Introduction
Worldwide, bladder cancer accounted for 573 278 new cases and 212 536 deaths in 2020 (Ref. Reference Sung1). Bladder cancer can present as non-muscle-invasive bladder cancer or muscle-invasive bladder cancer based on its invasion depth.
In addition to its high incidence, the clinical outcomes of bladder cancer remain highly unsatisfactory. Patients with advanced bladder cancers often develop metastases and resistance to chemotherapy. Advances in treatment will thus depend on an improved understanding of the underlying biology, especially the characterisation of bladder cancer with high-resolution technologies. In recent years, large-scale sequencing projects and gene expression studies have provided molecular insights into cancers, including frequently mutated genes, aberrantly regulated programmes, differentially expressed genes and molecular typing systems based on gene expression profiles (Refs Reference McGranahan and Swanton2–Reference Choi4). However, these data reflect mixed signals of bulk tissues, and often, fail to capture the diversity of individual cells and cellular subpopulations. Thus, it is essential to develop high-resolution strategies that can effectively dissect and systematically analyse tumour heterogeneity. Two pioneering studies formed the technical foundations for single-cell sequencing (SCS): (a) exponential amplification of the cDNA of single hematopoietic cells and (b) T7 RNA polymerase for in vitro transcription and linear amplification of cDNA (Ref. Reference Eberwine5). Recent advances in molecular biology, microfluidics and nanotechnology have accelerated the development of SCS technologies (Ref. Reference Stuart and Satija6) and revolutionised our understanding of cancer evolution (Refs Reference Gao7–Reference Casasent10), the tumour microenvironment (TME) (Refs Reference Li11–Reference Lambrechts14) and tumour heterogeneity (Refs Reference Carter15–Reference Chen17) and will continue to lead to new discoveries. The quick evolution of sequencing approaches has led to new discoveries in tumorigenesis, tumour metastasis, therapeutic resistance and precision medicine.
In this review, we will describe in brief single-cell technologies, summarise its applications in human cancers and bladder cancer, and propose major avenues for future investigation, with a focus on how this technology may influence clinical practice.
Single-cell DNA sequencing
In 2011, the first single-cell DNA sequencing (scDNA-seq) was reported by Navin to study the genetic heterogeneity of cancer and the evolution of tumorigenesis (Ref. Reference Navin18). The amount of DNA in a single cell (about 6 picogram) was not sufficient for next-generation sequencing, so whole-genome amplification (WGA) is always required to amplify DNA (Refs Reference Van Loo and Voet19, Reference Zhang20). Recently, alternative WGA technologies having degenerate oligonucleotide-primed-PCR (Ref. Reference Blagodatskikh21), multiple displacement amplification (Refs Reference Blagodatskikh21, Reference Xu22) and multiple annealing and looping-based amplification cycles (MALBAC) (Ref. Reference Zhang23). Among them, MALBAC can achieve low amplification bias with higher genome coverage during whole-genome sequencing. Currently, the most common applications of scDNA-seq are copy-number aberrations (CNAs) profiling and mutation detection. CNAs play an important role in moulding the genomes of cancers and has been proved to be useful in cancer prognostic prediction and therapeutic management. Utilizing scDNA-seq, one group was able to decipher the genetic heterogeneity within a breast tumour (Ref. Reference Baslan24). Their data highlighted the power of single-cell genomics in dissecting intra-tumoural genetic heterogeneity of CNAs, and the magnitude with which CNAs heterogeneity affects the genomes of breast cancers. Although next-generation sequencing (NGS) studies of breast cancer have identified many prevalent mutations, it is still unable to give us insights into the genomic diversity within tumours. Another group developed a whole-genome and exome SCS approach called nuc-seq (Ref. Reference Wang25) to sequence single normal and tumour nuclei from an oestrogen-receptor-positive (ER1) breast cancer and a triple-negative ductal carcinoma. Their data show that aneuploid rearrangements occurred early in tumour evolution and remained highly stable as the tumour masses clonally expanded. In contrast, point mutations evolved gradually, generating extensive clonal diversity. Despite these achievements,there are several challenges for scDNA-seq. For CNAs profiling, commercial platforms have achieved high-throughput cell sorting and processing, but they are challenged by lower data quality and limited genomic resolution. High-quality copy-number data at single-molecule resolution can be realised by using tagmentation chemistry methods but have modest throughput. In addition, mutation detection requires a higher coverage depth of genes of interest, but only a small number of cells can be profiled due to high costs. How to improve the throughput of scDNA-seq while reducing the cost remains to be a big challenge. In addition, technologies for unbiased WGA and the lower allelic dropout rates must be developed.
Single-cell RNA sequencing
Single-cell transcriptome sequencing, often referred to as single-cell RNA sequencing (scRNA-seq) was first reported by Tang et al. in 2009 (Ref. Reference Tang26). Recent commercialisation of SCS technologies has provided stable platforms that can be applied to cancer research and clinical applications, making it the most widely applied SCS approach. Single-cell mRNA sequencing not only reveals the quantitative and expression profile, but also exon splicing and allele-specific expression (Refs Reference Zhang20, Reference Marinov27, Reference Borel28). The structure of complex tissues is tightly linked with its function, thus determining the frequency and identity of cell types is crucial. A major advantage of scRNA-seq over conventional RNA-seq is the ability to perform cell-type-specific differential expression analysis,and the identified subpopulations can be further characterised to known cell types.
Additionally, based on calculation and simulation date from scRNA-seq, cell trajectories and pseudo time can be reconstructed in order to infer the state transition between cancer cells (Ref. Reference Chen, Ning and Shi29). Understanding the nature of the process and possible intermediate states can lead to the identification of key genes that act as switches and drivers of these processes. ScRNA-seq has facilitated the mapping of the landscape of tumour cells, and the inference of cellular interactions between malignant cells and stromal cells (Ref. Reference Chung30). The analysis of abnormal expression stares of malignant cells can provide a basis for precise targeted therapy (Ref. Reference Izar31). In addition, scRNA-seq of infiltrating immune cells provides new methods for patient stratification, helps to understand the function of tumour-infiltrating immune cells (Ref. Reference Zheng32).
It is however worth noting that due to limitations, scRNA-seq can only manage a small portion of the transcriptomes, with a bias for more highly expressed genes. In addition, scRNA-seq is very sensitive to sample quality and is not suited for the profiling of sub-optimally preserved or handled clinical specimen. Moreover, high costs limit the ability to profile large cohorts of tumours, and only a few to a few dozens of samples are typically profiled in each study. Although scRNA-seq has the highest throughput of single-cell methods, only a small number of cells can be measured.
Recent advances in single-cell technologies
Isolation of single cells is the first and crucial step in SCS. Early cell isolation technologies rely on manual or semi-automatic methods, such as micro-pipetting, fluorescence-activated cell sorting (FACS) and laser capture microdissection (LCM). The number of cells that can be isolated continues to increase with the emergence of new technologies. Now, microfluidic separation platform has become the mainstream methodologies, and the number of cells that can be obtained at a time has reached thousands or tens of thousands.
Multiple platforms have been established for automatic single-cell sorting and have significantly improved the efficiency of single-cell separation (Table 1). The Fluidigm C1 (Ref. Reference Brouzes33) first achieved this goal with a microfluidic chip that allows single-cell isolation, phenotyping and genomic profiling. This achievement inspired the invention of other platforms, such as the 10× Genomics Chromium, Rhapsody™ system from Bio-Rad and SureCell system from Illumina. These emerging commercial platforms have boosted sequencing efficiency to new levels. Efforts have also been made to reduce sequencing costs, such as Seq-well (Ref. Reference Gierahn34) and SPLit-seq (Ref. Reference Rosenberg35). Additionally, novel platforms, including high-density FACS systems (Ref. Reference Baslan36), nanowell (Ref. Reference Laks37) and combinatorial indexing systems (Refs Reference Yin38, Reference Vitak39) have also been developed with higher flexibility and lower costs.
Table 1. Isolation methods of single-cell sequencing

Single-cell genome and transcriptome studies have emerged as powerful methods for investigating the cancer mechanisms, and more single-cell technologies have been developed to explore the disease, including DNase-seq (Ref. Reference Jin46) and ATAC-seq (Refs Reference Buenrostro47, Reference Cusanovich48) for measuring regions of open chromatin, Hi-C for exploring chromosomal contacts (Ref. Reference Ramani49), ChIP-seq for histone position mapping (Ref. Reference Rotem50), single-cell bisulphite sequencing (Ref. Reference Smallwood51) and bisulphite-free (single-cell CGI-seq) sequencing (Ref. Reference Han52) for DNA methylation analysis. Simultaneous analysis at multiple regulatory levels in the same cell is another important field in SCS (Table 2). It provides an opportunity to comprehensively understand the phenotypes and mechanisms of malignant cells. Combinatorial analysis methods are available for the analysis of the genome and transcriptome (G&T-seq and DR-seq) (Refs Reference Macaulay53–Reference Macaulay55), epigenome and transcriptome (scMT-seq, scTrio-seq and scNMT-seq) (Refs Reference Angermueller56–Reference Hou58). Moreover, single-cell COOL-seq (Ref. Reference Gu59) enables the simultaneous analysis of chromatin states, CNAs and DNA methylation states.
Table 2. Single-cell multi-omics technologies

Accompanying the tremendous progress in SCS technologies are specialised algorithms developed to best interpret single-cell data. For example, the imputation of dropout events (Refs Reference Gong68, Reference Lin, Troup and Ho69), normalisation and correction of batch effects (Refs Reference Haghverdi70–Reference Bacher74), clustering cell types and identification (Refs Reference Butler71, Reference Kiselev75), pseudo-temporal trajectory inference (Refs Reference Ji and Ji76, Reference Setty77) and data visualisation (Refs Reference Ding, Condon and Shah78–Reference Levine80).
The tissue dissociation steps of SCS inevitably lead to the loss of information about the original cell locations and cellular interactions in the tumour tissue. How to retain spatial information remains a key question in the characterisation of tumour heterogeneity. The brute-force approach to capture spatial information of gene expression consists of the direct isolation of regions of interest, which are then placed in separate tubes for RNA extraction and subsequent gene expression profiling. Examples of this approach are LCM, as well as Geo-Seq (Ref. Reference Chen81) and Tomo-seq (Ref. Reference Junker82). However, these methods are often labour-intensive and difficult to use to cover large areas, so it is not feasible to obtain a complete picture of the specimens. Instead of collecting molecules from individual tissue sites (or cells), in situ hybridisation (ISH) can be used to directly visualise molecules of interest in the original environment through fluorescent labelling, such as smFISH (Ref. Reference Raj83), seqFISH (Refs Reference Lubeck84, Reference Shah85), MERFIS (Ref. Reference Chen86), or hybridizing and sequencing methods, including TIVA (Ref. Reference Lovatt87) and FISSEQ (Ref. Reference Lovatt87). However, these methods are limited by sequencing throughput or cell targets that can be measured. The ground-breaking technology of spatial transcriptomes realised the localisation of high-throughput tissue transcriptome information for the first time. Ståhl et al. (Ref. Reference Ståhl88) used a specially designed chip to retain the cell location information and applied NGS technology to achieve the perfect combination of ISH and high-throughput sequencing. Their endeavour generated a complete in situ gene expression image in the tissue. The development of SCS has remarkably promoted research on single-layer cell technology. Unlike the 10× Visium, which attaches the reverse transcription primers on a glass slide, Slide-Seq (Ref. Reference Rodriques89) achieves a higher resolution by using a ‘disc’ consisted of barcoded microbeads, and the newly improved Slide-Seq2 (Ref. Reference Stickels90) enables an RNA capture efficiency of up to 50% (approximately 10 times higher than that of Slide-Seq). The further developments in single-cell space technologies will greatly improve our understanding of cancer biology, and by integrating single-cell resolution genomic data with morphological features, it is expected to transform clinical pathology diagnostic models in near future.
Application of single-cell sequencing in cancer research
Bulk genomic analyses and expression profiling of clinical specimens have led to a preliminary understanding of cancer. Although we have gained some knowledge of genetic heterogeneity and evolutionary principles, we are still lacking knowledge of the molecular details behind complex phenomena in cancer. Single-cell genomic techniques have emerged as powerful approaches to dissect human tumours at the resolution of individual cells, providing a compelling approach to deciphering cancer biology. With such advantages, state-of-the-art technologies have been broadly employed for the study of cancer biology in several themes (Fig. 1).
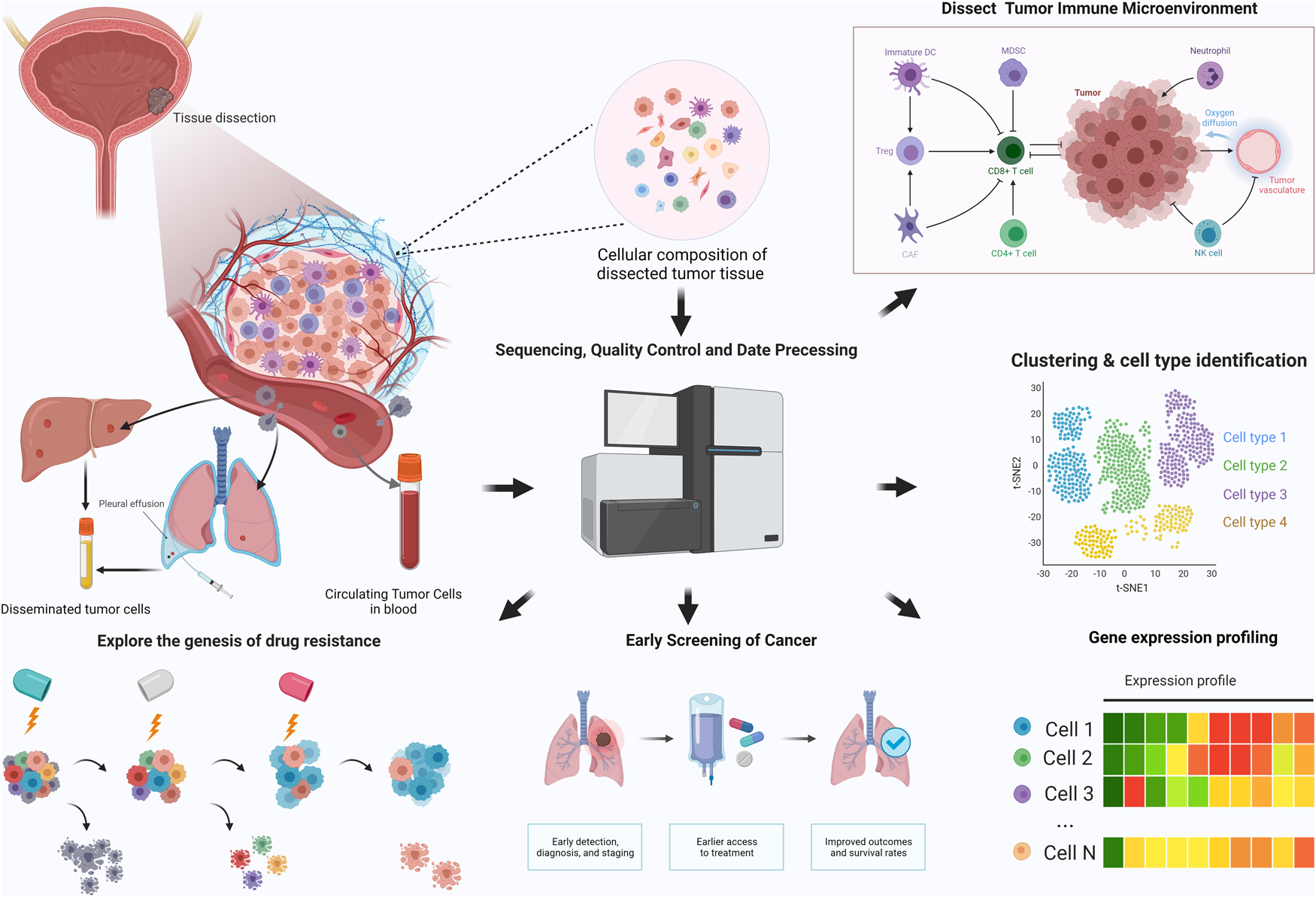
Fig. 1. Application of single-cell sequencing in cancer research.
Understanding the complex tumour heterogeneity
Cancer is characterised by its heterogeneity at both inter- and intra-tumour levels, and poses great challenges for precision medical therapy. There are several determinants of tumour heterogeneity: (1) genetic heterogeneity – during tumour evolution, accumulation of stochastic mutations resulting in increasing genetic diversity eventually leads to the coexistence of multiple sub-clones; (2) epigenetic effects – cells in the TME are often in different cellular states and thus show distinct functions; (3) spatial factors and different spatial sites have different cell compositions within the tumour, resulting in spatial heterogeneity; and (4) external factors, such as oxygen supply and nutrient availability. These factors work together to create a complex system with multiple layers of heterogeneity. Tumour heterogeneity determines tumour behaviour, including growth, metastasis, drug sensitivity and patient survival. Therefore, comprehensive characterisation of tumour heterogeneity is essential for tumour studies. By analysing the expression profiles of each cell and subclone, we can study the biological properties of tumour-initiating cells, the functions of different tumour cell subpopulations in the complex microenvironment, and the collaborative interactions among tumour cell subpopulations.
Characterization of the tumour immune microenvironment
The TME is a collection of various cell types and biochemical molecules that support the survival and expansion of tumour cells. The interactions between tumour cells, stromal cells and immune cells also play pivotal roles in regulating tumour growth, immune surveillance and metastasis. In the TME, fibroblasts can be reprogrammed into carcinoma-associated fibroblasts (CAFs) and have been shown to promote or suppress tumour growth by interacting with tumour cells (Ref. Reference Kalluri91). ScRNA-seq studies have revealed the different functions of heterogeneous CAF subtypes, such as mechanism construction, angiogenesis and immune regulation (Refs Reference Lambrechts14, Reference Bartoschek92, Reference Elyada93). Due to the growing use of immunotherapy in solid tumours, TME has received intense attention. Recently, detailed characterisation of T cells with scRNA-seq has revealed that decreased activation of T cells and increased T-cell exhaustion signatures are associated with the progression of several human cancers, and a suppressive immune microenvironment is associated with worse prognosis (Refs Reference Guo94–Reference Savas96), and tissue-resident memory T cells with higher cytotoxic activity are associated with better prognosis in triple-negative breast cancer (TNBC) patients (Ref. Reference Savas96). Analysis of tumour-associated macrophages has identified novel macrophage subtypes that have an expression profile associated with cell migration (Ref. Reference Zhang97) that are distinct from the traditional M1 (inflammatory) or M2 (tumour-promoting) subtypes. Single-cell technologies provide a more comprehensive insight into the diverse ecosystem of cancer and help us to understand the dynamic changes in the TME in immunotherapy.
Decoding the genesis of therapy resistance
Chemotherapy is still one of the preferred therapies for many malignant tumours; however, drug resistance is a major obstacle in the treatment of cancers. The idea behind chemotherapy is to kill cancer cells by taking advantage of their ability to differentiate faster than benign cells. However, small pockets of cancer cells can withstand their assault, allowing cancer to eventually return. A similar situation can be found in targeted therapies. Targeting therapy based on the discovery of genes altered by somatic mutations, functional characterisation and biochemical activities of malignant tumours has been widely applied. Unfortunately, the breadth and rapidity with which relapse and hold have been observed have surprised cancer biologists and clinicians (Refs Reference Lito, Rosen and Solit98, Reference Ma99). The tumour ecosystem consists of heterogeneous tumour cell populations. Successful tumour therapy requires complete elimination of genetically and phenotypically different subpopulations. Otherwise, even small surviving subpopulations may cause repopulation and refractory tumours. SCS technologies allow for a better profiling of the TME and provide the basis for more successful tumour treatments. The mechanisms underlying drug resistance have been revealed through the SCS of drug-resistant solid tumours. Whether drug resistance originates from pre-existing subclones with drug-resistant phenotypes or is induced by chemotherapy itself remains debated. In prostate cancer, single-cell transcriptome analysis of CTCs revealed activation of non-canonical Wnt signalling associated with resistance to androgen receptor inhibitors (Ref. Reference Miyamoto100). Shaffer et al. (Ref. Reference Shaffer101) investigated a BRAF inhibitor-treated melanoma cell line using scRNA-seq. A rare drug-resistant cell state was found prior to BRAF inhibitor treatment, which requires further genetic reprogramming and loss of SOX10 to achieve complete drug resistance. In another study of oral cancer cell lines (Ref. Reference Sharma102), scRNA-seq analysis revealed that heterogeneous phenotypes favoured selection of existing clones, while homogenous populations could be reprogrammed by increasing Sox9 expression and losing Sox2. ScDNA-seq can determine whether drug-resistant clones in tumour masses are present and selected after chemotherapy or alternatively induced by drug-resistant mutations during treatment. In another study of chemotherapy-resistant TNBC, combination analysis using scDNA-seq and scRNA-seq showed that drug-resistant genotypes were present in the tumour mass prior to chemotherapy. Further transcriptional reprogramming results in a fully resistant phenotype (Ref. Reference Kim103). In addition to intrinsic drug resistance, tumour cells exhibit different changes in response to chemotherapeutic drug-induced damage. Park SR et al. (Ref. Reference Park104) characterised the transcriptome response of cultured colon cancer cell lines to 5-fluorouracil (5FU)-induced DNA damage. After 5FU treatment, a single population of colon cancer cells adopted three distinct transcriptome phenotypes, with many genes showing group-specific expression patterns mediating DNA damage responses specific to the corresponding cell fate.
Single-cell sequencing in clinical management
Although most SCS studies have been research-focused, the development and maturation of SCS technologies provide new opportunities for clinical management. Early detection is paramount in high-risk groups because interventions can limit malignant diseases to early stages and improve clinical outcomes. In fact, the presence of disseminated tumour cells (DTCs) can be detected in many body fluids, such as urine of bladder and prostate cancer (Ref. Reference Nawroth, Weckermann and Retz105), peritoneal washing fluids of ovarian cancer and pancreatic cancer (Refs Reference Yamada106, Reference Naz107). In studies of multiple myeloma patients and healthy controls, researchers have identified the expression programme of rare aggressive plasma cells in several myeloma patients (Ref. Reference Ledergor108).
Circulating tumour cells (CTCs) are a population of cells shed from a primary or metastatic tumour into the peripheral blood and lymph (Ref. Reference Pantel, Alix-Panabières and Riethdorf109), and are also responsible for the progression of tumour metastases. They are regarded as important supplementary indicators in TNM tumour staging, prognosis assessment and tumour recurrence prediction. Single-cell approaches can identify the TME in primary and metastatic tumours and link the two ends via CTC, providing insight into the mechanism of metastasis. Thus, single-cell technology has become essential for investigating these cells. Puram et al. identified invasive gene expression programmes in tumour cells in head and neck carcinoma (Ref. Reference Puram12) that promoted tumour metastasis. In a recent study (Ref. Reference Sun110), CTCs from four different vascular sites were collected from patients with hepatocellular carcinoma (HCC). The transcriptomic landscape of CTCs reveals significant intra-vascular and inter-vascular heterogeneity between CTCs from different collection sites. In addition, CCL5 was found to be an important mediator of CTC immune escape, this may aid in new anti-metastasis therapeutic strategies in HCC. Accordingly, the integration of early screening and SCS technologies is expected to revolutionise the diagnosis of malignant tumours.
The diagnosis of tumours mainly depends on pathological evaluation, but it can be interfered with by many factors, such as micro-invasion, and tissue loss due to incomplete resection. The SCS method has demonstrated its high efficiency in the profiling of premalignant cells (Refs Reference Casasent10, Reference Barros-Silva111, Reference Bernard112). In invasive prostate cancer, SCS analysis uncovered that invasive clonal populations are well correlated with Gleason score, thus guiding surgical decisions (Ref. Reference Alexander113). Bian et al. suggested that SCS typing of colorectal cancer can be used to determine the optimum treatment options for individual patients (Ref. Reference Bian114). Moreover, SCS can uncover novel drug targets in primary, metastatic, especial in therapy-resistant disease. Hong et al. revealed serial adaptive changes by transcriptomic reprogramming and copy number changes in patients undergoing endocrine therapy (Ref. Reference Hong115). Methods for scRNA-seq and single-cell Assay for Transposase Accessible Chromatin with high-throughput sequencing (scATAC-seq) can be used to identify drug targets of stroma or immune cells that contribute to tumour metastasis and therapeutic resistance (Refs Reference Bian114–Reference Azizi116). Single-cell technologies have revolutionised cancer biology and with the developments of more SCS methods, the throughput will be higher, and sequencing accuracy and sensitivity will improve. We expect SCS technologies to accelerate the development of better diagnostic and prognostic biomarkers, lead to improved therapeutic efficacy and avoid tumour drug resistance.
Application of single-cell sequencing in bladder cancer
In recent years, the application of SCS in the field of bladder cancer has also improved our understanding of bladder cancer (see Fig. 2). Yu et al. (Ref. Reference Yu117) created a single-cell transcriptomic map of the human and mouse bladder and identified two novel types of human bladder cells that could be associated with nerve conduction and bladder emptying. The origin of bladder cancer and driver genes has always been the focus of frontier research. In a muscle-infiltrating transitional cell carcinoma of the bladder, four non-silent mutant alleles, including CFTR, NIPBL, ASTN1 and DHX57, were identified by analysing the spectrum and clonal structure of somatic mutant alleles, which may play a key role in the maintenance of ancestral clones and the muscle invasion ability of subclones (Ref. Reference Li118). Phylogenetic analysis of bladder cancer (Ref. Reference Yang119) revealed that bladder cancer stem cells may originate from bladder cancer non-stem cells or bladder epithelial stem cells. It was also found that co-mutations in ARIDA1, GPRC5A and MLL2 can enhance the stemness of bladder cancer. Chemotherapeutic drug resistance and tumour metastasis are the main causes of death in patients with advanced bladder cancer. It may be possible to overcome drug resistance by developing a personalised approach against relapsing cancers based on a comprehensive analysis of cell type-specific transcriptomic variations during the clinical course of the disease using scRNA-seq. By mapping the transcriptome of cancers treated with platinum-based chemotherapy using scRNA-seq, Tanaka et al. (Ref. Reference Tanaka120) uncovered a novel gene, COX7B, associated with platinum resistance, and found its surrogate marker, CD63. Low COX7B levels were significantly associated with poor response to chemotherapy in urinary bladder cancer. In a sequential clinical study, Lee et al. (Ref. Reference Lee121) used scRNA-seq to depict the tumour landscape of a chemo-resistant metastatic, muscle-invasive urothelial bladder cancer harbouring an activating HRAS mutation. They also applied scRNA-seq to the corresponding patient-derived xenograft (PDX) before and after treatment with tipifarnib, an HRAS-targeting agent. Although tipifarnib treatment was unable to achieve a complete response, comparative scRNA-seq analysis between pre- and post-tipifarnib-treated PDX revealed the nature of tipifarnib-refractory tumour cells and the tumour-supporting microenvironment. Accordingly, the pD-L1 inhibitor altezizumab was applied in patients to achieve a favourable response. Recently, an encouraging advance in the clinical treatment of bladder cancer has come from the advent of novel immunotherapy, but the responses to anti-PD-1 immunotherapy occur infrequently. In one study, scRNA sequencing and paired T-cell receptor sequencing were performed to identify differences in TME between tumour tissue and normal adjacent tissue (Ref. Reference Oh122). Surprisingly, there was no significant difference between CD8+ T cells in tumour tissues and control normal tissues, while CD4+ T cells showed several tumour-specific states, and these CD4+ T cells were suppressed by regulatory T cells. Multiple lineages of cytotoxic CD4+ T cells were clonally expanded. These CD4+ T cells can kill autologous tumours in an MHC class II-dependent pathway.

Fig. 2. Apllication of single-cell sequencing in bladder cancer.
Challenges and future directions
Single-cell technologies can resolve tumour heterogeneity with limited input materials, and therefore hold great potential in early detection, clinical diagnosis, non-invasive monitoring and new therapeutic target discovery. Early detection is critical in modern oncology since early interventions can significantly reduce morbidity and mortality. Early detection of cancers still relies heavily on imaging techniques or pathological analyses. DTCs can be detected in many body fluids near the origin of cancer, and these rare samples provide a unique opportunity for SCS technologies. In the early stages of cancer development, liquid biopsy can be used to isolate individual cancer cells, thus enabling early detection and evaluation of cancer in combination with single-cell technologies (Ref. Reference Russell123). Pathological diagnosis remains the primary method of cancer diagnosis. It relies on visual discrimination, which is susceptible to a variety of factors. SCS has been shown to be highly efficient in the analysis of precancerous cells in Dutal carcinoma in situ (Ref. Reference Casasent10), pancreatic precancerous (Ref. Reference Bernard112) and atypical adenomatous hyperplasia (Ref. Reference Allin124). In the future, as the cost of sequencing decreases, the efficacy of single-cell methods can be verified by clinical investigations, which may have a significant effect on tumour diagnosis. Additionally, in some cases where pathologic methods cannot provide a definitive diagnosis, single-cell technology has the potential to provide an adjunct or alternative diagnosis. Single-cell technologies provide a powerful tool for the unbiased discovery of novel actionable targets in primary, metastatic or drug-resistant malignant tumours. In androgen receptor inhibitor-resistant prostate cancer, scRNA-seq of CTC detected the activation of non-neoplastic Wnt signalling, providing a new therapeutic target for the treatment of advanced prostate cancer (Ref. Reference Miyamoto100). Kim et al. (Ref. Reference Kim125) found that sRNA-seq could characterise and predict the most aggressive tumour subsets in lung adenocarcinoma. Notably, when they compared the results of the single-cell approach with conventional sequencing, they found that the transcriptional profiles of the resistance subpopulations were often covered by the more prominent subpopulations, thus obscuring meaningful scientific findings. Thus, integrating SCS with traditional DNA sequencing may provide more optimised target selection and personalised therapeutic strategies.
Sequencing techniques for studying single-cell genomes, transcriptomes, epigenetic landscapes and chromatin accessibility have been established and optimised. Despite substantial advances, challenges still exist in SCS. The first challenge is the technological noise introduced during the amplification step. Notable allelic dropouts and non-uniform coverage would hamper the accurate detection of single nucleotide variants. Similarly, lowly expressed genes are likely to drop out and are susceptible to technological noise in single-cell transcriptome sequencing. In addition, the number of transcriptomes captured by single-cell transcriptome sequencing is unsatisfactory, which prevents us from understanding the full picture of the cellular transcriptional state. There is some plug-and-play software for the analytical process in SCS. While sequencing companies have developed ‘friendly’ software, the drawback is that they are somewhat of a ‘black box’, with a lack of transparency about the details and parameters of the precise algorithms used. In conclusion, it is necessary to understand the bioinformatics and computational issues involved in SCRNA-seq research; nonetheless, it would benefit clinicians to be supported by bio-informaticians.
Conclusion
SCS technology has been applied in various fields of research, especially in cancer research. It provides a robust tool to understand tumour heterogeneity at the single-cell resolution, so that we can divide cell clusters and identify rare cells. At the meantime, it provides a theoretical basis for early screening and individualised treatment strategies. Further advances and more insights will be made with the launching of Human Cell Atlas (HCA) project and Human Biomolecular Atlas Program (HuBMAP). However, the limitations of SCS technology should remain a concern. First, SCS tends to introduce noise in the step of nucleotide amplification, confounding the real biological variations. Second, the reliability and efficiency of cell separation still need improvement. In addition, most scRNA-seq methods are designed for 3’ or 5’ reads, and these methods, while having increased throughput, lack the ability to capture the full-length transcriptome. Third, batch effects may exist due to the use of different platforms and procedures. Single-cell sequencing technology is limited by its high cost, experiment time and less popular applications. Therefore, future development of single-cell sequencing technology should focus on reducing cost and improving accuracy, which will greatly promote the use of SCS. In addition, the application of SCS is limited by the high cost and long experimental time, as well as the need for professional technicians. While significant challenges remain, it is expected that the reliability, efficiency, sensitivity and accuracy of SCS will improve as related technologies evolve. In summary, we expect that the application of single-cell methods in cancer medicine will lead to significant improvements in the future.
Acknowledgements
This work was supported by the Improvement Project for Theranostic ability on Difficulty Miscellaneous disease (Tumor) and the Science & Technology Innovation Fostering Foundation of Zhongnan Hospital of Wuhan University (ZLYNXM202008,No. znpy2019050,znpy2019004, znpy2018027).
Author contributions
Fubing Wang, Wang Wei and Yuan Rong conceived the idea; Wang Wei wrote the initial draft of the paper, the rest of the authors were involved in writing and revising the paper.
Financial support
This work was also funded by the Medical Top-talented youth development project of Hubei Province by Medical talented youth development project in Health Commission of Hubei Province (No. WJ2019Q049). This work was supported by grants from the Cancer Research Program of the National Cancer Center (NCC201817B054). Thanks to the Department of Pathology, Zhongnan Hospital for the assistance of figure creation. All figures are created in BioRender, https://biorender.com/.
Conflict of interest
The authors declare that the research was conducted in the absence of any commercial or financial relationships that could be construed as a potential conflict of interest.