Multi-method research approaches have become increasingly popular in recent years as tools to make more robust causal inferences in the social sciences (Beach and Rohlfing Reference Beach and Rohlfing2018; Goertz Reference Goertz2017; Humphreys and Jacobs Reference Humphreys and Jacobs2015; Lieberman Reference Lieberman2005; Schneider and Rohlfing Reference Schneider and Rohlfing2013, Reference Schneider and Rohlfing2016; Seawright Reference Seawright2016).Footnote 1 The most common combination involves cross-case comparative analysis (e.g. statistically assessing mean causal effects of a large number of cases) and in-depth within-case analysis (e.g. process-tracing case studies).
The promise of multi-method research in comparative politics is that different methodological tools can compensate for each other’s relative weaknesses, enabling more robust causal inferences to be made. Yet while much progress has been made, there is still considerable confusion about the underlying assumptions and ontological/epistemological underpinnings of different methods for causal inference. The result is that scholars interested in using multi-method designs in the study of comparative politics will receive very different guidance in different accounts, making it into almost an ‘everything goes’ situation.
This contribution intends to clear up some of the confusion by identifying the key points of contention underlying the current debates about multi-method research. Drawing on recent developments in the broader philosophy of science literature (Clarke et al. Reference Clarke, Gillies, Illari, Russo and Williamson2014; Russo and Williamson Reference Russo and Williamson2011), and within social science methodology (Beach and Pedersen Reference Beach and Pedersen2016; Goertz and Mahoney Reference Goertz and Mahoney2012; Ragin Reference Ragin2000), I put forward that there is a larger methodological divide than commonly understood, making true multi-method research very difficult. The divide is between what can be termed a ‘bottom-up’ case-based approach that focuses on tracing how causal mechanisms play out in individual cases, and a ‘top-down’ variance-based approach that assesses the mean causal effect of variables within a population (or sample thereof).
This review article starts by introducing the ontological and epistemological underpinnings of different methods by differentiating approaches into a bottom-up, case-based, and the top-down, variance-based, approach, focusing in particular on their relative strengths and weaknesses in making causal inferences. The key strength of case-based studies is that we learn how a causal process actually works in a given case (or small set of cases); termed ‘how actually’ explanations in the literature. However, the downside is that we are left in the dark regarding how it works within a larger, more diverse population. In essence, we learn a lot about a little. In contrast, a variance-based design enables causal inferences about mean causal effects within populations of cases (or a large sample thereof), but because the inference about a trend is in the form ‘it works somewhere’ (Cartwright Reference Cartwright2011), it is very difficult to make meaningful inferences at the level of individual cases because of ever-present causal heterogeneity.
This article argues that the two approaches diverge on a set of fundamental assumptions that make it difficult for them to communicate with each other, and that make it impossible to claim that they can be combined in a form of methodological triangulation to compensate seamlessly for each other’s relative weaknesses. Here I go a step further than Jason Seawright’s integrative approach (2016: 4–10)Footnote 2 in arguing that the two approaches ask fundamentally different questions and have different types of evidence backing causal inferences.Footnote 3 Properly used, they can, however, supplement each other’s weaknesses because they ask different questions. Variance-based approaches enable the assessment of the magnitude of causal effects of an X on Y across a number of cases. Case-based approaches tell us how a mechanism linking X and Y together works in a particular context. Overall, causal claims are therefore strengthened when we have evidence of both ‘what is the causal effect’ and ‘how does it work here’. At the same time, the core challenge for both is dealing with causal heterogeneity on their way from populations to individual cases or vice versa, which makes it very difficult to find common ground.
The article then reviews recent attempts to develop multi-method social scientific frameworks, including Evan Lieberman (Reference Lieberman2005), Jason Seawright (Reference Seawright2016), Macartan Humphreys and Alan Jacobs (Reference Humphreys and Jacobs2015) and Gary Goertz (Reference Goertz2017). This article shows that existing frameworks for multi-method research take as their starting point one of the overall approaches (case-based or variance-based), but that this has implications for their ability to be combined with either case studies or variance-based comparisons. I argue that the reason that existing approaches are unable to compensate for these relative weaknesses is because they do not recognize the fundamental differences in the types of claims and evidence for these claims that are produced by case-based and variance-based approaches, respectively. The article concludes with a discussion of the challenges that these differences create for the study of comparative politics, and it makes suggestions for how we can move multi-method research on comparative politics forward by taking the existence of two fundamentally different approaches seriously, resulting in two parallel evidential hierarchies that shed light on causal relationships using very different approaches and types of evidence.
Case-based versus variance-based research approaches
A core distinction can be made between what can be termed ‘top-down’ and ‘bottom-up’ approaches to research (Cartwright Reference Cartwright2011; Russo and Williamson Reference Russo and Williamson2011), which maps nicely onto the divide between variance-based and case-based approaches identified by some social scientists (Beach and Pedersen Reference Beach and Pedersen2016; Goertz and Mahoney Reference Goertz and Mahoney2012; Ragin Reference Ragin2000). These differences also map onto the overall divide within comparative politics between scholars who recommend large-n comparisons across many countries and time periods (Lieberson Reference Lieberson1991; Lijphart Reference Lijphart1971), and those who favour bounded comparisons including only a few cases (Collier and Mahoney Reference Collier and Mahoney1996; Ragin Reference Ragin2000). What the work in the philosophy of science has made evident is the nature of the differences in the ontological and epistemological assumptions underlying these two approaches – which unfortunately make true multi-method research very difficult because we are asking fundamentally different questions.
The variance-based approach typically uses large-n statistical methods, although case studies are often subsumed under the umbrella when they are viewed as making the same type of claims (counterfactuals), and when the evidence used for making causal inferences is evidence of difference-making across cases (e.g. Gerring Reference Gerring2017; King et al. Reference King, Keohane and Verba1994). The case-based approach is sometimes termed ‘qualitative’, although this term is less helpful, given that the term is also used to refer to a variety of more interpretivist methods. The core of case-based methods is within-case tracing of causal mechanisms using process tracing, although cross-case comparisons are important for selecting appropriate cases and generalizing mechanistic findings (see Schneider and Rohlfing Reference Schneider and Rohlfing2013, Reference Schneider and Rohlfing2016).
It is important to note that despite many differences, logically the level at which causes are operative is always within a single case. A drug used to treat a sickness is operative in a single patient; it does not have causal effects across patients unless it can be administered to groups. Similarly, an increase in the number of veto players can produce deadlock and joint decision-traps within a political system, but a reform in one country will not produce deadlock across different countries unless there are diffusion or other dependencies across cases. One can potentially learn about the effect that the increase in veto players has by comparing a case where this took place with one where it was absent, where all other things are equal. But at the end of the day, causation always occurs within cases.
The two approaches differ at both the ontological level (causation as counterfactuals versus mechanisms) and the epistemological level. At the ontological level, the core distinction is whether causation is understood in counterfactual terms (Woodward Reference Woodward2003), or in mechanistic terms (Illari and Williamson Reference Illari and Williamson2011; Machamer et al. Reference Machamer, Darden and Craver2000; Waskan Reference Waskan2011). The epistemological distinction that flows from this ontological difference relates to how one learns about causal relationships.
Depending on where one starts, it then becomes difficult to move very far in the other direction. Starting top-down, an analysis can have found the mean causal effect of X on Y across the cases in a population. Yet knowledge of the mean causal effect does not tell us anything about the local causal effects of X on Y in a particular case. To go from mean to local requires either assuming the population is strongly causally homogeneous, or that one has extensive knowledge about all of the probability-raising Xs across cases that would enable one to meaningfully estimate propensity scores for individual cases based on their case scores, something that is highly unrealistic in the topics studied in comparative politics. When one takes individual cases as the analytical point of departure, the goal is to trace how mechanisms play out in individual cases, but having mechanistic evidence from one case tells us nothing about whether similar processes are operative in other cases unless one can also make strong homogeneity assumptions at the level of mechanisms.
Causal heterogeneity means that causes work differently across different cases (units). Causal heterogeneity refers here to all types of causal complexity across a set of cases, including situations where the same cause can produce different outcomes in different contexts (multifinality), different causes can produce the same outcome in different contexts (equifinality), and where the nature of a relationship differs across cases (e.g. positive in cases where factor Z1 is present, negative when factor Z1 is absent).Footnote 4
In contrast, the term mechanistic heterogeneity is reserved for the situation where the same cause and outcome are linked together through different mechanisms in different contexts, or the same cause triggers different mechanisms that are linked to different outcomes. For variance-based approaches, the solution to potential causal heterogeneity is to make probabilistic claims about trends across many cases, but this makes it difficult to say anything beyond educated guesses about individual cases. For case-based approaches, the solution is to bound populations into small sets to avoid flawed extrapolations from single cases to a broad, heterogeneous population.
We now turn to a discussion of the two approaches and the key differences that make true multi-method research so difficult.
Top-down, variance-based approaches: ‘it works somewhere’ claims
Nancy Cartwright (Reference Cartwright2011) has succinctly defined the essence of the types of claims about mean causal effects that variance-based approaches enable; ‘it works somewhere’. In variance-based approaches, the methodological gold standard is an actual experiment (randomized controlled trial, or RCT), which, if properly designed, enables strong causal inferences about the mean causal effect of a given treatment variable within the studied sample (Clarke et al. Reference Clarke, Gillies, Illari, Russo and Williamson2014; Gerring Reference Gerring2011).Footnote 5
Variance-based approaches build on a counterfactual understanding of causation – often developed as the potential outcomes framework (Angrist and Pischke Reference Angrist and Pischke2009; Rubin Reference Rubin2005; Woodward Reference Woodward2003). Counterfactual causation is defined as the claim that a cause produced an outcome because its absence would result in the absence of the outcome, all other things being held equal (Lewis Reference Lewis1986: 160; Woodward Reference Woodward2003). Without evaluating the difference that a cause can make between the actual and the counterfactual, no causal inferences are possible.
In order to assess a counterfactual causal claim, one needs to assess the counterfactual (also known as the potential outcome) empirically, holding the impact of all other potential causes and confounders constant. A counterfactual is relatively easy to see in an experiment, where we compare values of the outcome in cases that receive the treatment with those in the experimental control group that do not (i.e. the counterfactual state), holding other factors constant. Here the lack of treatment in the control group acts as the counterfactual, enabling us to infer that if there is a significant and substantial difference in values of the outcome in the two groups, this difference is the mean causal effect of the treatment. Given the need to compare across cases, variance-based approaches can be termed a ‘top-down’ form of research (Illari and Williamson Reference Illari and Williamson2011). Again, this is best seen in an experiment, where mean causal effects (the average ‘difference’ that the cause makes for the outcome across the treatment and control groups) are assessed within the population of cases in the study. The term top-down is therefore appropriate because causation is studied at the population level (or samples thereof) by assessing trends across cases.
Strictly speaking, observational data in the form of statistical covariation of causes and outcomes across many cases does not enable causal inferences to be made unless we assume that the data have the character of a natural experiment that enables us to claim that our population is split (either temporally or spatially) into a treatment and control group in which everything else is constant (Angrist and Pischke Reference Angrist and Pischke2009). Even more challenging is the claim that we can make causal claims based on counterfactuals when studying single cases. One way of proceeding is to transform ‘one case into many’ by disaggregating a case either spatially or temporally, enabling a (weak) assessment of the counterfactual in the form of a most-similar-system comparison (everything else is equal except variation in the cause) (King et al. Reference King, Keohane and Verba1994: 217–228). Another way of doing variance-based case studies involves using counterfactual single case studies, where hypothetical evidence about ‘what might have been’ is used as the counterfactual comparison. The logical argument is then made that if a particular cause had not occurred, the outcome would not have occurred (Fearon Reference Fearon1991; Goertz and Levy Reference Goertz and Levy2007; Lebow Reference Lebow2000; Levy Reference Levy2015; Tetlock and Belkin Reference Tetlock and Belkin1996).
Key to the ability to make inferences about mean causal effects are the assumptions of unit homogeneity and independence of units (Holland Reference Holland1986; King et al. Reference King, Keohane and Verba1994: 91–97). Unit homogeneity means that the same cause will produce the same results in two or more cases (i.e. causal homogeneity, also termed stable unit treatment effect; Morgan and Winship Reference Morgan and Winship2007: 37–40). Independence of units means that the potential outcome in one case is unaffected by values of the cause in other cases. If these two assumptions do not hold, we will have biased estimates of the difference that variations in X have for values of Y.
In variance-based research, these two assumptions hold when we have many units that are randomly selected into treatment and control groups, thereby ensuring that any differences between units wash out at the level of comparisons of large groups. Independence is ensured best in an experiment, where random selection ensures that the values of X are independent of values taken by Y.
In variance-based approaches there is a clear evidential hierarchy that relates to the evidential strength of causal inferences made within the given study (i.e. internal validity) with respect to whether these two assumptions hold (Clarke et al. Reference Clarke, Gillies, Illari, Russo and Williamson2014; Gerring Reference Gerring2011). Actual experimental designs are at the top, enabling strong causal inferences to be made, followed by natural experiments using observational data where one can assume that the treatment and control were ‘randomly’ assigned by nature. A natural experiment is in effect a most-similar-system design (MSSD) using observational data.
Findings from case studies are at the bottom of the evidential hierarchy because they tell us precious little about trends when causal heterogeneity is present in a population (see below). The assumptions of unit homogeneity and independence almost never hold when engaging in a small-n comparison of difference-making. For example, almost any one-into-many transformation of cases will result in a set of cases that are not causally similar, and there will also be serious violations of case independence where values of X in one case will be affected by values of Y in preceding or simultaneously occurring cases. With regard to unit homogeneity, disaggregating a negotiation as a case temporally into stages (t0, t1, . . . tn) results in cases that are quite causally dissimilar, where we can expect critical differences in how causes/mechanisms play out when comparing early stages (agenda-setting) and the end game. In addition, the ‘cases’ would not be independent of each other, because in a negotiation, what happens at the start (t0) naturally affects events later in the negotiation, meaning that values of Y in case t0 will influence values of X in subsequent cases (periods of the negotiation). If we disaggregated the negotiation into different issue areas instead of temporally, we should expect that deals or deadlock with respect to one issue (case) will affect other important issues (other cases), especially in a setting where package deals are typical forms of resolving negotiations. The different ‘cases’ would also not be homogeneous in that we would expect that factors such as expertise might matter more in low-salience issues and matter less in highly salient issues in which actors have incentives to mobilize the necessary informational resources to understand an issue. Gary King, Robert Keohane and Sidney Verba (Reference King, Keohane and Verba1994: 222) even admit that this is a problem, concluding, ‘When dealing with partially dependent observations, we should be careful not to overstate the certainty of the conclusions.’
At best, case studies can therefore help us detect measurement error or find potential confounders when engaging in more exploratory research that can help us improve the statistical models we use to explore population-wide difference-making (Seawright Reference Seawright2016: 45–69).
Similar problems occur when we try to identify two or more cases that can be compared using a MSSD. As Rosa Runhardt (Reference Runhardt2015: 1306) admits, ‘A similarity comparison in areas like political science is, however, difficult to defend.’ Because of the complexity of the social world, it is difficult to find cases in which the ‘all other things equal’ assumption required in a natural experiment (MSSD) actually holds (Ragin Reference Ragin1987: 48). Jack Levy (Reference Levy2015: 390) writes that ‘Controlled comparison and matching face the difficulty of finding real-world cases that are identical in all respects but one.’ But unless we can substantiate that all other things are equal except for the presence/absence of a cause, we cannot make a causal inference that its absence made a difference for the outcome.
There are two critical weaknesses of top-down, variance-based research that make it difficult to communicate meaningfully with case-based research, one of which can be resolved to some extent, the other not. First, because probabilistic claims are made about mean causal effects in a population (or a sample thereof), it is very difficult to move to the level of individual cases because of potential causal heterogeneity. If a population was completely causally homogeneous, unit homogeneity (stable unit treatment effect) would hold perfectly (Morgan and Winship Reference Morgan and Winship2007: 37–40; Rubin Reference Rubin1980: 961), meaning that population-level trends would be perfectly predictive for effects in individual cases (Cartwright Reference Cartwright2007: 154–159). But given the causal complexity of the real world, there can be many reasons that the relationship does not hold in individual cases, including omitted variables such as contextual factors (Williams and Dyer Reference Williams and Dyer2009: 210–211). Because of this, ontologically probabilistic claims are made about trends (i.e. mean causal effects).
When one then moves from population-level causal claims about trends to individual cases, causes become ‘probability-raisers’ (Gerring Reference Gerring2011: 199). Mean causal effects are averages across a study population, but there can be different combinations of other factors for any given case (Cartwright Reference Cartwright2012: 980–981; Leamer Reference Leamer2010). If there is a positive relationship between X and Y, a high value of X would make it more probable that we would find a high value of Y in case A. Based on what we know about mean causal effects of different independent variables and the impact of confounders, propensity scores can in theory then be estimated for individual cases. However, to do this requires either that we have evidence of a high level of causal homogeneity in the population being studied that enables one to assume overall treatment effects apply to individual cases in a predictable fashion, or we have in effect mapped the causal heterogeneity embedded within the population, enabling cases to be grouped together into more homogeneous subsets of cases (e.g. there is a negative relationship between X and Y when factor Z1 is present, whereas there is no relationship in cases where factor Z1 is absent). If neither holds, there is the significant risk of an ecological fallacy when inferring from population-level trends to individual cases (Robinson Reference Robinson1950). Actual experiments have the further difficulty that their inferences do not necessarily hold outside the controlled laboratory setting, meaning that the ability to infer to cases outside the lab is even further reduced.
Second, even if we were able to estimate accurately propensity scores for individual cases, studying causal claims by comparing values of X and Y across cases would not tell us how causes work within a case. In other words, we learn about the difference variation in X makes for values of Y, but we do not learn anything about the causal arrow linking the two – it remains firmly within a black box. An experiment does not tell us how a treatment works – only that there is a mean causal effect (Dowe Reference Dowe2011; Illari Reference Illari2011; Machamer Reference Machamer2004; Russo and Williamson Reference Russo and Williamson2007; Waskan Reference Waskan2011). In order to learn about how causes actually work within cases, we need to move away from counterfactual difference-making to explore how causal processes play out in actual cases.
Concluding, variance-based approaches are top-down methods that assess counterfactual causation in the form of mean causal effects across cases. Relative strengths include the ability to assess the magnitude of net causal effects, and the ability to make causal inferences about many cases (populations or samples thereof). The core weakness relates to our ability to say anything meaningful about individual cases because of the risk of causal heterogeneity within populations, meaning that at most we can make educated guesses using case propensity scores.
Bottom-up case-based approaches: how causes work in cases
Case-based approaches are ‘bottom-up’ because the in-depth study of individual cases is the analytical point of departure. Here the goal is to learn about causal mechanisms and how they operate in particular cases (Russo and Williamson Reference Russo and Williamson2011). Mechanisms are not causes; they are what link causes and outcomes together. In a case-based understanding, causal mechanisms are more than just lower-level counterfactual claims. If one takes mechanisms seriously, the goal is to explore what process actually was operative in a case (Groff Reference Groff2011; Machamer Reference Machamer2004: 31; Waskan Reference Waskan2011). A ‘mechanism explanation for some happening that perplexes us is explanatory precisely in virtue of its capacity to enable us to understand how the parts of some system actually conspire to produce that happening’ (Waskan Reference Waskan2011: 393). In the words of Jim Bogen (Reference Bogen2005: 415), ‘How can it make any difference to any of this whether certain things that did not happen would have or might have resulted if other things that did not actually happen had happened?’ Ruth Groff (Reference Groff2011: 309) claims that mechanisms are real processes that involve the exercise of causal powers in the real world, not in logically possible counterfactual worlds. The essence of mechanistic explanations is that we shift the analytical focus from causes and outcomes to the hypothesized causal process in between them. That is, mechanisms are not causes but are causal processes that are triggered by causes and that link them with outcomes in a productive relationship.
In case-based approaches, the focus is on tracing the operation of causal mechanisms within cases (Beach and Pedersen Reference Beach and Pedersen2016, Reference Beach and Pedersen2019). The core elements of a causal mechanism are unpacked theoretically and studied empirically in the form of the traces left by the activities associated with each part of the process. Each of the parts of the mechanism can be described in terms of entities that engage in activities (Machamer Reference Machamer2004; Machamer et al. Reference Machamer, Darden and Craver2000). Entities are the factors (actors, organizations or structures) engaging in activities, whereas the activities are the producers of change or what transmits causal forces or powers through a mechanism. Mechanisms are here viewed in a more holistic fashion than mere counterfactuals, meaning the effects of a mechanism are more than the sum of its parts. When a causal mechanism is unpacked theoretically as a system, the goal becomes to understand how a process actually works by tracing the operation of each part (or at least the most critical parts) in one or more cases.
Mechanisms are traced empirically by collecting mechanistic evidence, which is the observable fingerprints left by the operation of the activities associated with parts of mechanisms (Illari Reference Illari2011; Russo and Williamson Reference Russo and Williamson2007). Here there is no variation; instead it is the empirical traces and their association with activities that enable us to infer that we have evidence of a mechanism linking a cause (or set of causes) with an outcome (Beach and Pedersen Reference Beach and Pedersen2019; Clarke et al. Reference Clarke, Gillies, Illari, Russo and Williamson2014). Mechanistic evidence is observational data, trying to capture what really took place within individual cases.
In case-based research, the detailed tracing of processes using mechanistic evidence within individual cases is at the top of the evidential hierarchy. Below this are weaker within-case methods that only obliquely trace mechanisms (congruence studies and analytical narratives), thereby not enabling strong causal inferences. At the bottom are comparisons across cases using methods like qualitative comparative analysis (QCA) that can be used to find potential causes, select appropriate cases for within-case analysis and enable cautious generalizations about processes to small, bounded sets of cases.
There are two weaknesses of case-based approaches that are in many respects the antithesis of variance-based approaches. First, taking individual cases as an analytical point of departure requires making deterministic causal claims about mechanisms (Beach and Pedersen Reference Beach and Pedersen2016: 19–24; Mahoney Reference Mahoney2008). If one is interested in trends, why would one explore the trend within a single case? However, knowledge about detailed causal mechanisms that are operative in single cases cannot easily be exported to other cases because mechanisms are sensitive to even slight contextual differences (Bunge Reference Bunge1997; Falleti and Lynch Reference Falleti and Lynch2009; Gerring Reference Gerring2010; Goertz and Mahoney Reference Goertz and Mahoney2009). In Cartwright’s language (2012), we learn about how ‘it works here’, but it is difficult to extrapolate that it also ‘works there’. This means that mechanistic heterogeneity can be produced by contextual differences, defined as situations: (1) where the same causes trigger different processes in two or more cases, thereby resulting in different outcomes, or (2) where the same cause is linked to the same outcome through different processes. The risk of the first variant can be reduced through careful mapping of the population by scoring cases on their values of the cause, outcome and contextual conditions. However, the second scenario is more problematic because mechanistic heterogeneity might be lurking under what might look like a homogeneous set of cases at the level of causes/outcomes. Given this sensitivity, our ability to generalize from studied cases to other cases using comparisons is significantly weakened. We trade higher internal validity of causal inferences for a more limited ability to generalize beyond the studied population (i.e. lower external validity). Extrapolating from the individual (or small group) to the full population in this situation would result in an atomist fallacy.
The alternative to taking mechanistic heterogeneity seriously by appreciating the complexity of real-world cases and the limited bounds of generalization of mechanisms because of contextual sensitivity is to lift the level of abstraction about our theorized mechanisms to such a high level that our theorized mechanisms are in essence nothingburgers that tell us precious little, if anything, about how a process works in real-world cases. Yet this tells us nothing about how these processes actually play out in real-world cases. Instead of lifting the level of abstraction to the level of a one-liner, case-based scholars make more extensive claims about processes operative in smaller, bounded sets of cases.
Many variance-based scholars are sceptical about making relatively particularistic, bounded inferences. John Gerring (Reference Gerring2017: 234) writes that ‘social science gives preference to broad inferences over narrow inferences. First, the scope of an inference usually correlates directly with its theoretical significance … Second, broad empirical propositions usually have greater policy relevance, particularly if they extend to the future. They help us to design effective institutions. Finally, the broader the inference, the greater its falsifiability.’ Scholars within the case-based approach counter that complexity and contextual sensitivity are key features of 21st-century science, seen in developments in fields like systems biology or personalized medicine (Ahn et al. Reference Ahn, Tewari, Poon and Phillips2006; Bechtel and Richardson Reference Bechtel and Richardson2010; Cartwright Reference Cartwright2007, Reference Cartwright2012; Levi-Montalcini and Calissano Reference Levi-Montalcini and Calissano2006). Instead of research that aims to evaluate the effect of individual treatments in isolation across large heterogeneous populations, systems biology and personalized medicine seek to investigate how treatments work within subgroups of complex, real-world systems – in other words, small bounded populations of relatively similar cases. Appreciating complexity means that our claims become more contextually specific (Bechtel and Richardson Reference Bechtel and Richardson2010). Instead of engaging in a simple experiment that isolates the effect of a treatment in a controlled environment, researchers are increasingly interested in exploring how things work in particular contexts (Cartwright Reference Cartwright2011, Reference Cartwright2012). In the case of personalized medicine, this could mean that we understand how a treatment works in a particular type of patient (e.g. one taking other medications because of commonly occurring complications), but we do not assume that the treatment would work in other patients who may be taking other medications for other diseases. Instead of one-size-fits-all claims, personalized medicine would try to understand what might work in a particular patient type.
But appreciating complexity does not mean that we cannot engage in cumulative research. Ideally, after intensive collaborative research over a longer time period, the result would be an evidence-based catalogue of different mechanisms that are triggered by a given cause (or set of causes) in different contexts. Naturally, this type of research demands more resources, but this is not an excuse to engage in sloppy generalizations about mechanisms.
Finally, Gerring’s claim about policy relevance does not match recent developments in the field of policy evaluation, where there is increasing interest in the tracing of mechanisms as an analytical tool to study how interventions work in particular contexts instead of working with broad propositions that tell us little about how things work in the real world (Bamanyaki and Holvoet Reference Bamanyaki and Holvoet2016; Cartwright Reference Cartwright2011; Cartwright and Hardie Reference Cartwright and Hardie2012; Clarke et al. Reference Clarke, Gillies, Illari, Russo and Williamson2014; Schmitt and Beach Reference Schmitt and Beach2015; Wauters and Beach Reference Wauters and Beach2018).
The second key challenge is the problem of ‘masking’ (Clarke et al. Reference Clarke, Gillies, Illari, Russo and Williamson2014; Steel Reference Steel2008: 68). Masking means that a given cause might be linked to the same outcome through multiple mechanisms that can have different effects on the outcome. For instance, exercise triggers two different mechanisms: one related to weight loss through burning calories, and the other related to weight gain through building of muscles. Tracing the ‘burning calories’ mechanism between exercise and weight loss does not enable us to assess the overall causal effect of exercise on weight. For us to be able to study and assess net causal effects, variance-based designs are required.
Conclusion
In Figure 1, the left side depicts a bottom-up, case-based approach for making causal inferences. The core of research here is the detailed, within-case, tracing of causal mechanisms in individual cases. Cross-case analysis is typically done at the ‘meso-level’, here depicted as the mid-section where there are small bounded populations of cases. The comparative methods used are typically tools like QCA (Schneider and Rohlfing Reference Schneider and Rohlfing2013, Reference Schneider and Rohlfing2016), or even simpler applications of Mill’s methods (see Beach and Pedersen Reference Beach and Pedersen2016; Berg-Schlosser Reference Berg-Schlosser2012; Goertz and Mahoney Reference Goertz and Mahoney2012; Ragin Reference Ragin2000). The key downside is the risk of the atomist fallacy, where flawed generalizations are made from the individual to larger groups of cases.
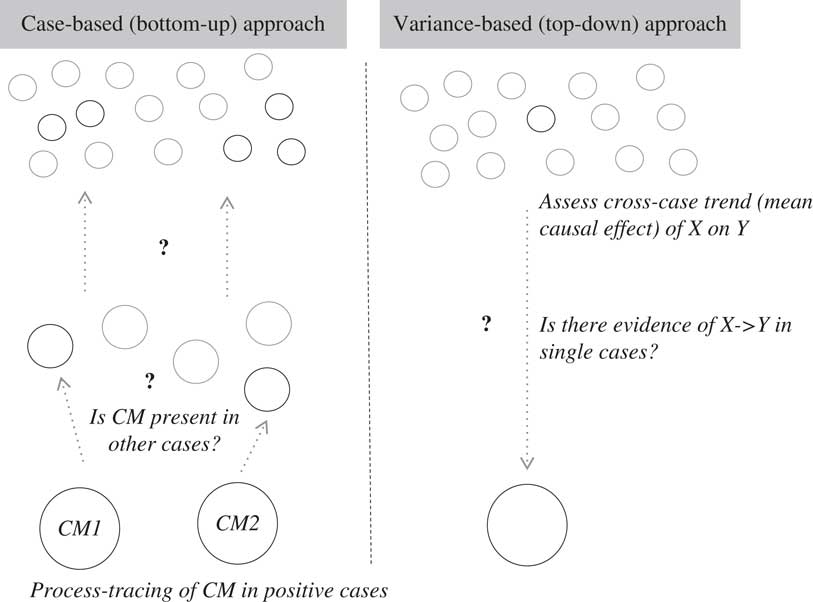
Figure 1 Case-Based versus Variance-Based Approaches
In contrast, in the variance-based approach, the core of research deals with experimental or quasi-experimental manipulation of a cause (independent variable) within a population, controlling for potential confounders. This enables inferences about the mean causal effects of X on Y. The downside is the risk of ecological fallacies when we go from trends to the individual.
These downsides make it very difficult – if not impossible – for inferences made within one approach to travel to the other. I now turn to a short review of several of the most prominent recent attempts at multi-method methodology, showing that they tend to stay within one approach, thereby also having the same strengths and weaknesses as the overall approach. I conclude by putting forward two complementary evidence hierarchies as a way to move forward, thereby also recognizing the fundamental gulf between case-based and variance-based approaches.
Existing multi-method frameworks
In the following, I will walk briefly through Lieberman’s (Reference Lieberman2005), Humphreys and Jacobs’ (Reference Humphreys and Jacobs2015), Seawright’s (Reference Seawright2016) and Goertz’s (Reference Goertz2017) frameworks for multi-method research. I show that each suffers the same weaknesses produced by the analytical starting point (top-down/bottom-up).
Lieberman: nested analysis
In a widely cited article from 2005, Lieberman put forward a framework for multi-method research that suggests that we always start with a large-n regression analysis. If the regression finds a robust correlation between X and Y, controlled for other factors, the analysis can then move on to testing the found X/Y relationship using small-n analysis.Footnote 6 Small-n analysis is defined as everything from ‘qualitative comparisons of cases and/or process-tracing of causal chains within cases across time, and in which the relationship between theory and facts is captured largely in narrative form’ (Lieberman Reference Lieberman2005: 436). When dealing with making robust causal inferences, the goal of the small-n analysis is to improve the model specifications used in the large-n analysis by exploring the causal order of variables and exploring the impact of rival explanations (Lieberman Reference Lieberman2005: 436, 440).
The small-n analysis proceeds by selecting a case (or small set of cases) that fits with the X/Y relationship found using the large-n analysis; in other words, they are on or near the regression line, with small residuals. Ideally, cases are selected that exhibit the widest degree of variation on the independent variables that are central to the large-n analysis model (Lieberman Reference Lieberman2005: 444). However, no guidance is given as to how many cases are required to update our confidence in the large-n analysis inferences about mean causal effects.
In the actual small-n analysis, Lieberman discusses many variants of case study research, and mentions the distinction between data set and causal process observations. But the core of a small-n analysis builds in his view on assessing a counterfactual. He writes, for instance, that small-n should ‘demonstrate within the logic of a compelling narrative that in the absence of a particular cause, it would have been difficult to imagine the observed outcome’ (Lieberman Reference Lieberman2005: 442). This suggests that at its core, evidence of difference-making is used for both the large-n and small-n analyses. After finding within-case evidence in one or more cases that fits with the large-n analysis, the analyst can conclude that the X/Y relationship is robust across different methods.
However, by starting with a large-n analysis, Lieberman’s framework runs into the same challenges of all variance-based approaches, which is to say something meaningful about individual cases. Despite suggesting that small-n analysis can counter problems related to ‘causal order, heterogeneity of cases’ (Lieberman Reference Lieberman2005: 442), his framework offers no solution to the heterogeneity problem. He suggests that one should focus more on studying a small number of cases, writing:
more energy ought to be devoted to identifying and analysing causal process observations within cases, rather than to providing thinner insights about more cases. Because the inherent weakness of small-n analysis is its inability to assess external validity, there is no point in trying to force it to do this when the large-n analysis component of the research design can do that work. (Lieberman Reference Lieberman2005: 441)
Yet how can a small-n study of one or a small number of cases that provides evidence of a local causal effect inform us about the mean causal effect across a population unless we impose unrealistic assumptions about cases being homogeneous, where X and all possible confounders work in the same fashion throughout the population? Unfortunately, large-n correlations can mask situations where one set of confounders is present in a set of cases that enable X to have a large effect on Y, whereas another set of confounders is present in other cases, resulting in a small effect of X, and for other cases with other combinations of confounders, there might even be a negative contribution of X (Cartwright Reference Cartwright2012: 981). This type of heterogeneity – which should be expected in most messy social science data – means that plucking a few ‘onlier’ cases from an X/Y regression tells us nothing meaningful about the mean causal effect.
If we understand the contribution of small-n case studies as being focused on providing evidence of what is going on in between, Lieberman’s advice to select regression ‘onliers’ also becomes highly problematic. Mechanisms are only present when the cause actually does something, meaning that mechanisms are only triggered in positive cases in which the value of X is above a certain threshold at which the mechanism kicks in. In cases with low values of X and Y, we should therefore not expect a mechanism to be present. Logically, if a person does not smoke, no mechanism is triggered that could link it with lung cancer. Therefore, mechanistic claims are inherently asymmetric, which means that they can only be studied in cases where the cause and contextual factors required to trigger a mechanism are present (Beach and Pedersen Reference Beach and Pedersen2016, Reference Beach and Pedersen2019; Goertz Reference Goertz2017). With Lieberman’s case selection advice, we might have selected a low X/Y case to trace a mechanism – which would mean that we would be trying to study it in a case where we know a priori based on case scores that it cannot be present.
Humphrey and Jacobs: Bayesian multi-methods
Humphrey and Jacobs’ Bayesian framework for combining within-case and cross-case analysis is focused on estimating mean causal effects across cases, with within-case analysis an adjunct tool to update our confidence in a cross-case trend by using a different data type to learn about causal effects. Large-n cross-case analysis has the goal of estimating the mean causal effect of X on Y across a population (Humphreys and Jacobs Reference Humphreys and Jacobs2015: 658–660), whereas within-case analysis using process-tracing is mustered to provide ‘clues’ (causal process observations) that shed more light on whether there is a causal relationship between X and Y in a given case (Humphreys and Jacobs Reference Humphreys and Jacobs2015: 656).Footnote 7 Information from the single case is then used to update our confidence in the population mean causal effect based roughly on the proportion of studied cases to the population.Footnote 8 Other things equal, the more cases studied as a proportion of the population of cases, the more confident one can be about the size of the average causal effect in the population. Here they impose strong assumptions about unit homogeneity on populations, assuming that clues about a relationship in a single case (i.e. local treatment effect) can be used to update our knowledge about the mean treatment effect. Humphreys and Jacobs (Reference Humphreys and Jacobs2015: 669) do admit that there can be situations where there is a risk of causal heterogeneity in the form of different causal effects across cases within a population. They suggest that, when heterogeneity is present, we should study more cases because each individual case does less to update confidence in mean causal effects. When there is very strong heterogeneity, they suggest that case studies no longer tell us anything about trends (Humphreys and Jacobs Reference Humphreys and Jacobs2015: 669).
While they provide a comprehensive multi-method model, it suffers from two weaknesses created by its variance-based starting point. First, while their framework enables single case-to-population updating to take place, they provide us with no tools for going in the other direction, i.e. estimating whether an individual case reflects a population-level trend.
Second, their framework treats process-tracing case studies as an adjunct method with no real inferential added value. But why use the term ‘process-tracing’ if one is not intending to trace something, i.e. a mechanism? The term ‘causal process observation’ tells us it is within-case evidence, but it sheds no light on what process the empirical observation is actually evidence of. And when we are not told explicitly what empirical material is evidence of, it is difficult to evaluate its probative value. Therefore, their framework leaves us in the dark about how things work, thereby black-boxing the causal mechanisms that are of intense interest to many case study scholars. In this respect, multi-method research only becomes possible when we downplay the very reason why we wanted to engage in within-case research in the first place.
Seawright: multi-method research
Seawright’s Reference Seawright2016 book develops the most sophisticated framework for multi-method research to date within the variance-based approach. His framework is explicitly based on counterfactual causality in the form of a potential outcome framework. At its core, the framework is focused on mean causal effects across populations, assessed ideally with experimental designs, meaning it sits squarely within the variance-based approach. At the same time, he contends that cross-case analysis and case studies answer different research questions, meaning he is talking about method integration and not triangulation (Seawright Reference Seawright2016: 4–10). In the book, many different potential uses of case studies are discussed (e.g. dealing with potential measurement issues), but here I focus on the applications relating to combining case studies and large-n regression analysis for making causal inferences.
As with other variance-based approaches, the core analysis is done at the population level, investigating the difference that causes make across cases, i.e. mean causal effects. This can be undertaken using either experiments, natural experiments, or large-n observational data. Seawright is very careful in flagging the importance of unit homogeneity as a key assumption that has to be fulfilled for valid causal inferences. This is of course not difficult to achieve in an experiment through the randomized selection of a large number of units, but experiments have the problem of whether the studied population matches other populations (Seawright Reference Seawright2016: 166–169). Natural experiments assume unit homogeneity and independence, but the validity of the independence assumptions in particular can be problematic. Seawright suggests that the solution to this is to test using case studies whether there are assignment effects that could bias estimates of mean causal effects (Seawright Reference Seawright2016: 125, 164–166). Even more problematic regarding making causal claims are simple observational studies, which he states can only be used to make causal inferences if all confounding pathways and control variables are included in a model (Seawright Reference Seawright2016: 38), which he views as an unrealistic situation.
Case studies as they relate to making causal inferences are viewed as tools for discovering confounding variables (Z) (i.e. causal heterogeneity) and for exploring pathways linking causes and outcomes together that can make us more confident about a causal link as regards non-experimental estimates of mean causal effects (Seawright Reference Seawright2016: 45–74). He first suggests that deviant cases can be used to find potential confounders by exploring the reasons for causal heterogeneity. Once a confounding variable is found that produces the heterogeneity, he suggests that one should then group cases into smaller, homogeneous subsets depending on scores on the confounder. Tracing causal pathways can also be used to explore whether there are unknown confounders lurking within regression estimates of mean causal effects, using causal process observations (CPOs) to explore whether there is a direct link between a cause and outcome.
For both purposes, the framework is relatively silent on how much knowledge about a population can be gained from studying single cases. In his book, Seawright discusses a regression analysis that finds that globalization produces consensus on economic issues, where overall level of economic inequality is used as a control variable. He compares this regression-based study to a case study of Turkey, in which it is found that globalization increased inequality, suggesting that it is not a control variable but part of the causal model, at least for the Turkish case. Seawright suggests that this information should lead the authors of the original regression either to present evidence that inequality is not produced in other countries (i.e. it can still act as a control variable), to re-estimate causal models without economic inequality as a control, or to present evidence for why the case study analysis of the Turkish case is flawed. However, the key methodological problem here is that we are left in the dark about what we should actually do based on Seawright’s framework, and we are unable to answer how many cases we would require to re-evaluate a mean causal effect. This does not mean that Seawright’s framework is wrong, but that it has just scratched the surface of these important questions.
Finally, Seawright does not see mechanisms as anything more than intervening variables, meaning there is not significant additional knowledge about causal relationships that can be gathered by tracing mechanisms in depth. This means that – in the end – case studies always act as adjunct methods for increasing our confidence in mean causal effects across populations. How causal effects actually work within cases is therefore left firmly within an analytical black box, thereby also downplaying the contribution that this type of knowledge can bring to the table.Footnote 9
Goertz: an integrated approach
Goertz’s integrated approach comes the closest of the works assessed here to being case-based. However, as will be explained in the following, the work ends up black-boxing mechanisms, meaning that we learn little about the actual processes at work within cases. Instead, Goertz claims that studying mechanisms – often using counterfactual hypotheticals – makes us more confident about the overall causal effect of X on Y.
Goertz proposes a research triad focused on studying causal mechanisms, using both case studies (process-tracing and counterfactual analysis) and cross-case analysis (including experimental and observational large-n analysis, or QCA). He suggests that analysis should start by mapping a population of cases within which a particular mechanism might be at work, although this is framed in terms of X and Y. Case studies are then conducted on three types of cases: cases where the mechanism can be present (X=1, Y=1), those where it should be but is not (X=1, Y=0) and equifinality cases (X=0, Y=1), where other causes and mechanisms are at play. Cross-case analysis enables generalizations to be made about X and Y, although Goertz’s framework suffers from many of the same problems as other recent attempts at multi-method research: how do we move from studied cases to a broader population and vice versa? However, he does put forward an innovative solution here, suggesting a combination of intensive analysis of a few cases and more cursory case studies of a larger number of cases in order to be more confident that there is no lurking causal heterogeneity within a larger population of cases.
However, mechanisms remain in an analytical black box in Goertz’s work. By keeping theorized mechanisms at a very high level of abstraction, it is not difficult to move relatively seamlessly back and forth from populations to individual cases. For instance, he suggests that Stephan Haggard and Robert Kaufman (Reference Haggard and Kaufman2016) theorize a mechanism that links repressive autocratic regimes and economic grievances (causes) with democratic transition (outcome) that can be present in a relatively large number of cases. In their book, Haggard and Kaufman (Reference Haggard and Kaufman2016: 128) describe the mechanism linking as being ‘credible and sustained mass mobilization’. They suggest (Haggard and Kaufman Reference Haggard and Kaufman2016: 110) that the same mobilization mechanism was present in cases as disparate as Argentina and Bolivia, Congo and Niger, and Poland; a claim only possible if the mechanism is theorized at such a high level of abstraction that it tells us nothing about what is really going on in the cases.Footnote 10 This means that the actual process remains firmly within a black box, preventing us from claiming that we have actually traced empirically how a process works in a given case.
Conclusion: accepting two evidential hierarchies in comparative politics
Given that case-based and variance-based research ask fundamentally different questions and study them using very different types of evidence, it is a mission impossible to try to reconcile them into a seamless methodological framework where the two complement each other’s weaknesses when engaging in comparative politics research. Recent developments in the philosophy of science suggest that we should accept these differences, acknowledging that there are two ‘gold standards’: in-depth within-case tracing of mechanisms using mechanistic evidence, and random controlled experiments.
However, this puts the study of comparative politics in an uncomfortable position. If experiments are a gold standard, given that many research questions in comparative politics deal with macro-level phenomena that occur at the country level – in which experimental manipulation is impossible – are we stuck with only making correlational claims? If within-case tracing of mechanisms is a gold standard, what role is there for comparisons?
A first consequence of this difference should be that scholars of comparative politics are more explicit in defining what they are comparing. Is it patterns of difference-making of causes across cases? Or is it comparing the processes operative within cases, and understanding the conditions under which particular processes are triggered? Acknowledging differences instead of trying to paper over them is the first step in a more productive debate about how to conduct research in comparative politics.
The next step is for scholars of comparative politics to develop stronger methodological tools within the two approaches for engaging in cumulative research. In variance-based approaches, one productive way forward would be to seek inspiration in developments in systems biology and personalized medicine. Scholars should drop the search for single-cause universal explanations by exploring mean causal effects across large numbers of very diverse cases. Instead, a more productive research programme would entail attempting to understand how causes and contexts interact with each other within sets of more causally homogeneous cases. This can involve some form of cluster analysis first, followed by a theoretical probing within the identified clusters to figure out the effects of causes and how they interact.
Cumulative research in a case-based approach deals with learning about how things work in particular contexts (i.e. mechanisms). Unfortunately, there is little guidance in the natural science literature for how to extrapolate mechanistic findings from individual cases to learn whether what ‘works here’ also ‘works there’. Case-based comparativists should therefore attempt to move beyond designs that are, in essence, often merely single case studies or that treat mechanisms as ‘one-liners’ that tell us nothing about how a process worked in any given case. Instead, they should strive to develop better methodological tools that would enable cumulative, mechanism-focused research programmes.