Introduction
In this paper, I wish to explore the limits and definition of life in the context of engineered life (distinct from artificial and synthetic life). Life on Earth is carbon-based and limited to a narrow temperature range defined by the existence of liquid water within the constraints of biochemical sensitivity to temperature – low temperatures reduce metabolic rates while high temperatures degrade enzymes. This gives us a narrow perspective on what life could be. I submit that from an astrobiological perspective, exploring engineered life is a useful and instructive exercise. If we can be sure of anything, it is that nature is innovative and inventive. Taking lessons from extrasolar planet astronomy, the galaxy of stellar system configurations discovered has shown that our own Solar System configuration, rather unexpectedly, is merely one of many different possibilities. If and when the first discovery of extraterrestrial life occurs, we can be sure that it will surprise us (unless it is of a panspermia or contamination origin). Firstly, we will briefly discuss a few definitions of life. Thence, we shall explore an experiment to artificially construct a lifeform (engineered life) that tests the boundaries of life and perhaps give us insights into the transition from non-life to life.
Part A: What is life?
Although some reckon that defining life is futile because it is a folk rather than a scientific concept (Machery Reference Machery2012), we shall explore such a definition here. Indeed, a folk-based test of life has been proposed similar to the Turing ‘imitation game’ test for machine intelligence (Cronin et al. Reference Cronin2006). It proposes the construction of a chemical cell (chell) – a chemoton – to imitate a biological cell, perhaps to ingest CO2 and excrete a more environmentally benign compound. The Viking biochemical experiments may be regarded as an abstraction of this concept (CO2 input – O2 output), suggesting that perhaps such a functional approach is not useful. Looking, however, to internal cellular mechanisms, a computer model of a network of chemical reactions as a primitive metabolism in an amphiphilic membrane has been simulated (Bersini Reference Bersini2010). It is not yet clear how illuminating this type of simulated biochemistry has been but we shall explore these approaches further. Returning for the moment to defining life, there have been some vague definitions:
(i) Life is a unity (Chaitin Reference Chaitin1970) – this refers to a mathematical unity as opposed to an autopoietic unity, the latter having specific and definable properties like autonomy with self-contained rules (Fleischaker Reference Fleischaker1989);
(ii) Life is a supple adaptation involving ongoing adaptation to the environment (Bedau, Reference Bedau1997, Reference Bedau1998).
These definitions are not particularly enlightening or conclusive. Similarly, information-theoretic definitions are purely syntactic and are devoid of semantic interpretation rendering them incomplete (Zuravlev & Avetisov Reference Zuravlev and Avetisov2006). The semantic aspect requires the interpretation of symbols in the context of the real world to understand the function of those symbols. There is, however, no obvious similarity between objects in the real world and the symbols to represent them (this is the correspondence problem). Hence, information defined at the level of syntax has little bearing on pragmatic information which based on its semantic value –it is at the pragmatic level (phenotype) at which evolutionary selection operates but it is at the syntactic level (genotype) that information is stored (Cohen & Perrault Reference Cohen and Perrault1979). The two levels are both required. A biological cell is a physical biochemical machine (Laing Reference Laing1979) – it comprises a power supply, a set of interacting components interconnected by a wiring system, and a regulatory control system to coordinate those components to perform self-reproduction as its major task (Tyson & Mackey Reference Tyson and Mackey2001). Many definitions of life have listed the various properties of life (Koshland Reference Koshland2002) – genetic program of instructions, evolution by natural selection, cellular compartmentalization, energy capture from the environment, regeneration for self-repair and growth, adaptability to stimuli, and isolation of different metabolic reactions being a fairly common and inclusive list. There are derivative or equivalent properties that might be favoured. For instance, reproductive capacity is implicit in the evolutionary property and thermodynamic openness is implicit in the energy capture property. The complex interchange of matter and energy with its environment is implicit in defining metabolic activity.
Futility of Linnaean Classification
Species cannot form clear-cut categories if we admit evolution which imposes mutability. Of course, this pre-supposes Darwinian rather than Lamarckian evolution. Similarly, there may be no clear demarcation between non-life and life if the latter evolves from the former. For instance, Strecker synthesis of amino acids is a chemical process while the reverse Krebs cycle is a biological process. There must be intermediate processes which allow the evolution of the biological from the inorganic (Szostak. Reference Szostak2012). One possibility in this specific case might be through the hydrolysis of thioesters CH3–CO–SCH3 formed from CO and CH2SH catalysed by FeS minerals (Wachtershauser Reference Wachtershauser1992). Similarly, self-organization through far-from-equilibrium thermodynamics exhibits increasing degrees of complexity from physical to biological manifestations – Benard cells in convecting fluids at Rayleigh number beyond 1760 are a physical phenomenon; the Belousov–Zhabotinski reaction is a chemical phenomenon of greater complexity; and the Kauffman NK model of autocatalysis is a pre-biological phenomenon of still greater complexity. Such self-organization involves local interactions between components resulting in emergent global properties without any central control or supervision. Self-organization is a commonly exploited property of biological systems. For example, self-organization of the global cytoskeletal architecture of eukaryotic cells is emergent from the reaction–diffusion effects of individual cytoskeletal filaments.
Furthermore, lack of categorizability was manifest in early biological evolution. It is believed that the last universal common ancestor (LUCA) that eventually diverged into the three main domains of Archaea, Bacteria and Eukarya was constituted from a community of cellular organisms that were engaged in lateral gene transfer with elevated mutation rates rather than vertical gene inheritance (Woese Reference Woese1998). RNA-based genetic translation was simple, acting on multiple and redundant short genetic segments, and was error-prone, limiting protein sizes. Different progenitors possessed different metabolic capabilities which would be shared through lateral gene transfer. This is compatible with the curious incidence of genes shared by archaea and bacteria simultaneously with the genetic replication mechanism shared by archaea and eukarya, yet there appear to be strong similarities in DNA polymerases across all three domains. There was thus no single evolutionary cell line prior to the divergence of the great domains whence individual evolutionary cell lines were established. If so, the early origins of life are shrouded - no wonder that we seek to gain insights a la Richard Feynman (‘what I cannot create, I do not understand’).
The demarcation of life/non-life illustrates the limitations of logic classification – such limitations in logic have been well known in artificial intelligence because logic admits no contradiction (x and not-x cannot both be true), no change over time (x is true or false at all times), and no exclusion (x is either true or false). This was the reason for the introduction of degrees of truth through a veritable host of shades of grey – Bayesian, Dempster–Shaferian, non-monotonic, circumscriptive, modal, temporal and fuzzy logics – into artificial intelligence. The most extreme variation of this is non-well-founded (NWF) set theory which eschews logic almost entirely (Stepney Reference Stepney2012). Bruylants et al. (Reference Bruylants, Bartik and Reisse2010) favoured a fuzzy definition of life though, if pressed, my preference would be for a Bayesian approach for its greater rigour and versatility. As an example of greyness, there is debate over the nature of viruses which are commonly classified as non-life. However, viruses may be controversially characterized as alive in the sense that they are complex evolving organisms integrated into their host's molecular machinery for their own replication – in this phase of the viral lifecycle, the infected host cell is a viral cell (Forterre Reference Forterre2010). Viruses are highly efficient replicators because their host provides the replicating machinery that is hijacked by the virus. Viruses are an example of the difficulty in differentiating between life/non-life and the degrees of uncertainty involved. Indeed, from the folk perspective with which we opened our discussion, they are very much alive because they are ‘germs’ (though the pre-scientific miasma folk theory would not have viewed viral infections as being transmitted through living organisms).
Metabolism
All physicochemical reactions tend towards the lowest Gibbs free energy state according to the second law of thermodynamics. Living systems maintain themselves in a far-from-equilibrium state through the consumption of external energy. Metabolism is a network of chemical reactions and implies that the provision and consumption of energy are necessary to maintain and grow itself. Due to the second law of thermodynamics, this energy must be supplied from the environment. Such thermodynamically open systems form dissipative structures characterized by energy dissipation through dynamically stable structures under far-from-equilibrium conditions. It has been suggested that life creates more entropy in the universe through dissipative processes over ecological timescales than non-life, essentially driven by their efficient consumption of energy (Ulanowicz & Hannon Reference Ulanowicz and Hannon1987). A high-energy gradient in the environment is limited in its dissipative capability, creating local, highly ordered complex structures (life) far from thermal equilibrium to increase energy dissipation (Schneider & Kay Reference Schneider and Kay1994). Biological processes exploit captured energy to move far away from thermodynamic equilibrium to a state of low entropy with respect to the environment by storing the maximum exergy available (the maximum exergy principle) (Jorgensen & Fath Reference Jorgensen and Fath2004). Exergy is defined as the energy available for useful work. The maximum exergy principle is a version of Le Chatelier's principle that energy inputs shift equilibrium compositions of reaction systems. From this, metabolism may be defined as a system of self-maintenance that controls energy flows through the system (Moreno Bergareche & Ruiz-Mirazo Reference Moreno Bergareche and Ruiz-Mirazo1999). This management of energy with precise control of energy flows is essential to organize the material construction of metabolic substrates. We may regard metabolism powered by energy sources as a fundamental ingredient of life. The most basic form of metabolism for the generation of energy is the physicochemical redox couple involving electron donors and electron acceptors, such as anoxygenic photosynthesis by green and purple sulphur bacteria. They reduce sulphate as the electron acceptor (oxidant) to release H2S as an electron donor for the reduction of CO2.
Physical metabolism involving both material and energy processes is a necessary component of life (Boden Reference Boden2003). This disavows artificial life which considers only informational and evolutionary aspects of life without consideration of physicality. If the autopoietic aspect of self-production is an essential component of life, then evolutionary robots are excluded because while their evolved behaviours are informational, their bodies are artificially pre-constructed without metabolic exchange with the environment. Furthermore, evolutionary algorithms are driven by user-defined fitness functions rather than the open-ended evolution of biology. Contrarily, a self-replicating machine is fundamentally self-created from its physical environment where self-replication is an extension of self-repair which itself is a form of self-maintenance. Its capability to create physical copies of itself from the environment is a form of self-production so this would admit self-replicating machines to the ranks of life.
Encapsulation
The autopoietic definition of life proposes that life is a cellular system capable of self-production and self-maintenance through a network of processes within a self-made boundary that adapts to its environment (Bich & Damiano Reference Bich and Damiano2007; Damiano & Luigi Luisi Reference Damiano and Luigi Luisi2010). Self-production implies that genetic information is available while self-maintenance necessitates that there are metabolic processes. Autopoiesis emphasizes identity (referred to as autonomy) as a continuous and dynamic self-regeneration – a process of autopoietic self-organization (Maturana Reference Maturana1975). Structural identity and physical integrity of the lifeform – the cell – is derivative from the processes it encapsulates from its environment, i.e. metabolism. It is this metabolic activity that allows the entity to adapt to its environment through a continuous cycling of anabolic and catabolic processes, i.e. self-production and self-maintenance are the result of the same underlying process. An autopoietic system involves homeostatic processes that are autocatalytic as a whole by continually producing and recycling its components. An illustrative minimal autopoietic model is based on a two-dimensional (2D) cellular array which may be occupied by one of three types of particles: S (substrate), K (catalyst) and L (link) subject to three chemical reactions (Varela et al. Reference Varela, Maturana and Uribe1974):



Chains of L particles can form a closed membrane that is permeable to S particles but impermeable to K and L particles, i.e. cell membrane structures. Initially, at least one K particle is enclosed within an L-membrane through which S particles permeate. These S particles are catalysed by K within the membrane to increase the concentration of L particles which cannot escape. As internal stresses build, the membrane ruptures due to disintegration. However, the high concentration of L ensures that they will seal the L-chain membrane. This models a continual cycle of anabolism and catabolism of the L-membrane. This cycle generates a steady state of the form:

where k is growth rate and g is decay rate. Dynamic equilibrium occurs when k = g such that dx/dt = 0. The inhibition of bonding of L particles near chains of L particles is an essential feature in autopoietic modelling to prevent premature L-chain formation (McMullin & Varela Reference McMullin and Varela1997). This computational model and others (Schwegler & Tarumi Reference Schwegler and Tarumi1986; Cui & Friedman Reference Cui and Friedman1999) prove the computability of autopoiesis (McMullin Reference McMullin2004), refuting postulations that autopoiesis is non-computable.
Membrane-enclosed vesicles that separate self from non-self are an important property of life (Weber Reference Weber2010). The importance of a cell membrane to separate an internally controlled environment from the external environment has long been recognized, but both the coacervate and proteinoid concepts were implausible in lacking continuity with biological membranes. Intriguingly, lipid-like amphiphilic compounds such as PAHs, pyrene and fluoranthene have been extracted from the Murchison meteorite (Deamer & Pashley Reference Deamer and Pashley1989). Amphiphilic molecules comprise a polar hydrophilic tail and hydrophobic head which induces them to spontaneously self-assemble into vesicles as proto-cells (Deamer et al. Reference Deamer, Dworkin, Sandford, Bernstein and Allamandola2002). Self-assembly is spontaneous with amphiphilic molecules – they form a single-layer surface membrane on water with hydrophobic heads oriented above the surface and hydrophilic tails in the water. High concentrations of phospholipids form a double layer which encloses vesicles formed by high surface tension. Amphiphilic molecules may form via Fischer–Tropsch reactions catalysed on mineral surfaces. The membrane must be semipermeable to allow the flow of selective materials and energy. Self-assembling lipid membranes are an obvious candidate for early biological membranes. Reverse micelles may represent a minimal autopoietic system (Luigi Luisi & Varela Reference Luigi Luisi and Varela1989). They spontaneously self-organize into cells within which host aqueous chemical reactions produce its boundary surfactants (comprising a polar head and aliphatic tail). Alternatively, proto-membranes may have been iron/sulphur-based, formed near hydrothermal vents (Martin & Russell Reference Martin and Russell2003). The vesicles enclose polymers which may be formed in a number of plausible ways. Simple precursors to organic molecules that underlie biology can be synthesized into polymers in Miller–Urey-type reactions catalysed by clays under reducing conditions (such as CH4 + NH3 + H2 or CO2 + H2 + N2 or CH4 + H2O + N2 atmospheres) with an energy source by Strecker condensation. Formaldehyde can be synthesized by photochemical reactions in Earth's primitive atmosphere as the primary step towards biochemical materials (Pinto et al. Reference Pinto, Gladstone and Yung1980):

From formaldehyde, many biological molecules may be synthesized:
(a) Production of the pentose sugar ribose:
$${\rm 5}{\rm H}_{\rm 2}{\rm CO} \to {\rm C}_{\rm 5}{\rm H}_{{\rm 1}0}{\rm O}_{\rm 5}$$
(b) HCN may be synthesized under primitive Earth conditions (Oro et al. Reference Oro, Kimball, Fritz and Master1959):
$${\rm H}_{\rm 2}{\rm CO} + {\rm N}{\rm H}_{\rm 2}{\rm OH} \to {\rm HCN} + {\rm 2}{\rm H}_{\rm 2}{\rm O}$$
(c) Amino acid glycine may be produced from HCN:
$${\rm 3HCN} + {\rm 2}{\rm H}_{\rm 2}{\rm O} \to {\rm C}_{\rm 2}{\rm H}_{\rm 5}{\rm O}_{\rm 2}{\rm N} + {\rm C}{\rm N}_{\rm 2}{\rm H}_{\rm 2}$$
(d) The purine base adenine may be produced from HCN:
$${\rm 5HCN} \to {\rm C}_{\rm 5}{\rm H}_{\rm 5}{\rm N}_{\rm 5}$$
Although Miller–Urey-type reactions have been questioned as pre-biotic processes on early Earth, similarly synthesized biochemical products may be concentrated into protocells by semi-permeable membranes that form spontaneously from phospholipids in water. More complex, multi-compartment lipid vesicles represent models of artificial cells in which multi-step biochemical reactions can be implemented (Elani et al. Reference Elani, Law and Ces2014). There have even been suggestions that a minimum of two membranes can implement computing functions (Paun, 2000). Liposomes are engineered vesicles for delivering drugs to the human body formed from the hydrophobic/hydrophilic properties of amphiphilic lipids in water (Mozafari Reference Mozafari2005). Self-assembled membrane bilayer vesicles formed by self-assembly of lipids constitute a minimal protocell – embedded primitive pigments such as porphyrins can convert photonic energy into electrochemical proton gradients for phosphorylation (Morowitz et al. Reference Morowitz, Heinz and Deamer1988). The cytoskeleton network of actin, microtubules and intermediate filaments in biological cells are fundamental in providing the basis for cell shape, compartmentation and intracellular traffic. The incorporation of hydrogel fibrillary networks self-assembled by hydrophobic interactions and hydrogen bonds in liposomes presents a simplified artificial analogue of a biological cell with an internal cytoskeleton (Brizard & van Esch Reference Brizard and van Esch2009). Artificial cells must include information-laden replicating molecules, polymerases to catalyse replicating molecules, and a translation system to convert genetic information into protein synthesis instructions (Pohorille & Deamer Reference Pohorille and Deamer2002). The protocell of the RNA world is premised on two RNA replicating molecules – one as a genomic template and the other as a ribozyme that catalyses the synthesis of amphiphilic lipids for the membrane (Szostak et al. Reference Szostak, Bartel and Luigi Luisi2001). Vesicles can then reproduce through amphiphilic growth until the lipid capsule becomes unstable and fissions into two vesicles. These arguments suggest that encapsulation from the environment is an essential feature of life.
Self-replication
The autopoietic view of life does not consider self-replication specifically, so autopoiesis may be regarded as a necessary but not sufficient condition for life (Luigi Luisi Reference Luigi Luisi2003; Froese & Stewart Reference Froese and Stewart2010). Indeed, according to autopoietic theory, reproduction is not a necessary property of living systems, but is merely a derivative property enabled by the unity of the living system for that unity to be multiplied (Varela et al. Reference Varela, Maturana and Uribe1974). It is conceivable that a living organism could exist that was incapable of self-replication – such an organism would have to emerge without any evolutionary heritage through pure self-organization. It has been suggested that a spontaneously emerging self-replicator on Earth would not have possessed an overall complexity exceeding 100–200 bits over the background environment (Jacobson Reference Jacobson1959). A necessary adjunct to autopoiesis is autogenesis which emphasizes the emergence of self-replication (Csanyi & Kampus Reference Csanyi and Kampus1985). Autopoiesis has been proposed to be part of a larger concept of (M, R) systems where M, metabolic activity and R, repair activity collectively imply that self-repairing metabolism is capable of replication (Letelier et al. Reference Letelier, Marin and Mpoodoziz2003). This implies that not all replicating systems are autopoietic. Autopoietic systems are further characterized by operational closure where metabolic processes feed into each other in a circular causal network. Autopoiesis must be supplemented with an open-ended evolution property (Ruiz-Mirazo et al. Reference Ruiz-Mirazo, Pereto and Moreno2004), for it is the evolutionary process that yields increased organismic complexity over time (Saunders & Ho, Reference Saunders and Ho1976).
Evolution is a learning process whereby information is extracted from the environment and incorporated into the lifeform. Darwinian evolution is a universal phenomenon premised on the notion of stored (genetic) information for which three properties occur: (i) variation (errors) due to random genetic mutation, (ii) selection favouring fitter individuals to a specific environment and (iii) inheritance whereby fitter individuals copy their characteristics onto the next generation through their offspring. The last implies the ability to generate copies, i.e. self-replication. Evolutionary capacity thus implies a self-reproductive capacity for mutation to operate during the copying process. Using λ-calculus, it has been demonstrated that self-replication logically precedes self-maintenance (Fontana & Buss Reference Fontana and Buss1994). By definition, autopoietic systems are to be differentiated from allopoietic systems. The latter converts raw material into a product (comprising an organized conjunction of those materials) other than itself, an example being a factory. Clearly, by definition, a self-replicating entity is not an allopoietic system because it yields products of itself. ‘The living is a factory that makes itself from within’ (Luigi Luisi Reference Luigi Luisi2003). Self-replication may thus be viewed as an essential ingredient of life. The so-called mule problem is readily dismissed in that reproductive capacity is potential rather than actual. Furthermore, evolutionary capacity is a property of populations rather than individuals – mules are part of an evolving gene pool of a population (Chodasewicz Reference Chodasewicz2014).
The earliest form of replication may have been uncoded and implemented through autocatalytic sets of organic polymers with Lamarckian inheritance (Gabora Reference Gabora2006). Regardless of the nature of early self-replication, life may have emerged when a non-metabolic replicator acquired metabolism to exploit external energy sources. It thus acquired purposefulness (Pross Reference Pross2008). Purposefulness as a characteristic of life has its roots in physicochemical reactions of metabolism during replication. Purposefulness arises through dynamic kinetic stability maintained by a persistent metabolism as distinct from thermodynamic stability representing a state of maximum decay. Kinetic stability is a unique property of persistent replicating systems and is dependent on the environment (Pross Reference Pross2005a). The competition for finite resources imposes a trend towards increasing kinetic stability –survival of the most stable. Complex replicators are kinetically more stable than simple replicators, hence driving evolution towards greater complexity (Pross Reference Pross2005b). These arguments suggest that self-replication is indeed, an essential property of life.
The definition of life is implicit in the origin of life question of whether metabolism or self-replication was the first property of life to emerge. In terrestrial life, folded molecular chains effectively implement computational processes as program strings, while molecular encoding in genes acts as a blueprint for the construction of these molecular chains, and through templating, the molecular codes implement their self-reproduction (Laing Reference Laing1975). The two aspects of life – genotype and phenotype – appear inextricable.
The RNA world attempts to circumvent the chicken-and-the-egg problem of metabolism versus genetic replication (Lazcano Reference Lazcano2010). RNA comprises a phosphate–ribose–phosphate backbone with nucleotide links forming a chain polymer. Within the RNA world, RNA acts as both a store of genetic information and as an organic catalyst for metabolic activity. This RNA world was enabled by its possession of both genotype (as RNA bases) and phenotype (as ribozymes). Information is held in the nucleotide base sequence while its folding pattern forms the catalytic structure. It suggests that RNA polymers (ribozymes) could catalyse their own synthesis and self-replicate, thereby establishing both metabolism and self-replication, perhaps encapsulated within a vesicle. The rRNA of the ribosome is a type of ribozyme. RNA replicase is an RNA molecule that is a template for genetic information and acts as an RNA polymerase to replicate itself. A simple protocell would comprise RNA replicase within a vesicle using nucleoside triphosphate (NTP) for energy (Szostak et al. Reference Szostak, Bartel and Luigi Luisi2001). As a single RNA molecule cannot simultaneously act as template and polymerase, two RNA molecules are required. Hence, molecular networks of RNA are required to sustain cooperative cycles of production (Pross & Pascal Reference Pross and Pascal2013). RNA replication requires the RNA replicase protein which begs the need for a supporting metabolism, i.e. an interconnected gene–metabolism–encapsulation triumvirate network (Rasmussen et al. Reference Rasmussen, Chen, Nilsson and Abe2003). Autocatalysis involves the presence of a product catalysing the original reagents – thus RNA acts as a template for assembling further RNA molecules. The emergence of genetic encoding of metabolic and lipid reaction rates is believed to involve some kind of autocatalytic feedback mechanism (Hordijk Reference Hordijk2013). Alternatively, peptide nucleic acid (PNA) has a hydrophobic polypeptide rather than phosphate–sugar backbone but with the same base pairing as DNA or RNA. PNA can act as an electron relay system with modifications. PNA replicates through ligation rather than the ribozymes that act as their own replicase required of RNA replication. The insertion of non-organic material into a proto-organism emphasizing a building blocks approach offers one possible transition between non-living and living matter (Rasmussen et al. Reference Rasmussen, Chen, Nilsson and Abe2003). However, the existence of RNA rather than PNA in today's terrestrial biology as a mediator between DNA and proteins favours the RNA world. RNA performs multiple functions – mRNA constitutes transportation mechanisms, tRNA constitutes jigs and rRNA constitutes the planner and controller for the ribosomes. However, RNA replication is more prone to error than DNA replication, and so subject to strong evolutionary processes. The DNA–protein world may have emerged from the RNA world through retroviral-like mechanisms. A protocell containing a self-replicating catalytic DNA may have represented the first living organism. Although specific to life on earth, the RNA world incorporated metabolism, self-replication and encapsulation. Life then is a collective set of properties.
Control systems
Cybernetic systems are another necessary and complementary component of living systems involving specific sensory inputs triggering specific actions, i.e. its internal processes are adapted to its environment (Bourgine & Stewart Reference Bourgine and Stewart2004). This implies that control mechanisms are an essential feature of life.
It may be instructive to examine synthetic biology in the Feynman tradition that creating yields understanding. To create artificial cells, simple biological cells may be simplified further (top-down) or assembled de novo from scratch from biological and non-biological materials (bottom-up) (Rasmussen et al. Reference Rasmussen, Chen, Deamer, Krakauer, Packard, Stadler and Bedau2004). In bottom-up synthesis, lipid vesicles may be self-assembled by amphiphilic aggregation encapsulating self-replicating RNA or the simpler PNA. Synthetic biology is also a bottom-up process that blurs the distinction between naturally evolved living organisms whose only goal is survival and human-designed and manufactured machines of inorganic material that serve human-dictated purposes (the latter being the subject-matter of this paper). In synthetic biology, new functionality to biological cells (acting as a chassis) can be introduced to complement natural biological nucleic acid/protein biochemistry (Benner & Sismour Reference Benner and Sismour2005). Self-replication in biology occurs through molecular template copying of genetic information which encodes physical properties of the lifeform. This requires molecular pattern recognition of which Watson–Crick pairing of large purines with small pyramidines (A–T and G–C) through hydrogen bonds is an example. An indispensable part of this recognition process is the phosphate–ribose backbone with its repeating charge. Shuffling hydrogen bond links permits the addition of four further base pairs forming a synthetic genetic alphabet (Sismour & Benner Reference Sismour and Benner2005). Similarly in proteins, different amino acids from the standard 20 can be introduced. Amino acids in polypeptide chains interact with each other due to their folding. Artificial polypeptides can be constructed from amphiphilic side chains of amino acids that replicate specific protein folding (Deming Reference Deming1997). This complements pre-existing biology within the constraints of biochemistry. Synthetic biology is exploring the limits of life further by attempting to re-design the biological realm to construct genetically engineered machines (Gibbs Reference Gibbs2004). There are three main thrusts: (i) synthesis of a minimal protocell; (ii) synthesis of minimal genomes; (iii) genetically engineered machines. Synthetic biology applies an engineering philosophy to biological components to build engineering functions from those biological components. It is the complement to the self-replicating machine concept explored later which uses engineered components to build a (biological) self-replication function. The major thrust of synthetic biology is the manufacture of a library or toolbox of logical functions from standardized biological genes that can yield biological components assembled to implement a specific device (McDaniel & Weiss Reference McDaniel and Weiss2005). These genetic segments of DNA – BioBricks – encode for biological components that implement known characteristics such as logic gates, sensors, actuators, etc that can be assembled like molecular Lego into cellular circuits such as logical implication and even more complex logics such as filters and feedback loops (Endy Reference Endy2005). Bistable switches have been developed which can act as memory elements which are heritable from one generation to the next. Similarly, the inverter BioBrick implements a NOT function which, with the AND BioBrick, provides logical universality through the composite NAND operator. These would be expressed within synthetic cells acting as chassis organisms. Nevertheless, they would retain their autopoietic character including self-production and self-reproduction and so be subject to evolutionary pressures. Hence, it would be human-designed in origin but will exist as a biological organism (Deplazes & Huppenbauer Reference Deplazes and Huppenbauer2009). This follows a similar logic to the self-replicating machine. However, in the Registry of Standard Biological Parts, many of the synthetic biology parts were of poor quality and did not function reliably, did not work when combined into circuits, were too complex to use, induced complex undesired reactions in the host cell, and desired functions often operated periodically rather continuously (Kwok Reference Kwok2010). These difficulties will not plague the engineered self-replicating machine presented later. There are more severe limits to synthetic biology (Zakeri & Carr Reference Zakeri and Carr2015). The chief problem in creating biological circuits is their long operation times compared with electronic circuits. Furthermore, as designed genetic circuits become larger and more complex, there is an increasing probability of mutation or genetic rearrangement. As manufactured functionality does not improve the fitness of the chassis organisms, there will be selection pressure to evolve out that functionality over time. Indeed, this occurs very rapidly, suggesting that fears of synthetic organisms escaping and engaging in damaging interactions with the outside environment are unfounded (Tucker & Zilinskas Reference Tucker and Zilinskas2006). Building in redundancy may improve the robustness and stability of the synthetic function but it will not suppress evolutionary processes. If a mechanism is found to suppress evolution, then these synthetic organisms would no longer be alive (Schark Reference Schark2012). Autopoiesis would be compromised because continuous self-regeneration would no longer operate. The organism would have a complex organization but no longer any self-organization capacity. However, it has been asserted that self-organization with complex global phenomena emerging from simple local microscopic phenomena is, in fact, a functional property of life (Bedau Reference Bedau, Varela and Bourgine1992).
Movement in some form is implicit in biological systems in order to acquire and transport nutrients (see the Appendix on molecular motors). All molecular motors convert chemical energy into mechanical work. Switching between biological conformations which is fundamental to biological molecules may be regarded as a form of self-assembly which can be described formally as graph grammars (Klavins Reference Klavins2004). Molecular motors evolved from such molecular switches – kinesin, myosin and G proteins appear to have evolved from an ancestral nucleotide-binding protein while F1-ATP synthase seems to have evolved from a different nucleotide-binding protein (Vale Reference Vale1999). Kinesins comprise a superfamily that has evolved a diverse set of functions through gene duplication while dynein molecular motors appear to have been conserved (Goldstein Reference Goldstein2001). All however evolved in early eukaryotes in a wide variety of cargo-handling types, sometimes with different motors recruited for similar functions – actin is used for membrane transport in plant cells while microtubules are used for the same in animal cells (Vale Reference Vale2003). While certain motors may be dominant in different species, mitotic kinesins are common to all eukaryotes. Porters – such as kinesin – that walk along microtubules have high duty ratio while rowers – such as myosin – that operate in teams have low duty ratio. Kinesin is the most abundant motor in most eukaryotic cells and is similar to myosin insofar as they both comprise two heavy chains and two light chains. The heavy chains fold into the two globular heads each containing the ATPase sites. Kinesin is the smallest motor protein of ~340 amino acids compared with ~850 amino acids of myosin and ~1000 amino acids of dynein. Kinesin and myosin motors respectively share a common core structure and function by ATP-powered conformation changes (Kull et al. Reference Kull, Sablin, Lau, Fletterick and Vale1996; Vale & Milligan Reference Vale and Milligan2000). One common feature in both motors is the almost identical γ-phosphate sensor to detect presence or absence of a single phosphate (ADP/ATP) – it comprises two loops that form hydrogen bonds with the γ phosphate. The relay loop moves like a piston with an upstroke on phosphate binding and downstroke on phosphate release. Kinesin and myosin appear to have evolved from a common ancestor with G proteins. Myosin V is a special myosin which transports organelles along actin filaments; kinesins have evolved similar mechanisms for multi-step motion. Motors also operate in prokaryotes. Plasmid and chromosome segregation in mitosing prokaryotic cells is undertaken by homologues of actin filaments acting as cables (Gerdes et al. Reference Gerdes, Meller-Jensen, Ebersbach, Kruse and Nordstrom2004). Furthermore, these and other actin-like proteins such as MreB and ParM act like centromeres for plasmid segregation during mitosis (Egelman Reference Egelman2003). MreB cell shape proteins form large spirals in rod-shaped bacterial cells acting as a precursor cytoskeleton – MreB proteins are absent in spherical bacteria, however. MreB proteins have a similar structure to filamentous actin in eukaryotes and indicate that the eukaryotic cytoskeleton originated in the prokaryotic domain (van den Ent et al. Reference van den Ent, Amos and Lowe2001a).
Bacterial FtsZ (filamentous temperature-sensitive) protein involved in bacterial mitosis is similar to eukaryotic tubulin that is the basis of its cytoskeleton (van den Ent et al. Reference van den Ent, Amos and Lowe2001b). FtsZ is a ubiquitous and highly conserved GTPase in most eubacteria including chloroplasts and mitochondria and is common in archaea. Although there is limited amino acid homology (10–18%) between FtsZ and α- and β-tubulins of eukaryotes, they are similar in structure with common folds suggesting a common evolutionary origin. In eukaryotic cells, however, chromosome segregation during mitosis is undertaken by microtubule fibres fixed to the centromere which pull the chromosomes apart (Taylor Reference Taylor2001). Eukaryotic cells can form extended mitochondrial filaments forming cable networks connected by intermitochondrial junctions for the transmission of energy through the cell (Skulachev Reference Skulachev2001). It has been suggested that fibres are a ubiquitous feature of biological material – they form the cytoskeleton as the basis for cytoplasmic streaming, cilia and flagella movement, chromosome migration, cytokinesis and muscle contraction (Frixione Reference Frixione2000). The cyclic reaction of a motor protein with a cytoskeletal fibre is a common architecture – the myosin motor on actin filaments and the dynein and kinesin motors on microtubule filaments. On binding with the filament, the motor protein in each case undergoes a conformation change (power stroke) to generate a movement step. The motor then releases the filament before rejoining further up the filament for a repeated step.
Bacterial flagella are powered by motors that can rotate in either direction (Blair Reference Blair1995). When the bacterial motor turns counter-clockwise, the filaments of the flagellum form a bundle that propels the cell along a smooth trajectory. If a motor reverses direction clockwise, the bundle unfolds into an array of individual uncoordinated movements generating tumbling. Typical motion is essentially alternation between runs and tumbles, i.e. a random walk. Directionality involves controlling the frequency of reversals in response to sensory detection of stimulants. The detection of attractor stimulants such as glucose suppresses the clockwise tumbling. Conversely, clockwise runs in unfavourable directions are suppressed by repellants. When bound to a repellant, Tar protein receptors in the cell membrane stimulate autophosphorylation of CheA which transfers its phosphate group to CheY. Phosphated CheY then interacts with the flagellar motor to increase the probability of tumbling by clockwise rotation of its flagellar motor. When bound to an attractor, CheY remains unphosphorylated and increases the probability of straight swimming by anticlockwise rotation of the flagellar motor. In fact, it is the change in concentration over time that is measured, requiring a short-term memory capability implemented by methylation of the chemoreceptors. Hence, the bacterium propels itself in the direction of higher concentrations of nutrients. Bacteria can also form highly complex colonies with the emergent functional and cognitive properties of a multicellular super-organism. These bacterial communities exhibit a number of modes of inter-cell biochemical signalling such as quorum sensing and chemotactic sensing (Ben-Jacobs Reference Ben-Jacobs2009).
In prokaryotes, most movement is passive though it is essential as a life function nonetheless. In terrestrial water-laden environments, nutrients flow passively so that static organisms can intercept them. Once acquired, these nutrients are ingested by bacteria and migrate within the bacterial cell by passive diffusive processes. Some bacteria have invented rotary motors to drive flagella to move in search of nutrients more efficiently. Furthermore, in some bacteria, the rudiments of internal transport tracks have evolved. In eukaryotes, such tracks are adopted for internal movement of cargo within the cell. They are also spanned by linear ‘legged’ motors which tend to be large in comparison with rotary motors. Is it not conceivable for some postulated exo-bacteria to have evolved proto-tracks spanned by bacterial rotary motors similar to a rack-and-pinion gear in, perhaps, a more hostile environment? My contention is that movement to acquire and transport nutrients is a necessary condition for life, a consideration not usually appreciated given that Earth's environment often provides this facility for free at the scale of bacteria. Furthermore, internal/external is defined relative to an enclosing membrane – movement internally and externally achieves the same goal of accessing resources for consumption. We contend that movement implies a cybernetic control system as a necessary condition of life.
Life as a list of properties
Hence, we are back to a list of properties of life embedded in an environment from which it must consume resources. The properties of life may be ascertained from the bacterial genome and its coding – a typical bacterial genome comprises 30% non-protein coding genes, 10% for energy metabolism, 17% for genetic transcription and translation, 12% for cell transport and 8% to cell envelope proteins such as adhesins (Bloom Reference Bloom1995). Our generalized list of properties is thus:
(i) Self-maintenance and growth through metabolic processes through the ingestion of matter and energy and the excretion of waste;
(ii) Self-boundary through cellular encapsulation from the environment by constructive metabolism;
(iii) Self-reproduction capability and therefore capacity to evolve in response to environmental pressures;
(iv) Cybernetic facility through adaptive control system mechanisms to adapt to the environment.
The unofficial NASA definition of life attributed to Gerald Joyce is: ‘Life is a self-sustained chemical system capable of undergoing Darwinian evolution’. Although there are dissenters to this definition (Luisi Reference Luisi1998), it is succinct and there are a number of characteristics implicit in this definition. Chemical system implies metabolic biochemistry and Darwinian evolution requires self-reproduction. As a chemical system, it excludes artificial life but does not exclude engineered life. Capacity for Darwinian evolution does not require life to have an evolutionary history, only an evolutionary future, though an evolutionary past may be inferred in natural systems. All life on the Earth is carbon-based involving water as the fundamental biochemical solvent and most astrobiology work is premised on this. The lack of detection of organic material on Mars by Viking's gas chromatograph mass spectrometer (though this result has since been contested) trumped the detection of labelled CO2 from its labelled release experiment on that basis (the overall conclusion has not changed even in the light of contested measurements). Hypothetical silicon-based life or liquid ammonia as a biochemical solvent has not been entertained seriously. For instance, Si does not form double sp or triple sp2 bonds but its d orbitals do permit the formation of dπ–pπ bonds. Nevertheless, there have been attempts to consider life from a broader perspective (Schulze-Makuch & Irwin Reference Schulze-Makuch and Irwin2008). The work presented here is part of an ongoing research programme that will explore several key open issues in engineered life including ‘How does life arise from the nonliving?’ (Bedau et al. Reference Bedau, McCaskill, Packard, Rasmussen, Adami, Green, Ikegami, Kaneko and Ray2000). In doing so, we retain the control system property of life. As pointed out in (Brack & Trouble Reference Brack and Trouble2010), robotics has been emulating many of the characteristics of life including replicating and evolving behavioural properties through genetic algorithms (Eiben Reference Eiben2014). Evolutionary robotics involves using genetic algorithms to evolve neural networks to implement specific behaviours on physical robots. Of great interest is coevolution of both behaviour and bodily morphology. Robotics to date, however, has not yet demonstrated self-replication of bodily form. This is what we explore here.
Part B: What could be life?
Self-replicating automata
Looking to artificial life as an exploration of non-terrestrial life, the key motivation is to broaden from life-as-we-know-it to life-as-it-could-be (Umerez Reference Umerez2010). There are three approaches: (a) soft artificial life involving software simulations; (b) hard artificial life involving hardware implementations; (c) wet artificial life involving chemical implementations (Bedau Reference Bedau2003). Our approach is a combination of all three to form a fourth approach: (d) engineered life involving manufacturing physical resources.
Cellular automata (CA) constitute the main modelling environment for artificial life. Indeed, there are many examples of CA models of different biological phenomena including developmental biology (Ermentrout & Edelatein-Keshet Reference Ermentrout and Edelatein-Keshet1993; Calabretta Reference Calabretta, Bandini, Serra and Suggi Liverani1998). CA are highly abstract but serve to illustrate the logic of life divorced from the physicality of life. CA comprise a chain (1D) or lattice (2D) of cells, each of which can be in one of a finite number k of possible states. They are updated every discrete timestep with a well-defined transition rule that depends on the state of its R neighbours. The simplest CA is a 1D string of cells, the states (with two states 0 and 1) of which are dependent on the flanking neighbours. For 2D arrays, there are two commonly adopted neighbourhoods: the von Neumann neighbourhood consists of the R = 4 neighbouring cells to the north, south, east and west; the Moore neighbourhood consists of the R = 8 neighbouring cells to the north, south, east, west, north-east, south-east, north-west and south-west. Hence, each cell is a finite state machine with transition rules applied only locally based on the local neighbouring states. The CA can simulate different Wolfram universes of logic (Wolfram Reference Wolfram2002): in class 1, initial configurations evolve to a fixed configuration; in class 2, initial configurations evolve to a periodic cycle of configurations; in class 3, initial configurations evolve into chaotic behaviour; in class 4, initial configurations evolve complex localized structures with global behaviours. Class 1 and 2 CAs exhibit only localized information transmission (λ = 0); in class 3 and 4 CAs, information transfer is however global (λ > 0). The dynamic Langton λ factor which varies from 0 to (1–1/k) – defined as the fraction of active output states in the rule table – attempts to quantify these classes and defines a critical value λc that corresponds to class 4 at the ‘edge of chaos’. It has been suggested that this class possesses the capacity for universal computation but it has been questioned whether CAs can be quantified in this fashion. Nevertheless, the notion of computability in CAs is an important property which defines the capacity of the specific CA (Mitchell Reference Mitchell, Gramss, Bornholdt, Gross and Mitchell1998). Central to computability is the Turing machine and the limit imposed by the Turing halting problem that there exists no general algorithm that can predict whether an algorithm halts, i.e. halting is undecidable (Turing Reference Turing1937). However, halting is decidable in a two-state CA with a von Neumann neighbourhood. The simplest CAs that support universal computation have k = 18 and R = 3 in 1D and k = 3 and R = 3 in 2D (Wolfram Reference Wolfram1985). A 1D CA with (m + n + 2) states can simulate a Turing machine with n states and m alphabetic letters.
Conway's Game of ‘Life’ is a CA system of 2D cells that illustrates the phenomenon of emergence. Each cell has two (binary) states – it is either alive (1) or dead (0). The next state is determined by the state of its eight neighbours (Moore neighbourhood) according to specific local rules. If there are three living neighbours, its state is alive; if there are two or less or four or more living neighbours, the cell dies of loneliness or overcrowding respectively. From the interaction of such simple cells, highly ordered complex structures can emerge (Crutchfield Reference Crutchfield1994) – the glider is a local pattern that moves through the CA array diagonally as an integrated unit. The glider gun configuration emits regularly spaced gliders every 30 time steps. If a glider encounters a square block of four cells, they collide and mutually disintegrate – a law of physics that is not specified in the CA rule set. Hence, complex structures can emerge from very simple cell rules. Although the rules are local, they can yield globally emergent phenomena such as universal computation. ‘Life’ is capable of universal computation by exploiting glider guns and gliders. Logic functions are constructed by interacting streams of gliders. For example, a NOT gate is implemented by two perpendicular gliders colliding and annihilating each other. AND/OR gates can be implemented similarly while memory is implemented through circulating gliders. Of course, such implementations of universal computation are cumbersome and impractical. Artificial chemistry (AC) such as Squirm3 has successfully modelled the emergence of self-replicating molecules (Hutton Reference Hutton2002). AC is a form of CA that defines a triple (S,R,A) where S = set of all possible molecules, R = set of collision rules and A = algorithm defining physical rules. Unlike CAs, artificial chemistries do not constrain the movement of molecules to a regular grid. Bonds are formed between atoms on collision but bonds can be broken by reactions. A set of eight reactions defining how two different atoms combine to form molecules are sufficient to permit the spontaneous emergence of self-replicators from a primordial soup of atoms.
The first systematic consideration of self-replicating machines was that of John von Neumann (von Neumann & Burks Reference von Neumann and Burks1966). His 2D CA-based self-replicating machine required each cell to have 29 states including an inactive state. The CA model comprised five major subsystems: (i) a program of instructions; (ii) a sequencer; (iii) a controller; (iv) a constructor arm; and (v) a workspace environment. A more physically realistic kinematic model of the von Neumann self-replicator comprised eight different components in two major systems – four logic components for control signals and four mechanical elements for structure and mobility. The two major subsystems were: (i) a tape unit, itself comprising a linear memory tape and a tape control unit, i.e. a universal Turing machine (computer); (ii) a constructing unit comprises a robot arm and an arm control unit, i.e. a universal constructor. The control program is stored on the memory tape that instructs the robotic arm to select, acquire and assemble components from a sea of pre-fabricated constituent parts in the working environment – in effect, it was a self-assembling machine. The construction unit signals the tape unit and reads the instructions as a set of (x 0, y 0) start coordinates and (Δx, Δy) movements for the robot arm. Thus, the universal Turing machine and the universal constructor send sequences of signals to each other to control the construction of the machine according to the program blueprint. The robotic arm picks up a part from the environment and inspects it to compare it with the program's specifications. If correct, it joins this new part with the ongoing assembly. The robotic arm model of the universal constructor is essentially a pick-and-place assembly manipulator. If the tape constitutes a description of itself, the constructor arm builds a copy of itself. It then copies its program and inserts it into the new machine. The machine (analogous to proteins) that is constructed is determined by the construction program stored on the memory tape (analogous to DNA) – it is a universal constructor comprising a universal Turing machine to store and interpret information on the program tape and a robotic arm to act on those instructions to build the phenotypic expression of those genetic instructions. The universal constructor can construct any machine specified on its tape given the required raw materials, energy and information. One such program is a self-specification to construct a copy of itself – a self-replicating machine (Freitas & Merkle Reference Freitas and Merkle2004). To pass on this self-replication capability, the tape must be copied into the offspring. Thus, the instructions on the tape are used in two ways – first, it is read and interpreted (and so executed) during construction (analogous to biological translation) then it is read passively (uninterpreted) during copying (analogous to biological transcription). A computer model of the von Neumann self-replicator has been simulated (Pesavento Reference Pesavento1995). In biology, the process is more complex. During transcription, the nucleotide base sequence of a strand of DNA is transcribed onto messenger mRNA with the complementary sequence. During translation, mRNA is associated with a ribosome (constructed from one large 50s and one small 30s ribosomal rRNA and proteins) to map to the equivalent amino acid sequences. The mRNA sequence is encoded into triplets, usually beginning AUG and ending with stop codons UAG, UAA or UGA. The mapping process is realized through tRNA and aminoacyl-synthetases with tRNA acting as an adapter molecule between mRNA and an amino acid. There are at least 64 different types of tRNA and specific types of aminoacyl-synthetase. mRNA is aligned with the ribosome to ensure that the ribosome reads the mRNA codons.
Simplifications to von Neumann's formulation exploit sheathed loops where the instruction sequence structure is surrounded by a sheath to provide a path for signal propagation. The sheathed loop was a modified Codd periodic emitter loop which implemented a time-delay/memory function which formed the basis of Codd's 8-state self-replicator (Hutton Reference Hutton2009). Data sequences circulating around the instruction loop would intercept the origin of the constructing arm causing a copy to propagate along the arm and causing it to extend or bend. If the data sequence is a replication code, then the sheathed loop becomes self-replicating with the arm printing a second loop that detaches and replicates ad infinitum (Langton Reference Langton1984). The Langton replicating sheathed loop of 86 active eight-state cells with 219 transition rules allowed abandonment of universal construction. The Langton loop is a pure self-replicator in which a closed loop transmits signals to perform the dual function of both interpreted and uninterpreted instructions. Replacing the sheath around the instruction loop with a growth cap at the tip of the constructing arm retains simple self-reproducing structures of 32 active eight-state cells with 177 transition rules (Reggia et al. Reference Reggia, Armentrout, Chou and Peg1993). In fact, even smaller self-replicating entities are permissible such as six active eight-state cells with 91 transition rules and 12 active six-state cells with 46 transition rules. Hence, universal construction is sufficient but not necessary for self-replication. Such pure self-replicators are not universal constructors and are highly simplified (analogous to prions) but cannot be considered models of life. We submit that universal construction is a universal feature of life.
The self-replicating machine concept – which reads its self-description – can be logically extended to a machine that can self-replicate by self-inspection, i.e. examine itself to infer a complete structural description of itself (Laing Reference Laing1979). The key capability is an active cellular string in sliding contact with another passive cellular string that accesses the passive string data. Hence, a universal constructor can, by self-inspection, can construct a copy of itself. This is biologically implausible as it represents a Lamarckian evolutionary process. However, the ability to self-inspect provides a mechanism for self-repair with reference to pre-existing instructions. The Godel machine is a further extension which is a universal computing machine that can rewrite itself if a proof search embedded in the initial algorithm can prove that the rewrite yields rewards to a utility function, i.e. it is a learning algorithm that interacts with its environment (Schmidhuber Reference Schmidhuber, Goertzel and Pennachin2007). Learning algorithms are premised on the notion that the future is a reflection of the past (as is evolution). In this way, such self-changes through self-introspection (in essence, self-consciousness) allow the system to step outside of itself thereby circumventing the limits of a closed arithmetic system. Self-reproduction by self-inspection rather than a stored program of instructions has been demonstrated in the form of analogue control circuitry (Suthakorn & Chirikjian Reference Suthakorn, Chirikjian, Ang and Khatib2006). Such circuitry is 2D in nature so does not possess volume, thereby ensuring that the system is completely observable. The system observed the configuration of colour-coded electronic components serially using a phototransistor sensor mounted on a Cartesian gantry system. It selected a copy of the currently observed component from a feeder. By serially observing the circuit components, component copies were assembled in sequence until the entire circuit was replicated. Of course, self-replication by self-inspection requires complete self-observability, a condition that in general will not be valid. Self-inspection illustrates that the logic of self-replication opens up possibilities that go far beyond the strictures of life on Earth and may be of interest to the astrobiological community.
Notwithstanding all this, von Neumann's self-replicator is more a self-assembling system rather than a self-replicating system as it involves assembling a series of parts or components to construct a complete configuration. A recent example of such a self-assembling system employed a set of cubes, each of which was divided into two halves that could rotate relative to each other in a diagonal plane (Zykov et al. Reference Zykov, Mytilinaios, Adams and Lipson2005). Each face of the cube was equipped with an electromagnet permitting cubes to attach to each other. A tower of such cubes can pick up other cubes in its environment to construct a copy of the tower, i.e. self-replicate after a fashion. Self-assembling structures are widespread in both chemistry and biology. Although molecular self-assembly is ubiquitous in the biological world, we shall not consider it further here. The environment in reality constitutes a soil of raw material rather than a sea of ready-made components. Practical self-replication involves extracting and processing materials into complete parts or components. An early example of this concept for the terrestrial environment was the artificial living plant concept which was to absorb air, water and soil as raw materials using solar energy to construct copies of itself (Moore Reference Moore1956).
For our self-replicating machine, we are attempting to demonstrate 3D printing of electric motors (the basis of the constructing arm) and electronic circuitry based on vacuum tubes (the basis of the computer) as the fundamental components of a self-replicating machine. These critical components will provide an existence proof that a 3D printer-based self-replicating machine can be constructed. We approach our self-replicating machine through four major biological principles.
Biological Principle 1: materials closure
The first issue to consider is the materials and component inventory required to construct our self-replicating machine. Materials (food) must be acquired from the environment – for an autotroph, this is inorganic material for both engineered and biological organisms. In the case of the Moon, the lunar regolith must be transformed into elementary parts to be assembled into the final product – this is in-situ resource utilization (ISRU). Self-replicating machines on the Moon were proposed in an early study (Freitas & Gilbreath Reference Freitas and Gilbreath1980) and subsequently developed further but the latter were self-assembling concepts based on Lego bricks (Chirikjian et al. Reference Chirikjian, Zhou and Suthakorn2002; Ellery Reference Ellery2016a,Reference Elleryb,Reference Elleryc). We begin with a raw materials list for our self-replicating machine. The materials ingested by the self-replicator are also the first radical departure from the materials adopted in synthetic biology. Engineering metals have high strength and stiffness (robust) – commonly metals – while biological materials have low strength and stiffness but high toughness and elasticity (adaptable) – commonly non-metallic elastomers – which is particularly useful for mechanical energy storage (Ellery et al. Reference Ellery2004). Biological materials are synthesized at moderate temperatures through the use of catalysts while engineering materials are manufactured at high temperature. An interesting question that emerges is ‘is life restricted to low-temperature regimes?’ Enzymes are biological catalysts that serve to increase the rate of biochemical reactions. If organic material is the elan of life, then life will be restricted to moderate temperature regimes. However, it is the chemical reaction that constitutes metabolic function, the enzyme merely facilitating it. If the logic of life is independent of its material substrate, then there is no reason why engineering materials cannot provide the basis for life. There are some precedents for such a notion.
Clays are non-organic minerals that provide surfaces and cavities that constrain the formation of 3D conformation structures from simple precursors such as HCN, HCHO, etc. It has been postulated that biological catalysts evolved from inorganic catalysts such as mineral surfaces (Cairns-Smith Reference Cairns-Smith1990). Montmorillonite (Al4(Si4O10)2(OH)4.xH2O) clay may have acted as one of the first organic catalysts in the origin of life through the RNA world (Ferris Reference Ferris2005) and perhaps even prior to the RNA world as the first medium of genetic information storage (Cairns-Smith Reference Cairns-Smith1987). The clays montmorillonite and illite provide catalytic mineral surfaces for the polymerization of 20–50 monomer-long oligomers of nucleotides and amino acids, respectively (Ferris et al. Reference Ferris, Hill, Liu and Orgel1996). Montmorillonite, in particular, can catalyse the polymerization of oligopeptides and oligonucleotides (Schwartz Reference Schwartz1996). Montmorillonite may have catalysed the synthesis of RNA polymers of length greater than 40 bases in length as required to act as both template and catalyst (Ferris et al. Reference Ferris, Joshi, Wang, Miyakawa and Huang2004). Specific catalysts for polymerization and replication could be implemented using clays within a protocell. The postulated RNA world was probably preceded by inorganic replicators such as crystal defects in clays, and mineral surfaces may have provided key metabolic processes in a protocell (Wachtershauser Reference Wachtershauser1990). Inorganic metabolism such as the iron–sulphur world is consistent with hydrothermal vents with such proto-metabolism, possibly enhanced with hypercyclic feedback systems (Szostak et al. Reference Szostak, Wasik and Blazewicz2016). If a catalyst (inorganic or organic) is replaced or supplemented by higher thermal energy, the chemical reaction is still hastened. Does this absolve it of its biological character? We suggest not. Rather than CHONPS, we are adopting a minimal suite of engineering materials that can implement all the functions required of a self-replicator in its environment – we focus on the Moon for a number of reasons not relevant to this argument (Ellery Reference Ellery2016a,Reference Elleryb,Reference Elleryc). This approach brings to mind the emphasis placed on physicochemical processes on the nature of life (Schultze-Makuch & Irwin Reference Schultze-Makuch and Irwin2004). Our self-replicator must extract its restricted set of feedstock materials – iron, silicon, nickel, cobalt, tungsten, selenium, silica-based glass, silicone plastic (siloxanes) and water – from raw materials (mineral ores). The restricted range of required materials must be able to implement all the material functions required – compressive/tensile structures (bones/tendon), thermal insulation and conduction (fat/bloodstream), electrical conduction and insulation (axons/myelin), switching logic (neurons), etc. – whilst minimizing the infrastructure required to extract it (Table 1).
Table 1. Materials inventory required for a lunar photolithoautroph
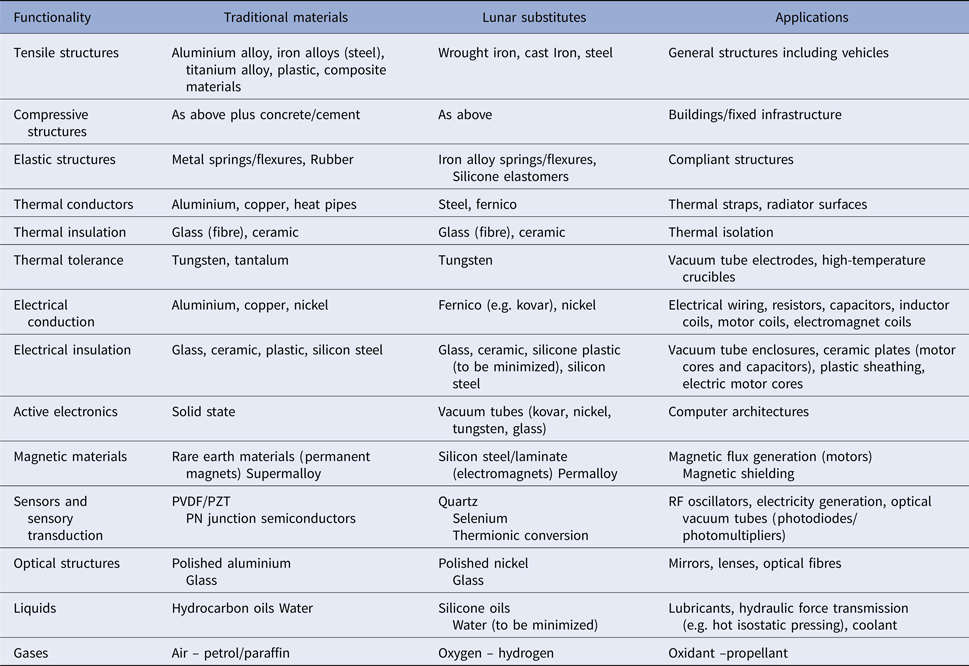
This materials inventory can be readily extracted from surface lunar minerals and near-surface NiFe asteroid material on the Moon. Over the aeons, solar wind has deposited volatiles at an average rate of 3 × 108 particles cm−2 s−1 into the top few mm of soil. However, impact gardening has impregnated this material to a depth of a few metres. Solar wind comprises 96% H2 which has accumulated into a regolith concentration of 20–200 ppm (average ~120 ppm) plus almost 4% He3 (with a He3/He4 ratio of 3 × 10−4 by mass) plus many minor constituents including carbon compounds. The common lunar mineral ilmenite (FeTiO3) in the 35–55 µm grain size range also preferentially adsorbs these volatiles compared with other minerals with the degree of volatile-enrichment increasing at finer grain sizes ~10 µm. Loosely bound volatiles may be thermally evolved from surface regolith at temperatures of 600°C to release 75% of the volatile inventory (this increases to 86% at 700°C, 95% at 800°C and 100% close to 1000°C). Although dominated by H2, additional volatiles – SO2, CO/CO2/CH4, N2, F2, Cl2, etc. will be released. Carbon in the form of CO, CO2 and CH4 volatiles is relatively scarce compared with mineral resources with an average abundance of 120 ppm (Fegley & Swindle Reference Fegley, Swindle, Lewis, Matthews and Gurrieri1993). Fractional distillation may be employed to separate the gas fractions due to their well-separated condensation temperatures – He (4.2 K), H2 (20 K), N2 (77 K), CO (81 K), CH4 (109 K), CO2 (194 K) and H2O (373 K). The synthesis of silicone oils and silicone plastics from syngas-derived from lunar volatiles is outlined in (Ellery Reference Ellery2016a,Reference Elleryb,Reference Elleryc). Silicones are chosen over hydrocarbons for their radiation hardness, high-temperature tolerance, functional properties and economic consumption of carbon.
Heating the mineral ilmenite to ~1000°C in the presence of H2 reduces it to pure Fe, TiO2 and H2O: FeTiO3 + H2 → Fe + TiO2 + H2O. The water may be electrolysed to recycle the H2 and store O2: 2H2O → 2H2 + O2. Iron forms the basis of our self-replicator's tissue but it requires the addition of further elements to provide a range of alloys for different functions – Ni, Co, Si, W and Se. In lunar regolith, Fe, Ni and Co particles are associated together as 5–60 µm inclusions in troilite of meteoritic origin (Wittmann & Korotev Reference Wittmann and Korotev2013). However, it would be desirable to gain access to these materials in more concentrated form. The cobalt content of lunar and terrestrial mantles is similar. The Ni/Co ratio of the Moon's mantle and the Earth's mantle are similar to that of chondrites (Ringwood & Seifert Reference Ringwood and Seifert1986) (Table 2). This suggests that the Moon's abundance of Ni should be similar to that of Earth's mantle. However, Ni is depleted in the lunar mantle by a factor of 3 suggesting a Ni-rich metal lunar core. This constitutes evidence that the Moon was derived from the Earth's mantle after the Earth's core had formed. Nevertheless, lunar igneous rock often contains high Ni (up to 56%) and Co (up to 9%) content in FeNi metal inclusions, especially olivines but sometimes also in plagioclase. Lunar mantle olivine ((Mg,Fe)2SiO4) offers the highest source of associated Ni and Co favouring the lunar south pole Aitken basin where lunar mantle material may have been excavated – however, the crater shallowness suggests that no mantle material was in fact excavated (Wieczorek & Phillips Reference Wieczorek and Phillips1999). The two chief question marks concern the availability of tungsten which is a critical material for thermionic processes and selenium for its photosensitive response. On the Earth, tungsten has an average concentration of 1–2 mg kg−1 and is typically found as wolframite ((Fe,Mn)WO4) and scheelite (CaWO4). It is enriched in several rock-forming minerals including igneous Fe–Ti oxide minerals which can contain up to 10 mg kg−1 W. Its availability on the Moon is limited.
Table 2. Ni and Co abundances in the lunar source material (ppm) [adapted from Ringwood A & Seifert S (1986)]

Meteoritic material is the most likely material to contain high concentrations of iron, nickel, cobalt and tungsten (Ellery Reference Ellery2016a,Reference Elleryb,Reference Elleryc). A NASA proposal to capture a small ~1 m diameter asteroid and re-direct it into lunar orbit offers a potential solution. If a NiFe asteroid were to be selected, a ready source of Ni, Co, W and Se would be available although it would require orbital access. However, asteroidal material is available on the Moon itself. The three largest terrestrial impact craters on Earth are the 250–300 km diameter Vredefort cater in South Africa (2.02 Gy old), the 200 km diameter Sudbury crater in Canada (1.85 Gy old), and the 170 km diameter Chicxulub crater in the Mexico Yucatan (65 My old). Large terrestrial impact craters such as the Vredefort South Africa and Sudbury Canada craters are sources of gold–uranium and nickel–cobalt–platinum, respectively (Reimold et al. Reference Reimold, Koeberl, Gibson, Dressler, Koebert and Henkel2005). The Vredefort deposits were formed from pre-existing archaean deposits prior to impact while the Sudbury Ni–Cu sulphide deposits were formed by the impactor itself. Most Sudbury ores are associated with a sublayer at the base of a 2.5 km thick elliptical igneous lens 27 × 60 km2 in extent with surrounding dikes. Large extraterrestrial impact craters might be expected to yield similar metallic sources. Mascons are high gravitational anomalies on the Moon which are believed to be formed by impact excavation (Melosh et al. Reference Melosh, Freed, Johnson, Blair, Andrews-Hanna, Neumann, Phillips, Smith, Solomon, Wieczorek and Zuber2013; Montesi Reference Montesi2013). Mantle material slowly flowed into the basin causing uplift of the basin as it cooled and contracted, increasing its density. Hence, it is generally concluded that asteroid material of the impactor was vaporized and either escaped into space or was diffused over the lunar surface to form the 1–2% of the meteoritic material in the lunar regolith. However, it is possible that there may be asteroidal ores located within 500 m of the lunar surface if some of the asteroid material survived impact. Low-velocity impacts of small meteorites up to 3 km s−1 permits partial survival of the impactor which will have accumulated over the aeons (Bland et al. Reference Bland, Cintala, Horz and Cressey2001). At high-impact angles, higher impact velocities of 7 km s−1 permit the survival of impactor material which remains localized at the centre of the resulting crater (Bland et al. Reference Bland, Artemieva, Collins, Bottke, Bussey and Joy2008). The fraction of surviving material is directly related to the impact angle, however. Around 2.6% of lunar craters will retain more than 50% of the impacting material – this correlates with at least 600 craters exceeding 10 km in diameter in the highlands and 70 craters of similar dimensions in the mare regions. Such craters may be identified by thin breccia lens thicknesses. These offer potential sources of asteroidal material on the Moon. Strong magnetic anomalies in the northern rim of the South Pole Aitken basin on the lunar farside indicate that they originate from magnetic iron minerals of the impactor (Wieczorek et al. Reference Wieczorek, Weiss and Steart2012; Collins Reference Collins2012). Most of the metallic core of the 200 km diameter asteroid impactor which struck from south to north at 15 km s−1 at a 45° angle was retained in downrange ejecta. In general, most projectile material from near vertical impact velocities below 12 km s−1 appears to fragment but they survive and concentrate into the central peak of the craters created (Yue et al. Reference Yue, Johnson, Monton, Melosh, Di, Hu and Liu2013). The rest of the impactor material is dispersed in ejecta blanket, the crater floor and rim. The olivine in many lunar crater peaks is of asteroidal rather than lunar mantle origin. Beyond 14 km s−1 impact speeds, however, vaporization of the projectile material occurs. Metals appear to have the high survivability to hypervelocity impacts suggesting their incidence in crater floors, walls and ejecta blankets as metal particle fragments (McDermott et al. Reference McDermott, Price, Cole and Burchell2016). This suggests that there will be significant near-surface regions of the Moon which will host high concentrations of NiFe material and its associated Co, W and Se resources (Table 3).
Table 3. Essential iron alloys

Both iron and nickel are plentiful in NiFe meteoritic sources. Cobalt is commonly found in association with Ni. If both are in sulphide form with troilite, they can be reduced with hydrogen by smelting at high temperature and pressure. Tungsten inclusions in NiFe meteoritic material represent one of the most accessible sources of tungsten. Forging by hammer, press, rolling or die is essential for tungsten. Tungsten has the highest melting point of all metals at 3422°C. As a structural material, it may be used for rocket engine linings but it is an essential ingredient in vacuum tube cathodes. It is processed in the non-molten state through powder metallurgy. Selenium is also a required resource for its photosensitive character necessary in light sensors and arrays of our engineered lifeform. Se/Te weight ratio of ~20 is approximately constant in stony meteorites while the Se abundance averages ~25 ppm Si in both stony and carbonaceous meteorites (Akafa Reference Akafa1966; Schuindewolf Reference Schuindewolf1980). Meteoritic sources are thus favoured for both elements W and Se.
There are material commonalities to our engineered creature and biological organisms. Important elements for the LUCA organism may have been Fe, Ni, Co and W metals (amongst others such as Mn, Mo, Cu, Zn and V) at temperatures ~100°C under high pressure maintained by hydrothermal flow reactors (Wachtershausen Reference Wachtershausen2006). These materials bear many similarities to our self-replicating machine's materials, though the biological temperature regimes are much more modest. Pioneer ligands included S and S2 (sulphides) and others such as SH, H2O, OH, CO and CN, many of which are available as volatiles in the lunar regolith. Metal sulphides such as troilite/pyrite are common in nickel-iron meteorite material. Iron has an important role in ancient biochemical systems prior to oxygenation of the Earth's atmosphere. Early ecosystems on Earth were driven by the anaerobic cycling of H2 and Fe2+ by phototrophs (Canfield et al. Reference Canfield, Rosing and Bjerrum2006). Banded iron formations from 3.8 By ago indicate Fe2+ was dissolved in ocean waters. Iron cycling occurred between Fe3O4 and Fe2+ in solution in a Fe2+/Fe3+ redox couple (Weber et al. Reference Weber, Achenbach and Coates2006). Insoluble Fe3O4 is the electron acceptor that is produced through anoxygenic photosynthesis with ferrous Fe2+ in solution as the electron donor (Bird et al. Reference Bird, Bonnefoy and Newman2011):

Fe2+ is then recycled by iron-reducing bacteria. Mars has large deposits of iron oxide for which the Tinto River Basin with its iron-based geomicrobiology is an analogue (Amils et al. Reference Amils, Fernandez-Remolar, Gomez, Aguilera, Gonzalez-Toril and Rodriguez2003). Its acidic waters are enriched in ferric iron and sulphates supporting iron oxidizers and iron reducers. Pyrite FeS2 is common under anaerobic conditions such as hydrothermal vents where it is a constituent of chimneys. It is formed from hydrothermal H2S and Fe2+ in solution: FeS + H2S → FeS2 + 2H+ + 2e−. Pyrite offers a powerful chemoautotrophic energy source through the oxidative formation of pyrite from troilite and hydrogen sulphide (Wachtershauser Reference Wachtershauser1990). Pyrite may also act as a reduction source for catalysing the formation of iron–sulphur enzymes NAD(P)H and FADH2 (Wachtershauser Reference Wachtershauser1992). Anaerobic oxidation of ferrous (FeII) to ferric (FeIII) iron by purple non-sulphur bacteria may have preceded oxygenic photosynthesis during the Archaean (Widdel et al. Reference Widdel, Schnell, Easing, Ehrenreich, Assmus and Schink1983). Pyrite minerals are commonly contaminated by FeS, CoS2 and NiS2 and have a typical Se content of 0.05% but may be enriched up to 0.9%. Finally, today, magnetotactic bacteria synthesize magnetite crystals inside magnetosomes (Rawlings et al. Reference Rawlings, Bramble and Staniland2012). Hence, iron has an important role to play in biology. Likewise, it has a central role in the metabolism and tissue of our engineered creature.
The natural lunar glass is ubiquitous on the Moon – typically 20–25% of lunar regolith – caused by impact heating followed by rapid quenching but it is not suitable for optical applications. Fused quartz/silica is the best option though it is produced by melting and shaping high-purity silica at 2000°C (compared with ~1000°C for soda lime glass). It has superior optical and thermal properties compared with most other types of glass and has a low thermal expansion coefficient (~5.5 × 10−7/°C). This makes fused silica suitable for lenses, polished mirrors, vacuum tubes, glassware and other transparent optical components across the IR–ultraviolet (UV) range. As well as glass, quartz is a piezoelectric material that may be deployed for displacement sensing and derivative measurements (Kolesar & Dyson Reference Kolesar and Dyson1995). Silica and its variant quartz are rare on the Moon due to the low water content of the Moon. It can be manufactured by deposition from silicates with the action of strong acids similar to natural weathering. For example, acid leaching by hot hydrochloric acid is a viable approach to reducing anorthite while avoiding high temperatures:

There are plastic pre-ceramic resins that can be 3D printed as a plastic gel into structures which are then fired into a high-temperature ceramic releasing much of its organic component (Wogan Reference Wogan2016). These pre-ceramic polymers are composed of mixtures of siloxanes and silazanes which are extruded. When pyrolized in the presence of UV light, the two polymers cross-link and form silicon oxycarbide ceramic structures. A simpler approach is to employ silicone plastics as precursors to ceramics without the need for silazanes (Narisawa Reference Narisawa2010). There are two options: silicone may be oxidized into silica or reduced to silicon carbide. In all cases, it requires a post-processing thermal step following 3D printing. Silicon carbide may be created by heating silica and carbon at 2400°C for an extended period – this far in excess of the temperatures required for metal extraction from minerals. However, pyrolysis of silicone at 1100°C yields SiOxCy which may be converted to silicon carbide by carbothermic reduction with excess C at 1800°C:

This imposes slightly more modest temperatures though the rarity of carbon sources suggests SiC should be reserved for specialized roles such as ball milling. Alternatively, silicone may be converted to silica by flame combustion in oxygen:

This would not have the hardiness of SiC but provides a mechanism for 3D printing ceramics without extreme temperatures. Silica is commonly used in biological systems –diatom shells, phytoliths in grasses, and spicules of sponges. Silicon has also been suggested as a replacement for carbon through its redox reactions between silica solid and silane (SiH4) gas. Such a silicon-based metabolism would require quite extreme conditions and the solidity of silica does present certain difficulties for its excretion.
Biomining is simpler and less energy-intensive than pyrometallurgy, offering a biological component to engineered self-replication. Of particular relevance is the biooxidation of sulphide deposits such as iron pyrite by Thiobacillus ferrooxidans in a cultured medium with ferrous iron as the energy source (Acevedo Reference Acevedo2000; Cockell Reference Cockell2011). Synthetic biology may introduce additional extraterrestrial applications such as enhanced biomining and biocement production, biological control systems (Menezes et al. Reference Menezes, Montague, Cumbers, Hogan and Arkin2015) and photosynthetic bacteria for capturing solar energy as a biofuel (Way et al. Reference Way, Silver and Howard2011). However, biomining using microorganisms as catalysts are unlikely to be feasible for extraterrestrial processing in the near term, especially on the Moon.
Biological Principle 2: solar energy for metabolism
Energy is essential for living entities to maintain their metabolic activity and growth. Whereas photosynthesis in chloroplasts is driven by pigment molecules which operate at moderate temperatures, the self-replicating machine autotroph uses thermal energy. Thermosynthesis has been suggested as a plausible energy source for extraterrestrial biota, though it was envisaged to apply to low-temperature gradients within ices (Muller Reference Muller2003). Such thermosynthesis would offer very low-quality energy but it is plausible that much higher temperature gradients might occur at hydrothermal vents with temperatures of 400 + °C under high pressure. Industrial temperatures offer even higher energy sources clearly beyond the capacity for carbon-based biology.
To extract our feedstock for the 3D printers, an intense source of thermal energy is required. Our self-replicator uses hearth-based chemistry to extract its restricted set of feedstocks materials. The primary energy source is solar energy which is captured and concentrated using solar concentrators – parabolic mirrors or Fresnel lenses. Solar furnaces using Fresnel lenses can provide the required temperatures for thermochemical extraction from minerals. Fresnel lenses concentrate sunlight onto a single focus – they are flat with a sculpted contour to reduce weight. They have not been discovered by biological evolution almost certainly because the advantage of Fresnel lenses over biconics is only apparent for much larger geometries than biological eyes. The temperature regimes our creature requires for its metabolism far exceed that of even Pyrolobus fumarii which like all terrestrial organisms is restricted by the limits of carbon-based organics. A silicon-based lifeform may not be so limited in temperature tolerance. The Fresnel lens-based thermal source allows us to create a local controlled environment for the self-replication process to be undertaken in a structured encapsulated environment to minimize variability in metabolic processes. This locally controlled environment begins with using Fresnel lenses to thermally fuse local lunar regolith at 1200–1500°C, thereby paving it into the glass to control the incidence of dust. This is the first step towards encapsulation.
Thermal energy can be converted into electrical energy through thermionic emission (implemented in vacuum tubes) (Ellery Reference Ellery2016a,Reference Elleryb,Reference Elleryc). This focusses light energy onto a small volume as heat onto the cathode of a vacuum tube. The cathode emits electric current through thermionic emission with an efficiency of around 5–20%. This may be enhanced significantly through PETE (photon-enhancement of thermionic emission) using photosensitive coatings (such as Se) (Schwede et al. Reference Schwede, Bargstin, Riley, Hardin, Rosenthal, Sun, Schmitt, Pianetta, Howe, Shen and Melosh2010). Thus, electric power is generated to enable the growth of the self-replicating machine. Both forms of energy – thermal and electrical – are required for our artificial creature's metabolism. Energy may be stored mechanically in motorized flywheels. According to Boden (Reference Boden2003), the first fundamental law of bioenergetics requires that a living organism must convert environmental energy into smaller energy currency units that can be tapped incrementally to power metabolic activity. In biological systems, it is ATP that provides this currency in discrete packages. In our self-replicating machine, flywheel energy storage provides a continuously tappable energy mechanism. It is not inconceivable that the bacterial flagellar motor could have evolved and been co-opted into a nanoscale-flywheel-type of organelle for storing biological energy (without the flagellum itself). Multiple flywheels in random orientations within the cell would sum to zero total angular momentum. Furthermore, as conceived in spacecraft (Varatharajoo Reference Varatharajoo2004), flywheels could provide combined energy storage and angular momentum control of cellular tumbling. The same two components discussed later for actuation and information processing – motors and vacuum tubes respectively – provide the basis for energy generation and storage respectively for our self-replicator. This multifunctional aspect of component use is common in biology where a component evolved for one task (such as feathers for thermal insulation) is co-opted for another task (control surfaces for bird flight). Motors are the central component in flywheels (electromechanical batteries) which offer high-energy densities. They provide the basis for all motive machines including 3D printers, other manufacturing processes and for energy storage (as flywheels); vacuum tubes provide the basis for all active electronics and energy generation (through thermionic conversion) (Ellery Reference Ellery2016d).
Biological Principle 3: manipulation of the environment
The feedstock to which our creature has access is fed to our ribosome which comprises a set of 3D printers for different material types. In biological 3D protein construction, 1D polypeptide strings are constructed from amino acid modules in a ribosomal assembly line using tRNA coding keys. The origin of tRNA–amino acid encoding is unknown, whether it is an arbitrary frozen accident or due to a specific physicochemical interaction (Edwards Reference Edwards1998). Coevolution theory suggests that the coding scheme evolved from relative positioning of precursor amino acids and tRNA on pyrite mineral surfaces. Polypeptide strings automatically configure themselves into their appropriate 3D shape. In our artificial creature, the construction process is not an assembly line, rather a suite of 3D printers. The artificial 3D structure is constructed from a series of modular 2D layers that are built into their appropriate 3D shape layer-by-layer. Furthermore, this 3D printed structure is employed in its entire volume rather than localized to active regions of the surface of a biological protein – this, of course, is a result of the difference of scale between the macroscopic engineered structure and the molecular biological structure. Our self-replicating machine is based on a 3D printing concept inspired by the RepRap 3D printer (Jones et al. Reference Jones, Haufe, Sells, Iravani, Olliver, Palmer and Bowyer2011). RepRap is a partially self-replicating 3D printer that can print its own plastic parts (Fig. 1). It cannot, however, replicate: (i) its steel bars; (ii) its connecting metal nuts and bolts; (iii) its glass work platform; (iv) its electric motors; (v) its electronics boards; (vi) nor can it self-assemble. Such additive manufacturing is well established for constructing structures of different materials such as plastics (fused deposition modelling), metals (electron beam freeform fabrication) and ceramics (selective laser sintering). All 3D printing involves relative motion between a printing head (xy-direction) and a work platform (z-direction). They are in effect Cartesian robots – all robots from rovers to drills to manipulators are kinematic configurations of electric motors. Hence, electric motors are central to our self-replicating machine.

Fig. 1. Assembled RepRap 3D printer.
The key components of the self-replicating machine are electric motors and electronics which can both be constructed from the available material set we considered earlier. These components are the fundamental components of any kinematic machine – 3D printers, manipulators, roving vehicles, drilling/milling machines, lathes, etc. The core of any robot is its actuators, sensors and controllers. Actuation involves manipulating the local environment – an essential pre-requisite of life. Our own experiments using shape memory alloys as artificial muscles driving a rotary shaft using cams illustrated the concept of an artificial linear bio-inspired motor but it was inferior in performance to the electromagnetic motor (Ellery Reference Ellery2015). We have been applying 3D printing methods to the construction of DC electric motors of different types. Although we have yet to 3D print an entire electric motor, we have 3D printed motor cores and are working in printing coils and electromagnets to complete the assembly (Fig. 2). In our self-replicating machine, the nature of the self/non-self-boundary is more fluid than in biological systems – its internal space is defined by its internal thermochemical processing, 3D printing, etc. Its external space is less clear as it must forage to acquire its nutrients for which it deploys rovers to perform extractive processes – they effectively extend ‘pseudopodia’ into the environment. The goal is to demonstrate 3D printing of electric motors and electronic control systems as the basic components of any kinematic machine. We thus next consider information-processing control systems.

Fig. 2. Polylactic acid (PLA)-based 3D printed motor core with 45% iron powder loading.
Biological Principle 4: information processing
It has been proposed that the Turing machine does not constitute a general model of computing (Goldin & Wegner Reference Goldin, Wegner, Cooper, Lowe and Torenvliet2005). Natural computation is a physical process that cannot be fully described by a Turing machine (MacLennan Reference MacLennan2004). Hence, there are physical machines that cannot be simulated by any Turing machine. The Turing machine model cannot account for interactivity with the environment of the real world because it is abstract rather than physical. Interacting computational units have emergent properties that are not algorithmic. A special Turing machine concept relevant to biological information processing is the interactive Turing machine. Biological organisms interact with their environment allowing for perpetual learning and so possess super-Turing capabilities (Wierdermann & van Leeuwen Reference Wierdermann and van Leeuwen2002). This concept may be abstracted as the interactive Turing machine with advice. Interactive Turing machines have perpetual exchange of information with their environment – essentially infinite computations. This models any cognitive system equipped with sensors to acquire information from the environment and effectors with which to modify that environment. This process is ongoing and continuous. This capacity by itself however, lies within the power of the universal Turing machine through its input/output tapes. The key to introducing super-Turing capabilities lies with the admission of unpredictability to its computations. This is introduced to the Turing machine as advice to implement non-computable external information. The advice takes the form of a separate advice tape that computes the advice value on its tape in a single step. The Turing machine with advice models computability with interaction with the external environment. The advice tape models inputs from a social community of interactive Turing machines – the advice is non-computable within the Turing machine itself. Hence, the interactive Turing machine with advice constitutes a super-Turing machine. A corollary of this suggests that the physical version of the Church-Turing thesis (every finite physical process subject to physical laws can be simulated by a universal Turing machine operating a finite program) (Deutsch Reference Deutsch1985) is incorrect and that biological neural function cannot be implemented by a universal Turing machine.
Our interest in feedback control systems is reminiscent of a cybernetic definition of life emphasizing multiple feedback control systems as a major characteristic (Korzeniewski Reference Korzeniewski2001). We need to 3D print computational control systems for those motors, i.e. electronics. The vacuum tube – constructed from our restricted suite of materials in a relatively simple geometric configuration – should lend itself to 3D printing (TBD). A typical triode has a simple construction comprising a heated tungsten cathode surrounded by a nickel control grid and a nickel anode enclosed in a glass tube. The control grid voltage controls the current between the cathode and anode. It provides the basis for electronic amplification and switching (Han & Meyyappan Reference Han and Meyyappan2014). Solid state electronics requires too many specialized reagents and conditions to be incorporated into a practical self-replication scheme. The vacuum tube may potentially supersede solid-state transistors for unconventional applications as they have a high tolerance to temperature and radiation (Teuscher Reference Teuscher2014). Self-replication technology is one such unconventional application. In fact, vacuum channel transistors are under development in which a thin layer of SiO2 insulates a gate electrode from the upper source and drain electrodes (Han & Meyyappan (Reference Han and Meyyappan2014)). The cathode and anode are sharpened into points to intensify the electric field at the tips emitted via field emission. The distance between the cathode and anode is less than the electron mean free path (200 nm in the air or 1 µm in He) – in a vacuum, this is not a constraint. However, the bulky nature of traditional vacuum tubes does not lend itself to traditional general purpose computational architectures.
Digital computations require many more devices than analogue circuits for the same function – the addition of two numbers requires hundreds of transistors. It requires Boolean circuits of size O(n 1+e) to compute multiplications with an error e > 0. For this reason, we adopt neural network architectures over traditional computer architectures to compensate for the larger size of vacuum tubes over solid-state transistors. The complexity of a neural network increases only as the logarithm of the task complexity unlike the exponential increase in circuit complexity of digital architectures. Similarly, to train a neural network of N nodes and W weights, m random training samples are required such that $m \ge O\left( {{\textstyle{W \over e}}\log {\textstyle{N \over e}}} \right)$ where 0 < e ≤ 1, e = acceptable error fraction (Baum & Haussler Reference Baum and Haussler1989). Neural net circuits can incorporate vacuum tubes rather than difficult-to-manufacture solid state circuitry but without the scaling problem of digital circuitry. To that end, we have adopted a simple analogue neuron circuit as our computing element modified from the Yamashita–Nakaruma neuron (Yamashita &Nakamura Reference Yamashita and Nakamura2007; Larson & Ellery Reference Larson and Ellery2015) (Fig. 3).

Fig. 3. Analogue neuron circuit – part of a two-neuron circuit for rover obstacle avoidance behaviour.
The electronic neuron models the nonlinear input–output relation where the output is given by:

where x i = input, w ij = weighting factor, f(.) = non-linear squashing function. The weight matrix of the network of neurons determines the functionality of the network. Simple reactive behaviours have been implemented readily using these analogue neurons. Braitenburg vehicles comprise two sensors, left and right, and two motors to drive their respective wheels, left and right. The connections between the sensors and the motors determine the vehicle's behaviour. We have demonstrated a two-neuron circuit implementing a Braitenburg control architecture of BV2/BV3 class (Braitenburg Reference Braitenburg1984) performing automatic obstacle avoidance on a simple desktop mobile robot.
Artificial neural networks can implement any nonlinear mapping function and are Turing-complete (Siegelmann & Sontag Reference Siegelmann and Sontag1995) perhaps even offering super-Turing capabilities (Siegelmann Reference Siegelmann1995). Neural nets are efficient as universal Turing machines. Recurrent neural nets with feedback are more powerful than standard feedforward neural nets (Goudreau et al. Reference Goudreau, Giles, Chakradhar and Chen1994). A neural network simulator with r = m + n + 2 layers of neurons and two sets of recurrent connection weights with feedback can simulate in real time a Turing machine with m-symbols and n-states (Sun et al. Reference Sun, Chen, Lee and Giles1991). The Siegelmann–Sontag universal neural network-based Turing machine comprised 886 neurons, though this has been reduced in more recent work to 96 then 25 and finally to just 9 switched affine neurons that implement Boolean functions (Siegelmann & Margenstern Reference Siegelmann and Margenstern1999). A recurrent neural network with rational weights is computationally equivalent to a Turing machine model of digital computing machines. The computational capacity of recurrent neural networks may be enhanced beyond that of the Turing machine using real-valued weights which offer the infinite precision of analogue information (Balcazar et al. Reference Balcazar, Gavalda and Siegelmann1997). This allows them to compute non-recursive functions. The recurrent neural network with real weights offers super-Turing capabilities as an interactive Turing machine with advice (Cabessa & Siegelmann Reference Cabessa and Siegelmann2012). Hence, neurons with rational weights yield Turing machine capability but neurons with real weights are more powerful than Turing machines (Margenstein Reference Margenstein2000). Analogue recurrent neural networks are equivalent to oracle Turing machines. An oracle machine is a black box augmenting a Turing machine that makes a decision in a single step even if that decision is complex. The Turing machine can copy symbols onto the oracle tape. The oracle is not a Turing machine itself. The oracle itself has its own tape and read/write head but has special states – Query and Response. When the machine reads the Query state, the read/write head subsequently moves to the next square and writes the Response (yes/no) as a solution in a single step. State Yes or No depends on whether the current string is on the oracle tape. The oracle introduces the possibility of inputting new non-deterministic or non-computable data to the machine. An example of a decision is the halting problem where the oracle determines whether the Turing machine halts given its inputs, i.e. the oracle augments the Turing machine with non-computable capabilities. As a physical adjunct circuit, the Wheatstone bridge can act as an oracle to a Turing machine extending the computational powers of such a system beyond the Church-Turing limit (Beggs et al. Reference Beggs, Costa and Tucker2010). It is the measurement of a physical process – in this case, measurement of analogue electrical resistance by the Wheatstone bridge – that extends the computational power of the abstract Turing machine. We select the analogue neural network (to which feedback connections can be added) as the computational architecture of choice yielding greater computational capabilities over traditional computational architectures. Evolving recurrent neural networks where synaptic weights change over time (i.e. learning) are equivalent to interactive Turing machines with advice, i.e. they are capable of super-Turing computations – this is the case for both rational and real weights (Cabessa Reference Cabessa, Villa, Wermter, Weber, Duch, Honkela, Koprinkova-Hristova, Magg, Palm and Villa2014). In rational-weighted recurrent neural networks, these super-Turing capabilities can only be realized if the synaptic weights evolve in a non-recursive manner – recursive learning limits such networks to Turing-computable functions. However, in real-weighted recurrent neural networks, there are no such requirements for the learning to achieve super-Turing capacities. Super-Turing machines include analogue recurrent neural networks with real-valued nonlinear squashing functions (Teuscher & Sipper Reference Teuscher and Sipper2002). Hence, our analogue neuron may offer super-Turing capabilities when in recurrent configuration. These circuits – based on vacuum tube active components – are to be 3D printed. The core of our universal Turing machine segment is a 3D printer which takes data stored in magnetic core memory (based on the same technology as motors) and prints out analogue neural net circuits that physically encapsulate programs. It is envisaged that such neural network circuits implement specific programs printed by a 3D printer (representing the read–write head of a universal Turing machine) according to information held in magnetic core memory (based on the same principles and materials as an electric motor). Embedded software interacts with its underlying hardware and is subject to temporal constraints on execution time, this constraint being undecidable for Turing machines (Lee Reference Lee2005). The analogue neural net is an extreme version of the embedded software in that the software is embedded in the connection weights without the temporal undecidability problem. Although the analogue neuron is far simpler than the biological neuron, it nevertheless implements basic functions of information processing and transmission.
Noise can be exploited to advantage – dithering is the process of imposing random mechanical vibrations onto motors to compensate for sticking behaviour. This is an example of stochastic resonance (Wiesenfeld & Moss Reference Wiesenfeld and Moss1995). Similarly, biological neurons, by virtue of their nonlinearity, can exploit stochastic resonance to enhance the detectability of small signals by amplifying the combined signal and noise to overcome the threshold for detection. The noise signal from broadband background entrains and resonates with the low signal to amplify it. Stochastic resonance requires low levels of noise otherwise excessive noise will swamp it – the signal-to-noise ratio rises with noise to an optimal value and then decreases. Analogue neurons are characterized by constant low levels of intrinsic noise but above a threshold they fire digitally, thereby amplifying a low signal. Networks of neurons provide the ability to handle signals with a range of amplitudes and noise levels. Hence, analogue neural circuits offer the prospect of exploiting stochastic resonance for enhanced signal processing.
Biological and analogue neural systems go beyond Turing machines because they are self-modifying and computationally open so cannot be captured in an algorithm. This is precisely the realm of autonomic computing which emphasizes self-configuration, self-optimization, self-repair and self-protection (Kephart & Chess Reference Kephart and Chess2003). Hence, our adoption of analogue recurrent neural networks offers similar computational capabilities as biological systems.
Discussion & conclusions
Our self-replicating machine concept possesses all the primary elements of life. We have outlined how the elements of a self-replicating machine might be constructed physically and how some fundamental components might be 3D printed. We are using robotics as existence proofs for physical mechanisms of self-replication – a similar approach of using robotics in the service of biological sciences has been cognitive robotics and robotic zoology. The steps towards realization of a self-replicating machine are thus:
(i) 3D printing basic control system elements – actuators and electronic circuits – from raw material – this is the most critical step which is underway;
(ii) 3D printing general robotic systems (essentially protein analogues) – mining vehicles, drills, crusher jaws, etc. –as different configurations of motors and their control systems;
(iii) 3D printing 3D printers as Cartesian robot configurations of motors and their control systems (essentially ribosome analogues);
(iv) There have been several attempts to build embryonic but simple self-replication architectures from Lego to exploit its standard blocks and mechanical interfacing (Chirikjian et al. Reference Chirikjian, Zhou and Suthakorn2002; Moses et al. Reference Moses, Ma, Wolfe and Chirikjian2014) but none consider the complexities of raw material acquisition and processing (essentially cellular networks);
(v) Encoding a self-replicating machine into a heritable program of instructions (essentially genes) to be stored in magnetic core memory (including error detection and correction codes);
(vi) Demonstrate magnetic memory-to-memory program copying through magnetic induction (similar to the two sides of a transformer).
So, can building an artificial robotic lifeform using engineering materials provide any insight into the astrobiology quest – to understand the limits and scope of life beyond the Earth? It is worth noting that our artificial creature possesses most of the properties of life. There are open questions however, whether this self-replicating machine could be alive. In the digital ecosystems Tierra and Avida, populations of self-replicating programs compete for computer memory space and CPU time forming their computational ecosystems (Adami Reference Adami2006). The code itself corresponds to genetic information while their execution implements the phenotypic function. Instruction processing correlates to energy consumption and varies in proportion to code length. Replication is imperfect while population pressure imposes environmental constraints. Fitness is determined by the copying efficiency and replication rate. Replication is subject to mutation and faster replicators tend to have more offspring (r strategy) but new phenotypic traits increase fitness (K-strategy). Limited environments cause evolutionary regression but co-evolutionary arms races imposed by continually changing environments overcome this (Red Queen effect). Evolvability however, requires a balance between exploration (plasticity) and exploitation (stability). Experiments in Avida indicate that self-replication does not guarantee evolvability (LaBar et al. Reference LaBar, Adami and Hintze2015). Within Avida, a lack of evolvability can occur if all possible mutations to a specific genetic sequence prevent further self-replication, but this would be rare. Autopoietic definitions of life appear to deny the possibility of engineered life as they emphasize process rather than components and, in doing so, they impose evolutionary history as a requirement for life. A minimal Mycoplasma genitalium cell has been engineered to survive with only 265 genes without loss of biological function from its natural 475 genes (it is the smallest natural genome because it lacks genes for cytochrome c and the Krebs cycle) – it did not evolve this state, so by this definition, it is not alive, yet this is clearly not the case. The original precursor organism with its evolved cellular components – many of which were retained in the minimal organism – was alive. Hence, we submit that evolutionary capacity rather than evolutionary history is a property of life. This implies that the self-replicating machine we have described would be alive in a potential sense. If permitted to evolve, it would constitute life; if prevented from evolving, it would not. An important question then becomes whether the machine is too brittle for evolutionary change – this will be dependent on the architecture of its genetic program of instructions. We envisage that the engineered system will not use phylogenetic, ontogenetic and epigenetic representation schemes though these have been explored in engineered systems (Sipper et al. Reference Sipper, Sanchez, Mange, Tomassini, Perez-Uribe and Stauffer1997) and indeed, 3D printing provides a mechanism to implement ontogenesis in self-replicating structures. This is an issue for the future. The question to the astrobiology community is whether this quest to create a self-replicating machine yields any insights into astrobiology questions.
Appendix: Biological motors
We briefly review biological molecular motors because motors figure centrally in our engineered life approach. They are commonly implicit in definitions of life through the property of motion. Biological motive capability may be very ancient – it has been suggested that internal chemistry (such as HCN hydrolysis) in proto-cells might have induced internal stresses thereby generating physical movement, i.e. motility (Hanczyc Reference Hanczyc2011). All molecular motors generate mechanical forces using intermolecular chemical binding energy either through a power stroke or a Brownian ratchet to exploit random thermal fluctuations. All biological linear motors operate through sliding along filaments. There are three protein motors that act as rotary engines – the bacterial flagellar motor and F0 and F1 motors of ATP synthase (Oster & Wang Reference Oster and Wang2003). All these biological rotary motors are based on torqueing a load shaft. We consider only the main biological molecular motors but others exist. For example, gene expression begins with RNA polymerase (RNAP) sliding along DNA in search of the promoter. RNAP then binds to the promoter to initiate DNA transcription.
ATP powered molecular motors include kinesin and dynein which actuate cytoskeletal fibres of tubulin (microtubules), and myosin which actuates cytoskeletal fibres of actin (microfilaments) (Tyreman & Molloy Reference Tyreman and Molloy2003). All these motors comprise of a force-generating head (N-terminal) connected by a neck to a cargo-carrying tail (C-terminal). Both myosin and kinesin operate through the Brownian ratchet. ATP synthase (ATPase) catalyses the reversible formation of ATP from the nucleotide ADP and a P group by releasing energy. ATPase is ubiquitous in the biological world as the universal form of energy currency. It occurs in mitochondria, chloroplasts and bacteria (Senior & Wise Reference Senior and Wise1983). ATPase is the smallest biomolecular motor at ~10 nm in size and comprises two major parts organized as a rotor and stator assembly (Karplus & Gao Reference Karplus and Gao2004; Wang & Oster Reference Wang and Oster1998): (i) a hydrophobic (insoluble) membrane segment F0 for proton movement, and (ii) a hydrophilic (soluble) F1 segment for ATP hydrolysis and synthesis. ATPase's two opposing rotary units are connected in series on a common shaft. The shaft comprises a coiled coil of γ subunits. The F1 motor drives the shaft clockwise (powered by ATP) while the F0 motor drives it anticlockwise (powered by proton gradients). F1-ATPase comprises three α-subunits and three β-subunits alternately forming a segmented ring (F1) surrounding an α-coiled γ-subunit forming the shaft (stator). The F0 motor comprises a cylinder of 10–14 subunits (rotor). It can act as a proton pump. The stator stalk links αβ and F0, though it varies structurally in its mitochondrial, chloroplast and bacterial forms (Weber Reference Weber2007). The foot of the γ-subunit connects to the ring of F0 unit. Rotary motion of the γ-subunit of the F1-ATPase motor is energized by the hydrolysis of ATP with water (Itoh et al. Reference Itoh, Takahashi, Adachi, Noji, Yasuda, Yoshida and Kinosita2004). Proton flow through F0 rotates the γ unit clockwise to synthesize ATP while hydrolysis of ATP rotates it anticlockwise and reverses the proton flow. β-subunits are fixed into a αβ-stator ring which pushes the γ-subunit to rotate. It rotates through 120o steps and generates a torque of 40–80 pN.nm with near 100% efficiency (almost fully reversible) because it converts chemical energy into elastic strain energy of the αβ stator rather than acting as a heat engine. F1 could be unrolled to form a linear motor so that the αβ-subunits crawl along the γ-subunits (Kinosita K et al. Reference Kinosita, Yasuda, Noji, Ishiwata and Yoshida1998). Artificial control of ATP concentration as the fuel supply effects control over the movement speed of ATPase molecular motors such as kinesin and myosin in synthetic devices (Hess & Vogel Reference Hess and Vogel2001). F1-ATPase has been integrated with an engineered nano-propeller to demonstrate the rotation of the mechanical nano-propeller energized by ATP (Soong et al. Reference Soong, Bachand, Neves, Olkhovets, Craighead and Montemagno2000).
Flagella are widespread in archaea and bacteria indicating its early evolution. The flagellum probably evolved from an ancestor core flagellum of around 20 proteins – motor, rod, hook, filament and protein-export machinery. All major archaea possess flagella – halophiles, methanogens, thermophiles and hyperthermophiles. Furthermore, the archaeal flagellum is very different to the bacterial flagellum such that there are no genetic homologues of bacterial flagellar genes in archaea (Thomas et al. (Reference Thomas, Bardy and Jarrell2001)). This suggests an independent evolution in the early prokaryotic kingdoms, though this picture is complicated by lateral gene transfer (Ochman et al. Reference Ochman, Lawrence and Groisman2000). Archaeal flagella are very thin ~10 nm in diameter but operate similarly to bacterial flagella with runs alternating with tumbles. Morphologically, archaeal flagella incorporate the hook feature but lack the rings of bacterial flagella being anchored into cell walls rather than cell membranes.
The bacterial flagellum is morphologically similar to engineered DC electric motors though they are electrostatic with their torque output balanced by viscous drag, both properties resulting from its small size (Berg Reference Berg1974). Without torque, the flagellum would halt within under 10−6 revolutions due to this viscosity. The flagellum control system including chemoreceptors is specified by around 50 genes while the bacterial flagellum itself is constructed from 20 proteins and synthesized and controlled by 40 of those genes. The bacterial flagellar motor, powered by proton gradients, includes 8 stator segments, each consisting of 4 MotA and two MotB subunits – MotA conducts protons through the C-ring of the rotor while MotB anchors MotA to the cell wall. The bacterial flagellum is driven by a rotary motor at the base of the flagellum powered by a flow of protons across the bacterial membrane – it is an electrostatic motor. It rotates rather than flexes like eukaryotic flagella – anticlockwise rotation generates thrust while clockwise motion causes tumbling in response to attractor gradients. Escherichia coli have a set of four flagellar clusters around their cell bodies. The chemoreceptors are clustered into a ‘nose’ at the poles of the cell. When activated, a conformation change is transmitted through the membrane to the flagellar motors. The bacterial flagellum is composed of a basal motor embedded in the cell wall, the hook and the filament. The bacterial flagellum comprises a 45 nm diameter rotary motor that drives the base of a 10 µm long, rigid helical propeller of ~20 nm diameter with a 2 µm pitch. The propeller is connected to a flexible hook by two junction proteins. The hook is a universal joint so the propeller can rotate around multiple axes. The outer L (lipopolysaccharide) and P (peptidoglycan) rings act as bushings for the propeller shaft through the outer cell membrane but neither contribute to torque generation. The 25 µm thick inner MS (membranous/supermembranous) ring is the bushing attached to the inner cell membrane. The MS ring is central to the flagellar structure, assembly and operation and is one of several rings around 20 nm in diameter with a rotating rod inside. Attached to the rod is the hook linked to the 5–15 µ long filament – it is related to the type III secretion system (T3SSs) complex embedded in Salmonella that transports toxins from inside the cell into needle-like structures on the outside to inject poisons into its victim. Within the cell cytoplasm is the 45 µm thick C ring. Around the flagellar motor between the MS and C rings is a ring of ten studs. Flagellar proteins are hydrophobic (Blair Reference Blair1991; Berg Reference Berg2003) comprising the flagellum assembly – FlgE (hook), FlgB, FlgC, FlgF and FlgG (shaft) and FliC proteins (flagellum). FlgH and FlgI proteins form the L and P rings respectively. FliG, FliM and FliN are cytoplasmic proteins that form the bistable switch complex for torque generation and switching between clockwise and anticlockwise directions. FliN is part of the C (cytoplasmic) ring and stabilizes the switch structure; FliM is part of the C ring and involved in switching control of the rotation direction of the flagellum (protein CheY-P switches clockwise rotation otherwise rotation is anticlockwise); FliF is the structural core of the MS ring (stator); FliG is part of the MS ring and is involved in torque generation and switching; the C and MS rings form part of the rotor. The two Mot proteins (MotA and MotB) are part of the ten studs (stator) and together anchor and drive the motor, transport protons through the membrane and position the torque generators (Stahlberg et al. Reference Stahlberg, Schuster, Bauer, Baueurlein, Zhao, Reese and Khan1995). Phosphorylation of the CheY protein (supplemented by other Che proteins) induced by chemoreceptors changes the switching probability through bonding to FliM. The flagellar motor spins at rates that depend on the proton flux ~10–100 Hz with a torque of ~10−18 Nm. Assuming a rotor radius of 20 nm, the flagellum generates a force of ~20 pN at speeds of ~1 µm s−1 (Berg Reference Berg1995). During self-assembly, the flagellum is constructed from the inside out beginning with the shaft, followed by the hook then the flagellar filament (Ferris & Minamino Reference Ferris and Minamino2006). It is reckoned that flagella, like myosin, dynein and kinesin operate as thermal ratchets driven by thermal fluctuations (Cordova et al. Reference Cordova, Ermentrout and Oster1992). In the latter linear molecular motors, cross-bridges fluctuate about equilibrium positions against an elastic restoring force with ATP hydrolysis (Maxwell's Demon) imposing unidirectionality to the random movements. This means that molecular conformation switching is absent in biological molecular motors as chemical conformation switching in developing molecular electronic devices has been considered attractive (Carter Reference Carter1984). A hierarchical regulation process is based on two assemblies – shaft-hook and flagellar filament. The sub-assemblies are: the basal body that transmits torque from the motor to the hook; the flagellar motor comprises a stator and rotor; the switch determines the rotary direction; the hook functions as a universal joint; the flagellar filament acts as a propeller; finally, a series of assembly proteins (MacNab, (Reference MacNab2003). A molecular automata model of bacterial flagellar self-assembly was based on the affinity between binding sites as a similarity metric quantified by the Hamming distance (Lahoz-Beltra Reference Lahoz-Beltra1997).
The bacterial flagellum differs fundamentally from the eukaryotic flagellum (DeRosier, (Reference DeRosier1998). The eukaryotic flagellum is ~20–100 µm long and is based on dynein motors which bend and step along microtubular tracks as sliding filaments (Dutcher Reference Dutcher1995). The nine parallel microtubule fibres forming the sheath of the flagellum is hollow occupied by two central microtubules. Between the core and sheath microtubules are many inner and outer dynein arms which link the microtubules together and ratchet up and down. The dynein motors are synchronized through entrainment of coupled oscillators (Wemmer & Marshall Reference Wemmer and Marshall2004). The compliant tail undulates like a snake to generate movement powered by ATP. Eukaryotic cilia ~2–15 µm long are also constructed from dynein motors along microtubules powered by ATP but beat in oar-like movements. Cilia function by relative sliding of outer pairs of microtubules linked at their proximal end to a basal structure by connecting proteins (Huitorel Reference Huitorel1988). By contrast, the bacterial flagellum is powered by an electromotive gradient of protons through the cell membrane. Torque is generated through electrostatic interaction between a ring of proton-binding sites on the rotor and transmembrane protons (Schuster & Khan Reference Schuster and Khan1994). A fixed number of protons correlate to a single revolution so proton flow generates stepper motor behaviour. It has been postulated however that cyclic conformation changes generate travelling waves through elastic behaviour of the C ring (Atsum Reference Atsum2001). Unlike eukaryotic flagella, bacterial flagella can rotate in either direction. They can reach rotation rates up to 100–200 Hz at a constant torque of ~4500 pN/nm generating speeds of ~50 µm s−1 much faster than myosin or dynein biological motors.
In eukaryotic cells, molecular motors transport molecules and organelles along cytoskeletal tracks. The eukaryotic cytoskeleton is a dynamic system that provides structural integrity to the cell and rapidly re-organizes itself. Microtubules are the primary cytoskeletal systems in the eukaryotic cell and provide the basis for cellular events like mitosis through the mitotic spindle assembly. Microtubules move cargo along long distances while actin is used for local transport of cargo. Microtubules also form the basis of centrioles, cilia, flagella, pseudopodia, phagocytosis, growth and morphogenetic development. Microtubules form through dynamic self-assembly of tubulin proteins powered by the hydrolysis of GTP (Engelborghs Reference Engelborghs1994; Tuszynski et al. Reference Tuszynski, Trpisova, Sept and Sataric1997). Microtubules spontaneously self-organize based on reaction–diffusion process of dissipative structures at far from equilibrium conditions. This self-organization process appears to require gravity (Tabony et al. Reference Tabony, Pochon and Papaseit2001). Tubulin proteins – α- and β-tubulins (sharing 40% amino acid identity) – provides the basis of microtubules while γ-tubulin (sharing 30% amino acid identity with α- and β-tubulins) is associated with anchorage at the centrosome and mitotic spindle formation in eukaryotes (Oakley Reference Oakley2000). Microtubules are hollow tubes comprised 9–18 protofilaments of similar but non-identical α- and β-tubulin dimers (8 nm long by 25 nm diameter) forming a helical lattice: for 13 protofilaments, they run parallel to the long axis of the microtubule; for less than or greater than 13 protofilaments, they are twisted around each other. Microtubules reconfigure themselves through cutting of long immobile microtubules by enzymes katanin and spastin to convert them into short mobile microtubules which move into new configurations (Baas et al. Reference Baas, Karabay and Qiang2005). Microtubules function as linear tracks along which motor proteins (kinesin and dynein) travel for long-range cargo transport. Kinesins move towards the fast-growing (plus) end of the microtubule while dyneins move towards the slow-growing (minus) end. Microtubules also form the mitotic spindle that separates chromosomes. Viruses as obligate parasites appropriate host kinesin and dynein motors to propel themselves along microtubules (Dohner et al. Reference Dohner, Nagel and Sodeik2005).
Most molecular motors have one or more heads-on-a-stalk configuration with the head binding to a track and the stalk or tail binding to cargo either directly or indirectly (Cross & Carter Reference Cross and Carter2000). They operate by cyclically stepping along the track be it circular or linear through conformation changes driven by ATP metabolism – myosins along actin and kinesins and dyneins along microtubules (Karcher et al. Reference Karcher, Deacon and Gelfand2002). Both kinesins and myosins have a head (attaching to the actin or microtubule filament) that undergoes a conformation change on hydrolysis. This is amplified by the lever arm connecting the head to the tail. The affinity of the head to the filament determines attachment or detachment. Kinesin and myosin V are processive and remain attached with at least one head while myosin II is non-processive and detaches after the power stroke. Processivity permits continuous movement. Filaments of actin form tracks for myosin motors for local transport of cargo molecules. Actin filaments comprise two thin strands of polymer that are twisted around each other which in turn, are crosslinked to rigidize the structure. Myosins are actin-based molecular motors comprising one or two heavy chains, each connected to between one and six light chains. The motor head binds to actin powered by ATP hydrolysis which causes a myosin conformation change (power stroke). The motor head on the myosin thick filament forms elastic cross-bridges with the actin thin filaments. Myosins move along these actin filaments – myosin II and myosin V move in the direction of the barbed end. Myosin is 160 nm in length incorporating a 140 nm α-coiled tail and two 20 nm long heads which catalyse ATP hydrolysis and bind with actin. Thick filament myosin is energized by ATP hydrolysis and the release of phosphate to move cargo along tracks of thin filament actin which forms a local network. The myosin motor has multiple functions – muscle contraction, cytokinesis, endocytosis, cell shape, vesicle transport and signal pathways. Myosin comprises a catalytic core the binds nucleotide to actin linked to an 80 Å lever arm which rotates to move along the actin filament (power stroke). Each working stroke is powered by a single ATPase cycle. Myosin moves 100 Å displacement per ATP hydrolysed. ATP binding initiates the recovery stroke of recocking the lever arm. A new ATP molecule binds to myosin to repeat the cycle for further steps along the actin filament. The sliding filament model of myosin–actin action underlies muscle contraction driven by cross-bridges (Huxley Reference Huxley1996; Huxley Reference Huxley2000). The average rate constant for cross-bridge sliding is given by k = k 0e −Q/RT where $Q = {\textstyle{1 \over 2}}\kappa h^2$ = work done, h is the work stroke between 45° and 90° states and κ, the cross-bridge stiffness. A sliding force of ~3–5 pN between the myosin tail and the actin filament is generated yielding a step displacement of ~5 nm (sliding filament hypothesis). The force generated is linear with stroke with the gradient (dF/dh) determined by myosin–actin overlap (number of cross-bridges). This is followed by a highly non-linear hysteretic force recovery. In muscle, myosin and actin filaments overlap with the degree of overlap determining force production. Shortening of muscle occurs through sliding of thick filaments (myosin) along thin filaments (actin). Heads of myosin project from the thick filament forming cross brides with the thin filament – they exert a force and then detach repeatedly along the filament with a sliding step distance of 60 nm.
Kinesin is a motor protein that is powered by ATP hydrolysis to move cargo by walking along the surface of microtubules to specific destinations. Kinesin walks on tubulin with its two heads alternating hand-over-hand generating 8 nm steps. The kinesin motor is involved in mitosis and meiosis, mRNA and protein transport, organelle and vesicle transport and signal pathways. Kinesin comprises two identical globular heads at one end of a long coiled-coil stalk and a pair of light chains bound at the tail end of the stalk (Cross Reference Cross2004; Miki et al. Reference Miki, Okada and Hirokawa2005). The head includes a catalytic pocket for ATP hydrolysis and binding sites for microtubules and is the active mover. Kinesin moves through a conformation change in its 15-amino acid long neck linker triggered by binding to ATP (power stroke). The neck linker swings one of the two heads along the microtubule by 80 Å, in turn, generating hand-over-hand movement of 16.6 nm (Yildiz & Selvin Reference Yildiz and Selvin2005). Force-velocity curves of single kinesin molecules suggest that kinesin is a loosely coupled motor with a maximum force of 5–6 pN (Svoboda & Block Reference Svoboda and Block1994). The stalk and tail bind with cargo molecules while the neck between the stalk and head determines the directionality of walking. Kinesin is associated with microtubules and takes 50–100 steps at a time (processive) before dissociating from a microtubule equating to several microns (Howard et al. Reference Howard, Hudspeth and Vale1989; Mandelkow & Mandelkow Reference Mandelkow and Mandelkow2002) while myosin associated with actin takes a single step at a time. ATP hydrolysis and releasing phosphate induces the recovery stroke. While kinesins are directed to the plus end of microtubules, dynein is directed to the minus end. Movement is undertaken by dynein which interacts with the microtubules by generating a force towards the minus-end of microtubules (Vallee & Stehman Reference Vallee and Stehman2005). Dynein and kinesin are the two microtubule-binding proteins associated with the motor protein ATP synthase at the origin of the movement. Dyneins are the largest and most complex of the three linear motors of eukaryotes (dynein, kinesin and myosin), being some ten times larger than kinesin, the other microtubule motor. The dynein motor has a ring-shaped core of seven proteins enclosing a cavity with protruding two levers – a 20–30 nm tail for binding to cargo and a 10–12 nm stalk (B-link) for binding to the microtubule track (Koonce & Samso Reference Koonce and Samso2004; Asai & Koonce Reference Asai and Koonce2001). It is thus quite distinct from kinesin and myosin but shares features with them. The dynein head rotates through one-quarter turn against the stem and pulls on the α-coiled stalk powered by ATP hydrolysis. The base of the stalk translates 708 nm and the tip of the stalk by 16 nm. Dynein undertakes 8 nm steps similar to that of kinesin (8 nm) and some myosins (5 nm). The stalk acts as a tension cable; thus, dynein appears to act as a winch (Burgess & Knight Reference Burgess and Knight2004).