although ‘causal theories should be built with due attention to the rules of logic, both qualitative and quantitative researchers are better off presenting their causal arguments in the general form ΔX → ΔY, meaning that a change in property X causes a change in property Y, and treating the covariation between X and Y as an essential means for checking whether their causal arguments are true’. In the social and political domains, convincing covariational models identify mediation effects (e.g. Seawright, Reference Seawright2019: 33) – which, once again, QCA would be unable to render.
Against this backdrop, the article addresses the question of whether Standard QCA as a technique is ill-equipped to contribute to causal knowledge. It considers the specificity of its analytic strategy in three sections. The first summarizes the state of the art in causal analysis, and brings attention to the move from design to models that the Structural Causal Model framework has developed to improve the counterfactual approach. In light of that move, the second section portrays QCA as a technique equipped for specifying models with credible explanatory import, with special diagnostics for omitted factors, irrelevant additions, and confirmation bias. The third section illustrates how the causal structures of the Structural Causal Model framework can illuminate the relationship between explanatory configurational findings. The conclusions highlight the special contribution that the explanatory usage of QCA can bring to the methodological agenda on causation in the social and political sciences.
The issues with causation as the response to a stimulus
The Potential Outcome framework (hereafter, PO: Rubin, Reference Rubin1974, Reference Rubin1978; Rosenbaum and Rubin, Reference Rosenbaum and Rubin1983; Imbens, Reference Imbens2004; Morgan and Winship, Reference Morgan and Winship2015) provides the default choice for analyzing causal relationships. The framework narrows on a stimulus T with two mutually exclusive states, either realized (1) or not (0). T is recognized as a causal effect when the claim stands that any i-th unit under the realized stimulus T(1) returns a response Y i(1) that would not have been yielded under the unrealized stimulus T(0). The claim that the difference between the actual response Y i(1) and its counterfactual Y i(0) gauges the causal effect lies on a design that ensures statistically comparable samples of units except for the state of T. The design circumvents a reality in which each unit instead comes as a specific bundle of covariates u i that blur comparability and make the calculation of the effect inaccurate.
Rubin's canon conventionally conceives of covariates as features that are exogenous to the stimulus–response relationship, yet bias the ‘unit's propensity to receive’ T i(1) through some ‘mechanism of self-selection’ that the analysis holds as unknown. The experimental setting, the argument goes, rules out this bias by randomly exposing the units to the realized or the unrealized stimulus. Together, randomization and forced exposition defuse the self-selection mechanism and license two key tenets about the average net effect: first, the covariates do not confound it; second, the assignment mechanism bias is (strongly) ignorable. Beyond randomization, the canon maintains that unconfoundedness and strong ignorability can still be ensured by discounting the units' heterogeneity through their propensity score. Thus, estimations of the average treatment effect become valid on units that display a comparable propensity to self-select into the treatment.
Despite the efforts to make propensity scores as little ‘model-dependent’ as possible, the strategy adopted to deal with units' natural heterogeneity decides the credibility of the causal claim in observational studies and raises a modeling issue. In their structural rendering of the potential outcome, Winship and Morgan (Reference Winship and Morgan1999: 668 ff) define the issue by decomposing the error term u i of the individual response Y i to the stimulus T i into $u_i = u_i^{T0} + T_i( {u_i^{T1} -u_i^{T0} } )$. Their decomposition emphasizes that the set of covariates $u_i^{T1}$
, featuring the group under T i = T(1), is possibly different from that of the baseline group $u_i^{T0}$
. Moreover, they consider that the value of the assignment mechanism T i ultimately depends on two sources of self-selection bias: the set Z i of observed exogenous variables, and the set v i of unobserved or missing variables. When the ‘selection on the observables’ occurs, the propensity score Pr(Z i) can license the assumptions of unconfoundedness and ignorability of the assignment. Under the ‘selection on the unobservables’, instead, estimates require further assumptions about the shape of the unobservables and, eventually, lower interpretability.
The key issue, in short, remains how to ensure that the relevant covariates are identified so that the unobservables only contain irrelevant ‘noise’. To address it, the Structural Causal Model framework (hereafter, SCM: Pearl and Paz, Reference Pearl, Paz, Duboulay, Hogg and Steels1987; Pearl and Verma, Reference Pearl, Verma, Allena, Fikes and Sandewall1991; Pearl, Reference Pearl2009; Bareinboim and Pearl, Reference Bareinboim and Pearl2016; Pearl and Mackenzie, Reference Pearl and Mackenzie2018) invites researchers to shape the setting of counterfactuals along the lines of Simon (Reference Simon1977).
While working on the definition of political power, Simon noted that conventional representations via single equations could not render the intuition of a relationship between two agents, A and B, such that A's preferences cause B's choices while the converse does not hold. To him, power relationships unveiled the intrinsic asymmetry of any causal relationship and called for a decomposition. ‘To say that X is a cause of Y is to say that there is a certain order in which the equations must be solved – specifically, that we must first solve for X and insert its value in another equation which we then solve for Y. Correspondingly, to say that P causes Q is to say that we have a set of propositions (Boolean equations) such that we first determine the truth or falsity of P from some subset of these, and then use the truth value of P to determine the truth value of Q’ (ivi: 50, notation adapted).
The SCM trades design for models that render structural assumptions of dependence. Any structural model consists of three sets – the exogenous variables ${\bf {\cal U}}$, collecting the covariates; the endogenous variables ${\bf V} \colon \{ X, \; Y, \; Z, \; \ldots \}$
, selected from ${\bf {\cal U}}$
as the causal model of the response; and the functions ${\bf F} \colon \{ f_{XZ} , \; f_{XY}, \; f_{YZ}, \; \ldots \}$
that connect them. The model is then ‘augmented’ by a causal graph $ {\cal G}_{M}$
in which variables are ‘nodes’, functions are (missing) directed arrows or ‘edges’, and any consecutive edges build a ‘path’ between nodes. The outcome becomes the endpoint of at least one path whose structure conveys the mechanism of data-generation – the ‘mechanism’ being the type of process to the outcome that the equation captures in some aspect of interest (Simon, Reference Simon1977: 115). Then, the SCM framework identifies three ideal graphs to which any causal structures can be reduced – namely, the linear chain ${\cal G}_{l}$
, the fork ${\cal G}_{f}$
, and the collider ${\cal G}_{c}$
.
The linear graph ${\cal G}_{l}$ entails four key relationships: of dependence of Y from Z; of dependence of Z from X; of ‘likely’ dependence of Y from X when the connecting functions grant transitivity; and, more important, of independence of Y on X conditional on Z.


The relationships in $ {\cal G}_{l}$ convey that two nodes arranged in a linear chain are independent conditional on the mediator in between that captures the whole of the relevant variation. Effective mediators, then, support the claim of a causal connection between X and Y – by dissolving it.
The second prototypical shape is the fork. Two variables, X and Y, both descend from a third variable, Z – as in ${\cal G}_{f}$. In it, Z, Y are dependent; Z, X are dependent; Y, X are likely dependent and again become independent when conditioned on Z.


The fork renders the confounder that makes the dependence of Y on X spurious and, again, explains it away. It shares all the features of the chain except the dependence between X and Z, which in ${\cal G}_{f}$ runs in the opposite direction.
The last fundamental shape is the collider ${\cal G}_{c}$. In it, X and Y together determine the values of Z: thus, X, Z are dependent; Y, Z are dependent; X, Y are independent but display a dependence when conditioned on Z.


The collider portrays the situation in which the output has two inputs. The shape makes knowing the value of the one input irrelevant when we know the value of the other and the output. Beyond that, a collider does not establish any backward causation – instead, it provides a further reason to handle the relationship of correlation and causation with caution.
In SCM, the three basic shapes with their sets of conditionalities offer criteria to accept or reject hypotheses about the relevant covariates beneath an outcome. The acceptable hypothesis may not be univocal, though, as graphs with the same edges and ‘v-structures’ arise the same testable implications, hence belong to the same equivalence class. Mostly, they offer ‘inference rules for deducing which propositions are relevant to each other, given a certain state of knowledge’, and conceptual tools to develop models where ‘knowing z renders x irrelevant to y’ (Pearl and Paz, Reference Pearl, Paz, Duboulay, Hogg and Steels1987). Against this backdrop, the covariates are relevant that turn dependence into independence and vice versa (Kuroki and Pearl, Reference Kuroki and Pearl2014).
Modeling complex conditionality
Explanatory QCA, too, is interested in the bundle of relevant factors that, together, make the ‘stimulus’ effective and, hence, account for it. The viewpoint is slightly different, however.
The strategy follows Mackie (Reference Mackie1965, Reference Mackie1980) in observing that, although we usually explain the burning of a house by a short-circuit, this explanation is ‘gappy’ unless we bring specific background features to the fore. The short-circuit initiated the fire because, for instance, it fired a spark on an oily rag, and there was enough oxygen in the room, and the sprinklers were broken. Thus, the causal response only takes place under the right conditions beyond the initiating factor. Moreover, the conditions and the ‘initiating’ factor stand on an equal footing. ‘Cause’ is just a conventional label for the anomaly in the field that attracts our attention: had the fire followed from a gas leak, we would have mentioned it instead of the sparkle. Besides, different particular conditions can unleash the same type of outcome in equivalent situations: a burning match or enough pressure would have done the same job as the spark. If we abstract the details away, eventually fire can be reduced to the consequence of ‘heating’, and ‘combustible’, and ‘oxygen’ being in the same place at the same time under ‘no impediments’. The abridged formula pinpoints the types of elements that, together, sort the same type of effect irrespective of further features of the context. Hence, such a formula is complete enough to travel and, once that the labels are properly assigned to actual things and events, specific enough to allow us to expect and explain the outcome at any timepoint and place.
The hallmark of this perspective lies in the relationship that the elements entertain with each other and their consequent. That any of them is in the right state is insufficient to the outcome: the causal power lies in their compound. Each of them, however, is non-dispensable: a component in the wrong state makes the compound fail. In turn, the compound is sufficient for the outcome, but its occurrence is an unnecessary event that only happens when all the components are properly arranged. In Mackie's terms, then, each component is a partial, inus cause – an insufficient yet necessary part of an unnecessary yet sufficient compound.
Cartwright (Reference Cartwright and Jacobs2017) enhances the understanding with a functional account that makes further sense of the compound. She assumes the entities in the world ground capacities to do some job, such as, operate a change or preserve stability. Capacity, then, is a concept close to those of ‘potential’, ‘disposition’, ‘tendency’ in other accounts, and related to that of ‘causal power’. It entails a productivity that relationships of sheer association do not display: it is only at the intersection of the right capacities that something happens. The compound of these right capacities arises a ‘nomological machine’ – that is, a ‘sufficiently stable arrangement’ (Cartwright, Reference Cartwright and Jacobs2017) that makes a realization certain until all the relevant elements remain in the right state. Working nomological machines make sure that anything else is irrelevant before the same type of outcome across time and space. Neurons, engines, scientific labs are illustrations of these arrangements. Their relevant components can be understood as inus factors that, in the right team, perform basic tasks such as triggering (${{T = \{{T_1, T_2, {\ldots} \}}}}$), enabling (${{E = \{{E_1, E_2, {\ldots} \}}}}$
), and shielding (${{S = \{{S_1, S_2, {\ldots} \}}}}$
) the special process to a state of an outcome.
Thus, a basic inus model can read ${\it{T \cap E \cap S \to Y}}$ (${\cap}$
reading ‘and’, → ‘is sufficient to’). With binary variables, the probabilistic illustration of this model would portray head (1) and tail (0) flips from three coins (t, e, s), and an unmodeled endogenous data-generation process ensuring a bell (y) always rings (1) when all the three coins land on head, else leaving it silent (0). This model arises a finite sample space with eight possible realizations, ${\rm \Omega }_{{{TES}}} = \{ {TES} , \;{TE\bar{S}} , \; {T\bar{E}S} , \; {T\bar{E}\bar{S}} , \; {\bar{T}ES} , \; {\bar{T}E\bar{S}} , \; {\bar{T}\bar{E}S} , \; {\bar{T}\bar{E}\bar{S}} \}$
. Of them, the first only, ω 1 := tes, yields y given the mechanism in place. QCA dubs the sample space ‘truth table’ and the realizations ‘primitive configurations’, but still understands them as possible states of the world and sets or partitions of the universe of reference ${\cal U} = \{ {{\it u}_1, \;\ldots , \;{\it u}_n} \}$
.
The peculiarity of QCA lies in its eliminative nature. Its solutions arise from the dismissal of logically irrelevant conditions from sufficient primitives (Ragin Reference Ragin2014: 125 ff; cfr. Thiem, Reference Thiem2019). In itself, hence, pruning cannot determine causality. However, its protocol can be geared to probing the claim that a selection of conditions is a credible inus machine (Mackie, Reference Mackie1980: Appendix) when
(a) the same bundle of conditions accounts for both states of the outcome without contradictions;
(b) the solutions of each outcome are properly specified to their respective subpopulation.
Requirement (a): establishing sufficiency for explanatory purposes
As a technique, QCA relies on an algebra of set that preserves the equivalence to a first-order logic (Stone, Reference Stone1936). Thus, the technique addresses Simon's knowledge problem – the determination of the truth or falsity of P – as the problem of gauging a unit's membership in the set of things that are or have P (e.g. Sartori, Reference Sartori and Id1984; Ragin, Reference Ragin2008; Goertz and Mahoney, Reference Goertz and Mahoney2012; Ragin and Fiss, Reference Ragin and Fiss2017). QCA affords two such gauges: crisp and fuzzy. The crisp gauge assigns binary truth values in line with the Boolean canon: the 0-membership in p means 1-membership in the negated set ${{\bar{P}}}$. The fuzzy gauge refines the assignment with that which, from an explanatory perspective, can be understood as an ambiguity penalty. Fuzzy scores incorporate such a classification error in the value assigned to each unit. They span from 0.00 to 1.00 and have their point of highest ambiguity at 0.50: thus, units scoring 0.50 are instances of neither p nor ${{\bar{P}}}$
; units with extreme values, instead, are sure instances of either a set or its negation.
The difference in gauging slightly changes the operationalization of the three logical axioms on which QCA can build valid causal claim. As summarized in Table 1, the three rules establish, respectively, the negation of a state as its arithmetic complement in the universe of reference; the rule of non-contradiction as the impossibility for the same unit to be in a state and its negation at the same time; the rule of the excluded middle as the necessity of a unit to display either one or the other of the two possible states. These gauges allow enforcing the conventional understanding of sets as ‘conceptually uniform’ partitions of the universe with respect to a condition's state.
Table 1. Axioms, set renderings, and gauges

Notes: ψ cs is for the crisp-set membership score, ψ fs is for the fuzzy-set membership score.
$\psi _{P_i}$refers to membership score that the i-th unit from a universe ${\cal U}$
of size N takes in the set of instances sharing the condition P in a state; $\psi _{{\bar{P}}_i}$
is the membership score of the same unit in the set of the condition in the negated state.
The overbar here reads ‘not’. The alternative notation in QCA is the curl ~, or the lowercase set name.
The backlash \ indicates the set difference.
$\cup$ reads ‘union’ and corresponds to the logical inclusive (weak) ‘or’. The alternative logical notation is the vee ∨; in QCA's applications, it is common to use the plus sign +.
$\cap$ reads ‘intersection’ and corresponds to the logical ‘and’. The alternative logical notation is the wedge ${\wedge}$
; in QCA's applications, the operator is a dot (⋅) and often omitted.
$\emptyset$ indicates the empty set; the corresponding logical notation is the empty curly braces. QCA conventionally renders it with a 0, although 0 is also assigned to the ‘fully out’ observed instance.
The construction of sets as uniform partitions provides the ground of the set-theoretical gauge of sufficiency. The relationship is, quite conventionally, a conditional. The parameter that captures it in any QCA application is the ‘consistency of sufficiency’ (S.cons for short: Ragin, Reference Ragin2000, Reference Ragin2008; Duşa, Reference Duşa2018). The parameter is defined as the ratio of the size of the intersection of the outcome and a primitive, and the size of the primitive itself: $S.cons_{\omega _j{\it{\subset Y}}} := \vert {\omega_j {\it{\cap Y}}} \vert / \vert {\omega_j} \vert$. It closely recalls Kolmogorov's measurement of conditional probability as the ratio of success to trials of a certain kind (e.g. Hájek, Reference Hájek, Bandyopadhyay and Forster2011). Indeed, without any loss, the $S.cons_{ \omega _j {\it{\subset Y}}}$
can be rewritten as π(y|ω ji), being π the size of the sets; in the special case of crisp QCA, the size of the set is the number of its instances and Kolmogorov's conditional probability coincides with the consistency of sufficiency. In both cases, moreover, the parameter renders the long-honored regularity criterion that ‘if ω j causes y, then any instance of ω j is an instance of y’; at the same time, it accounts for the challenge that contradictory evidence rises to the modal understanding of causal regularity as ‘if ω j causes y, it cannot be the case that an instance of ω j is an instance of ${{\bar{Y}}}$
’.
The regularity claim of the S.cons stands when the parameter takes either its highest value of 1.00 or its lower value of 0.00, indicating that the primitive draws a uniform partition of the positive or the negative outcome set. For explanatory purposes, violations of this subset relationship make the primitive ‘contradictory’. Contradictions weaken the claim that the team of conditions renders an inus machine, and suggest that the team is ill specified – possibly, due to some omitted components. From a logical perspective, a contradiction makes the inus hypothesis ‘false’, as its realizations cannot establish the subpopulation of y-instances as a separate set from that of ${{\bar{Y}}}$-instances.
The diagnosis of the contradiction through the S.cons is straightforward with crisp scores (Rihoux and De Meur, Reference Rihoux, De Meur, Rihoux and Ragin2009) but can prove harder with fuzzy scores. The violation can be downgraded to an acceptable inconsistency when it arises from units for which Y i ≥ ω ji + 0.1 (Ragin, Reference Ragin2000); truly contradictory instances instead remain those crisp consistency outliers for which ω ji > 0.5 while y i < 0.5 (Rohlfing and Schneider, Reference Rohlfing and Schneider2013; Rubinson, Reference Rubinson2013; Rohlfing, Reference Rohlfing2020). The misalignment of the crisp and the fuzzy consistencies depends on the fuzzy scores leaving arithmetic residuals in intersections: to witness, $\psi _{P} ^{\rm fs} = 0.8$ yields $\psi _{( {P_i \cup {\bar{P}}_i} ) }^{\rm fs} = 0.2$
, which indicates an empty intersection by the rules in Table 1, yet inflates the diagnostic of the S.cons. To overcome the problem, a corrected version of the parameter has been devised. The Proportional Reduction of Inconsistency (PRI), calculated as $PRI_{\omega _j {{\subset Y}}} := \vert {\omega_j {{\cap Y}}} \vert - \vert {\omega_j {{\cap Y \cap \bar{Y}}} \vert / \vert {\omega_j} \vert - \vert {\omega_j {{\cap Y \cap \bar{Y}}}} \vert}$
, explicitly borrows the rationale of the Proportional Reduction of Error. The gauge of inconsistency is $\vert {\omega_j {{\cap Y \cap \bar{Y}}}} \vert$
, which assigns higher penalties to fuzzy values of the outcome closer to 0.5. The PRI, hence, displays a steep fall in the values of the parameter, often for primitives with S.cons equal to 0.85 or lower. The convention maintains that a configuration is usually sufficient to an outcome when its S.cons is 0.85 or higher and supported by a similar PRI – although model specifications may suggest otherwise (Schneider and Wagemann, Reference Schneider and Wagemann2012).
Requirement (b): handling the overspecification of inus machines
The additional relevant information in Standard QCA comes from the coverage of sufficiency, or S.cov for short, defined as $S.cov_{\omega _j {\it{\subset Y}}} := \vert {\omega_j {\it{\cap Y}}} \vert / \vert {\it{Y}} \vert$. The parameter indicates the empirical relevance of the realization ω j to the instances of the outcome y (Ragin, Reference Ragin2006, Reference Ragin2008). It takes its highest value of 1.00 when the j-th realization is shared by all the instances of Y and tends toward 0.00 the more there are instances of the outcome set (ψ Y > 0.50) outside the configuration set $( \psi _{\omega _j} < 0.50)$
.
The raising of uncovered instances may follow from omitted alternative paths to the outcome (e.g. Rohlfing and Schneider, Reference Rohlfing and Schneider2013; Oana and Schneider, Reference Oana and Schneider2018). Usually, their presence is deemed of little or no threat to the standing of a consistent hypothesis. If we are only interested in the machine triggered by our model, any uncovered instances of y can be a red herring. However, coverage outliers can have another and more concerning source. They are also diagnosed on the overspecification of the model that occurs when unrelated conditions are added. In explanatory QCA, the minimization algorithm offers a strategy to handle this threat while providing an argument in favor of the often-deplored practice of selecting on the dependent (e.g. King et al., Reference King, Keohane and Verba1994: 130). Indeed, a renowned ‘paradox of confirmation’ illustrates the absurd conclusions that standard analyses can reach when blindly applied to instances selected on a ‘wrong’ independent (Salmon, Reference Salmon1989: 50).
The paradox portrays the case of the table salt that is believed to dissolve (y) once put in hexed (h) water (w). The underlying inus model, then, reads ${\it{H \cap W \to Y}}$. The paradox arises as natural diversity presents us with the primitives as in Table 2.
Table 2. Truth table from the model ${\it{ H \cap W \to Y}}$

An analysis narrowing on π(Y|ω 1) would consider the relationship sound, even by contrast with π(Y|ω 4). The proof of the irrelevance of ${{\bf H}}$ emerges from the evidence that π(Y|ω 1) = π(Y|ω 3) and π(Y|ω 2) = π(Y|ω 4), once the distinction is made between hexed and non-hexed water. Under the improved model specification, the comparison of configurations to the same outcome pinpoints the irrelevant component as the one whose variation does not affect the state of the outcome. Its removal follows from a logical operation that the original pruning algorithm of QCA, the Quine-McCluskey, performs systematically. To witness: in Table 2, the non-contradictory antecedent of ${{{Y}}}$
is ω 1, ω 3; hence, we can rewrite the solution of ${{{Y}}}$
as $( {{{H\, \cup\, \bar H}}} ) {{W}}$
. By the axioms in Table 1, ${{H\, \cup \,\bar H}\, =\; {\cal U}}$
; hence, h can be dismissed as ‘noisy’ background variation. Run on the instances of y, then on those of ${{\bar{Y}}}$
, the operation licenses the conclusion that the non-irrelevant implicant of ${{\bf \it{Y}}}$
is ${\it{W}}$
and that the inus model is truer to the cases at hand when specified as w → y.
Addendum to requirement (b): handling unobserved realizations
The previous example has assumed a saturated truth table in which all the possible realizations were observed. Technically, observed realizations are those in which at least one unit has a crisp membership score of 1. In actual explanatory QCA, however, an inus model easily allows for a wider array of realizations than the units may afford from a certain universe. The problem is understood as ‘limited diversity’ and provides a further version of the curse of dimensionality. It is independent of the mere ratio of the number of cases and variables, and may not be properly addressed by adding cases: infinite instances of the same primitive in an analytic space of four still make three primitives unobserved and provide no analytic leverage.
The strategies to handle unobserved realizations are many, each addressing a possible source of the problem. Observed diversity may increase if we widen the space-time region of the analysis – the ‘scope condition’ for case selection (Marx and Dusa, Reference Marx and Dusa2011). The dimensionality of the analytic space can decrease if some gelling interactions are hardened into measures of coarser factors (Berg-Schlosser and De Meur, Reference Berg-Schlosser, De Meur, Rihoux and Ragin2009; Schneider, Reference Schneider2019). If inadvertently added, empirical constants may be dropped before the analysis of irrelevance as they double the analytic space but leave half of the primitives unobserved (Goertz, Reference Goertz2006).
The latter consideration has evolved into a whole step of the standard protocol. The ‘analysis of individual necessity’ calculates the same parameters as the analysis of sufficiency, but of conditions and with a reverse meaning. Constants are degenerate necessary conditions, that is, limiting cases of supersets of an outcome-set. They arise when |Y| ≤ |P| and entail a lower-triangular fit. The membership in the condition given that in the outcome renders the individual consistency of necessity ($kN.cons_{{\it{Y \subset A}}}: = \vert {\it{A \cap Y}} \vert / \vert {\it{Y}} \vert$), whereas the membership in the outcome given that in the condition provides the individual coverage of necessity ($kN.cov_{{\it{Y \subset A}}}: = \vert {\it{A \cap Y}} \vert / \vert {\it{A}} \vert$
)– where ‘individual’ is referred to the k-th inus condition. The Relevance of Necessity ($RoN_{{{Y \subset A}}} : = \vert {{\bar{A}}} \vert / \vert {{\bar{A} \cup \bar{Y}}} \vert$
: Schneider and Wagemann, Reference Schneider and Wagemann2012) is a later addition aimed to verify the meaningful variation of each inus components. It is calculated as the reciprocal of the membership in the unrealized outcome conditional on that in the unrealized factor. The standard recommendation is to consider dropping as trivial the factors with kN.cons close to 1.00 and low RoN. However, the crucial test and consistent with the inus rationale remains whether the model requires any seemingly trivial condition to prevent the rising of contradictions in the truth table (Damonte, Reference Damonte2018; Rohlfing, Reference Rohlfing2020).
However, the protocol of reference to handle limited diversity is that of the standard minimizations that treat unobserved primitives as counterfactuals (Ragin, Reference Ragin2008; Schneider and Wagemann, Reference Schneider and Wagemann2012) and, ultimately, a problem of missing outcomes. The related counterfactual question asks whether any instance of these configurations, known yet unobserved, would have displayed y instead of ${{\bar{Y}}}$ (Ramsey, Reference Ramsey and Mellor1929). The standard protocol provides three answers – ‘conservative’, ‘parsimonious’, and ‘intermediate’ – and draws its conclusions under as many alternative assumptions.
The conservative stipulates that no unobserved realization would have obtained; so, its minimizations only operate on observed diversity. The parsimonious maintains that any unobserved realization would have obtained that finds a perfect observed match but for a single minimizand – the component to be declared irrelevant. The intermediate corrects the parsimonious assumption with plausibility concerns. It requires the unobserved minimizand to be in the state that the inus theory considers ‘right’ to the outcome. The minimization under such ‘directional expectations’ provides the intermediate or ‘plausible’ solution. Given all non-contradictory primitives, the conservative and the parsimonious solutions provide the tighter and looser boundaries of a ‘confidence solution space’ in which the intermediate solution usually offers the plausible estimate to the best of knowledge – with a caveat.
Concerns have been raised that directional expectations might introduce confirmation bias in solutions. Minimizations are geared to disconfirming the relevance of a component of the model, not to establish it; so, relying on directional expectations to drop a term rather uses the theory against itself. Instead, the blind application of the plausibility rules may arise a different version of the ‘paradox of confirmation’ in which the belief in a wrong theory prevents the dropping of irrelevant conditions from the solution.
To witness, let us take the hexed salt example of Table 2, but now with an unobserved realization, $\omega _3^\ast \colon {{\bar{H}W}}$ in Table 3. Let also assume that our directional expectations about ${\it{H}}$
reduce to the belief that, teamed with the right inus factors $ {\Phi}$
, hexing is an inus component of the machine to y when present: $ {\it{H}} \Phi {{\subset Y}}$
.
Table 3. Truth table of ${\it{H \cap W \to Y}}$ with unobserved ω3

Given Table 3,
-
– ω 1 is the only realization to $ {{Y}}$
, and the conservative solution reads $ {{HW}}$
: the salt dissolved because it was hexed and in water.
-
– the parsimonious minimization matches ω 1 and $\omega _3^\ast$
and yields $ {{W}}$
as the prime implicant: the salt dissolved just because in water.
-
– the intermediate minimization considers that $\omega _3^\ast$
is an implausible or ‘hard’ counterfactual instead, as it carries the minimizand in the wrong state according to the directional expectations. Thus, $\omega _3^\ast$
is barred from the minimization with ω 1; the resulting plausible solution overlaps the conservative, and the irrelevant factor is not dropped.
To some, the proven inability of the intermediate solutions to get always rid of known irrelevant components disqualifies it as valid, and leaves the parsimonious solutions as the finding to be discussed (e.g. Thiem, Reference Thiem2019). To others, parsimonious solutions are too dependent on observed realizations, and their sufficiency far less ‘robust’ when tested on shrinking diversity for providing a reliable result (e.g. Duşa, Reference Duşa2019). Besides, plausible assumptions are required to make complete structures emerge (e.g. Fiss, Reference Fiss2011; Damonte, Reference Damonte2018; Schneider, Reference Schneider2019).
The debate disregards the possibility that data contain handy information to establish the plausibility of the inus theory. The values of the analysis of individual sufficiency provide indications on the tenability of our directional expectations in ${\cal U}$. When calculated on the conditions of the hexed salt as in Table 4, the kPRI and the kS.cons values show h being equally insufficient to y and $ {{\bar{Y}}}$
, while the RoN values warn that expectations of necessity are untenable, too.
Table 4. Analysis of individual necessity of the conditions in Table 3

In short, the analysis of individual necessity may suggest whether directional expectations stand evidence and can be forced onto solutions.
QCA and local causal structures
The previous section showed that explanatory QCA is equipped to identify inus compounds with established tools, although adapted to its special gauges, and to diagnose the challenges from ill-selected conditions and untenable theoretical expectations. Once the sufficiency requirements are satisfied and the irrelevant conditions dismissed, the solutions provide a regularity answer to why the outcome occurred in some of the cases at hand and not in others. The answer is, the complete realizations of the inus machine were at work in the positive instances, and in its incomplete or obstructed realizations prevented the generative process in the negative instances. That the two answers make two halves of the same causal story, hence, depends on both being based on a single bundle of inus conditions (Verba, Reference Verba1967; cfr. Schneider and Wagemann, Reference Schneider and Wagemann2012).
Still, the further question remains open whether QCA solutions can be granted a causal interpretation beyond the theory that drove the original definition of the inus machine. The shapes that the SCM assumes as causal – namely, the chain ${\cal G}_{l}$, the fork ${\cal G}_{f}$
, and collider $ {\cal G}_{c}$
– all capture conditionality as Kolmogorov's probability; hence, they can be applied to illuminate the relationship between intermediate and parsimonious solution, as in the example below.
Drawing an inus model
The illustrative model accounts for differences in the national perception of corruption – the outcome – with the differences in how effective the accountability constraints are perceived to be to the discretion of the policy-makers in the public sector – the explanatory factors.
The underlying theory connects corruption to Elinor Ostrom's second-order social trap in which perceptions play the role of triggers. The basic mechanism considers that high perceived corruption fuels distrust in the fair working of institutions; distrust, in turn, makes people convinced that resorting to corruption remains the safest way of accessing public services and benefits even when they hold the right to it. These tragedies, in Ostrom's framework, can be fixed if communities restore fairness and delegate the task of detecting and sanctioning violations to an independent ‘monitor’. The fixing can nevertheless fail, too, when the monitor is perceived as ineffective or complacent. Unsanctioned violations and forbearance trigger a ‘second-order’ social trap: the choice of free-riding control becomes individually rational, and corruption institutionalizes. In short, the mechanism suggests that the trigger fires when accountability designs are perceived as poor or incomplete; vice versa, a credible accountability design should provide the inus machine that preserves trust and keeps the perception of corruption low (e.g. Ostrom, Reference Ostrom1998).
The next question asks which components constitute such an inus machine. The theories of public accountability distinguish between internal and external systems, and assign higher effectiveness to the latter. While internal managerial or somehow hierarchical lines of oversight may invite forbearance to avoid blame, external systems are expected to deter corrupt practices by making oversight public, and be more effective when the institutional design maintains the chances low that a single concern can capture the whole attention of the decision-maker. The mechanism also suggests that a special place in the inus machine should be granted to the perceived effectiveness of the judicial system as the warrant against complacency and forbearance (e.g. Weingast, Reference Weingast1984; Mungiu-Pippidi, Reference Mungiu-Pippidi2013; Damonte, Reference Damonte2017).
The operationalization borrows the raw explanatory conditions from the sub-indices of the Rule of Law Index maintained by the World Justice Project. The gauges are composite, but their contents are consistent with the underlying concept, and all point in the same direction (Lazarsfeld and Henry, Reference Lazarsfeld and Henry1968). From the dataset related to 2017, the following gauges are used:
-
– subindex 1.3. ‘Government powers are effectively limited by independent auditing and review’, for the condition ‹atec›. The raw variable gauges the perception that comptrollers or auditors, as well as national human rights ombudsman agencies, have sufficient independence and the ability to exercise adequate checks on and oversight of the government.
-
– subindex 1.5 ‘Government powers are subject to non-governmental checks’, to calibrate the condition ‹asoc›. The raw variable gauges the perception that independent media, civil society organizations, political parties, and individuals are free to report and comment on government policies without fear of retaliation.
-
– subindex 3.1 ‘Publicized laws and government data’ to calibrate the condition ‹apub›. The raw measure gauges the perception that basic laws and information on legal rights are publicly available, presented in everyday language, and accessible. It also captures the quality and accessibility of information published by the government in print or online, and whether administrative regulations, drafts of legislation, and high court decisions are promptly accessible to the public.
-
– subindex 3.2 ‘Right to information’ to calibrate the condition ‹rta›. The underlying raw measure gauges the perception that requests for relevant information from a government agency are timely granted, that responses are pertinent and complete, and that the cost of access is reasonable and free from bribes.
-
– subindex 7.6 ‘Civil justice is effectively enforced’ as the condition ‹enfor›. The raw variable gauges the perception of effectiveness and timeliness of the enforcing practices of civil justice decisions and judgments in practice.
The operationalization of the outcome ‹clean›, instead, relies on the Corruption Perception Index maintained by Transparency International. This again provides a suitable gauge of the perceived level of corruption of the administrative bodies, collected from surveys, and, since 2012, validated through a transparent methodology.
As the measures of the outcome and the inus factors come from surveys and discount the variations in the year of reference, no need for lagging the effect is envisaged. The perception of the accountability of the administration and the perception of corruption in the public sector count as aggregate responses to the same state of the policymaking system.
The data of the Corruption Perception Index and the World Justice Project are all collected from a variety of world regions, although not from the same countries. When combined, the more comprehensive coverage is of the countries in the European Union, the European Free Trade Area, and the core Anglophone countries. Together, their administrative and institutional systems provide enough diversity to make patterns emerge. At the same time, they all are uninterrupted democratic systems, although at different degrees of maturity, which ensures the gauges of the conditions in the model can be given unambiguous interpretations.
After dropping the cases with missing values, the population suitable for the analysis includes 26 cases, whereas the specification of the model includes five explanatory conditions and reads ${{ATEC \middot ASOC \middot APUB \middot RTA \middot ENFOR} \to {\it{CLEAN}}}$.
The raw values are reported in the online Appendix.
Analysis and findings
Following theory and gauging, directional expectations are that each condition contributes to low perceived corruption (clean) when present, and high perceived corruption (clean) when absent. The k-parameters of the calibrated measures, reported in Table 5, support all of them.
Table 5. k-parameters of fit

The conditions' states are consistent with one outcome's state as expected and symmetric in their set-relationships with the outcome and its negation. Moreover, their kN.cons is never trivial. Together, they yield the truth table as in Table 6.
Table 6. Truth table: observed realizations and consistency to the outcomes
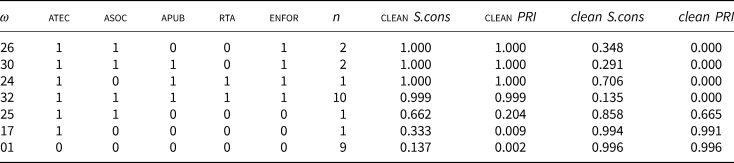
Of 32 possible realizations, seven only are observed and neatly associated with either one outcome or the other with one exception (ω 25), which does not affect the analysis when run with crisp scores. Units concentrate in the two polar realizations: nine of 11 instances of the negative outcome are the best instances of ω 1 while 10 out of 15 positive instances are best instances of ω 32. Were already the model a well-specified inus machine, the concentration should be higher. Dispersion suggests alternative specifications and/or redundancies, which justifies minimizations.
The minimizations retrieve the solutions in Table 7.
Table 7. Prime implicants
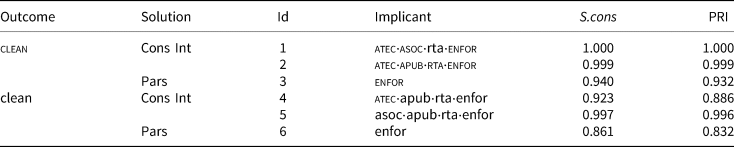
Note: Prime Implicant 1 covers 4 of the 15 instances of aut, bel; aus, can.
PI 2 covers 11 of the 15 instances of clean: fra; nzl, deu, dnk, est, fin, gbr, nld, nor, swe, usa.
No instances of clean are overdetermined.
PI 3 covers all the 15 positive instances.
PI 4 covers 2 of the 11 instances of clean: ita; prt.
PI 5 covers 10 of the 11 instances of clean: bgr, cze, esp, grc, hrv, hun, pol, rou, svn; ita.
One instance of clean is overdetermined – namely, ita.
PI 6 covers all the 11 negative instances.
The parsimonious solutions identify a single factor (the effectiveness of civil justice enforcement) that captures the whole difference between the instances of the positive and the negative outcome. The intermediate solution instead overlaps the conservative; their prime implicants use all the conditions in the model, but differently specified to special subpopulations. The settings suggest that alternative inus machines are at work in groups of instances of the realized outcome. The instances of the unrealized outcome contain one overdetermined case, in which the failure can be ascribed to one or the other of two compounds.
Letting the theoretical interpretation aside, the last open question asks which causal standing can be recognized to the information in the parsimonious and intermediate solutions.
Exploring the relationship between solution types
Fiss (Reference Fiss2011, Soda and Furnari Reference Soda and Furnari2012) dubs the conditions in the parsimonious term the ‘core’ element of the solution, while the conditions added under directional expectations are ‘peripheral’ contributors. The S.cons and PRI reported in Table 6 prove that the core provides a worse explanation when alone than in conjunction with the peripheral terms. Hence, the peripherals are relevant to the outcome, although maybe not causally so. The SCM provides the diagnostic device that clarifies the causal nature of their relationship.
Tables 8 and 9 report the conditionalities that identify the structures of a chain, a confounder, and a collider, computed between the core condition (z), the peripheral conditions in each solution term (x 1, x 2), and the outcome (y) for each outcome state after turning the fuzzy scores into crisp. A fundamental causal structure is assigned to the solution term when all the identifying conditionalities are satisfied. The conditionalities are inevitably deterministic given the gauges and the single observation point. Nevertheless, in such a thin slice of the world, structures do emerge.
Table 8. Structures of the intermediate solution terms to clean

Keys: the nodes in the graphs are given the following values: y = CLEAN; z = ENFOR; $x_1 = {\rm TEC} \cap {\rm PUB} \cap {\rm RTA}$; $x_2 = {\rm TEC} \cap {\rm SOC} \cap \text{rta}$
.
N = 26 for each node.
Table 9. Structural dependencies of the intermediate solution terms to clean
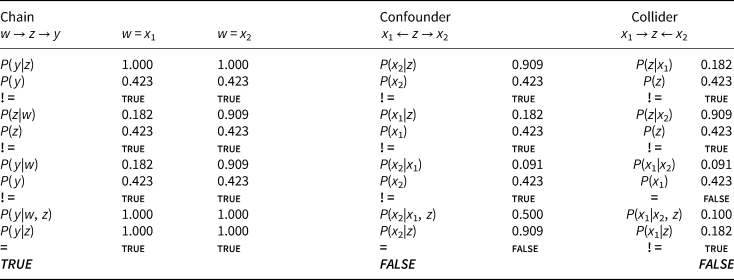
Keys: the nodes in the graphs are given the following values: y = clean; z = enfor; $x_1 = {\rm TEC} \cap \text{pub} \cap \text{rta}$; $x_2 = \text{soc} \cap \text{pub} \cap \text{rta}$
.
N = 26 for each node.
The conditionalities of the ‘chain’ structures indicate that the core provides the mediating node between the peripheral conditions and the outcome in both the positive and the negative solutions. The conditionalities, moreover, support the claim that the core term provides neither the ‘confounding’ background common factor nor the ‘collider’ in any subpopulations – regardless of whether the peripheral terms display full set-independence [P(x 2|x 1) = 0.00 and P(x 1|x 2) = 0.00 in Table 8] or a slight dependence [P(x 2|x 1) = 0.091 and P(x 1|x 2) = 0.091 in Table 9].
These findings suggest that each of the solutions identified by the plausible minimizations renders the settings of a mechanism, and the core elements provide the ‘mediator’. Moreover, the shape suggests that the peripheral conditions do not offer alternative starting points, but equivalent backgrounds.
Concluding remarks
The article offers arguments and evidence that important reasons for discontent with QCA may apply to the inductive usage of the technique, yet are unjustified when addressed to its explanatory, theory-driven application (cfr. Schneider and Wagemann Reference Schneider and Wagemann2012, Thomann and Maggetti Reference Thomann and Maggetti2020).
When carefully implemented, explanatory QCA inevitably displays some commonalities with the probabilistic family. Both identify causality with the capacity to affect a key state of special units and consider causation as an asymmetric phenomenon. The quasi-experimental scholarship recognizes the issue as the difference in ‘propensities’ or ‘covariates’ presiding over the self-selection mechanisms to receive the stimulus. Explanatory QCA models the covariates and the stimulus as the team of inus conditions entailing the capacity to arise or maintain a state of the outcome. Thus, explanatory QCA offers a set-theoretic answer to the question asking which combination of conditions ensures the units' response to a key factor and which ones make them unresponsive instead. The PO may work it out as the unwelcome heterogeneity that biases the estimation of the effect. Just the opposite, explanatory QCA joins the SCM in considering settings as the special background that accounts for the firing of a trigger. Along this line, the solutions from explanatory QCA can provide credible information on which relevant features identify the features that make some units responsive and others ‘inert’.
Moreover, the article shows that QCA solutions from an inus model can be tested for SCM causal structures, and meaningfully so. In the example, the parsimonious solution term – the 'difference-making' components of the model – is proven to take the position of a mediator to the outcome. In turn, the peripheral conditions provide the ‘covariates’ that complete the inus machine and account for its effectiveness.
These considerations speak to the QCA scholarship interested in the debate on the standing of the intermediate solution. The findings suggest that the parsimonious term remains a key component but seldom makes the whole of an explanation. In terms of the example in section 3, a civil justice perceived as effective supports the perception of low public sector corruption as it backs the belief that other accountability devices and holders are trustworthy, first. Reducing the explanation to the sole perceived effectiveness of civil justice makes it gappy, as it does not clarify the ground on which it stands.
The considerations possibly speak to the wider causal scholarship, too. They suggest the equivalence of SCM mediators and core QCA conditions, and of PO-SCM ‘covariates’ and peripheral conditions. The equivalences establish the relevance of configurational results to probabilistic models, as they offer a logical specification of the components of a graph. Moreover, the equivalence suggests the possibility of cumulating and refining causal knowledge by nesting and triangulating techniques. Hopefully, these considerations will contribute to widen the dialogue across causal strategies.
Funding
This research received no grants from public, commercial or non-profit funding agency.
Data
The replication dataset is available at http://thedata.harvard.edu/dvn/dv/ipsr-risp.
Supplementary material
The supplementary material for this article can be found at https://doi.org/10.1017/ipo.2021.2.
Acknowledgments
An earlier version of this work was presented to the 2nd Workshop of the Standing Group on ‘Metodi della Ricerca per la Scienza Politica’ (MetRiSP) of the Italian Political Science Association (SISP), held in 2019 at the Università degli Studi di Catania. I am especially grateful for the comments that I received at the time, and for the suggestions from the IPSR's anonymous referees.