The topic of employee turnover has garnered the attention of companies and researchers for some time (Hom, Lee, Shaw, & Hausknecht, Reference Hom, Lee, Shaw and Hausknecht2017), and rightfully so. Turnover has detrimental effects to company productivity, financial performance, current employee skillsets, and the morale of existing employees (Felps, Mitchell, Hekman, Lee, Holtom, & Harman, Reference Felps, Mitchell, Hekman, Lee, Holtom and Harman2009; Heavey, Holwerda, & Hausknecht, Reference Heavey, Holwerda and Hausknecht2013; Shaw, Gupta, & Delery, Reference Shaw, Gupta and Delery2005). As such, research has tackled this topic and produced theoretical advancements in understanding employee turnover and its effects (Holtom, Michell, Lee, & Eberly, Reference Holtom, Mitchell, Lee and Eberly2008). These advancements span the impacts of general withdrawal research (e.g., Harrison, Newman, & Roth, Reference Harrison, Newman and Roth2006; Hulin, Reference Hulin, Dunnette and Hough1991) to models such as the push-pull model (e.g., Becker & Cropanzano, Reference Becker and Cropanzano2011; Jackofsky, Reference Jackofsky1984), the referent cognitions model (Aquino, Griffeth, Allen, & Hom, Reference Aquino, Griffeth, Allen and Hom1997), the unfolding model (e.g., Lee, Mitchell, Holtom, McDaniel, & Hill, Reference Lee, Mitchell, Holtom, McDaniel and Hill1999), and the job embeddedness model (Mitchell, Holtom, Lee, Sablynski, & Erez, Reference Mitchell, Holtom, Lee, Sablynski and Erez2001), among others. Collectively, this work has advanced our understanding of what leads people to leave organizations.
While our theoretical understanding of turnover is becoming increasingly refined, there has been less of a focus on how to use this research in practice, and there are applied questions that the current literature does not succinctly answer (for discussion of this issue in the field of organizational research at large, see Ones, Kaiser, Chamorro-Premuzic, & Svensson, Reference Ones, Kaiser, Chamorro-Premuzic and Svensson2017). This has become increasingly salient with the rapid rise into the so-called field of “talent-” or “people-analytics” (Bersin, Reference Bersin2015; Bersin, Collins, Mallon, Moir, & Straub, Reference Bersin, Collins, Mallon, Moir and Straub2016; Chamorro-Premuzic, Winsborough, Sherman, & Hogan, Reference Chamorro-Premuzic, Winsborough, Sherman and Hogan2016; Conway & Frick, Reference Conway and Frick2017; Davenport, Harris, & Shapiro, Reference Davenport, Harris and Shapiro2010; Guzzo, Fink, King, Tonidandel, & Landis, Reference Guzzo, Fink, King, Tonidandel and Landis2015; Starbuck, Reference Starbuck2017), which essentially involves the analysis of people-related data to improve business outcomes. Applications of data analysis and psychological theory to understanding human-related work outcomes are nothing new, and yet interest in this type of work has exploded in recent years (e.g., Bersin et al., Reference Bersin, Collins, Mallon, Moir and Straub2016; Guzzo et al., Reference Guzzo, Fink, King, Tonidandel and Landis2015; McAbee, Landis, & Burke, Reference McAbee, Landis and Burke2017). The intersection of advanced computer-based data science with the study of human behavior has further made the field of people analytics a hot topic. As part of the increased interest in data-driven ways to understand the workforce, companies are tackling the prediction of employee turnover with frequency (Chambers, Reference Chambers2016; Es-Sabahi & Deluca, Reference Es-Sabahi and Deluca2017; Gray, Reference Gray2017; Kahabka, Peterson, & Padalia, Reference Kahabka, Peterson and Padalia2017; McCloy, Smith, & Anderson, Reference McCloy, Smith and Anderson2016; Mitchell, Blair, & Speer, Reference Mitchell, Blair and Speer2015; Reeder, Purl, Hughes, Wolters, & Kirkendall, Reference Reeder, Purl, Hughes, Wolters and Kirkendall2016; Rosett & Leinweber, Reference Rosett and Leinweber2017; Shagam, Reference Shagam2017; Yu, Reference Yu2017). This work involves using available data to predict the likelihood of employee and group turnover, essentially producing expected turnover probabilities. This in turn feeds organizational decision making and workforce planning. For example, a company may wish to predict turnover rates across each of its major divisions for the next year to aid in budget and training planning. Another company may wish to identify high-performing employees at risk to terminate and create programs to facilitate their retention.
This type of work is referred to as attrition modeling. Attrition modeling, which may also fall under names such as attrition analysis, flight risk modeling, or turnover modeling, is defined as the application of statistical algorithms to explain, understand, and predict employee attrition (i.e., turnover from the organization). Attrition modeling takes data (i.e., inputs; e.g., test scores, tenure) and systematically transforms via statistical and machine learning procedures such as regression, survival analysis, or random forests (among others) to produce future turnover estimates (outputs) and explain turnover phenomena. This also involves the interpretation of statistical findings to determine the drivers of turnover. Unlike more generalized turnover research endeavors (e.g., benchmarking), which may be more reliant on idiosyncratic interpretations of turnover data, the key to attrition modeling is the data-driven usage of standardized algorithms to explain or project turnover rates. Collectively, this work should then feed into action planning to reduce the phenomena, or general workforce planning and human resources (HR) strategies.
The interest in attrition modeling is not surprising, given how important retention is to business leaders and the rise of analytics work in HR (e.g., Deloitte, 2015, 2016, 2017). The analyses are also a relatively quick win for analytics teams, as data are usually readily available within basic human resource information systems (HRISs). Attrition modeling is a direct application of extant turnover research, and one that yields great value to organizations. For these reasons, it is important for researchers and practitioners to possess the knowledge and skills to guide an organization through these analyses.
Unfortunately, the academic field has been slow to provide concrete applied guidelines for conducting such turnover analyses, at least in a single summarized source. Research allows an understanding of what relates to and impacts turnover, but less guidance on the many practical decisions that must be made when modeling turnover outcomes within organizations, or how to apply psychological research to messier operational data. To our knowledge there is no peer-reviewed resource in the organizational sciences literature that summarizes how to align turnover research with the emerging interdisciplinary practice of attrition modeling specifically, which would be helpful for applied researchers to understand the many nuances and decisions encountered in attrition modeling, and to understand ways in which new scholarly research can impact attrition modeling.
This focal article seeks to introduce the concept of attrition modeling and align the extant literature with this applied practice. Our review is based on the extant turnover literature and our own experiences building turnover models for organizations. It is intended for applied researchers (internal, external) with knowledge of statistical concepts (e.g., logistic regression) and a general understanding of turnover phenomena (e.g., causes and correlates). The article serves as a general introduction and guide to the practice of attrition modeling, and thus it is particularly relevant to those conducting this type of work or just starting it for the first time. Additionally, although this manuscript does not offer a novel theoretical framework, it does help form a concrete bridge between turnover science and practice, and therefore it should also be relevant to academic researchers. This article outlines how turnover research is and can be influencing applied practices, and it highlights gaps where practice would benefit from sound research. Thus, we believe this to be a timely guide that simultaneously provides benefits to both practitioners and academics.
Defining the scope of turnover modeling
Identification of a problem is the first step of consulting work, and this focal article works under the assumption that an organization has already expressed need to predict or understand turnover risk. Under this assumption, the goal is to then to calculate likelihoods and expected turnover rates for employees and organizational groups. However, before even considering input variables and statistical modeling, researchers must understand the task and how to meet organizational expectations. These in turn impact the data analysis strategy. The following section outlines considerations when determining the scope of the turnover definition, understanding the level of operationalization, determining the timeframe of the calibration dataset and how it impacts analysis, and incorporating adjustments for new hires and replacement hires.
Types of turnover
Turnover can be conceptualized in many ways, and considering the classification of turnover types is important for several reasons. For one, all forms of turnover influence workforce planning and the broad span of HR activities that occur as part of that planning. Two, for the sake of explanation or prediction, separation will allow stronger conceptual matching and more accurate predictive modeling. For instance, while age is often negatively correlated with voluntary turnover (Griffeth, Hom, & Gaertner, Reference Griffeth, Hom and Gaertner2000), it is the primary positive correlate of retirements (Adams & Beehr, Reference Adams and Beehr1998). Without separation, this nuance is muddled.
To classify turnover, reliance on the three dimensions described by Allen, Bryant, & Vardaman (Reference Allen, Bryant and Vardaman2010) is useful; they break turnover into either voluntary or involuntary, dysfunctional or functional, or avoidable vs. unavoidable.Footnote 1 These dimensions can be used to mix-and-match turnover into types that make sense within a particular organization. Categorization should be made by the researcher but balanced with organizational needs (i.e., how does the company code turnover), and when doing so it is recommended to balance parsimony, predictor-criterion matching, and analysis needs. For example, the example classifications provided in Table 1 might be used to isolate retirements, involuntary turnover, and voluntary turnover as distinct turnover types with different predictors, and to bucket unavoidable terms in a separate category with less emphasized prediction. If sample size allows adequate analysis, one might further break down some of these groups (voluntary, involuntary) into functional vs. dysfunctional turnover.
Table 1. Example turnover classifications
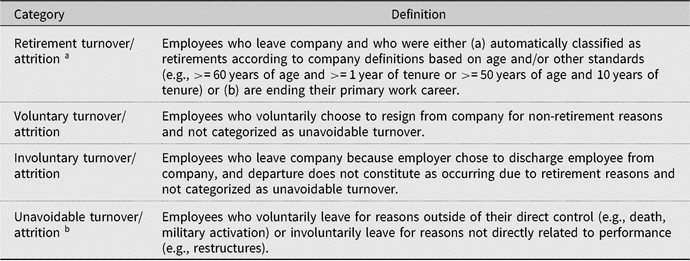
a Feldman (Reference Feldman1994) defines retirements as leaving positions or careers that have been held for long periods of time, stating this is often following a decreased commitment to work. b The “unavoidable” category is akin to an “other” or “catch all” category. We use the phrase “direct” to qualify that the primary driving reason for change is not in employees’ control. Historical rates could be used instead of traditional covariates to provide a likelihood of this form of organizational exit.
It should be noted that there is another common form of employee movement that is often overlooked by researchers: movement from one area of the organization to another (e.g., one division to another division) and labeled “employee churn” or “internal transfers” here. So often the focus of researchers is on exit from a company, but within an organization there are many formal separations in work structures, and leaders frequently care about the employees who leave their area for opportunities in (or escapes to) another part of the company. Once again, this is particularly important for workforce planning and understanding the flow of employee headcount. While the reasons for churning will likely vary some from traditional turnover, there are many reasons for theoretical overlap and why models such as the referent cognitions model (Aquino et al., Reference Aquino, Griffeth, Allen and Hom1997), general dissatisfaction models (e.g., Harrison et al., Reference Harrison, Newman and Roth2006; Hulin, Reference Hulin, Dunnette and Hough1991), and the unfolding model (Lee et al., Reference Lee, Mitchell, Holtom, McDaniel and Hill1999) will apply to churn. Churn is a topic that could greatly benefit from scholarly research attention, and one that should not be ignored when attempting to predict employee turnover/movement.
Timeframe
An important consideration in predicting turnover is defining the timeframe of interest for which the event is expected to occur. Creating a relative rank ordering of employee turnover propensity is relatively easy using basic methods such as logistic regression. However, providing the probability that someone will turn over in the next 3 months, or to project the number of terms (i.e., the number of people who terminated) within the next year, takes a little more thought and in most cases stronger statistical methods, such as survival analysis. This affects the amount of calibration data necessary (at a minimum, the range of calibration data must be equal or longer than the desired prediction period) and the type of calibration analysis performed. It also impacts model effect sizes due to the effects of base rate.Footnote 2 If there is very little turnover occurring during a time period, the lack of variance will attenuate relationships and hurt prediction. For instance, looking at data from a large Midwestern financial company, if we correlate age with retirements over the next year, we get a correlation of .21. In this case, only 2% of the employee population retired during that year. If we perform the exact same analysis but instead conceptualize retirements as occurring over the next ten years, we get a correlation of .60, and 24% of the population has retired. This has direct effects on the assumed accuracy of models. The latter model explains more variance, but the exact same variable is being used for prediction.
Level of analysis
Whether one cares about individual employee turnover or group (i.e., collective) turnover affects operationalizations for both the criterion and predictors. For individual turnover, the focus is on identifying or understanding which individuals will leave an organization. For example, one could rank order employees within a department who are more likely to terminate next year. However, another conceptualization is group-level turnover, labeled collective turnover by Heavey et al. (Reference Heavey, Holwerda and Hausknecht2013) and defined as aggregate employee departures within groups. For collective turnover, the focus is at the group level, whether that be a specific team, a division, or even the entire company. Work done at this level could include creating individual turnover probabilities and then aggregating to group levels for estimates of collective group risk, or simply using group-level variables in the modeling.Footnote 3
Defining the level of turnover is particularly important when considering how results will be used. While creating and presenting individual turnover probabilities is certainly an exciting endeavor, one must balance how that information will be leveraged by the company. Would leaders invest more developmental resources and time in employees likely to leave? Would high flight-risk employees be prematurely pushed out the door? Would low-risk employees have greater advancement opportunities? These factors could have large cultural impacts, and potentially legal impacts, depending on what variables are included in the modeling (e.g., EEO-protected classifications) and whether outputs affect employment decisions. Furthermore, this choice should be balanced with the predictive strength of developed models. If data are limited, it may be challenging to explain large portions of variance in turnover, which could jeopardize trust and increase legal risk. For these reasons, we recommend to not provide individual turnover probabilities, instead opting for group-level predictions to maintain confidentiality (requiring N ≥ 5 or some standard to present a group’s results). This is not to say individual predictions are never warranted. They are riskier though, and at a minimum a predictive model should explain a substantial proportion of variance in turnover (often much easier when predicting retirements). There should also be firm guidelines in place for how to use those data in a specific setting.
New hires and replacements
Another consideration is whether the researcher cares about newly hired employees during the prediction period, as well as replacements to the employees the model deems will turn over. For example, if you are asked to project turnover probabilities for the next year and you use available data from all current employees to do this, these data will not include the new employees who will be hired during the period of interest (because they do not currently exist in the dataset). During this period, the company may increase headcount and hire employees to fill new roles, or the employees who leave may be replaced with new hires. For instance, call centers often experience mass amounts of turnover, and employees who leave a company will usually be replaced by new workers who also have some degree of turnover risk.
This is a tricky and often overlooked issue. In practice, adjusting for new hires due to company growth is more a practice of workforce planning. Without an in-depth planning procedure in place though, it may be reasonable for an analyst to operate under the assumption that anyone who turns over will be replaced, and that the replacement has some degree of attrition risk that should be taken into account. This would be the case if the goal is to provide group-level turnover estimates as a function of a fixed operating headcount.Footnote 4 In this case, the researcher could calculate the probability of new hire turnover by looking at archival data, potentially even fitting it specifically based on the type of employee (e.g., exempt individual contributor vs. non-exempt individual contributor). Then, constant rates of risk can be added to the total probability of termination for a group that serves as the “replacement” risk. In this way, a group (e.g., division, unit) can project the total number of terminations as a function of its expected headcount throughout a period of time.
Summary of scope definition
Before even considering input variables and statistical modeling, researchers must fully understand the problem and the nature of data in front of them. This includes balancing the pros and cons of granularity when categorizing turnover type, defining whether turnover results will be disseminated at the individual or group level, specifying the time frame of analysis and what limitations it may pose on modeling and results sharing, and considering the impact of new or replacement hires when estimating results. Upon considering these factors, researchers should then evaluate what predictors might be available to explain variance in turnover.
Identifying input variables
The academic literature provides an excellent foundation for identifying variables that correlate with turnover (e.g., Griffeth et al., Reference Griffeth, Hom and Gaertner2000; Rubenstein, Eberly, Lee, & Mitchell, Reference Rubenstein, Eberly, Lee and Mitchell2018; Zimmerman, Reference Zimmerman2008; Zimmerman, Swider, Woo, & Allen, Reference Zimmerman, Swider, Woo and Allen2016). Providing an exhaustive list of potential turnover predictors is beyond the scope of this article, and in this section we briefly cover indicators that covary with turnover, building loosely off Holtom et al.’s (Reference Holtom, Mitchell, Lee and Eberly2008) turnover model and Heavey et al.’s (Reference Heavey, Holwerda and Hausknecht2013) causal turnover model to classify variables into broad categories. This classification scheme, which is displayed in Table 2, is one we believe is a helpful taxonomy to guide researchers in identifying groups of predictors, and it is likewise accompanied by example data-sources where variables can most often be found.
Table 2. Turnover modeling input variables and common data sources

To this point, applied researchers have access to a great deal of data in the form of HRIS’s and data lakes, and turnover modeling will often result in larger, more diverse samples than much academic research covers. Additionally, many constructs covered by academic research may not have available measures readily accessible to practitioners. In these cases, practitioners must leverage whatever data sources exist and get creative when forming variables. They must also contend with the fact that even if a type of data exists, there may be challenges in obtaining the data, either due to privacy or liability issues, or internal politics and “data hoarding.” Probably the most focal consideration in people analytics work is determining what data are available and whether and how easily they can be integrated into an analysis. Here we provide some suggestions on what to look for. Thus, while we acknowledge the many predictors of turnover, we try to focus on where practitioners might obtain data most often in practice.
Additionally, it is worth mentioning that different variables correlate with turnover for different reasons. For instance, variables such as job autonomy will affect turnover through mediating effects on attitudes and states (e.g., Holtom et al., Reference Holtom, Mitchell, Lee and Eberly2008), whereas a job shock such as one’s spouse relocating will have direct effects. We do not dive deeply into the theoretical nature of constructs and causal paths, leaving that instead to the extant literature.
Individual worker KSAOs
Research shows that individual abilities, affective dispositions, personality traits, and other individual characteristics (e.g., core self-evaluations) are predictive of turnover and turnover intentions (e.g., Maltarich, Nyberg, & Reilly, Reference Maltarich, Nyberg and Reilly2010; Pelled & Xin, Reference Pelled and Xin1999; Rubenstein et al., Reference Rubenstein, Eberly, Lee and Mitchell2018; Zimmerman, Reference Zimmerman2008; Zimmerman et al., Reference Zimmerman, Swider, Woo and Allen2016). These constructs are most often measured by online or paper-and-pencil assessments, such as those administered for pre-employment purposes, although newer methods can leverage online footprints to derive scores for psychological constructs (Kosinski, Stillwell, & Graepel, Reference Kosinski, Stillwell and Graepel2013). Biodata, which generally include questions about past experiences and behaviors (Mael, Reference Mael1991), can also be included here and are predictive of turnover (e.g., Breaugh, Reference Breaugh2014). As mentioned previously, it is important to consider the contextual nature of the job and organization for how these characteristics might be related to turnover in specific contexts (see Zimmerman et al., Reference Zimmerman, Swider, Woo and Allen2016).
While these characteristics explain individual differences in turnover propensity, it may be rare to have data on all employees in a company, with this being more likely to occur when the scope of analysis is only on a small population (e.g., a single job family). The alternative might be interview or resume data, which are common for most hires in an organization, although it may be tough to reliably score specific constructs. Often, researchers may be forced to rely upon biographical individual difference variables, such as organizational tenure, job tenure, age,Footnote 5 gender, ethnicity, military experience, education, and so forth. These variables will be related to certain forms of turnover and will be readily available from HRISs.
Job characteristics
Decades of research has demonstrated the importance of job design, including its impact on job attitudes and turnover (e.g., Holtom et al., Reference Holtom, Mitchell, Lee and Eberly2008; Rubenstein et al., Reference Rubenstein, Eberly, Lee and Mitchell2018). Job design includes task characteristics such as autonomy, task variety, task significance, task identity, and feedback from the job (Hackman & Oldham, Reference Hackman and Oldham1980). It can also highlight a range of other characteristics, many of which are nicely covered by Morgeson and Humphrey (Reference Morgeson and Humphrey2006). In addition to task characteristics, Morgeson and Humphrey discuss characteristics related to the knowledge requirements of the job (e.g., job complexity), social characteristics (interdependence), and contextual characteristics of the work environment (physical demands). Many of these attributes are best measured using surveys, but researchers might consider leveraging O*NET or existing job analyses for contextual information that can be used for modeling. O*NET provides downloadable job information that describes jobs using a number of characteristics (e.g., abilities) and does so in one common language. Thus, researchers can download these data and match them to the jobs within their organizations to provide a rich set of contextual information. In addition, basic classifiers of job type such as full-time status, exempt status, entry vs. senior exempt roles, and manager level will be readily available in most HRISs and useful in predicting turnover.
Attitudes
The literature is rich with research correlating attitudes and turnover (e.g., Griffeth et al., Reference Griffeth, Hom and Gaertner2000; Harrison et al., Reference Harrison, Newman and Roth2006; Rubenstein et al., Reference Rubenstein, Eberly, Lee and Mitchell2018). Relevant attitudes for turnover modeling include job satisfaction, organizational commitment, job involvement, stress and strain, exhaustion and well-being, psychological uncertainty (e.g., Heavey et al., Reference Heavey, Holwerda and Hausknecht2013; Holtom et al., Reference Holtom, Mitchell, Lee and Eberly2008), and engagement (e.g., McCloy et al., Reference McCloy, Smith and Anderson2016; Rubenstein et al., Reference Rubenstein, Eberly, Lee and Mitchell2018), among a probable many others. Once again, these are most often measured via survey research, and therefore it might be challenging to obtain scores for turnover modeling across an entire organization. Additionally, the frequency of survey collection will matter, with frequent pulse surveys being one option to get up-to-date data. In cases where individual data are not available (perhaps due to vendor confidentiality), using group-level attitudes can be effective, such as leveraging unit-level engagement scores.
Because of the difficulty in obtaining survey data for all employees, applied researchers might rely on proxies for broad attitudes. Online message boards may signal organizational commitment, not to mention well-developed social networks. For example, we have seen companies use “like” vs. “dislike” icons on all internal newsletters, which could then be leveraged to represent attitudes toward the organization and organizational initiatives. Exit interview surveys (e.g., Kulik, Treuren, & Bordia, Reference Kulik, Treuren and Bordia2012) may be another useful data point. If enough employees exited from a department, one could aggregate attitudes expressed in the exit interview (e.g., perceptions of job growth) as proxies for group-level perceptions.
Social relationships
Social integration is an important component of job embeddedness research (Mitchell et al., Reference Mitchell, Holtom, Lee, Sablynski and Erez2001). As a result, it is no surprise that the social context of work is important to employee turnover. One significant aspect of the social work context is the manager (Nishii & Mayer, Reference Nishii and Mayer2009); employee relationships with supervisors are vital to employee perceptions, including job satisfaction and turnover (Graen, Liden, & Hoel, Reference Graen, Liden and Hoel1982). Thus, data representing leadership quality or effectiveness may be good indicators of turnover. Some examples might include leader performance ratings (such as from an annual review or a 360), leader experience, number of electronic communications with the leader, and so forth. Some more indirect indicators might be team size or span of control, as these variables may indicate situations where leaders are less able to provide attention to individual employees. Finally, experiencing a change in direct manager can produce a shock and can be easily operationalized from the HRIS.
Workers with stronger social networks, more friends, and more cohesive units are less likely to term (e.g., George & Bettenhausen, Reference George and Bettenhausen1990; Griffeth et al., Reference Griffeth, Hom and Gaertner2000). Obtaining data points to reflect this may be a challenge, but surveys can be used asking employees about the number of close connections at work. Additionally, email and instant messaging data can be leveraged to create social networks and communication ties. This requires working with the IT department and getting senior-level buy-in, but the data source offers abundant opportunities. Participation in online communities and internal networking might also signal increased retention likelihood (Porter, Woo, & Campion, Reference Porter, Woo and Campion2016). Finally, because similarity breeds liking, group diversity statistics are also helpful (Heavey et al., Reference Heavey, Holwerda and Hausknecht2013; O’Reilly, Caldwell, & Barnett, Reference O’Reilly, Caldwell and Barnett1989), such as calculating a person’s similarity to their unit in terms of age, generation cohort, race, tenure, or technical background. These variables can all be custom-formed based on HRIS data.
Human resource management practices
Indicators relating to HR programs will primarily include pay and benefits, performance management, and training functions. Pay, relative pay (such as pay compared to coworkers or an external benchmarks), and change in pay (e.g., absolute or percent) can be easily gathered and will correlate with turnover (Heavey et al., Reference Heavey, Holwerda and Hausknecht2013). More elaborate pay exploration might include bonuses and variable pay, which could be very important for some jobs (e.g., sales), as well 401k usage or pension data.
Researchers should also pull job performance data, looking at recent performance and also changes in performance, which could indicate disengagement or a person is about to be pushed out of the company. Turnover has long been shown to be related to job performance, and particularly involuntary turnover (Barrick, Mount, & Strauss, Reference Barrick, Mount and Strauss1994; Bycio, Hackett, & Alvares, Reference Bycio, Hackett and Alvares1990; Stumpf & Dawley, Reference Stumpf and Dawley1981; Wells & Muchinsky, Reference Wells and Muchinsky1985). Annual performance ratings will be readily available in most companies, but other performance indicators could include high-potential ratings, ratings of organizational citizenship behaviors, ratings of counterproductive work behaviors, number of promotions, write-ups for employee relations cases, or objective performance indicators. The latter of these is only likely to occur for some jobs. For example, call centers often track metrics such as average handle time, schedule adherence, and customer satisfaction. Similarly, IT jobs may have time to service tickets, and sales jobs will usually have adequate sales data. If fortunate to have these sorts of data, it would be wise to take advantage. Once again, getting access may require close ties to other parts of the organization or access to an internal datamart. Finally, training data may also provide insights. Aside from indicating gains in skills or knowledge, voluntary participation in training courses may also be an indicator of certain individual characteristics (e.g., conscientiousness) or job/company involvement.
Withdrawal
Most companies should possess data that directly reflect employee withdrawal. Such data include absences, lateness, turnover intentions, and withdrawing from long-term company programs such as a 401k. Absences are well known as correlates of turnover, but researchers may also consider operationalizations such as increases in absences or increased occurrence during non-weekend-adjacent days (presumably because the person is interviewing for jobs). Entering a formal leave of absence might also be an indicator of future permanent turnover. Tardies will also have value (e.g., Harrison et al., Reference Harrison, Newman and Roth2006; Rubenstein et al., Reference Rubenstein, Eberly, Lee and Mitchell2018), although this might be limited to non-exempt employees, as few exempt positions will formally track lateness.
Obviously, turnover intentions will be a great predictor of turnover given the conceptual overlap and relationship between intentions and actions (e.g., Griffeth et al., Reference Griffeth, Hom and Gaertner2000; Harrison et al., Reference Harrison, Newman and Roth2006; Rubenstein et al., Reference Rubenstein, Eberly, Lee and Mitchell2018). Collective group intentions might also spur turnover contagion. Despite these points, many companies do not administer turnover intentions surveys to employees on a regular basis, though as noted by an anonymous reviewer, these sorts of questions are often embedded within larger engagement surveys. Of course, new technology may increase other opportunities to capture turnover intentions behaviors, such as job searches and profile updates to work networking sites such as LinkedIn. However, caution needs to be maintained in using external data such as this. An excellent example is the lawsuit of LinkedIn v. hiQ, where LinkedIn issued a cease-and-desist letter to startup hiQ demanding it stop scraping LinkedIn as a data source to facilitate attrition modeling for hiQ clients. LinkedIn claimed that scraping its site is illegal under the Computer Fraud and Abuse Act. Although lower courts have ruled in favor of hiQ, cybersecurity laws are being questioned (epic.org, 2017). Especially given the changing nature of laws around privacy of data and enforcement of the General Data Protection Regulation (GDPR) and European privacy laws, one must be careful about how data (external or internal) are being procured and used.
Organizational context
Holtom et al. (Reference Holtom, Mitchell, Lee and Eberly2008) and Heavey et al. (Reference Heavey, Holwerda and Hausknecht2013) outline a number of macro organizational context factors that are related to turnover, and many are easily leveraged from HRISs. For instance, work location can be an important predictor, as different geographical locations reflect different labor markets, different cultures, may or may not be part of the national headquarters and therefore have more or fewer advancement opportunities and resources, or may involve working from home or not. Researchers could also calculate the distance between home and work locations to create “distance to work or commute” variables, which have been suggested as predictors of turnover (Breaugh, Reference Breaugh2014).Footnote 6 Indicators of site quality and number of people may also be worth exploring (Heavey et al., Reference Heavey, Holwerda and Hausknecht2013), as well as seasonality, such that turnover might be more likely at certain times of the year. In the same vein, researchers should incorporate location or department turnover rates. Divisions within companies will vary in yearly turnover rates, and if the purpose of analysis is to produce collective turnover predictions, it only makes sense to model in the division or location as factors. Similarly, culture and climate affect turnover (Heavey et al., Reference Heavey, Holwerda and Hausknecht2013; Rubenstein et al., Reference Rubenstein, Eberly, Lee and Mitchell2018), and we know that subcultures exist within organizations (Jermier, Slocum, Louis, & Fry, Reference Jermier, Slocum, Louis and Fry1991). However, like many of the variables we have discussed thus far, obtaining survey data on culture perceptions would be the biggest challenge for inclusion in modeling.
Finally, companies frequently go through restructures, which may or may not include downsizing. Any sort of restructure or change to one’s job or immediate team will constitute a shock (Lee et al., Reference Lee, Mitchell, Holtom, McDaniel and Hill1999) and likely will have a drastic effect on attitudes and self-image. Because of this, restructure indicators could be powerful in turnover modeling, if of course there is a way to systematically identify which employees are about to or have just gone through a restructure.
Job alternatives and embeddedness
Lots of research has focused on job embeddedness and job alternatives. We have already covered some job embeddedness indicators (e.g., social connections), but there are other variables that might indicate embeddedness too, such as whether a person was a referral when hired (Pieper, Reference Pieper2015). There is also job and organization tenure, and the number of job changes or the number of division changes within a company might indicate commitment to that company. The same could potentially be said for number of promotions.
People with fewer real or perceived job alternatives are at a lesser threat to leave as well (March & Simon, Reference March and Simon1958). We know that labor market conditions and unemployment rates impact turnover (Schervish, Reference Schervish1983; Terborg & Lee, Reference Terborg and Lee1984), such that lower unemployment rates indicate stronger economies and more opportunity for movement. Census data can be easily leveraged by location to determine rates for different areas of the company. In fact, a wide range of external variables might be beneficial (company stock price, external market availability of skills of the job categories experiencing the most loss). It has also been widely believed that people with higher education levels are more attractive applicants and thus greater flight risks (Becker, Reference Becker1962), and therefore educational data from the HRIS can be leveraged in modeling. Once again, direct measures of job search activity can also be applied, such as increased usage of job networking sites (LinkedIn), or survey methods to investigate perceived job alternatives or external networking (Porter et al., Reference Porter, Woo and Campion2016).
Recap on determining input variables
We have outlined many potential variables to include in turnover modeling. These variables include individual differences, job design factors, attitude and well-being perceptions, social characteristics, HR practices, direct withdrawal indicators, organizational context factors, embeddedness indicators, and job alternatives. Admittedly, obtaining applied measures of many of these characteristics is optimistic, and most researchers would be happy to have measures for just a few. Even then though, this list is certainly not exhaustive, and new and creative ways to measure psychological variables and situational features will be introduced in the future.
With a set of input variables identified, the researcher is prepared to begin analyses to predict turnover. In the next section, we outline analytical strategies for modeling turnover.
Turnover modeling methods
Many different analytical methods can be used to predict turnover (Allen, Hancock, Vardaman, & McKee, Reference Allen, Hancock, Vardaman and McKee2014). In this section, we briefly review several approaches, starting with an overview of general analysis considerations. This section is intended as a general overview, as no thorough review could be given for any of the methods discussed here, let alone all of them. Instead, this coverage is meant to orient the reader toward the common methods used in attrition modeling, some pros and cons of each, and considerations when conducting analyses. Those interested in particular methods should refer to the provided references for more information.
Early analysis considerations
Calibration and holdout samples
Predictive models are developed by using existing data to determine what variables should be used and how those variables should be weighted in the prediction of some outcome. Traditionally in the organizational sciences, researchers have done this by forming models within a single sample and evaluating model fit. This approach, while useful for understanding and theory development, is widely known to produce estimates that perform poorly in future samples due to local overfitting (Kuhn & Johnson, Reference Kuhn and Johnson2013). Instead, researchers should be leveraging calibration and holdout samples, where models are developed on a subset(s) of available data (i.e., calibration sample) and then model performance tested on an independent subset(s) of data (i.e., holdout sample) to determine accuracy in predictions.
At a most basic level, a researcher might split a sample into a majority for calibration (e.g., 70%) and then use the remaining (30%) as an independent holdout. In the calibration sample, a model is developed and variables are selected through decision rules (e.g., forward regression). The final model is applied to the holdout sample, where turnover probabilities are calculated and evaluated using fit statistics, allowing for an independent test of how well the model is expected to perform in future samples. However, because data partitioning into calibration and holdout groups will capitalize on chance, it has become increasingly common to perform repeated k-folds cross-validations as opposed to single, static data splits. Under such an approach the data are partitioned and aggregated multiple times to give more stable estimates of cross-validation.Footnote 7 Assuming cross-validation statistics are acceptable, it is then recommended to derive the final model parameters across the entire sample.
At a more advanced level, researchers might break up samples into calibration sets, validation sets, and testing sets, where the validation sets are used to determine tuning parameters that are applied for variable selection and parameter estimation, which are then incorporated into model formation within the full calibration set. Like before, that is then used to predict turnover in the independent holdout (i.e., testing) sample. Putka, Beatty, and Reeder (Reference Putka, Beatty and Reeder2018) recently used this approach with a k-folds cross-validation to produce machine-driven algorithms to predict performance from biodata, and the same principles can easily be applied to the turnover domain (see Es-Sabahi & Deluca, Reference Es-Sabahi and Deluca2017; Rosett & Leinweber, Reference Rosett and Leinweber2017). While we strongly encourage such data-driven methods, a thorough review of this practice is beyond the scope of this article, though it should be noted that machine-learning analogues do exist for the analytical methods covered in this section (Kuhn & Johnson, Reference Kuhn and Johnson2013).
Finally, the need to split data into multiple groups highlights the importance of large sample sizes for attrition modeling. This is coupled with the fact that the outcome is dichotomous; in situations with a low turnover base rate and a small sample, it is more likely that a predictor will perfectly separate the outcome, therefore resulting in an inability to obtain proper predictor weights. Thus, larger samples are necessary and desired, though we do not know of a rule-of-thumb that applies broadly across the various analytical methods. Hosmer and Lemeshow (Reference Hosmer and Lemeshow2000) provide logistic regression guidelines suggesting there should be at least 10 cases for the rarest outcome for every parameter in the model. Under this logic, if there are 500 employees with a 10% turnover rate (50 terms), the model should include no more than five parameters. Thus, our informal recommendation would be to stick to this guideline for the calibration sample. In most analyses we have conducted, however, sample sizes have been in the thousands, which lends much more confidence in predicting an outcome as complex as turnover. As is the case with N, more is always better.
Data cleaning and missing data
Applied data are messy. Like with any analysis, the researcher should undergo a careful inspection of all input variables for inadmissible or excessively influential observations. Typical methods are recommended for diagnosing and treating these data points, such as flagging values 3–4 standard deviations from the mean, inspecting studentized residuals, and evaluating influence, leverage, or Mahalanobis distance statistics. Remedies could include correction, value transformation, value replacement, or case deletion (Cohen, Cohen, West, & Aiken, Reference Cohen, Cohen, West and Aiken2003).
Regarding missing data, we refer the reader to in-depth reviews and contemporary studies for guidance (e.g., Little & Rubin, Reference Little and Rubin1989; Lüdtke, Robitzsch, & Grund, Reference Lüdtke, Robitzsch and Grund2017; McKnight, McKnight, Sidani, & Figueredo, Reference McKnight, McKnight, Sidani and Figueredo2007; Sinharay, Stern, & Russell, Reference Sinharay, Stern and Russell2001). However, we do want to specifically highlight instances of listwise deletion in the turnover modeling context. Many modeling procedures default to listwise deletion, and because of this, researchers must be careful that important subpopulations are not systematically deleted from the calibration sample based on missing data. For instance, newly hired employees will not have performance ratings, but the new hire population is important to include in initial modeling. Thus, some version of imputation will be necessary for some cases.
Once the model is established and applied to use, the researcher is faced with the reality that turnover probabilities need to be calculated for all people (e.g., new hires), no matter what predictor data is missing. This makes listwise deletion once again unrealistic, and one would only use it if employees were missing so much data that a credible probability could not be computed. Instead, the researcher is better off using some sort of imputation technique, or constraining or transforming the outlying data points so to ensure there is output for all cases. Either way, it is wise to put a good deal of thought into imputation and missing data rules early into the project, and especially for how rules will be applied within new data samples. We encourage commentary as to how others have approached these challenges in the field.
Simple bivariate relationships
Research articles and meta-analytical summaries often present turnover findings as zero-order correlations (or technically, point-biserial correlations). Here, turnover is dichotomous (e.g., 0 = stay, 1 = termed) and correlated with other variables. This approach is simple, as one can produce a large correlation matrix of variables quickly and interpret similarly as fast. The correlation is non-partialled from other covariates too, allowing for easy understanding of how variables relate to turnover. Coupled with scatterplots and other visual mechanisms, calculating these simple bivariate relationships is useful, and especially when trying to understand basic relationships between predictors and turnover.
Unfortunately though, bivariate relationships are also subject to some of the issues previously mentioned, such as dependency on time frame, which affects variance. If researchers have data over a long period of time, they can specify time periods to dichotomize turnover (e.g., turnover after 90 days, turnover after 180 days, turnover after 365.25 days), knowing that variance for a dichotomous outcome is maximized when 50% of the cases have experienced the event (Cohen et al., Reference Cohen, Cohen, West and Aiken2003). Although correlations can be corrected so as to represent the relationship had the base rate been .50 (Griffeth et al., Reference Griffeth, Hom and Gaertner2000; Kemery, Dunlap, & Griffeth, Reference Kemery, Dunlap and Griffeth1988), this is still not entirely ideal. Furthermore, while correlations are helpful from a descriptive standpoint, they are not typically used for prediction. The multivariable analog is of course ordinary least squares (OLS) regression, but it is widely known that this procedure is inappropriate for predicting dichotomous outcomes (Huselid & Day, Reference Huselid and Day1991; Morita, Lee, & Mowday, Reference Morita, Lee and Mowday1989, Reference Morita, Lee and Mowday1993) because predictions will not be constrained within 0 and 1. Thus, one must seek statistical alternatives.
Logistic regression
A frequently used procedure for turnover research is logistic regression (Allen et al., Reference Allen, Hancock, Vardaman and McKee2014; Cohen et al., Reference Cohen, Cohen, West and Aiken2003; Tabachnick & Fidell, Reference Tabachnick and Fidell2001). This procedure is similar to OLS in that series of variables can be used to predict an outcome of interest. In fact, it is a special case of the generalized linear model (Cohen et al., Reference Cohen, Cohen, West and Aiken2003). Like OLS, logistic regression produces interpretable regression weights, although they are interpreted on a log odds scale. Also like OLS, various forms of fit statistics can be calculated to aid in variable selection and model evaluation (e.g., likelihood ratio tests, pseudo-R 2 s, as outlined by Cohen et al., Reference Cohen, Cohen, West and Aiken2003). The major difference is that logistic regression is used for dichotomous outcomes and allows for calculation of outcome probability.Footnote 8 Given a particular set of covariates, a researcher can estimate the odds or probability of an event occurring, therefore making it well-suited for turnover research.
While logistic regression is commonly performed on a single dichotomous outcome variable, there can be multiple types of employee departure of interest to researchers within a single study (e.g., voluntary, involuntary). Here, the researcher could run separate logistic regressions for each type of turnover and treating “competing outcomes” (e.g., if focusing on voluntary turnover, then involuntary turnover would be a competing outcome) as censored cases where the outcome did not occur. One could also remove the alternative cases entirely. However, there are issues to these approaches, in that competing outcomes may not be independent (e.g., if someone had been involuntarily terminated, they would likely have voluntarily terminated at some later point if they had not been fired), therefore biasing mean predicted probabilities. An alternative is to conduct multinomial logistic regression, which simultaneously models k nominal outcomes (Tabachnick & Fidell, Reference Tabachnick and Fidell2001). Here, a baseline group is specified (i.e., non-terminations) and k-non-ordered term groups are specified (i.e., voluntary and involuntary turnover as separate categorical outcomes). Then, distinct regression functions are simultaneously calculated for each type of termination, resulting in regression weights and probabilities specifying the likelihood of each possible event.
All told, logistic regression is well-suited to rank-order subjects by turnover likelihood. However, when the timing of the event is of interest (e.g., knowing when someone will terminate), researchers must use a calibration sample that spans a time frame equivalent to the desired prediction time range. To overcome this weakness, researchers have been leveraging a statistical procedure well-suited to predict time dependent outcomes: survival analysis.
Survival analysis
Survival analysis is a family of techniques that analyzes the time it takes for some outcome to occur, such as a death, or in this case, employee turnover. This procedure has increasingly been used for turnover-related research (Allen et al., Reference Allen, Hancock, Vardaman and McKee2014), largely because of how powerful it is for analyzing time-dependent outcomes (e.g., Harrison, Virick, & Williams, Reference Harrison, Virick and William1996; Morita et al., Reference Morita, Lee and Mowday1993). With survival analysis, the expected time for survival (i.e., not experiencing an outcome) is modeled with or without a series of covariates to explain differences in these rates. It works under the assumption that everyone in the data will eventually experience the event, meaning it assumes that anyone currently employed by a company will ultimately turnover (i.e., those who have not yet are said to be right censored).
An in-depth review of survival analysis is beyond the scope of this manuscript (interested readers should see Cohen et al., Reference Cohen, Cohen, West and Aiken2003; Morita et al., Reference Morita, Lee and Mowday1993; Tabachnick & Fidell, Reference Tabachnick and Fidell2001), but we would like to highlight several aspects. First, it is worth noting that the primary advantage of survival analysis over logistic regression is that the dependent variable is time. This allows a researcher to calculate the probability that a subject with a particular set of covariates will terminate after a specified amount of time, as opposed to logistic regression, which requires setting a dichotomy of stayers vs. leavers. With survival analysis, a researcher can calculate the probability of termination at multiple time points (e.g., 90 days, 180 days, 1 year, 5 years), thus allowing for more dynamic and informative output.
In addition to the flexibility of projecting turnover probability across a variety of time points, survival analysis also allows for the modeling of time-dependent predictor variables (Morita et al., Reference Morita, Lee and Mowday1993). These are covariates that change throughout the life of one’s employment, such as promotion to a new job level (example outlined nicely in Morita et al., Reference Morita, Lee and Mowday1993). This could include a range of other variables too, such as attitudes or job performance, where changes have meaningful impacts on turnover (Harrison et al., Reference Harrison, Virick and William1996; Sturman & Trevor, Reference Sturman and Trevor2001).
A number of different types of survival analyses exist, but turnover researchers frequently use the Cox proportional-hazards model, which is a semi-parametric function that requires a hazard function that is proportional for all subjects. Like with logistic regression, one might also want to consider “competing risks” models that account for the different classes of turnover, as survival analysis also allows for multinomial modeling in such instances. Regardless of model chosen, researchers should thoroughly understand the required conditions of survival analysis, such as expectations regarding hazard proportionality, left censoring, and right censoring. Also like with logistic regression, survival analysis will produce predictor weights that can be interpreted to compare predictor influence, and various likelihood ratio tests can be leveraged for variable selection and model evaluation (Rahman, Ambler, Choodari-Oskooei, & Omar, Reference Rahman, Ambler, Choodari-Oskooei and Omar2017; Tabachnick & Fidell, Reference Tabachnick and Fidell2001). Collectively, survival analysis is a powerful tool for the purposes of turnover prediction and should be given consideration when planning turnover analyses.
Classification and regression trees
A widely used method for turnover analysis is tree-based algorithms (e.g., Breiman, Friedman, Olshen, & Stone, Reference Breiman, Friedman, Olshen and Stone1984), which are adaptable at solving either classification or regression problems. These models break respondents into smaller and smaller subgroups based upon independent variables, in essence splitting the sample into multiple branches that resemble a tree; the end result is a set of nodes at the end of branches that provide output values (i.e., probabilities) based on some configuration of the independent variables. As an overly simplistic example, Figure 1 shows how age and tenure might be used to predict retirements. Employees were first split by whether their ages were less than fifty, and then several additional partitions were made. At the end of each path is an expected probability of retirement based on employee characteristics, with employees who are greater than 62 years old and having more than 10 years of tenure having the highest probability of retirement (22.8%). This procedure of splitting into branches is generated by an automatic feature selection based on which variables best differentiate current and termed employees.

Figure 1. Simplistic example classification tree with two predictors.
There are several appealing aspects to classification and regression trees in a turnover context. For one, the nodes (i.e., individual splits) naturally function as a “yes” or “no” distinction, like “term” vs. “not-term.” Two, decision trees can provide nonlinear insights in a way that logistic regression and survival analysis cannot (i.e., do so without a prior specification). This is particularly helpful in not only modeling nonlinear relationships, but also in discovering them in the first place, as the tree creation process will automatically do this. Three, trees are conceptually easy for laypeople to follow, as one must simply trace a tree path to see what combination and levels of variables are associated with a given probability of attrition. Collectively, this nonparametric method is quite appealing for solving turnover problems.
That said, overfitting is an inevitable issue for decision trees, as the method is highly biased to training sets (Kuhn & Johnson, Reference Kuhn and Johnson2013). As such, pruning (i.e., reducing unnecessary complexity) and cross validation methods should be undertaken. Other solutions are to use bagged trees, random forests (Breiman, Reference Breiman1996, Reference Breiman2001), or gradient boosted trees (Friedman, Reference Friedman2001), which from a general standpoint involve forming multiple samplings of many slightly different trees, and then predictions are aggregated across the samplings. This increases the stability of predictions and makes the outcome variable, in a way, more continuous (Putka et al., Reference Putka, Beatty and Reeder2018). The result is superior prediction power, as it takes the average predictions from multiple trees that vary in terms of which cases are used and which variables are used. The downside is that it is a complex algorithm; given the multiple trees, it be hard to explain to the business. One potential alleviation is the use of variable importance indices, which make interpretations easier for nontechnical audiences by providing variable importance rankings across all trees. Either way, classification and regression trees are frequently being used for turnover prediction and are a nice option for turnover researchers.
Additional techniques and ensemble models
There are a wide range of additional analytical techniques beyond those already discussed. These include the likes of neural nets and support vector machines, which can be readily applied to dichotomous outcomes such as turnover. These methods are generally more elaborate and require greater computational power than regression, survival, and tree-based approaches. The interested reader is encouraged to review these methodologies (e.g., Kuhn & Johnson, Reference Kuhn and Johnson2013), but those we have covered in this manuscript should be sufficient for most turnover researchers. A related alternative that also has potential merit is to use several analytical methods to predict an outcome, and then average the predictions across those different methods. This approach, referred to as ensemble modeling, involves combining estimates from multiple predictive algorithms, in effect averaging or canceling out the inefficiencies or biases from each of them (Berk, Reference Berk2006). As a result, ensemble models generally perform quite well (e.g., Berk, Reference Berk2006). In fact, ensemble approaches were used by winners of the Society for Industrial & Organizational Society’s Machine Learning Competition, held during the 2018 annual conference, to predict attrition. Within a turnover context, turnover probability one year from now might be calculated using logistic regression, survival analysis, and decision trees. These individual approaches would each be evaluated individually and as an average across the three to determine the model (or combination of models) that performs best in the holdout sample. In the next section, we discuss various methods of model evaluation for how one might do this.
Model evaluation: Individual predictions
Attrition model evaluation involves interpreting how well a model explains turnover, and it is applied in an iterative fashion to understand first how models should be improved (e.g., adding or removing predictors, altering hyper parameters) and then to generally interpret how well a developed model is working. Regarding the first of these concerns, a poorly performing model should be considered for revision by tweaking model inputs (e.g., adding or removing predictors). Machine learning is particularly powerful in this sense, as models can be automatically tested on holdout samples to determine the optimal hyper-parameters that will influence the final model (which subsequently affects variable selection and variable weights).
Once a final model is formed, we then must evaluate how well it performs in predicting our outcome. Once again, for all these analyses we assume that best practices are used by testing model performance in independent holdout samples. When evaluating models, the emphasis should be on the derived attrition probabilities, which are continuous, and how well they relate to actual turnover. Goodness of fit statistics (e.g., pseudo-R 2 ; Rahman et al., Reference Rahman, Ambler, Choodari-Oskooei and Omar2017; Hosmer & Lemeshow, Reference Hosmer and Lemeshow2000) are recommended, though they will vary based on analysis method. A simplistic index would be to simply correlate predicted probabilities with the actual termination indicator, which produces effects on a scale well-known to industrial and occupational psychologists. However, unless the predicted probabilities contain only two discrete values (not realistic), the maximum observed correlation will be smaller than |1.0| (Nunnally, Reference Nunnally1978), and increasingly smaller as the turnover rate veers farther from 50%. Thus, while researchers might interpret effect sizes in line with traditional standards such as Cohen’s (Reference Cohen1988) correlation benchmarks of .10 (small), .30 (moderate), and .50 (large), researchers should be cognizant of these challenges and potential remedies (Griffeth et al., Reference Griffeth, Hom and Gaertner2000; Kemery, Dunlap, & Griffeth, Reference Kemery, Dunlap and Griffeth1988).
Though model interpretation should be done using continuous estimated probabilities, we also extend our discussion to evaluation of dichotomized predictions, or when researchers classify people into attrition or non-attrition groups. There are times when researchers will dichotomize their predicted outcomes, which, though frowned upon from a statistical sense, may be of practical use for the organization. When decisions are portrayed in this way, it is helpful to organize groupings into classification tables that portray predicted outcomes on one axis (e.g., predicted stayer vs. predicted leaver) against actual outcomes (stay vs. leave) on the other. An example 2 × 2 matrix is shown in Table 3. Once formed, a variety of statistics can be used to describe model performance (e.g., Streiner, Reference Streiner2003). The most basic of these is to compute percent accuracy (or similar statistics that adjust for chance agreement, like kappa). The classification matrix can also allow for the calculations of the number of true positives (model identified cases to term that did term), true negatives (model identified cases to stay that did stay), false positives (model identified cases to term that stayed), and false negatives (model identified cases to stay that did term). The former two represent the number of correctly identified cases.
Table 3. Example 2 × 2 classification matrix

Note. Precision = .30, recall = .38, specificity = .92, percent accuracy = 88%. The number of true negatives is 85, true positives is 3, false negatives is 5, and false positives is 7.
In practice, specifying where to dichotomize the scores is often both arbitrary and difficult for two reasons. One, it is unclear where the cutoff should be set. Many models will use a 50% cutoff, but this cutoff will produce few predicted “terms” unless base rates are high. For more advanced professional positions where turnover rates might be lower, determining an appropriate cut score will be much more challenging. Two, and in relation, base rates affect accuracy conclusions and can lead to misleading statements. For instance, it is not uncommon for consulting firms to tout their models as predicting an outcome with accuracy around 90%. However, if a company has very low turnover, say 5% per year, even a predictor-free model assuming everyone will stay (i.e., naive model) would achieve a 95% accuracy rate!
One potential solution to at least this last issue is to leverage statistics that control for event prevalence, such as precision, recall (i.e., sensitivity), and specificity statistics. Precision identifies the percentage of cases that a model identified as terming that actually did [TP/(TP+FP)]. Recall identifies what percentage of total terminations the model correctly identified [TP/(TP+FN)], and specificity depicts the proportion of stayers who were correctly identified as staying [TN/(FP+TN)]. In Table 3, precision = .30, recall = .38, and specificity = .92. Thus, even though the model correctly identified most stayers, identifying actual attrition was neither precise nor comprehensive. Taken together, these statistics are helpful to avoid misleading conclusions regarding accuracy rates.Footnote 9 At the same time, they still suffer from the fact that it may be challenging to determine how to dichotomize a predicted turnover probability.
A procedure built upon similar principles is the use of receiver operator characteristic (ROC) curves and the accompanying statistic of area under the curve (AUC). ROC curves plot true positive rates [TP/(TP + FN)], which is the percentage of terms who were accurately classified as such and we want to maximize (i.e., sensitivity), against false positive rates [FP/(TN + FP)], which is the percentage of non-terms who were classified as terms and we want to minimize. An example ROC curve is shown in Figure 2, which plots the true positive rate on the y-axis and the false positive rate on the x-axis.

Figure 2. Example receiver operator characteristic curve.
For ROC curves, a completely naive predictor would result in a perfectly diagonal line from the origin (as represented by the gray line in Figure 2), whereas a perfect predictor with a perfect true positive rate and a zero false positive rate would result in a point at the upper left corner of the graph (0, 1). The closer to this top left corner, the better the predictor. To reflect this, AUC statistics are computed to represent how well a model balances these two aspects. This provides an estimate of how much of the plane falls below the plotted line, producing a nice visual to guide cutoff decisions. For instance, if you want to balance the true and false positive rates, you will want to specify a probability threshold (cut-score) with the highest AUC (curve bends close to top left corner). If you care about identifying all terminations irrespective of false positives, you may be willing to choose a lower cut-score threshold, resulting in a point higher on the y-axis but also further on the x-axis. In Figure 2, the AUC is .94, which indicates that the classifier is performing very nicely.
Hosmer and Lemeshow (Reference Hosmer and Lemeshow2000) suggest that AUC values of .70 are considered acceptable and anything above .80 considered excellent. Rice and Harris (Reference Rice and Harris2005) also provide a table comparing r, d, and AUC values. At Cohen’s traditional .1, .3, and .5 cut-offs, the corresponding AUC values are .56, .67, and .79. Given that attrition models usually incorporate many variables in prediction, we have found models frequently approach or surpass .80 to .90, and especially when predicting narrow types of turnover, such as retirements. However, when used on actual predictive holdout samples (i.e., future data), one might find major company events thwarting prediction. One author achieved a .90 AUC on his holdout sample when developing the model, only to find it plummet a year later. Inspection of the data revealed that a large division drastically altered work structure, resulting in a wave of voluntary and involuntary terminations for a traditionally stable group of employees. Thus, statistics alone may not tell the full story.
Model evaluation: Group-level predictions
If the purpose of the analysis is to produce group-level turnover rates, either by aggregating individual probabilities or by using group-level statistics in the modeling itself, it is best practice to determine how the model will perform with group-level predictions. We recommend first conducting model evaluation on individual-level predictions, if they exist. In this case, the model should perform well at the individual level.
When focusing explicitly on the group-level estimates, the outcome is not binomial but continuous (e.g., group 1 turnover for a year was 7%, and group 2 was 15%). As such, researchers might evaluate model performance using deviation-based statistics such as root mean square error (RMSE) or R 2 using the predicted and actual values. When doing this, it is suggested to inspect residual plots to understand the normality of estimates and whether certain types of groups are over- or under-predicted. This might inform what predictors are omitted and should not be (e.g., such as division- or department-level identifiers). If interested in calculating confidence intervals, it also informs whether one can assume normality or whether one should consider a percentile bootstrapping method to produce confidence bands for prediction accuracy. We encourage commentaries on approaches taken by researchers in practice.
Additional practical considerations
Thus far we have described planning turnover projects, outlined potential predictors, and reviewed analytical strategies. Before turning to future research recommendations, we thought it appropriate to highlight two additional considerations when conducting an attrition study: a return to ethical and legal considerations when sharing results, and a brief discussion of strategies when presenting results to the business.
Ethical and legal considerations when sharing results
When sharing findings, the amount and level of results should be balanced with practical, ethical, and legal considerations. We have already addressed the ethical and legal concerns to a degree, but we feel these points should be reiterated. While potentially useful for targeted interventions, individual turnover probabilities could not only impact the behavior of those who are aware of such estimates (e.g., the employee’s manager), but also introduce risk of legal and ethical challenges (even if the model demonstrated perfect prediction). Especially in the case where attrition modeling informs decisions, it would be wise to use group-level output. For example, safer applications might include large-scale interventions on medium-to-high flight risk employee groups where the focus is on addressing attrition drivers or promoting practices to encourage retention; this could have positive influences on the employees most at risk for termination and likely be beneficial for others as well. In either case of individual- or group-level estimates, predictions will have error, and when applied these errors pose legal concerns and jeopardize the legitimacy of the model and therefore a researcher’s ethical role in recommendations. A researcher must fully understand and truthfully present the model’s utility. Also, given the rise of data privacy laws and regulations, a researcher must be well-versed and communicate clearly how the data and results will be used. In fact, the issue of employee data usage is one that researchers should consider up front, and particularly how HR analytics teams determine consent of data use and communication around data usage. We welcome further perspectives on these points from experts in the field, given the ever-changing legal landscape and new policies such as the GDPR.
Presenting information
Ethical and legal considerations in check, results should be shared when they have an opportunity to impact the business. It is wise to restrict the audience to relevant parties who are affected or are capable of making an impact. Often, the results that are shared will vary by leadership level, such that high-level, strategic overviews are given to senior leaders and narrower, potentially more detailed views to lower-level managers who are experiencing the direct impact of attrition. In any case, care should be made to present the information in a digestible way that avoids much of the technical detail behind it. While the researcher should be prepared to answer esoteric questions about the statistics, study findings can be easily dismissed if they are not palatable. This will require good visuals, storytelling, and ideally interactive dashboards to present information. We refer readers to a recent The Industrial-Organizational Psychologist (TIP) article by Litano, Collmus, and Zhang (Reference Litano, Collmus and Zhang2018) for some creative ways to share statistical information to the business. To better illustrate results sharing here, we present an actual use case of attrition modeling.
In our example, one of the study authors was tasked with developing turnover prediction at the individual and department/unit levels for the purposes of workplace planning and potential interventions to limit attrition. Academic research and data availability led to a priori hypotheses about what variables should be included, and then a series of analyses was performed to select final variables and models (separate models developed for voluntary, involuntary, and retirement attrition). Ultimately, a random forest model was generated to produce individual attrition probabilities (for the next year) after examining a suite of options that included logistic regression, neural networks, survival analysis, and random forests. Some variables included from the final model were job performance, tenure, job level, manager flux (i.e., the number of managers an employee had reported to in the past year), job flux (i.e., the number of jobs/positions an employee had held in the past year), regional economic trends (e.g., unemployment rate), pay changes, and demographic isolation (e.g., an employee is the only female member or only ethnically diverse member of a team).
Once the model was formed, individual attrition probabilities were aggregated to the department level and communicated via HR business partners, who presented customized findings to vice presidents and directors across the organization. As the process was still relatively new within the organization and to protect against concerns with using individual predictions, only the group results were shared. Results were shared using interactive Tableau dashboards (allowing for customized filtering of results to specific areas of the company), and the business partners then consulted with each area on results interpretation, use in workforce planning, and to determine any possible interventions. Note that at this step the researchers were reliant on the skills of the HR business partners to communicate information, though there were times when the analysts presented the results themselves. This process worked relatively smoothly in this example, and there was not great difficulty in obtaining access to data at the onset of the project. However, we have heard horror stories of internal politics restricting access to data. We have also gone through lengthy efforts at times to obtain and clean data (e.g., via web scraping) only to find that the variable(s) is not related to turnover. We imagine many readers have experienced their own, similar problems in the field.
Future research to inform the practice of turnover modeling
Throughout this manuscript we addressed a great deal of content, and we have called on readers to provide their expertise on many topics. There is much nuance in conducting a turnover study or building an attrition model, which this article sought to explicate. Perhaps the most salient aspect of this review is that while research outlines what drives turnover, obtaining good measures of those drivers in operational settings is challenging. If there is a need to predict attrition for an entire organization, attitude, intention, trait, and state variables often cannot be measured according to traditional, psychometrically sound methods. Well-developed surveys will always hold a focal position in organizational research, but there are limits when data are needed for an entire workforce and company-wide survey administration is not feasible. Instead, researchers are often forced to rely on proxies and do their best to understand how those proxies might reflect the underlying constructs that are touted in academic research. This situation results in several research initiatives that would benefit practitioners.
First, we need a better understanding of how new measurement methods and operationalizations impact construct validity, as only with construct validity evidence can a researcher be confident that past research findings apply in a given setting and with a given measure. Multimethod measurement studies with simultaneous collection of criterion data would be helpful regarding whether, for example, that intranet communication sentiment overlaps enough with engagement to treat it as a measure of engagement. Many new data sources exist within organizations, and companies are mining those data irrespective of what they measure. Research can do a better job of aligning new measures with existing taxonomies, constructs, and theories to help guide applied researchers. Some of the work on collective turnover (e.g., Heavey et al., Reference Heavey, Holwerda and Hausknecht2013) has provided helpful frameworks in this regard, and many HRIS variables can be aligned. Still, more can be done. For instance, the unfolding model provides a particularly dynamic understanding of employee turnover, but attempts should be made to demonstrate how the data that are available to most companies can be leveraged to make predictions about it. One needs to get creative to operationalize factors such as shocks (e.g., changes in manager, team restructure, low performance rating), scripts (e.g., use exit interviews to create script profiles and use antecedents to predict these profiles), and other aspects of the model.
It should be noted that these alternative operationalizations, as well as the potential for overreliance on empirical approaches, veer from traditional academic norms of having sound, well-defined, and reliable measurements of psychological constructs. We hope that although much of our focus has seemed overly empirical, our intention is in no way to dispel the importance of theory and theory building. Kurt Lewin argued for the practicality of theory, and we certainly agree that reliance on theory is vital to practice; it is core to industrial and occupational (I-O) psychology. Yet, our field is currently faced with access to mass amounts of data, and applied researchers are trying to make sense of those data and determine strategies for appropriate use. The challenge that attrition modelers face is obtaining good measures that assess constructs that we know are highly related to attrition and why they are related to attrition, for all employees within an organization. However, practical concerns, such as not having available measures of well-defined turnover correlates, often creates a situation where attrition modeling has a greater potential for reckless use of data (i.e., using whatever is available) in pursuit of maximum prediction.
Thus, we urge caution, and suggest practitioners attempt to leverage measures that reflect well-known correlates of turnover. For example, if we want to ensure we are including attitudinal variables in the modeling, a compromise to overcome a company’s resistance to long surveys might be to administer brief surveys to all employees (such as pulse surveys) of just a few items for the constructs we know are highly predictive of turnover. On the surface, this would seem to be a nice balance of practical and scientific concerns. Of course, this is not a new idea, and it still does not offer a solution to the context in which there is reason to maintain anonymity of survey responses. Still, the practice of attrition modeling is at least one more selling point to convince leadership of the importance of such surveys.
Beyond these points, this article also highlights the need for meta-analytical studies to conceptualize turnover in its multiple forms, which should involve primary studies providing enough information about the types of turnover to do so. For example, companies care about regrettable turnover, where top-performing employees leave the organization. Parsing samples by performance and KSAO levels would be a way to display these data such that they could be used in meta-analyses. Other splits defining additional types of turnover would also help guide applied researchers in their work, not to mention provide a more refined understanding of turnover in general. Additionally, work on internal turnover, or churn, is limited. A better understanding of this phenomenon would be greatly beneficial to organizations.
From a methods standpoint, quantitatively oriented researchers can provide guidance, via direct empirical or simulation studies, regarding comparisons of analytical procedures (e.g., data partitioning, model choice, necessary sample sizes, evaluation techniques), as well as to how practitioners should deal with the “new hire” and “replacement hire” conundrum (adjusting estimates or predicting turnover risk for employees who do not exist within the local dataset) when performing attrition modeling. While the suggestions outlined in this article are certainly applicable, firmer guidance can be had. Additionally, as advanced machine learning techniques (e.g., deep learning) more frequently infiltrate the business environment, scholarly attention to the application of more automated attrition prediction methods (i.e., “artificial intelligence”) is warranted.
Other questions arise in terms of evaluating return on investment and impact of workforce planning using attrition models. How do such practices affect organizations’ capacities to efficiently hire and train an employee population, as well as long-term staffing goals? What sort of information should be communicated to organizational leaders to get them to adopt projections and use them to alter strategies? How concerned should researchers be of bias and adverse impact of estimations when performing attrition modeling, and especially when group-level variables (e.g., job type) are used? These questions are areas where research would greatly inform practice.
Conclusion
Attrition modeling is an important HR practice that has not received adequate research attention in the organizational sciences. Current literature has much to say about understanding turnover, and much can be applied to attrition modeling. Yet despite the prevalence of this practice, there is little guidance on how to initiate a successful attrition modeling program and how to leverage available research to do so. This focal article rooted the practice of attrition modeling within the extant literature and provided guidance on the many decisions involved when conducting these analyses. First, researchers must understand the company’s needs and design a study that focuses on the relevant type(s) of turnover, conceptualizes turnover at the correct level of analysis, designs an analysis that can appropriately model turnover for the desired projection timeframe, and contemplate additional factors that might impact estimates, such as the need to consider new or replacement hires. Second, researchers must identify theoretically relevant yet measurable variables that might predict turnover. Third, modeling procedures should adequately deal with the likelihood of missing data, use calibration and holdout samples, account for time-dependent prediction needs, and appropriately evaluate the accuracy of developed models. Last, successful attrition modeling should be presented to the business in a way that is applicable, and where return on investment can be derived.
Attrition modeling may be new to some, but it is a powerful tool that allows organizational researchers to directly influence organizations. This article provides background and guidance on this practice, and it acknowledges the many challenges involved when performing this work. Our hope is that this manuscript spurs interest in this practice and stimulates additional research investigating ways to improve turnover management practices within the field.