The National Healthcare Safety Network (NHSN) and Centers for Medicare and Medicaid Services (CMS) track rates of central-line–associated bloodstream infections (CLABSIs) and catheter-associated urinary tract infections (CAUTIs) for quality improvement and comparisons between hospitals. To maintain a high degree of reliability for the data, the NHSN provides definitions in the Patient Safety Component (PSC) Manual.1 Because CLABSI and CAUTI rates are reported as standardized infection ratios, the PSC Manual defines both events and how to determine the size of the at-risk population (the denominator).
In the current PSC Manual, device-associated data for denominators can be collected from electronic sources if the values obtained from the electronic health record (EHR) are within 5% of the values from manual counts over a 3-month validation period.2 Our institution recently changed its EHR system, which mandated validation of the new electronic data source. This validation requirement has been a challenge for several institutions and has been cited as a reason for needing to use supplemental infection control systems beyond the EHR.Reference Masnick, Morgan, Wright, Lin, Pineles and Harris3
Our infection control team devised a strategy of daily validation for a 3-month period that was intended to catch and correct errors nearly immediately. Discrepancies were resolved and attributed to manual errors in counting or to electronic errors in charting and reporting logic. These data were used in a quality project to determine when to use educational interventions or to implement changes in the new EHR to ensure valid denominator data.
Manual data collection is notoriously unreliable, and using it as a gold standard for validation is problematic.Reference McHugh4,Reference Gliklich, Khuri, Mantick, Mirrer, Sheth, Terdiman, Gliklich, Dreyer and Leavy5 Data entry and charting errors can occur with manual systems just as easily as electronic logic errors can occur with an EHR. The 3-month validation was ultimately performed, but we sought to use the data collected in this initiative to assess whether there was an earlier point in the validation period when the electronic system proved sufficiently accurate that continuing manual validation was not valuable.
Methods
This study was a secondary data analysis of aggregate deidentified data obtained in a quality improvement project. The Mayo Clinic Institutional Review Board deemed the study exempt from approval.
EHR transition
Mayo Clinic and the Mayo Clinic Health System converged their EHRs into 1 EHR (Epic Systems) in 2017 and 2018. The first hospitals to adopt the converged EHR were in the health system sites. In July 2017, 7 of the hospitals (with 15 inpatient units) began reporting into the device-associated module for the NHSN. We developed a validation strategy intended to collect manual and electronic data simultaneously for the first 3 months in the Mayo Clinic Health System in Wisconsin.
Validation
The NHSN device counts require units to do a cross-sectional survey of their patients at the same time each day to determine (1) how many patients are present, (2) how many patients have at least 1 central venous catheter, and (3) how many patients have at least 1 indwelling urinary catheter. Central venous catheters terminate in the central circulation, necessitating a differentiation between midline catheters and peripherally inserted central catheters, which are virtually indistinguishable by external exam. In hematology units, central catheters must also be divided into “temporary” (nontunneled) or “permanent” devices, and for port devices, determining whether and when a device was accessed first determined if it would be included in counts. In neonatal intensive care units (NICUs), all devices and data must be stratified by patient birth weight for reporting and validation purposes. The electronic system conducts these cross-sectional analyses at 00:01 a.m. each night. Aside from being an accepted time to do this, the timing has some advantages in accuracy because activity is often a nadir at midnight, so the chances of a miscount due to an influx of admissions or dismissals should be relatively small. However, this timing also presents a challenge for manual validation. Because all patient care units should be simultaneously checked at 00:01 a.m., the most accurate way to manually survey the number of patients present would be to have a surveyor on each unit at 00:01. Although an alternative time could have been selected, given the geographic separation of units and limited staff from infection control available as surveyors, we elected to pursue midnight validation as the benefits of lower transit outweighed the costs of other times.
We determined the most effective way for our institutions to validate would be to have the nurses working on the patient care units act as surveyors. Nurses performing patient care tally the devices on the patients for whom they were providing direct care; the charge nurse would then add the tallies and enter them into a report form. This process was consistent throughout; quick reference guides were created to ensure consistency in definitions. For 2 weeks before the transition, data were entered and compared to the data logged in our validated legacy system to ensure consistency and accuracy of manual counts.
We worked with EHR program colleagues to design a report that provided a daily census that identified which patients were being counted toward the following denominatiors: patient day, central venous catheter day, and urinary catheter day. These totals were then compared with manual totals provided by each unit, and discrepancies were investigated. Unit champions (ie, nursing leaders associated with each hospital unit) would then engage in a short root-cause analysis to examine the documentation, patient, and process by which counts were performed. Any errors in the manual counts were logged and corrected, and the persons responsible were educated. Any technical issues were referred to the EHR program support team with a ticket registered through our information technology help desk system. Our data collection tools for manual counts are reported in Appendix 1 (online).
Comparison of manual causes of error and electronic causes of error
We reconciled the manual and electronic counts daily. If a unit’s electronic total census, central venous catheter count, or urinary catheter count did not match the manual totals, we worked with unit champions to identify the source of the discrepancy and corrected the total count. Causes of discrepancies were coded as electronic if they related to the logic of reporting (the programming behind the electronic query), the documentation user interface, or patient mapping in the EHR. Manual errors were identified as such when the electronic count was correct and the reported manual counts were ultimately determined to be incorrect because of entry errors into the REDCap system or misunderstandings about which patients or devices qualified for the counts. The manual counts were corrected to provide accurate data for reporting. We instituted a policy of a data freeze on electronic counts; data from the midnight count would be exported to our total record on a daily basis and neither updated nor changed, thereby avoiding potential disruption due to patient transfers or altering data that would be finalized by the end of each morning shift.
Statistical analysis was performed using JMP software (SAS Institute, Cary, NC).
Results
During the 3-month initial validation, we followed 13,911 patient days, 2,422 central venous catheter days, and 2,265 urinary catheter days across 15 inpatient units. We identified 406 discrepancies over the period across all counts (2.2% of measurements.). Of these, 62 (15.3%) were adjudicated as errors with the electronic count process (“electronic discrepancies”), and the remaining 344 (84.7%) were attributable to manual data entry errors (“manual discrepancies”). A total of 62 electronic discrepancies and 344 manual discrepancies occurred during the validation period. Electronic errors peaked early, with 50% of the total number of errors occurring within the first 10 days, and 66% occurring by day 30, at which point, discrepancies leveled off, with no errors identified after day 72. Manual errors, by contrast, remained relatively constant, occurring at a nearly fixed rate of 4 per day over the entire period (Fig. 1).
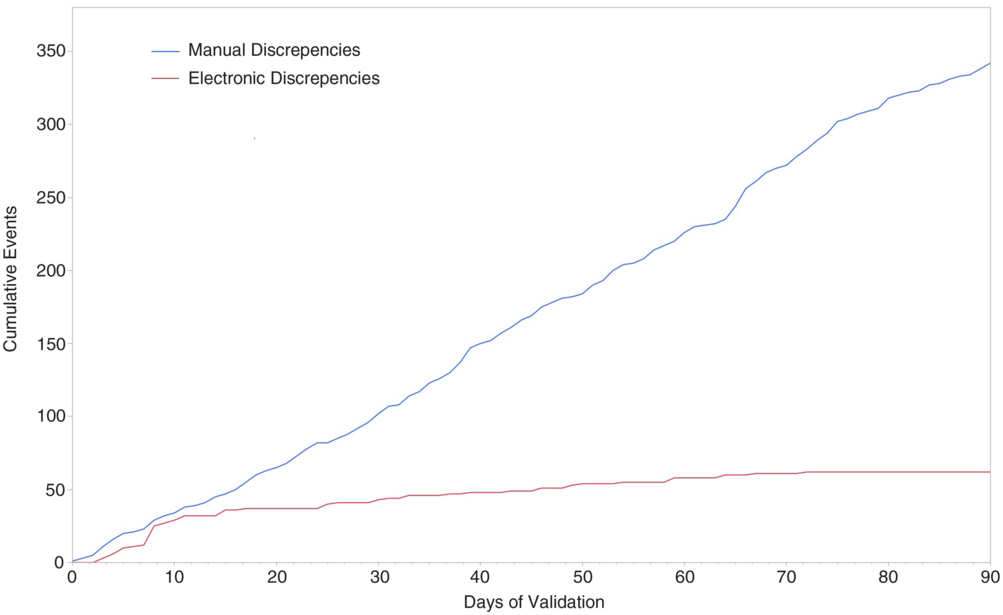
Fig. 1. Cumulative incidence of electronic errors and manual errors during the 90-day validation period.
The types of errors differed when electronic versus manual; census errors accounted for 22.6% of electronic errors and only 3.5% of manual errors. Central line errors were comparable at 51.6% electronic and 56.7% manual errors. Urinary catheter errors were predominantly manual, with 25.8% electronic and 39.8% manual errors. All comparisons were statistically significant (P < .01). However, when analyzed by time period, error types were only significantly different in the first 30 days, converging to similar rates of errors by metric type (see Table 1).
Table 1. Errors by Denominator Metric

a % of errors in each time period is shown as % of electronic errors/% of manual errors.
b Values in bold are statistically significant (P < .01).
Attribution of the cause of the errors was analyzed as well. Of the 62 electronic errors, 45 were attributable to challenges with the logic and programming of the electronic search queries, with the balance being due to mis-charting. More than half of those occurred in the first 2 weeks after transition, and 98% in the first 30 days. Manual errors were universally due to device miscounts.
Discussion
Our data suggest that a 90-day validation period for electronic systems is excessive if manual counts are used as the gold standard. Intensive work in the first days can and should improve the reliability of the electronic system to exceed what can be reasonably expected with a manual process. The limits of manual data collection have been well described in other areas of research. In 2012, a blinded secondary analysis of an out-of-hospital trauma patient cohort compared manual and electronic data processing and found agreement in values between the 2 methods with superior case acquisition from electronic processing.Reference Newgard, Zive, Jui, Weathers and Daya6
Additionally, errors in manual data capture are well known. An assessment of data quality in clinical trials that compared source-to-database capture methods with case report form–to-database capture methods found similar error rates, whereas previous studies have reported manual data abstraction as the largest source of error in clinical trials.Reference Nahm, Pieper and Cunningham7 Digital reporting has also been shown to be highly reliable. In a head-to-head comparison, an automated digital algorithm to identify Charlson comorbidity index comorbidities outperformed chart review in sensitivity, specificity, and positive and negative predictive values for all but 1 comorbidity.Reference Singh, Singh and Ahmed8 These findings echo our results, which demonstrate that electronic data capture can be an equally or more reliable method of validation than the gold standard manual method. Effective use of electronic data is a point of interest for the NHSN, which stated “Conventional methods of data collection … involve a series of procedural steps that are time-consuming, costly, and potentially error prone.”Reference Edwards, Pollock and Kupronis9 To wit, over the 60 days when electronic capture was consistently superior to manual review, nursing time spent on validation was ~930 hours. With the national average nursing hourly wage of $37.37, the estimated cost would be ~$35,700.10 This cost may be mitigated if employees earning a lower wage were used for this task, but for our facility, we determined that nurses would best serve in this role.
The NHSN also suggests ongoing and periodic validation, though the exact mechanism for this is not as specific. Abundant justification can be found for this; staff turnover, renovations or new bed and unit openings, and new devices all may impact device mapping, charting, and the accuracy of data. Although not addressed in the present study, the aforementioned data suggest that periodic rechecks would not need to of a protracted duration if reasonably accurate at outset.
Our findings report only a single health system’s experience with validation, and a single approach to validation; thus, they may not be generalizable to other electronic health record systems. Our daily reconciliation process may not be used at other institutions, and this process may have impacted the rate at which electronic errors were corrected. A system that was totaling errors monthly or weekly may not have yielded the same results.
In conclusion, our experience suggests that a different approach to validation of electronic systems than the current regulatory standard would be more efficient. An appropriate alternative to the currently required 90-day validation period would involve daily reconciliation until the electronic data are within the 5% target for 5 days. This early intensive strategy can reduce the time to achieve validation of an electronic data capture system and could greatly reduce validation-associated expenses. Ongoing intermittent manual review may provide a more efficient method of continued assessment after 30 days.
Supplementary material
To view supplementary material for this article, please visit https://doi.org/10.1017/ice.2020.1
Acknowledgments
The contents of this article are solely the responsibility of the authors and do not necessarily represent the official views of the National Institutes of Health.
Financial support
This publication was supported by a Clinical and Translational Science Award (grant no. UL1 TR002377) from the National Center for Advancing Translational Sciences.
Conflicts of interest
The authors have no conflicts of interest to disclose.