1 Introduction
The present study continues a line of research initiated in Szmrecsanyi et al. (Reference Szmrecsanyi, Grafmiller, Heller and Röthlisberger2016) which combines the main principles of the probabilistic grammar framework (e.g. Bresnan Reference Bresnan, Featherston and Sternfeld2007), which argues that grammatical knowledge has a probabilistic component shaped by speakers’ linguistic experience, with work on postcolonial varieties of English (e.g. Schneider Reference Schneider2007). Our overarching goal is to determine how similar or dissimilar the probabilistic knowledge of grammar is on the part of speakers with different regional backgrounds and to assess the extent to which the degree of probabilistic indigenization corresponds to an alternation's lexical specificity (as has been claimed in the literature). More specifically, we propose a corpus-based variationist method for quantifying the extent to which syntactic constraints that influence the choice between competing variants behave homogeneously across varieties of English and compare these results to the degree to which the variation between the competing variants depends on the lexical items that instantiate the constituents (i.e. the alternation's lexical specificity). As a case study, we discuss similarity patterns between three varieties of English around the world, namely British English (BrE), Indian English (IndE) and Singapore English (SgE), in four syntactic alternations that offer speakers a binary choice: the genitive alternation (e.g. Rosenbach Reference Rosenbach2014), as in (1); the dative alternation (e.g. Bresnan & Hay Reference Bresnan and Hay2008), illustrated in (2); particle placement (e.g. Gries Reference Gries2003), as in (3); and subject pronoun omission (e.g. Torres Cacoullos & Travis Reference Torres Cacoullos and Travis2014), exemplified in (4).

Previous research on probabilistic indigenization effects has largely focused on only three of these alternations (dative, genitive and particle) in a similarly small set of varieties (e.g. Szmrecsanyi et al. Reference Szmrecsanyi, Grafmiller, Heller and Röthlisberger2016) or has analyzed variable patterns in one alternation but across several varieties (e.g. Heller et al. Reference Heller, Szmrecsanyi and Grafmiller2017; Röthlisberger et al. Reference Röthlisberger, Grafmiller and Szmrecsanyi2017; Grafmiller & Szmrecsanyi Reference Grafmiller and Szmrecsanyi2018; Hundt et al. Reference Hundt, Röthlisberger and Seoaneto appear). While these studies provide various explanations for probabilistic indigenization effects – drawing on general cognitive processes of language acquisition, language contact and dialect drift – the degree to which the lexical items used in each variant might influence variant choice differently in the alternations has received little attention (but see Röthlisberger et al. Reference Röthlisberger, Grafmiller and Szmrecsanyi2017: 698–9). The variationist approach adopted here has previously been proposed in Grafmiller & Szmrecsanyi (Reference Grafmiller and Szmrecsanyi2018) and Szmrecsanyi et al. (Reference Szmrecsanyi, Grafmiller and RosseelMS) and is extended in the current study to four syntactic alternations, providing thus a more comprehensive view of morphosyntactic probabilistic indigenization effects than has hitherto been attempted. Overall, our findings suggest that the three varieties we examine share a common probabilistic grammar in all four alternations in that the constraints that influence the outcome of syntactic variation behave, for the most part, in a homogeneous manner across varieties. Probabilistic indigenization effects, however, can be observed to different degrees, largely depending on the lexical specificity of the alternation involved.
The rest of this article is structured as follows. Section 2 summarizes the main theoretical issues which the study addresses, with a focus on the connection between the emergence of cross-varietal probabilistic indigenization effects and the lexical specificity of syntactic alternations. In section 3, we describe the datasets and methods used. Section 4 deals with the results of the study, followed by a discussion of their implications in section 5. Finally, section 6 concludes with some final remarks and suggestions for future research.
2 Theoretical background
This article lies at the interface of two well-known research paradigms. On the one hand, it adheres to the probabilistic grammar framework in that it assumes that grammatical knowledge is partially probabilistic and that multiple constraints operate simultaneously, sometimes with opposite effects, on the alternation between competing variants (e.g. Bresnan Reference Bresnan, Featherston and Sternfeld2007; Bresnan et al. Reference Bresnan, Cueni, Nikitina, Harald Baayen, Bouma, Krämer and Zwarts2007; Bresnan & Hay Reference Bresnan and Hay2008; Bresnan & Ford Reference Bresnan and Ford2010). Research in that spirit has shown that speakers are able to predict, with high accuracy, the odds of finding a particular linguistic variant in a particular context. This, in turn, entails that speakers’ grammatical knowledge must necessarily include intuitions about the underlying probabilistic constraints governing linguistic behavior. Bresnan and colleagues further show that grammatical knowledge is gradient and subject to restructuration as a result of changes in speakers’ experience with language, which is at least partly dependent on their sociocultural environment. The present study combines this probabilistic viewpoint with an interest in the connection between the structural characteristics of varieties of English and their sociohistorical background, in the spirit of the World Englishes framework (e.g. Schneider Reference Schneider2007; Mesthrie & Bhatt Reference Mesthrie and Bhatt2008). Crucial to the structural characteristics of varieties of English is the concept of nativization or indigenization. Nativization or indigenization refers to the process whereby speakers of postcolonial varieties make English their own, expressing themselves by means of ‘locally characteristic linguistic patterns’ (Schneider Reference Schneider2007: 6). Indigenization processes have been claimed to exist mainly at the lexis–syntax interface (Schneider Reference Schneider2003: 249): rather than inventing novel syntactic patterns from scratch, these postcolonial varieties of English are characterized by innovative combinations of lexical items and existing syntactic constructions.
A growing body of literature has recently emerged from the incorporation of the principles of probabilistic grammar into the World Englishes paradigm with the aim of exploring and delimiting the extent to which the strength of probabilistic constraints fluctuates across varieties of English (e.g. Rosenbach Reference Rosenbach2002, Reference Rosenbach, Rohdenburg and Mondorf2003; Hinrichs & Szmrecsanyi Reference Hinrichs and Szmrecsanyi2007; Bresnan & Hay Reference Bresnan and Hay2008; Szmrecsanyi & Hinrichs Reference Szmrecsanyi, Hinrichs, Nevalainen, Taavisainen, Pahta and Korhonen2008; Bresnan & Ford Reference Bresnan and Ford2010; Bernaisch et al. Reference Bernaisch, Gries and Mukherjee2014; Szmrecsanyi et al. Reference Szmrecsanyi, Grafmiller, Heller and Röthlisberger2016; Heller et al. Reference Heller, Szmrecsanyi and Grafmiller2017; Röthlisberger et al. Reference Röthlisberger, Grafmiller and Szmrecsanyi2017; Szmrecsanyi et al. Reference Szmrecsanyi, Grafmiller, Bresnan, Rosenbach, Tagliamonte and Todd2017; among others). Common to most of these studies is the observation that varieties share a fairly robust probabilistic grammar in that the constraints affecting a particular syntactic phenomenon are largely stable across varieties and fuel the same kind of syntactic choices. However, gradient regional differences seem to exist with respect to the strength with which such constraints impact speakers’ constructional choices in each variety. For instance, American English (AmE) and BrE speakers differ in that speakers of AmE favor the s-genitive over the of-genitive more strongly with inanimate possessors and as the length of the possessum increases than BrE speakers (Hinrichs & Szmrecsanyi Reference Hinrichs and Szmrecsanyi2007; Szmrecsanyi & Hinrichs Reference Szmrecsanyi, Hinrichs, Nevalainen, Taavisainen, Pahta and Korhonen2008). Similarly, Bresnan & Hay (Reference Bresnan and Hay2008) report that the animacy of the recipient impacts the choice of dative variant more strongly in New Zealand English (NZE) than in AmE, with inanimate recipients being more likely in the ditransitive dative variant in the former than in the latter variety.
In order to refer to these gradient regional differences, Szmrecsanyi et al. (Reference Szmrecsanyi, Grafmiller, Heller and Röthlisberger2016: 133) extended the notion of indigenization from the World Englishes paradigm to the probabilistic domain and coined the term probabilistic indigenization, which they defined as ‘the process whereby stochastic patterns of internal linguistic variation are reshaped by shifting usage frequencies in speakers of post-colonial varieties’. Probabilistic indigenization thus refers to a linguistic process that leads to statistical differences across varieties in the effects of probabilistic constraints. Szmrecsanyi et al. (Reference Szmrecsanyi, Grafmiller, Heller and Röthlisberger2016) argue that divergences in the odds of finding a given syntactic variant in a given context across varieties, even if these patterns are not stable, are evidence of the existence of variety-specific grammars tied to unique sociolinguistic backgrounds. Comparing the effect of probabilistic constraints in three syntactic alternations – the genitive, dative and particle placement alternations – across four varieties (i.e. BrE, Canadian English (CanE), IndE and SgE), they show that the four varieties largely share a common probabilistic grammar, since the effect direction of constraints remains stable across varieties. Nonetheless, quantitative differences emerge with regard to the strength of these constraints. For instance, they observe that the influence of a directional prepositional phrase after the verb phrase on the particle placement alternation was weaker in IndE than in the other varieties they studied. Interestingly, the three alternations in Szmrecsanyi et al. (Reference Szmrecsanyi, Grafmiller, Heller and Röthlisberger2016) turned out not to be equally sensitive to probabilistic indigenization effects, with the particle placement alternation exhibiting stronger variety-specific patterns than the dative and genitive alternations. Szmrecsanyi et al. associate this difference in sensitivity with the lexical specificity of the alternation in question: they conclude their study by suggesting that probabilistic indigenization effects arise as a function of the lexical specificity of syntactic alternations, with those that are strongly connected to specific lexical items being the ones most likely to exhibit cross-varietal indigenization effects. Their argumentation finds support in previous work in World Englishes that has shown that cross-varietal differences mainly emerge at the lexis–syntax interface. Similar tendencies were found by Szmrecsanyi et al. (Reference Szmrecsanyi, Grafmiller, Bresnan, Rosenbach, Tagliamonte and Todd2017), a study of dative and genitive variation in spoken language in four native varieties of English, namely AmE, BrE, CanE and NZE. Their data also shows that syntactic alternations are not equally homogeneous across varieties, with the dative alternation displaying stronger variety effects than the genitive alternation.
In the present article, we extend our previous knowledge of the probabilistic grammars of varieties of English in two ways. First, we propose a corpus-based variationist method for calculating the extent to which syntactic alternations display probabilistic indigenization effects: what counts is not if and/or how often people use particular constructions, but how – that is, subject to which probabilistic constraints – they choose between ‘alternate ways of saying “the same” thing’ (Labov Reference Labov1972: 188). Our approach is inspired by the Variation-Based Distance and Similarity (VADIS) method proposed in Szmrecsanyi et al. (Reference Szmrecsanyi, Grafmiller and RosseelMS), which assesses the degree of alternation-internal homogeneity across varieties of English along three lines of evidence, as proposed in the comparative sociolinguistics literature (see Poplack & Tagliamonte Reference Poplack and Tagliamonte2001: 92; Tagliamonte Reference Tagliamonte, Chambers and Schilling2002: 731):
1. Statistical significance: Do the same constraints have a statistically significant effect across varieties?
2. Size and direction: Are probabilistic constraints similar with respect to the size and direction of their effects? Are there any constraints that have, e.g. a stronger effect, or an effect in the opposite direction in one variety as compared to rest?
3. Constraint ranking: Is the overall ranking of constraints homogenous across varieties? In other words, do the constraints have the same relative importance in all the varieties considered?
Second, we quantitatively test the hypothesis in Szmrecsanyi et al. (Reference Szmrecsanyi, Grafmiller, Heller and Röthlisberger2016) according to which an alternation's degree of probabilistic indigenization is proportional to its lexical specificity, that is, the more lexically specific an alternation, the more indigenized it is.
3 Data and method
For the purposes of this article, we investigate variation in the choice of syntactic variants in three varieties of English, namely BrE, IndE and SgE.Footnote 2 BrE is an Inner Circle L1 variety, while IndE and SgE are Outer Circle L2 varieties which are considered to have reached phase 4, endonormative stabilization, in Schneider's (Reference Schneider2007) Dynamic Model. Despite their similarities regarding typological classification, IndE and SgE differ from each other in that the English in India has been described as being in a ‘steady state’ in which both progressive and conservative forces are at play (Mukherjee Reference Mukherjee2007: 158). Moreover, whereas the number of L1 speakers of SgE has been on the rise since the 1980s – in 2010 more than 32 percent of Singaporeans claimed that English was their dominant language (Leimgruber Reference Leimgruber2013: 9) – L1 users represent only about 0.25 percent of the total number of IndE speakers (Sharma Reference Sharma, Kortmann and Lunkenheimer2012: 523). Therefore, the set of varieties of English studied, despite being restricted in number, represents very different variety types, evolutionary stages, and even more fine-grained distinctions as regards the varieties’ social ecologies.
The data for the present study were extracted from the British (ICE-GB), Indian (ICE-IND) and Singaporean (ICE-SIN) components of the International Corpus of English (ICE). Each national component in ICE contains 500 texts, which amount to a total of approximately one million words: 600,000 words of spoken material and 400,000 of written material. A useful feature of ICE is that all its components follow the same design and annotation scheme, which makes them particularly appropriate for establishing comparisons between varieties. Interchangeable instances of the genitive, dative, particle placement, and subject pronoun omission alternations were retrieved as follows:
– In the case of the genitive alternation, being a relatively frequent syntactic phenomenon, 10 percent of the texts in ICE was enough for statistical analysis. A sample of texts containing text one, eleven, twenty-one and so on was created and then used to extract tokens of the genitive alternation in an automatic fashion. Appositive genitives, classifying genitives, double genitives, idiomatic genitives, partitive genitives and genitives involving indefinite possessums were excluded to make sure that both variants could have been used interchangeably (see Rosenbach Reference Rosenbach2002, Reference Rosenbach2014; Wolk et al. Reference Wolk, Bresnan, Rosenbach and Szmrecsanyi2013). This process yielded 3,108 genitive tokens (for further details on the genitive database, see Heller et al. Reference Heller, Szmrecsanyi and Grafmiller2017; Heller Reference Heller2018).
– Instances of the ditransitive and prepositional dative variants were retrieved using a list of dative verbs, shown in (5), adapted from previous literature (Levin Reference Levin1993; Mukherjee & Hoffmann Reference Mukherjee and Hoffmann2006; Bresnan et al. Reference Bresnan, Cueni, Nikitina, Harald Baayen, Bouma, Krämer and Zwarts2007; De Cuypere & Verbeke Reference De Cuypere and Verbeke2013; Wolk et al. Reference Wolk, Bresnan, Rosenbach and Szmrecsanyi2013). To ensure interchangeability, we excluded tokens involving particle verbs, passivized verbs, elliptical structures, coordinated verbs, clausal or non-overt constituents, beneficiary constructions, constructions containing a spatial goal and idiomatic expressions. In addition, extremely long recipients (more than 18 words) and themes (more than 23 words) were eliminated from the prepositional and ditransitive dative variants respectively. This rendered a database of 3,012 tokens of the dative alternation (for further details on the dative database, see Röthlisberger et al. Reference Röthlisberger, Grafmiller and Szmrecsanyi2017; Röthlisberger Reference Röthlisberger2018).
(5) accord, advise, allocate, allot, allow, answer, appoint, ask, assign, assure, award, bequeath, bid, bring, call, carry, cause, cede, charge, concede, convey, cost, deal, deliver, demonstrate, deny, develop, drop, entrust, explain, extend, feed, flick, flip, forward, get, gift, give, grant, guarantee, hand, impart, inform, issue, keep, lease, leave, lend, loan, lose, mail, name, offer, owe, pass, pay, permit, play, pose, post, prescribe, present, promise, propose, provide, quote, read, recommend, refuse, render, sell, send, serve, set, show, sing, slip, submit, suggest, supply, take, teach, tell, throw, toss, vote, wish, write, yield
– All verb-particle combinations including a transitive particle verb and one of the ten following particles were extracted from the corpus: around, away, back, down, in, off, on, out, over and up. Cases involving passive sentences, sentences with extracted direct objects, modified particles, names, titles, or other fixed expressions, and prepositional verbs were subsequently filtered out. In addition, instances involving pronominal direct objects and direct objects longer than six words were excluded. This process returned 2,480 tokens of the particle placement alternation (for further details on the particle placement database, see Grafmiller & Szmrecsanyi Reference Grafmiller and Szmrecsanyi2018).
– Due to the difficulty of automatically identifying all the relevant instances of omitted subject pronouns in the corpus, these had to be manually extracted. A balanced sample of 40 texts per ICE component was created by randomly selecting 10 spoken informal, 10 spoken formal, 10 written informal and 10 written formal texts, from which 1,229 interchangeable instances of omitted pronominal subjects were retrieved.Footnote 3 A random sample of 1,229 overt subject pronouns was then automatically obtained from the same texts, totaling 2,458 instances of both omitted and overt subject pronouns. Interchangeable tokens excluded non-referential omitted/overt subject pronouns, cases in which both the subject pronoun and the auxiliary verb of the clause were dropped, imperative sentences, and overt pronouns in tag questions (for further details on the subject pronoun omission database, see Tamaredo Reference Tamaredo2018).
The datasets were then annotated for several language-external and language-internal constraints although, for the purposes of the present article, we restricted our analysis to the five most important language-internal predictors of each syntactic alternation. This was a measure to ensure model convergence but, as the findings of Röthlisberger et al. (Reference Röthlisberger, Grafmiller and Szmrecsanyi2017) suggest, the most prominent constraints of a syntactic alternation are also the ones most sensitive to probabilistic indigenization effects (see also Grafmiller Reference Grafmiller2014). Therefore, we can be relatively confident that our set of constraints capture most potential differences between the varieties at hand. The five most important predictors were selected on the basis of per-alternation random forest analyses (see below) fitted to the whole dataset of all three varieties. Language-external constraints, such as register or medium of production, were excluded from this study because they did not consistently show up among the five most important predictors in all alternations. External factors have been shown to vary considerably across varieties of English, as they basically boil down to cultural and social differences (e.g. Heller et al. Reference Heller, Szmrecsanyi and Grafmiller2017), so including them would have potentially added extra heterogeneity to some alternations but not to others. Tables 1 to 4 display the five probabilistic constraints chosen for each syntactic alternation.
Table 1. The five most important predictors of the genitive alternation

Table 2. The five most important predictors of the dative alternation

Table 3. The five most important predictors of the particle placement alternation

Table 4. The five most important predictors of the subject pronoun omission alternation
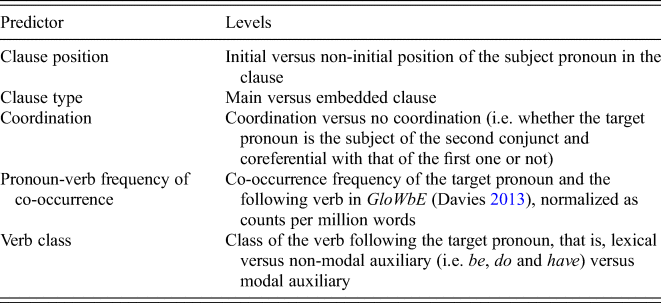
In addition, the data were annotated for the lemmas of the specific lexical items occurring in each of the instances: genitive tokens were annotated for possessor and possessum head nouns, datives for verb lemma, recipient and theme head nouns, tokens of the particle placement alternation for verb lemma, particles and verb-particle combinations, and subject pronouns for the following main verb lemmas.
The degree of alternation-internal homogeneity across the three varieties was estimated in three steps. First, we fitted a mixed-effects binary logistic regression model and a random forest per variety using the same model formula per alternation. Statistical analyses were carried out in R (R Core Team 2017) using the glmer() function in the lme4 package (Bates et al. Reference Bates, Maechler, Bolker and Walker2015) for mixed-effects models and the cforest() function in the party package (Hothorn et al. Reference Hothorn, Buehlmann, Dudoit, Molinaro and Van Der Laan2006; Strobl et al. Reference Strobl, Boulesteix, Zeileis and Hothorn2007, Reference Strobl, Boulesteix, Kneib, Augustin and Zeileis2008) for random forests. Both mixed-effects models and random forests seek to predict the choice between variants of a syntactic alternation (e.g. s-genitive vs of-genitive) given a set of predictors (here: restricted to five) and, as we detail below, they enable us to assess the strength, direction and relative predictive importance of our predictors. While mixed-effects models make their predictions on the basis of a mathematical equation, random forests establish the usefulness of a predictor through trial and error. Mixed-effects models are well-suited for analyzing corpus data of the kind used here because they allow us to take into account the non-independence of our observations via random effects adjustments for, e.g., lexical items or speakers sampled. Mixed models thus provide more reliable generalizations about broader patterns beyond the specific lexical items or speakers observed in our datasets. Random forests, on the other hand, enable us to explore more idiosyncratic patterns within our datasets, e.g. non-linear effects and interactions. Random forests, as implemented in the most common packages, are not well suited to deal with so-called random effects, since they cannot handle categorical predictors with very large numbers of levels.Footnote 4 However, an advantage of random forests is that they are quite robust to common issues in linguistic analyses, such as data sparseness or predictor non-linearities (see also Tagliamonte & Baayen Reference Tagliamonte and Harald Baayen2012: 158–61 for details). The method averages over a defined number of conditional inference trees using random subsampling and a permutation scheme (see Strobl et al. Reference Strobl, Boulesteix, Kneib, Augustin and Zeileis2008 for details).
The computed mixed-effects models included the five most important language-internal predictors per alternation as fixed effects and the lemmas of lexical items as random effects (see Appendix). Due to the low number of some lexical items (and ensuing issues with model convergence), we grouped infrequent items together for the modelling process. For each alternation and random predictor, we identified the frequency value which separated lexical items into two groups: one containing 10 percent of the items, which occurred more often than the selected threshold, and another group containing the remainder 90 percent, which occurred less often than the specified frequency value. Lexical items in the low frequency group were subsequently bundled together, with the exception of particles in the particle placement alternation and verbs in the dative alternation which were not grouped due to their high frequency. No interaction terms were added. We are well aware of the fact that previous research on the alternations that we study often show robust interaction effects, although mostly between language-internal and -external predictors and hardly ever between language-internal predictors. Hence, we decided not to test for interaction effects in order to keep our models simple and because large models with many interactions often lead to serious fitting/convergence problems. Random forest model formulas comprised only the five most relevant constraints per alternation.
In a second step, we calculated the similarity between varieties along the ‘three lines of evidence’ (Tagliamonte Reference Tagliamonte, Chambers and Schilling2002; see section 2) using the method proposed in Szmrecsanyi et al. (Reference Szmrecsanyi, Grafmiller and RosseelMS):
1. Statistical significance: the number of shared significant and non-significant constraints in per-variety mixed-effects models (at p < 0.05, following Szmrecsanyi et al. Reference Szmrecsanyi, Grafmiller and RosseelMS).
2. Relative strength: the inverse of the (Euclidean) distance between the coefficient estimates in per-variety mixed-effects models (calculated without the intercept).
3. Constraint ranking: Spearman's rank correlation coefficient between the predictors’ variable importance values obtained from per-variety random forests using the varimpAUC() function in the party package (Strobl et al. Reference Strobl, Boulesteix, Kneib, Augustin and Zeileis2008).
Note that when calculating Euclidean distances using coefficient estimates, a change in the reference and predicted level(s) of the constraints and dependent variable might lead to different results. To overcome this potential issue, we transformed all binary predictors to numbers (e.g. recipient animacy = {animate, inanimate} changed to = {0, 1}) and centered the values around the mean (following Gelman Reference Gelman2008). Furthermore, the reference level and predicted levels were set the same for each alternation. We chose Euclidean distance instead of Spearman's rank correlation to calculate similarity between varieties on the basis of coefficient estimates because the latter does not take into account patterns across the sizes of the predictors’ effects, only their relative (absolute) size. Coefficient estimates with the same values but opposite signs would thus be maximally similar using Spearman's rank correlation, while Euclidean distance would recognize the distance between them.
For each of the three lines, we obtained one similarity score for each alternation separately by variety (see tables 6 to 9). On this basis, we calculated a mean similarity score for each line and each alternation averaging across all varieties (see table 5). And lastly, we calculated the mean similarity between the varieties for each alternation as a measure of its overall stability by averaging across all three lines, which we interpret here as reflecting the alternations’ degree of probabilistic indigenization across BrE, IndE, and SgE: the lower the value, the more heterogeneous the alternation and thus the greater its degree of probabilistic indigenization.
Table 5. Similarity scores across alternations

Table 6. Per-variety similarity scores of the genitive alternation for each line

Table 7. Per-variety similarity scores of the dative alternation for each line

Table 8. Per-variety similarity scores of the particle placement alternation for each line

Table 9. Per-variety similarity scores of the subject pronoun omission alternation for each line

Finally, the alternations’ lexical specificity was operationalized on the basis of the concordance index C, which represents how well the model discriminates between the levels of the response variable. In order to tease out the lexical effects from the random structure in the mixed-effects models, we additionally computed fixed-effects only models per variety and alternation by means of the glm() function in R (R Core Team 2017). After computing the C statistic for both the mixed-effects and fixed-effects model, we subtracted the C statistic of the fixed-effect models from the C index obtained from mixed-effects models. To calculate C, we made use of the somers2() function in the Hmisc package (Harrell Reference Harrell2014). The resulting value indicates the increase in discriminative power from a fixed-effects only model to a model comprising both fixed effects and lexical items as random effects, thus signaling the importance of lexically specific constituents. We also considered an alternative heuristic to quantify lexical specificity by making use of R 2 values. R 2 is a goodness-of-fit statistic which is usually equated to the proportion of variance accounted for by the model: an R 2 value of 1 would correspond to 100 percent of the variance accounted for by the model. An alternation's degree of lexical specificity could hypothetically be operationalized as the increase in R 2 values from a fixed-effects only model to a model with both fixed effects and lexical items as random effects. This would in theory reflect the importance of random effects in the model and, therefore, indicate how strongly associated each alternation is with specific lexical items. However, we refrained from using R 2 as a measure of lexical specificity since its interpretation is not as clear as in linear regression models, where it accounts for the proportion of variance in the response variable that is explained by the predictors (see Levshina Reference Levshina2015: 259). Furthermore, R 2 values are usually lower in logistic regression than in linear regression models, even when they are equivalent in terms of goodness of fit. This is why the concordance index C is commonly reported in logistic regression analysis instead of R 2 (e.g. Hosmer & Lemeshow Reference Hosmer and Lemeshow2000: 162), and why we chose to rely on lexical specificity values calculated on the basis of the former statistic.
4 Results
Before zooming in on the variety-specific similarity scores per line and alternation (tables 6 to 9), we first take a cross-varietal aggregate perspective. Table 5 displays the values for the averaged similarities across all varieties per alternation and by line of evidence. Means for each alternation across all three lines of evidence are given in the last row, means of each line of evidence are provided in the last column, and a global mean in the bottom right cell. All values range from 0 to 1, with 0 indicating no similarity between varieties and 1 indicating complete overlap. Overall, the numbers suggest that there is a great deal of grammatical homogeneity across the varieties at hand. This is noticeable in the global mean across alternations and lines of evidence (i.e. 0.782), as well as in the individual means for each alternation and line, which all range above 0.700. The proposed similarity between varieties on the probabilistic level is striking: speakers’ choices between competing variants seem to be influenced by language-internal constraints that behave very similarly – within each alternation – across varieties irrespective of regional distinctions. Differences do exist, however, between the alternations: looking at the overall mean across all three lines of evidence (last row), the genitive alternation exhibits the highest mean homogeneity (0.881) of the four syntactic phenomena, followed, in increasing order of heterogeneity, by subject pronoun omission (0.806), the dative (0.731) and the particle placement (0.708) alternations. This means that particle placement is the most probabilistically indigenized alternation across the set of varieties studied, closely followed by the dative alternation. On a global level, probabilistic indigenization is mostly driven by the relative importance of predictors, i.e. the constraint ranking, as indicated by the mean value of 0.750, and by relative strength (mean of 0.771 across all alternations). In contrast, statistical significance, i.e. whether or not a predictor is significant in variety A and variety B, adds less to the global heterogeneity across all alternations (mean value of 0.824). Note also that the genitive and dative alternations follow the global pattern in that statistical significance is mostly similar across varieties, while particle placement and subject pronoun omission are most cross-regionally homogeneous with regard to relative strength of the predictors.
Leaving the aggregate perspective in table 5, we now turn to the similarity values for each variety separately across all three lines of evidence (see tables 6 to 9) to provide us with a more fine-grained perspective on alternation-internal differences between varieties.
The genitive alternation (table 6) is overall highly homogeneous, with values over 0.800 in all varieties and each line of evidence. The last column of table 6, which contains the mean values per variety across the three lines, reveals that the genitive alternation is more homogeneous in BrE, followed by SgE and, lastly, IndE, where it exhibits the greatest degree of heterogeneity.
In the dative alternation (table 7), the lowest values are found with regard to relative strength (BrE: 0.545, IndE: 0.558, SgE: 0.673) and, particularly in IndE, in the constraint ranking scores (0.600). The mean values across the three lines (last column) reflect a cline of varieties in which the dative alternation is more homogeneous in SgE than in BrE with IndE exhibiting the least homogeneity across all three lines (0.686).
Moving on to particle placement alternation (table 8), the similarity scores indicate more heterogeneity than in the dative or genitive alternation with most values ranging between 0.600 and 0.800. The lowest values, 0.600 and 0.650, are found in constraint ranking. SgE displays the most heterogeneity across all three lines of evidence while IndE is the most homogeneous variety, with BrE occupying an intermediate position.
Finally, the subject pronoun omission alternation is again overall highly homogeneous, with most values exceeding 0.800, except for statistical significance (0.667) and constraint ranking (0.700) scores in SgE and IndE respectively (see table 9). Regarding the varieties’ alternation-internal homogeneity, subject pronoun omission is more homogeneous in BrE, followed by IndE and, lastly, SgE.
Next, we averaged the varieties’ mean values across all four alternations to calculate the mean cross-alternation homogeneity per variety. Results show that the alternations are most homogeneous in BrE (0.802), with SgE (0.777) and, particularly, IndE (0.766) exhibiting a greater degree of cross-alternation heterogeneity. This finding is consistent with the Inner Circle/Outer Circle and L1/L2 statuses of the varieties: we would expect Outer Circle/L2 varieties to display more probabilistic indigenization effects than Inner Circle/L1 varieties as suggested by the literature (e.g. Grafmiller & Szmrecsanyi Reference Grafmiller and Szmrecsanyi2018, and references therein), and this is in fact what our results seem to indicate.
To follow up on the second main objective of the present study, we next examined the extent to which the degree of probabilistic indigenization reflects an alternation's lexical specificity. If, as Szmrecsanyi et al. (Reference Szmrecsanyi, Grafmiller, Heller and Röthlisberger2016) suggest, the degree of probabilistic indigenization of a given alternation is proportional to its lexical specificity, we should observe a correspondence between the cline of alternations regarding their homogeneity and the amount of variance accounted for by the lexical effects per alternation from the random effects structure in the per-variety mixed-effects models. Recall that we calculated the lexical specificity of an alternation as the difference in C-statistic between those mixed-effects models and fitted fixed-effects models using the same model formula for the fixed effects. To this end, we subtracted the C values obtained from fixed-effects-only models from the C values of the mixed-effects models. The larger the value, and thus the larger the difference between fixed- and mixed-effects models, the more the random effect structure contributes to the model's discriminative power. Results are shown in table 10: particle placement emerges as the most lexically specific alternation (0.065), followed by the dative (0.060), genitive (0.047), and subject pronoun omission (0.021) alternations.Footnote 5
Table 10. Lexical specificity across alternations and varieties – values indicate difference in C statistic between mixed-effects and fixed-effects models

The distribution of the three varieties as to the alternations’ level of lexical embedding according to the models’ C values is summarized in (6) from the most to the least lexically specific variety.
(6) Genitive alternation: SgE > BrE > IndE
Dative alternation: SgE > BrE > IndE
Particle placement alternation: SgE > BrE > IndE
Subject pronoun omission alternation: IndE > SgE > BrE
The genitive, dative and particle placement alternations are more lexically specific in SgE than in BrE and IndE, while subject pronoun omission is more tightly associated with individual lexical items in IndE than in SgE and BrE. The mean values across alternations (right-most column in table 10) reveal that, overall, the alternations are more lexically specific in SgE (0.055), surpassing both BrE (0.049) and IndE (0.042).
The varieties’ cline in probabilistic indigenization (from most to least indigenized) and their cline in lexical specificity (from most to least lexically specific) are shown in (7). The order obtained from the C-statistic (7b) almost mirrors the one based on the degree of an alternations’ probabilistic indigenization across varieties (7a) with the exception of the genitive and subject pronoun omission alternations whose order is reversed.
(7)
(a) Varieties’ cline in probabilistic indigenization
particle > dative > pronoun omission > genitive
(b) Varieties cline in lexical specificity according to C-statistic
particle > dative > genitive > pronoun omission
The comparison between (7a) and (7b) shows a high degree of overlap between the two clines and thus provides preliminary support for our initial hypothesis, namely that an alternations’ degree of probabilistic indigenization is proportional to its lexical specificity. The implications of these and the rest of the findings described in this section are discussed next.
5 Discussion
The present study has investigated the extent to which an alternation's degree of probabilistic indigenization is proportional to its lexical specificity in a comparison of three varieties of English using a novel approach that applies comparative sociolinguistic methods to compare probabilistic grammars quantitatively. Results show that, overall, the varieties investigated are very homogenous in their alternation-specific probabilistic grammar. Our findings thus support previous claims in the literature that varieties of English are overall grammatically similar, since the same probabilistic constraints tend to influence speakers’ constructional choices across varieties. Our results further highlight that this grammatical stability persists across a wide range of syntactic alternations, suggesting that English is indeed syntactically very stable regardless of differences in the regional backgrounds of its speakers and irrespective of whether it is spoken as a first or second language. We should add at this point that including a larger number of varieties and probabilistic constraints – we considered only three varieties and five predictors per alternation – could increase the degree of grammatical heterogeneity observed so far (Szmrecsanyi et al. Reference Szmrecsanyi, Grafmiller and RosseelMS). This limitation certainly warrants further investigations in the future.
Despite the overall similarities observed, the four alternations, in line with previous studies, are not equally prone to exhibit probabilistic indigenization effects. Particle placement seems to be more sensitive to probabilistic indigenization effects than the dative alternation, which in turn is more sensitive than the genitive alternation (see, e.g., Szmrecsanyi et al. Reference Szmrecsanyi, Grafmiller, Heller and Röthlisberger2016; Szmrecsanyi et al. Reference Szmrecsanyi, Grafmiller, Bresnan, Rosenbach, Tagliamonte and Todd2017). Subject pronoun omission emerged from our analysis as being a highly homogeneous syntactic alternation, situated between genitives and datives in terms of its cross-varietal stability. This alternation-internal homogeneity is surprising considering that the main substrate languages of IndE and SgE, namely Hindi and Mandarin Chinese respectively, allow the omission of pronouns in subject (and other) positions more frequently and in a wider range of contexts than is commonly assumed to be the case in Standard English (e.g. Kachru Reference Kachru2006: 258–9, for Hindi; Li & Thompson Reference Li and Thompson1989: 657–62, for Mandarin Chinese). The influence of the substrate languages could, hypothetically, have resulted in IndE and SgE manifesting a preference for the omitted pronominal subject variant vis-à-vis BrE. Since the effect size of probabilistic constraints has been shown to be sensitive to language contact in the form of substrate influence (Rosenbach Reference Rosenbach2017) or second language acquisition effects (Heller et al. Reference Heller, Szmrecsanyi and Grafmiller2017), we could have observed a weaker effect of predictors favoring the overt variant and a stronger effect of those selecting the omitted variant, or even a change in the direction of the effect of certain constraints in favor of omitted pronouns. However, no such substrate effects were discerned in the present data on subject pronoun omission. To the contrary, the mean similarity score for subject pronoun omission is the second highest (with 0.806) in the comparison.
Our results also showed that most differences between the four alternations arise as a function of relative strength and constraint ranking. In other words, alternations do not generally differ with regard to which constraints influence speakers’ syntactic choices across the three varieties but more with respect to (a) the extent to which the constraints have an effect on the choice between the variants and (b) the constraints’ relative importance. For instance, direct object length is a significant predictor in all three varieties in particle placement, with longer direct objects disfavoring the verb–object–particle order. However, the strength of this effect fluctuates across the varieties as indicated by the mixed-effects models: IndE, with a direct object length coefficient estimate of –3.608, disfavors the discontinuous particle–verb order variant more strongly with each one-letter increase in the length of the direct object phrase than SgE (–2.508) and BrE (–2.091) (see Appendix). Similarly, constraint ranking emerges as a prominent locus of variation in the particle placement alternation. As shown in table 11, all five predictors get a different rank in at least one of the varieties. Moreover, the rankings of three predictors – direct object definiteness, semantics and verb surprisal – are never constant across the three varieties.
Table 11. Constraint ranking of five predictors in the particle placement alternation

Regarding variety-specific patterns, our results indicate that the two Outer Circle/L2 varieties are less homogeneous and more probabilistically indigenized than BrE. Furthermore, IndE displays a lower mean alternation-internal homogeneity score than SgE. A variety's degree of probabilistic indigenization thus seems to correspond directly to its variety type: broadly speaking, L1 varieties are less indigenized than L2 varieties. Note, however, that any generalizations obtained on the basis of only three varieties have to be taken with a pinch of salt and need further substantiation by future studies aggregating over a larger number of varieties and alternation phenomena.
Following Szmrecsanyi et al. (Reference Szmrecsanyi, Grafmiller, Heller and Röthlisberger2016), we hypothesized to find a correspondence between the extent to which a syntactic alternation exhibits cross-varietal probabilistic indigenization effects – measured as its degree of internal homogeneity across varieties – and its lexical specificity, that is, how strongly associated the alternation is with concrete representations containing specific lexical items. Lexical specificity was operationalized in the present article on the basis of the concordance index C, by computing fixed-effects only models and subtracting the C statistic from the C index obtained from mixed-effects models. The order of alternations as to their lexical specificity almost perfectly matches our hypothesis: particle placement is more lexically specific than the dative alternation, which in turn is more specific than the genitive and subject pronoun omission alternations. Only the specificity values of the latter two alternations are somewhat inconsistent with the hypothesis in that genitives emerged from the analysis as being slightly more strongly connected with particular lexical items than subject pronoun omission. Therefore, and despite the existence of small inconsistencies, the four syntactic alternations behave largely as we had hypothesized with respect to their sensitivity to probabilistic indigenization effects and their lexical specificity.
With respect to the alternations’ degree of lexical specificity in each of the three varieties, there are no discernible patterns owing to Inner Circle/Outer Circle and L1/L2 distinctions. SgE is the variety where alternations are overall most lexically specified, followed by BrE and then IndE. It has been suggested that L2 varieties rely on concrete instantiations of syntactic constructions involving specific lexical items more strongly than other varieties (e.g. Hoffmann Reference Hoffmann, Buschfeld, Hoffmann, Huber and Kautzsch2014: 175–6; Röthlisberger et al. Reference Röthlisberger, Grafmiller and Szmrecsanyi2017), but this does not seem to hold in our data: even though the alternations are indeed more lexically specific in SgE than BrE, this is not the case in IndE compared to BrE. This finding is surprising considering that IndE emerged as being highly lexically specific in those studies and, in particular, as being more specific than SgE and BrE. Note, however, that especially Röthlisberger et al. focused on recipients in the dative alternation, while our study includes all lexical items that instantiate a construction and averages across their discriminative power in syntactic choice making. Another crucial difference is the method used to measure the level of lexical embedding of alternations. Whereas we calculated lexical specificity as the increase in discriminative power from a fixed-effects only to a mixed-effects model, Hoffmann (Reference Hoffmann, Buschfeld, Hoffmann, Huber and Kautzsch2014) and Röthlisberger et al. (Reference Röthlisberger, Grafmiller and Szmrecsanyi2017) did so on the basis of the degree of collostructional strength between lexical items and particular constructions. By way of a somewhat ad hoc explanation, we would like to suggest that different heuristics may provide diverging results as they seem to measure different aspects of lexicality. Also, lexical specificity seems to depend on the construction investigated as shown in our results and in the comparison to Hoffmann (Reference Hoffmann, Buschfeld, Hoffmann, Huber and Kautzsch2014). Comparing methodologically mismatching studies, then, can strengthen our understanding of the limitations of measuring lexical specificity across varieties.
6 Conclusion
The aims of the present article were twofold. First, we sought to estimate the extent to which four alternations – the genitive, dative, particle placement and subject pronoun omission alternations – exhibited probabilistic indigenization effects, that is, the occurrence of locally characteristic stochastic patterns of syntactic variation, across three varieties of English, namely BrE, IndE and SgE. We did this by delineating a corpus-based variationist method for quantifying differences in the underlying probabilistic constraints that regulate the choice between competing syntactic variants across varieties. Three lines of evidence, as proposed in the comparative sociolinguistics literature, were considered: the statistical significance of predictors, their relative strength, and the order of constraints as to their relative importance in the alternation-internal grammars of the varieties. The results obtained from the application of this methodology to our data allowed us to arrive at two important conclusions. First, English is on the whole highly syntactically stable as a world language, since there is a great deal of alternation-internal grammatical homogeneity across varieties regardless of regional differences between speakers. Second, probabilistic indigenization effects can be observed to different degrees across syntactic alternations: in our study, particle placement emerged as the most heterogenous alternation, followed, in increasing order of homogeneity, by the dative, subject pronoun omission and genitive alternations. This order coincides with the findings of previous studies, in which particle placement also surpassed datives and genitives in terms of grammatical instability across varieties, thus providing independent validation for the method proposed here.
A second aim of this study was to assess the lexical specificity of the four syntactic phenomena investigated, that is, the strength of the association of each alternation with concrete representations of more abstract schemas involving specific lexical items. To this end, we employed a procedure to quantify an alternation's degree of lexical specificity which relied on the C goodness-of-fit statistic and reflected the importance of individual lexical items in order to account for the variance observed in the data. The order of alternations as to their lexical specificity across varieties was (almost) a mirror image of the cline based on their grammatical homogeneity: particle placement turned out to be the most lexically specified alternation, followed, in decreasing order of specificity, by the dative, genitive and subject pronoun omission alternations. Even though further research is still needed to ascertain the most appropriate way of measuring the role of individual lexical items in the choice between competing syntactic variants, our study provides empirical evidence supporting the connection between an alternation's sensitivity to cross-varietal probabilistic indigenization effects and its degree of lexical specificity.
The VADIS-method employed is (still) in an experimental stage and will need further applications to other alternations and datasets, preferably also more complex syntactic phenomena such as verb complementation, alternations with more than two variants (see Gerwin & Röthlisberger, Reference Gerwin, Röthlisberger, Röthlisberger, Zehentner and Collemanto appear) and alternations on other levels of the grammar, e.g. lexical variation, semantic variation or pragmatic variation. Aggregating then over multiple analyses that capture different parts of speakers’ grammar would enable us to paint a more complete picture of variation in probabilistic indigenization. Especially the calculation of Euclidean distances on the basis of coefficient estimates from regression modeling needs further testing regarding concept validity and reliability (see also Heller Reference Heller2018: 199–204, who tested concept validity, and Röthlisberger Reference Röthlisberger2018: 175, 215–16, who used a bootstrapping procedure to assess reliability). As one reviewer rightly pointed out, the way we tested lexical specificity here ignores the fact that the same character strings might express different meanings depending on the other lexical items used in the variant (e.g. give back to the community is different in idiomatic meaning from give back to my mother). At the moment, we only measured lexical specificity by focusing on individual lexical items neglecting the wider context of usage. Other useful additional heuristics to assess the importance of lexical constituents would need to be considered in future work, e.g. collostructional analysis (Stefanowitsch & Gries Reference Stefanowitsch and Gries2003), as applied, e.g. in Röthlisberger et al. (Reference Röthlisberger, Grafmiller and Szmrecsanyi2017) to the dative alternation. Such additional methods can provide more data to help us validate the results obtained here and thus to overcome the limitations of the present study with regard to the number of varieties and alternations studied. Furthermore, and despite the fact that our models had an outstanding predictive capacity, it would be desirable to include more than five predictors per alternation to reach a more representative description of the phenomena at hand. Lastly, other methods could be used to compare varieties of English as to their degree of grammatical homogeneity, such as the Akaike Information Criterion (Grafmiller & Szmrecsanyi Reference Grafmiller and Szmrecsanyi2018). These and other measures would enable us to be in a better position to delimit the scope of variation within and across varieties of English around the world.
Appendix
Table A1. Per-variety mixed-effect models of the genitive alternation
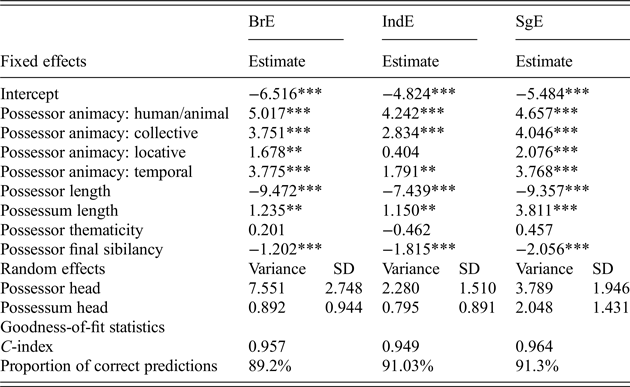

Figure A1. Per-variety random forests of the genitive alternation
Table A2. Goodness-of-fit statistics for per-variety random forests of the genitive alternation

Table A3. Per-variety mixed-effects models of the dative alternation


Figure A2. Per-variety random forests of the dative alternation
Table A4. Goodness-of-fit statistics for per-variety random forests of the dative alternation

Table A5. Per-variety mixed-effects models of the particle placement alternation


Figure A3. Per-variety random forests of the particle placement alternation
Table A6. Goodness-of-fit statistics for per-variety random forests of the particle placement alternation

Table A7. Per-variety mixed-effects models of the subject pronoun omission alternation


Figure A4. Per-variety random forests of the subject pronoun omission alternation
Table A8. Goodness-of-fit statistics for per-variety random forests of the subject pronoun omission alternation
