Systematic reviews and health technology assessments (HTA) summarize the best evidence available for carefully formulated research questions. Nevertheless, despite clearly defined eligibility criteria, included studies often differ with respect to population characteristics, interventions and co-interventions, control groups, outcomes, settings, methodological rigor, or specific features of study design. For a systematic review or HTA to provide meaningful results, researchers must determine whether such differences lead to clinically relevant variations in treatment effects.
Identifying differences that have an impact on the magnitude of treatment effects (often termed effect-measure modifiers) is important to decision-makers and patients alike because it helps them understand who is likely to benefit the most, who is likely to benefit the least, and who has the greatest (or preferably least) risk of experiencing adverse outcomes. Examples of factors that may influence the magnitude of treatment effects are age, sex, severity of disease, baseline risks, comorbidities, or cointerventions. For instance, if sex acts as an effect-measure modifier, the magnitude of either the treatment effect or the risk of harms differs between males and females for one or more measures of those outcomes.
In clinical literature, differences among studies that have the potential to influence treatment effects are often termed heterogeneity (Reference Kravitz, Duan and Braslow18). If differences pertain to population characteristics, interventions, and outcomes measured, commonly used terms are clinical heterogeneity, clinical diversity, or heterogeneity of treatment effects (Reference Higgins and Green15;Reference Kravitz, Duan and Braslow18).
Any specific intervention is unlikely to benefit everyone equally, even with a clinically relevant overall treatment effect (Reference Kravitz, Duan and Braslow18). A hypothetical intervention with a number needed to treat of 3 to achieve a beneficial outcome would be considered highly effective. Nevertheless, in this scenario, on average, two of three treated patients would not experience any benefit from the intervention. Moreover, they might even experience harm from the treatment with no gain or benefit. Exploring clinical heterogeneity in a large body of evidence such as in systematic reviews and HTAs can help identify patterns of variations of treatment effects that are not obvious in clinical trials or similar studies but that need to be understood to arrive at appropriate conclusions.
Assessing clinical heterogeneity, however, is not always straightforward. The main challenge is that part of the observed variation of treatment effects is spurious, caused by random error (chance). Historically, the impact of clinical heterogeneity has been both under- and over-estimated, based on incautious subgroup analyses or anecdotal clinical evidence leading to over- as well as under-treatment of some populations.
This study summarizes a project funded by the United States (US) Agency for Healthcare Research and Quality (AHRQ) to identify, discuss, and synthesize best practices for addressing clinical heterogeneity in systematic reviews and HTAs (Reference West, Gartlehner and Mansfield25). Table 1 summarizes definitions of all relevant terms used in this study.
Table 1. Glossary of Terms for this Study
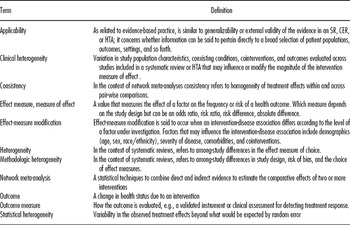
CER, comparative effectiveness review; HTA, health technology assessment; SR, systematic review.
METHODS
Identification of Best Practice Methods
To identify best practice methods we used a three-tiered approach. First, we sought a convenience sample of guidance reports and manuals prepared by international organizations engaged in preparing systematic reviews or HTAs. The target organizations are referenced in the underlying report (Reference West, Gartlehner and Mansfield25) of this study and included: Agency for Healthcare Research and Quality (AHRQ, www.ahrq.gov), United States; Centre for Reviews and Dissemination at the University of York (CRD http://www.york.ac.uk/inst/crd/), United Kingdom; Cochrane Collaboration (http://www.cochrane.org/); European Network for Health Technology Assessments (EUnetHTA http://www.eunethta.net); Institute for Quality and Efficiency in Health Care (IQWIG [Institut für Qualität und Wirtschaftlichkeit im Gesundheitswesen] http://www.iqwig.de), Germany; HuGENet (Human Genome Epidemiology Network http://www.cdc.gov/genomics/hugenet/default.htm); National Health and Medical Research Council (NHMRC http://www.nhmrc.gov.au/), Australia; National Institute for Health and Clinical Excellence (NICE, http://www.nice.org.uk), United Kingdom; Oregon Health & Science University Drug Effectiveness Review Project (DERP, http://www.ohsu.edu/ohsuedu/research/policycenter/DERP), United States.
Second, we conducted a PubMed® literature search that focused on how systematic reviews and meta-analyses handle heterogeneity. We sought to identify whether guidance on the conduct of systematic reviews and HTAs (i) differentiated among different types of heterogeneity and (ii) described how to identify factors causing clinical heterogeneity, including evaluating particular subgroups. We conducted our search on May 1, 2009 (search strategy provided upon request).
Third, we conducted citation analysis using the Science Citation Index (http://thomsonreuters.com/products_services/science/science_products/a-z/science_citation_index) to identify publications that had cited three seminal articles (Reference Berlin2;Reference Colditz, Burdick and Mosteller7;Reference Thompson24) about the importance of evaluating clinical heterogeneity in systematic reviews and meta-analyses.
Our searches rendered 1,065 citations. Of eighty-three articles selected for full-text review, eighty addressed heterogeneity among studies.
Extraction of Information
Two authors independently assessed the title and abstract of each document. We conducted a full-text review of those articles that appeared to have useful information on handling clinical heterogeneity and retained those that had relevant information.
We then abstracted information from included publications into standardized data abstraction forms. We captured information on whether the various methods manuals and manuscripts addressed clinical heterogeneity at all and, if so, how they defined the term. We also evaluated whether manuals defined statistical heterogeneity and whether they explored or provided examples about the relationship between clinical and statistical heterogeneity. We documented the recommendations on how to deal with clinical and statistical heterogeneity.
Synthesis of Information
Throughout the study we have summarized the information qualitatively. Because varying and sometimes contradictory views regarding clinical heterogeneity and methods on how to deal with clinical heterogeneity exist in the literature, our analysis must be viewed as a synthesis and interpretation by the authors of this study, vetted by the peer reviewers of the underlying AHRQ report (Reference West, Gartlehner and Mansfield25).
RESULTS
Following we will first define clinical heterogeneity and highlight distinctions and relationships between clinical and other forms or heterogeneity. We will then discuss ways to assess clinical heterogeneity and provide recommendations for researchers conducting systematic reviews and HTAs.
Definitions of Clinical Heterogeneity in Guidance Manuals
Of the nine methods manuals reviewed, only five—AHRQ, CRD, Cochrane Collaboration, DERP, and EUnetHTA— provided explicit definitions of clinical heterogeneity (1;5;9;10;Reference Higgins and Green15). The definitions were similar, although AHRQ, Cochrane, and the CRD used the term clinical diversity rather than clinical heterogeneity. Drawing from these definitions, for this study, we define clinical heterogeneity as the variation in study population characteristics, coexisting conditions, (co)interventions, and outcomes evaluated across studies included in a systematic review or HTA that may influence or modify the magnitude of the intervention measure of effect (e.g., odds ratio, risk ratio, risk difference). This definition emphasizes that clinical heterogeneity may, but does not necessarily have to, cause variations in treatment effects.
Distinctions between Clinical and Other Forms of Heterogeneity
Some guidance manuals and various methods articles distinguish clinical heterogeneity from two other forms of heterogeneity: methodological heterogeneity and statistical heterogeneity.
Methodological heterogeneity refers to between-study differences in methodological factors such as adequate randomization, allocation concealment, or use of blinding that can lead to differences in observed treatment effects. The Cochrane Collaboration defines methodological heterogeneity as “the variability in study designs and risk of bias” (Reference Higgins and Green15). Empiric studies have shown that poor study design and conduct can lead to an overestimation of the magnitude of the effect. Methodological heterogeneity, however, can also entail variations in patient eligibility criteria, follow-up periods, or other differences in study designs among a group of studies. Methodological heterogeneity, however, does not necessarily indicate that the true intervention effect varies.
Statistical heterogeneity refers to the variability in the observed treatment effects that is beyond what would be expected by random error (chance); it is detected by a statistical test and can be viewed as a global assessment of the variability of treatment effects across studies within a given body of evidence. Both clinical and methodological heterogeneity can, but do not have to, result in statistical heterogeneity (Reference Thompson24).
Relationships among Clinical, Methodological, and Statistical Heterogeneity
Although it is important conceptually to distinguish among clinical, methodological, and statistical heterogeneity, in practice, they are strongly intertwined. For example, methodological heterogeneity can directly affect statistical heterogeneity even if no true variations in treatment effects exist. The choice of outcome criteria, for instance, such as the percentage of improvement that defines a response to treatment can cause statistical heterogeneity. Even if all trials measure response to treatment, some trials may use more sensitive outcome criteria whereas others may use more specific criteria. Methodological heterogeneity can also indirectly affect statistical heterogeneity by leading to clinical heterogeneity. For example, differences in patient eligibility criteria can result in heterogeneous patient populations among trials. Some clinical trials may recruit patients with severe disease only, while others may recruit patients with both mild and severe disease. Such clinical heterogeneity (disease severity) can modify a measure of treatment effect and lead to statistical heterogeneity.
Although clinical, methodological, and statistical heterogeneity are closely intertwined, they do not have a linear relationship. In other words, high clinical or methodological heterogeneity do not always cause statistical heterogeneity. For example, the inclusion of in- and outpatients in a meta-analysis of second-generation antidepressants for the treatment of major depressive disorder did not lead to statistical heterogeneity, despite important clinical differences in disease severity between in- and outpatients (Reference Gartlehner, Hansen and Thieda11). The choice of the effect measure can also influence whether clinical heterogeneity causes statistical heterogeneity.
Conversely, statistical heterogeneity does not always indicate a high degree of clinical or methodological heterogeneity. In addition to biases that threaten the validity of individual studies and that are captured under methodological heterogeneity, various other biases, including funding and reporting (publication) biases, may affect variability in treatment effects estimated across studies (Reference Poole and Greenland22). For example, small trials with statistically nonsignificant findings have a higher risk of remaining unpublished than small trials producing significant (or very large) effect-measure estimates.
Assessing Heterogeneity
For researchers conducting systematic reviews or HTAs, assessing whether clinical heterogeneity is present in a given body of evidence begins with a qualitative step to determine differences among populations, interventions and co-interventions, and outcomes across included studies. If clinical heterogeneity is present, analysts can determine its impact on treatment effect estimates with statistical tests.
Statistical tests investigate whether existing variations in treatment effects exceed what would be expected by chance fluctuations alone. Assessing statistical heterogeneity involves testing the null hypothesis that the studies have a common treatment effect given a chosen type I error probability. Commonly used statistical methods to detect and quantify the degree of heterogeneity are Cochran's Q test (Reference Cochran6) and the I2 index (Reference Higgins and Thompson13). Supplementary Table 1, which can be viewed online at www.journals.cambridge.org/thc2012005, summarizes common statistical approaches to test for and to measure heterogeneity (Reference Berlin, Santanna, Schmid, Szczech and Feldman3;Reference Borenstein, Hedges, Higgins and Rothstein4;Reference Higgins, Thompson, Deeks and Altman14;Reference Higgins and Green15;Reference Ioannidis, Patsopoulos and Evangelou16;Reference Morton, Adam, Suttorp and Shekelle20;Reference Petitti21).
If researchers determine that clinical, methodological, or statistical heterogeneity is present, they need to explore such heterogeneity. Three common approaches can be used to further investigate the impact of heterogeneity:
Subgroup analysis Subgroup analysis is an “analysis in which the intervention effect is evaluated in a subset” of particular study participants or in groups defined by study characteristics (Reference Higgins and Green15). For example, the subgroup might be defined by sex (male versus female patients) or by study location (e.g., urban versus rural setting). To be able to draw causal inferences, subgroup analyses should be defined a priori; that is, they should be part of the systematic review protocol. Although subgroup analyses that are conducted post hoc are mainly hypotheses generating, they are an important tool to explore heterogeneity.
Sensitivity analysis is used to “assess how robust the results are to assumptions about the data and the analytic methods that were used” (Reference Higgins and Green15). Generally, sensitivity analyses are frequently post hoc, that is, they occur during the analysis phase of the study but they can also be defined a priori. For example, analysts might conduct sensitivity analyses to determine whether the inclusion of studies published only as abstracts changes the conclusions substantially.
Meta-regression. This type of analysis enables investigators to explore sources of heterogeneity in terms of study-level covariates. For example, analysts can explore the impact of study duration (a study-level covariate) on the magnitude of the pooled treatment effect in a hypothetical meta-analysis using meta-regression. The impact of age (a patient-level covariate), if assessed by meta-regression in the same hypothetical meta-analysis, has to be interpreted very carefully because it can lead to erroneous results for individual patients based on ecological fallacy.
The three approaches to explore heterogeneity outlined above are useful when making pair-wise comparisons. Often, however, studies directly comparing two or more alternative interventions are sparse or entirely missing. Statistical methods such as network meta-analyses have evolved as an additional analytic tool in HTAs when head-to-head evidence is insufficient to derive estimates of comparative treatment effects. Researchers conducting network meta-analyses have to determine the presence of heterogeneity within but also across pair-wise comparisons. A fundamental assumption for the validity of network meta-analyses is that effect estimates of direct and indirect evidence are consistent, which means that direct and indirect estimates vary around the same mean effect (in a random effects model). Therefore, network meta-analyses require analyses to consider another type of heterogeneity, namely the heterogeneity across pair wise comparisons (sometimes termed consistency). Several statistical approaches for both frequentist and Bayesian network meta-analyses have been proposed (Reference Dias, Welton, Caldwell and Ades8).
Recommendations and Considerations for Researchers Conducting Systematic Reviews and HTAs
For addressing clinical heterogeneity in systematic reviews and HTAs, we identified two common recommendations from the existing literature and guidance manuals. The first was that authors should identify factors that may cause clinical and methodological heterogeneity during their protocol development stage. The second recommendation involves subgroup analyses—namely that researchers should limit the list of factors to as few as possible to avoid misleading results. Subgroups identified post hoc during data analysis are often considered a product of data dredging; these subgroups are likely to be ambiguous and not confirmed in future studies.
Both of these recommendations have been suggested to prevent authors of systematic reviews and HTAs to over-interpret apparent relationships between statistical heterogeneity and clinical variations based on results at hand (Reference Thompson24). Nevertheless, identifying factors that have not yet been recognized as effect-measure modifiers may be important. Such discoveries should not be casually dismissed as inconsequential results of data dredging just because the factors were not defined a priori.
In practice, assessing clinical heterogeneity also requires a judgment whether observed variations of treatment effect are clinically relevant. Table 2 outlines the different relations between clinical and statistical heterogeneity under the assumption that chance does not play an appreciable role. The “possible underlying situation” (right column) explains what inferences might be drawn and whether reviewers need to examine the situation further.
Table 2. Summary of Relationships Between Clinical and Statistical Heterogeneity
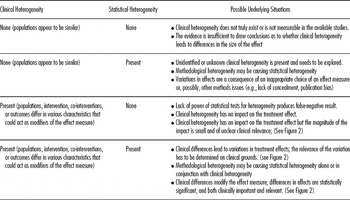
If clinical heterogeneity leads to differences in estimated treatment effects, reviewers should gauge whether the magnitude of such a variation might be clinically meaningful. The final conclusion about clinical importance, however, has to be a shared decision between clinicians and patients. Figure 1 illustrates three underlying situations.
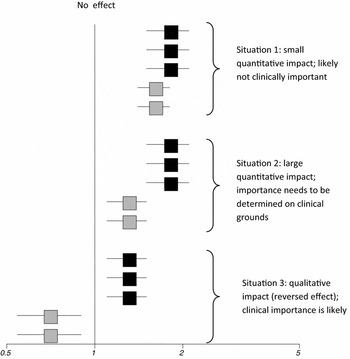
Figure 1. Three different situations of the potential impact of clinical heterogeneity on the treatment effect. Squares with horizontal lines in the figure depict hypothetical point estimates of individual studies with 95% confidence intervals on a log odds ratio scale.
In the first situation (Figure 1), differences in treatment effects are small and likely not clinically relevant. Depending on the outcome of interest, researchers might dismiss the difference as clinically irrelevant. For example, a large cohort study based on data of prescription event monitoring reported that the risk for hallucinations in patients treated with tolterodine for overactive bladder is significantly higher for women than for men (women 8/24,212 versus men 1/11,083; p = .013) (Reference Layton, Pearce and Shakir19). Given the minimal (albeit statistically significant) absolute difference in risks between women and men, findings are unlikely to have an impact on routine clinical practice.
In the second situation (Figure 1), the impact of clinical heterogeneity is larger than in the example above, but the importance of this in terms of interpreting treatment effects has to be determined on clinical grounds. For example, a pooled data analysis of randomized controlled trials in patients treated with paroxetine or bupropion for major depressive disorder reported higher rates of medication-related sexual dysfunction for men treated with paroxetine (Sex Functional Questionnaire [SFQ] change: men −4.16 versus women +2.32 points on SFQ; confidence intervals and p-value were not reported) than for women (Reference Kennedy, Fulton and Bagby17). Such a difference was not detected in men and women treated with bupropion (men +1.79 versus women +0.46). Such information, depending on the population of interest, could lead to the choice of one medication over another.
The third situation (Figure 1) is the most clinically relevant one although presumably much rarer than the situations of weaker and stronger effects described above. Whereas the two previous examples described quantitative differences in treatment effects (both groups experienced the same effect and only the magnitude of the effect differed), in some situations clinical heterogeneity can cause opposing effects. Such reversed effects (also termed qualitative differences) are likely to be clinically important and to have a profound impact on clinical as well as health-policy decisions.
DISCUSSION
Current definitions of clinical heterogeneity are not consistent. Differences among most definitions, however, appear to be more semantic than substantive. Some authors use the term “clinical heterogeneity,” and others may use “clinical diversity” or “heterogeneity of treatment effects.” Most authors distinguish clinical heterogeneity from both statistical and methodological heterogeneity.
The lack of a common definition is accompanied by a lack of guidance on how to assess and deal with clinical heterogeneity in systematic reviews and HTAs. Few review groups or institutions provide guidance on how to best approach clinical heterogeneity. Nevertheless, identifying potential clinical characteristics that have the potential to modify effect measures is important from the planning stages of the review to the synthesis of the evidence.
Clinical heterogeneity is closely related to a broader issue of systematic reviews and HTAs: namely, the assessment of the applicability of findings and conclusions. Applicability (also termed generalizability or external validity) has been defined as inferences about the extent to which a causal relationship holds over variations in persons, settings, treatments, and outcomes (Reference Shadish, Cook and Campbell23). Deciding to whom findings of systematic reviews and HTAs apply requires a close understanding of which patient groups benefit the most and which the least from a given medical intervention; with this information clinicians and health policy decision-makers can appropriately tailor treatments to individuals.
In turn, being aware of treatments for which clinical heterogeneity is not a significant issue is also important. A common criticism of systematic reviews is that they provide average results that are not applicable to individual patients with varying risks and prognostic factors. To identify treatments that are only minimally affected (if at all) by clinical heterogeneity can lead to a more rational use of interventions and help avoid both over- and undertreatment. Exploring clinical heterogeneity in systematic reviews and HTAs helps provide some treatment- and condition-specific information of use in routine clinical care and is, thus, of benefit to patients and their caregivers.
CONCLUSION
Researchers need to consider clinical heterogeneity at all stages of a health technology assessment: as key questions are developed, when considering the inclusion and exclusion criteria, and in the analysis of the evidence. Recognizing clinical heterogeneity and clarifying its implications helps decision makers to identify patient populations who benefit the most and the least from a particular intervention.
SUPPLEMENTARY MATERIAL
Supplementary Table 1 www.journals.cambridge.org/thc2012005
CONFLICT OF INTEREST
Gerald Gartlehner, Suzann West and Alyssa Mansfield have received funding to their institute from the US Agency for Healthcare Research and Quality; Charles Poole has received a consulting fee from Research Triangle Institute; Elisabeth Tant has received funding to her institute from RTI International; Linda Lux has received funding to her institute from the US Department of Health and Human Services and the US Agency for Healthcare Research and Quality; Kathleen Lohr has received funding to her institute from RTI International, and she is the vice-president of the PROMIS Health Organization.
CONTACT INFORMATION
Gerald Gartlehner, MD, MPH, Chair and Professor, Department for Evidence-based Medicine and Clinical Epidemiology, Danube University, Krems, Austria; Senior Health Research Analyst, RTI International, Research Triangle Park, North Carolina
Suzanne L. West, MPH, PhD, Senior Research Analyst, RTI International, Research Triangle Park, North Carolina
Alyssa J. Mansfield, MPH, PhD, Research Analyst, RTI International, Research Triangle Park, North Carolina
Charles Poole, MPH, ScD, Professor, Department of Epidemiology, Gillings School of Global Public Health, University of North Carolina at Chapel Hill, Chapel Hill, North Carolina
Elizabeth Tant, BS, Research Associate, RTI International, Research Triangle Park, North Carolina
Linda J. Lux, MPA, Project Manager, RTI International, Research Triangle Park, North Carolina
Kathleen N. Lohr, PhD, Distinguished Fellow, RTI International, Research Triangle Park, North Carolina