One of the most difficult disaster response tasks in a major mass fatality incident is the prompt identification of the deceased's remainsReference Morgan, Sribanditmongkol, Perera, Sulasmi, Van Alphen and Sondorp1 to facilitate returning the body to surviving family members. Rapid victim identification is particularly problematic after sudden-onset mass fatalities, and the delay in identifying and releasing human remains adds an additional burden to survivors and the community response as a whole.2 Expediting the identification process while maintaining identification accuracy can be invaluable, particularly in disaster settings in which advanced technological identification resources are scarce or unavailable.
THE DISASTER VICTIM IDENTIFICATION PROCESS
The practice of processing a large number of fatalities resulting from a major mass fatality incident is known as the disaster victim identification (DVI) process. One of the primary purposes of the DVI process is to establish each victim's identity, so that each body can be returned to the correct family members.Reference Jensen3 The activity set within this DVI process often varies across disasters, according to planning and local circumstances. The activities in each body-identification process, however, can be divided into three major functional areas (Figure 1)4Reference Fixott, Arendt, Chrz, Filippi, McGivney and Warnick56: (1) postmortem (PM) data collection phase, (2) antemortem (AM) data collection phase, and (3) PM-AM data comparison and reconciliation phase.4

Figure 1. Current Disaster Victim Identification (DVI) Process Model.
The PM data collection phase is a major activity at the incident site. Responders locate human remains, process the scene (document the location of the remains, personal effects, and evidence), and conduct the systematic removal of the remains and personal effects, transferring them to the morgue.6 At this stage, the activities of the PM phase transition to the morgue stations, where each unidentified decedent is passed through a series of forensic examinations and analyses, and different types of evidence related to the remains are collected. This evidence is later used in the comparison and reconciliation phase to establish the identity of the victim.
The methodologies employed by forensic experts to collect evidence from the remains range from simple observations of facial and external body features plus personal artifacts (referred to in this study as conventional methods) to more complex technological processes, such as DNA, fingerprints, or dental features (designated as technological methods). The type of evidence collected in this PM phase may depend on several factors, such as the forensic protocol adopted, the type of incident, the condition of the human remains, the type and amount of resources available, and the desired outcome.2Reference Jensen3
The AM data collection phase runs concurrently with the PM phase. The immediate responsibility of personnel conducting the AM phase is to compile a missing persons list. This list provides a means of organizing the collection of information from relatives and friends about the individuals believed to be among the incident's deceased victims. The information assembled during the AM phase generally includes: (1) basic biological features related to the reportedly missing person (eg, gender, estimated age at death, race, identifying characteristics), (2) a description of the clothes and items that may have been in the victim's possession, and (3) a time and location the missing person was last seen. Family members may also be asked to provide medical and dental records for medical/dental profiles and biological samples to develop a DNA profile.7 All of the data are entered into the AM database, organized appropriately, and then transferred as AM records to the morgue and made available to the identification team for the data comparison and reconciliation phase.
In the final phase, PM and AM records are evaluated to discover possible matches. If this comparison is accomplished using computer-based systems, the suggested matches are then reviewed and verified directly by the respective forensic specialists. This activity should narrow down the number of possible matches between the designated PM data record and all of the AM data files from the missing person's cohort until just a single possibility remains.7 The proposed identification match is then presented to a reconciliation board that reviews the case and makes a final decision. If the weight of evidence supporting the identification is considered satisfactory to the board members and no irreconcilable differences remain, then the authority in charge of the identification process issues a death certificate and the body is released to the family designated in the matching AM record.Reference Jensen3Reference Lain, Griffiths and Hilton8 Otherwise, the case is kept open and additional identifying evidence is sought.7Reference Keiser-Nielsen9
Issues Associated With the Current DVI Process
The victim's identity is determined through an assessment of the likelihood of the “match” between PM and AM dataReference Thompson and Black10; under ideal conditions, it is reached using all available techniques to minimize the statistical uncertainty associated with the matching process.Reference Brannon and Morlang11 However, in the currently used DVI approach to victim identification, the major dependence is placed on technological methods, because this matches features from DNA, fingerprints, or dentition that are considered relatively unique to each individual and generally expected to produce a more certain positive identification.Reference Jensen3 Based on this belief, every unidentified body undergoing PM examination is commonly processed through each station operating in the morgue, both conventional and technological, as depicted in the current DVI process (Figure 1). Consequently, both AM conventional and technological data must be available from the morgue examination before conducting the comparison and reconciliation phase (Figure 2).The PM-AM data collection process is therefore rigid, with its steps dictated by the specific forensic protocol adopted for the incident and influenced by the condition of the human remains (eg, severely burned human remains may skip the fingerprints station).

Figure 2. Current Conceptual Disaster Victim Identification (DVI) Decision Process.
The collection and processing of technological PM and AM data are usually the rate-limiting steps for the DVI process, since technological processing is the most time intensive and depends on more sophisticated and specialized forensic personnel, equipment, and supplies. This DVI process may significantly delay the comparison and reconciliation phase, thereby considerably postponing each victim's identification. For example, the PM dental data-gathering process, which is the slowest among the PM examinations,Reference Lain, Griffiths and Hilton8 may require an average of three work-hours for each case to be extracted and recorded.Reference Mühlemann, Steiner and Brandestini12 Likewise, the AM technological data-gathering process may be a major rate-limiting step for the DVI process. Medical or dental reports, for example, can be obtained from relatives, but more often are acquired from a victim's physicians, dentist, and hospitals (if the victim was ever hospitalized before the incident). The time and effort required for tracking down all of these sources for each missing person can therefore be considerable. In addition, these records—when found—can create a logistical burden and may take a significant amount of time to reach the identification center, which is usually located in the proximity of the disaster area.Reference Mühlemann, Steiner and Brandestini12 All of this must be accomplished before the time-consuming technological analysis of the AM data can begin. Constraints on resources, particularly common in mass fatality scenarios, may therefore limit the DVI surge capacity for these technological forensic tasks.
Data processing and matching techniques that employ conventional physical evidence are less labor intensive, and conventional methods have significantly lower technological requirements. These physical features do not require advanced technological equipment or extensive expertise to be analyzed and evaluated, but instead rely primarily on visual observations or straightforward body measurement techniques. Individually, these identity elements are considered less specific or unique, as compared to features established by technological methods. Conventional methods have historically produced results that were qualified only as possible or presumptive identification, requiring further forensic analysis to confirm identity. When considered individually, in fact, conventional elements are usually not sufficient to definitively establish the victim's identity at a satisfactory level of uncertainty. The subjectivity and lack of objective rigor that is common in collecting this conventional evidence, plus the lack of standardized application of rules of comparison, have diminished the forensic credibility of these methodologies.Reference Warren13 However, if conventionally acquired features are collected carefully and the individual feature categories are combined according to appropriate criteria, then matching among combinations of conventional elements may provide enough evidence to establish accurate body identification within the acceptable level of uncertainty.Reference Farinelli-Fierro14 The application of these criteria could potentially lead to a reduction in the use of costly, time-consuming, and labor-intensive technological forensic methods and consequently would increase the efficiency of the DVI process. In certain mass fatality incidents, technological identification methods could be the primary identification techniques relied on only when the condition of the human remains makes conventional-identification techniques ineffective. In all other circumstances, however, carefully conducted conventional methods of identification could be emphasized when possible.
Study Objective
The objective of this study was to develop a decision-support tool (DST) that facilitates body identification in mass fatality incidents, particularly when the availability of technological resources and advanced forensic expertise is limited. The purpose of the tool is to improve the efficiency of victim identification, promoting rapid matching in mass fatality incidents by using selected conventional DVI methods. The DST uses the widely accepted DVI process as its conceptual framework and selected human physical features (external biological attributes) as identification data. This easily documented, physical information is gathered through the external examination of a victim's remains (PM data) and through missing person reports and interviews or questionnaires at the community's family assistance center (AM data).
The information is then entered into an electronic record and recorded in a format that reduces the subjectivity of the data-gathering process and promotes rapid matching. A file sorter evaluates the similarities between pairs of attribute records. The DST constructed in this study specifically evaluates the similarity between each conventional feature from a selected PM record and the feature's paired category from each record included in the AM database. An overall similarity score is then computed between the PM record and each evaluated AM record. The score from this comparison is derived from established similarity measures in the DST's framework. The AM file with the highest score represents the most probable match with the selected PM file. All AM files similar to the PM file can then be directly accessed for closer examination to determine if a preliminary match can be presumed and therefore referred to the medical examiner, law enforcement personnel, or other authorized persons to complete the formal identification process. The methods used in this DST are therefore designed to assist a medical examiner's mass fatality plan, based on the DVI model proposed by the International Criminal Police Organization (INTERPOL).4
METHODS
The methodological steps to develop this DVI model were as follows: (1) objectively describing the detailed DVI process model (Figure 1) that conducts objective, consistent AM and PM data gathering and accurate entry into AM and PM records; (2) defining a list of external physical attributes of interest, with each attribute-type objectively defined; (3) delineating the process for collecting these selected types of conventional attributes and recording them into AM and PM records; and (4) implementing a framework for assessing the similarity between confronting PM-AM attribute records. A system simulation was then developed to analyze the model's outputs.Reference De Cosmo15
The purpose of the multiple simulation runs was 2-fold. First, the simulations were designed to investigate the DST's accuracy under varied mass fatality incident scenarios, such as missing data, incorrect data, or many victims with similar physical features. Second, the simulation provided a means to evaluate the effect on identification accuracy created by combining selected conventional attributes rather than considering them individually in the AM and PM record comparison.
External Biological Attributes of Interest
The specific attributes of interest for the study were extracted from a review of multiple DVI forms used by a range of organizations involved in human remains management and identification.4161718 Among the variety of conventional attributes available in these forms, 31 attributes were chosen for the study (Table 1). The selected attributes were then grouped into two different categories: general and specific. General attributes, such as gender, age, or height (attribute-types 1 to 25 in Table 1), are not unique in nature and so convey less individuality. Specific attributes, such as a distinctive scar, piercing, or tattoo, are assigned to the “peculiarity group” (attribute-types 26 to 31 in Table 1) when reported in AM or PM records. The peculiarities can often, although not always, be unique elements and therefore contribute significantly to establishing identification. The specificity of these features is drawn from both their location on the body as well as their describable size, shape, and color. A body mapping was therefore created, starting with a body figure derived from an INTERPOL form. This mapping partitions the human body into 33 regions (βi) as shown in Figure 3.

Figure 3. Body Mapping Improves Information Accuracy by Promoting a More Specific Depiction of a Peculiar Attribute's Location.
Table 1. External Biological Attributesa

The Attribute Tables
Forms were developed to collect the attributes of interest, constituting the conventional data, and formatted to promote rapid matching. The design characteristics selected to achieve this purpose were:
• A single-page template collects the attribute-values: All data commonly used for the preliminary match between confronting AM and PM records are presented on a single page. The comprehensive missing person or body examination report may also include additional pages as sketches, instructions, or supplementary information that can be accessed during later identification activities, when a closer examination is needed to confirm or reject this preliminary match.
• Consistency plus flexibility: The form promotes consistent reporting of the designated attributes and allows a certain degree of flexibility by introducing appropriate fields to collect additional information or to include further attributes.
• Minimize input error: Control fields are introduced to prevent, or at least minimize, inadvertent input errors.
The developed form was designated the attribute table (Figure 4). The AM and PM attribute tables were constructed with identical designs to allow data collected from unidentified bodies to be specifically and directly compared to data collected when missing persons are reported, particularly when paper forms and direct visual comparison are used in lieu of computer matching. The tables are clearly distinguished by their color code: yellow for AM records and pink for PM records.
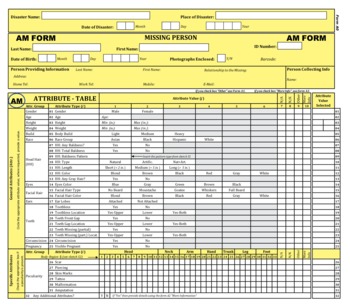
Figure 4. The Antemortem (AM) Attribute Table.
The Similarity Framework
The comparison process to judge the similarity between pairs of attributes from AM and PM records required several sequential development steps. First, the attributes of interest were classified based on common characteristics and behaviors; subsequently, appropriate metrics (similarity functions) for each defined class of attributes were established. Metrics for each attribute reflected a typical classification scheme that distinguishes between the domain size and the scale of measurements.Reference Anderberg19 The classification according to the size and discreteness of the range set had to distinguish between continuous attributes, such as for the weight of a person, and discrete attributes, such as race. The classification according to the scale of measurement, for which the scale is interpreted as a means of ordering data,Reference Andritsos20 distinguishes between nominal, ordinal, and interval attributes. Nominal attributes are those defined over a finite set of descriptive values; for example, gender has an assumed value of male or female, but these values bear no internal quantitative relationship and thus cannot be ordered. Conversely, ordinal attributes are characterized by values that can be ordered linearly from lower to higher, such as body build, which takes the values light, medium, or heavy. These values, however, do not provide any information regarding how close or far these elements are to each other. This relationship, however, can be established through interval attributes, for which the difference between attributes can be quantified numerically. Examples include the height or the weight of a person. By combining both classification schemes, the attributes selected in the study were categorized in three distinct cross-classification categories: nominal-discrete, ordinal-discrete, and interval-continuous. Each attribute was assigned to the appropriate cross-classification category, and a similarity function was defined for each category, based on accepted formulaic approaches in the published literature.Reference Andritsos20Reference Bozdgan21Reference Wilson22Reference Wilson and Martinez23Reference Han and Kamber24 This approach established specific metrics to measure similarity for each specific attribute (Figure 5).

Figure 5. Similarity Functions.
The similarity functions measure the similarity between the different classes of attribute-values included in antemortem and postmortem confronting records.
An analysis of the three similarity functions in Figure 5 indicates that these expressions weight all the attributes equally. In reality, this is not true because some attributes can occur less frequently than others, and some can provide more reliable information than others; thus, they should be weighted differently. An attribute weighting system was therefore considered to account for the varying contribution provided by each individual attribute in the computation of the overall measure of similarity. To address this, an algorithm was introduced to weight attributes according to their “importance.” The weighting system combines two different components: a frequency component named rarity factor [represented byP (i, j)] and a reliability component named classification-accuracy factor (represented by δi). The weighting factor w is thus defined by the expression w (i, j) = P (i, j) × δi, with i being the index that identifies the attribute-type and j the index that identifies the value of the attribute.
The rarity factor concept is best described by Keiser-Nielsen.Reference Keiser-Nielsen9 This component of the weighting system takes into consideration the fact that “while never unique, per se, any given physical feature does possess a certain distinguishing quality—a discrimination potential—according to its frequency of occurrence; the more frequently it occurs, the less characteristic it is.” Reference Keiser-Nielsen9 Therefore, the lower the frequency of occurrence, the higher the rarity attribute-value, and the greater the ability for that specific attribute to discriminate between two individuals. The rarity factor is defined as the inverse of the frequency of occurrence of the attribute-value in the data set and is computed as the total number of times a specific attribute-value occurs in the data set divided by the total population size N (ie, total number of records in the database).
The rationale for the development of the second component of the weighting system was driven by the consideration that the description of some biological attributes are more apt to undergo individual interpretation or subjective judgment than others. For example, describing the preexisting amputation of a body part has much higher potential of being accurate than describing the shape of the eyes or the size of the nose. Thus, the classification-accuracy factor of an attribute can be interpreted as an approximate measure of the likelihood that the attribute will be correctly classified. The values of classification-accuracy adopted in the study (Table 2) were approximated using expert judgment. Further studies should be conducted to formally assess this weighting factor. Higher weight was assigned to attributes that have higher probability of providing more reliable information for classification. For example, gender, under optimal body conditions (defined later in this section), generally has higher classification-accuracy compared to eye color. In fact, recognizing the correct gender value of an unidentified body (PM data) or providing the correct gender value of a missing person (AM data) is extremely likely. Conversely, the description of eye color is generally more likely to be subjective, so the level of uncertainty in classification is higher and the attribute should be weighted less because it provides less reliable information.
Table 2. Classification-Accuracy Factor (δi)a

It is noteworthy that the classification-accuracy could be different if the description of the attribute comes from an AM or PM record. For example, the height provided by a family member for a missing person's AM record could be more subjective (ie, less accurate) than the data recorded in the PM record by a medical examiner during the PM examination, as the latter can be measured directly from the body. For simplification purposes, this DST model adopted a single classification-accuracy weight for each attribute, assuming that an attribute has the level of greatest uncertainty in the classification for either the PM or AM records. This approach assumes that if either the AM or PM attribute is uncertain, then the matching of the attribute is less predictive; the attribute class is therefore reduced, based on the less certain AM or PM attribute.
Also, the condition of the victim's body could deeply affect the reliability of the PM attribute classification. Factors such as the PM interval, the context in which the body is found (eg, factors such as temperature and humidity, submersion in water), or the type of trauma (eg, explosion, fire, crushing) and the area of the body affected (eg, head) can drastically affect the degradation process of the body or the degree of human remains available for examination. Consequently, these factors affect the possibility of correctly describing many of the attributes. For example, if the body recovered from the incident suffered severe burns, even the gender could be unreliable and its classification-accuracy factor could be lower than the value normally assigned to that attribute when the victim's body is intact. To address this issue, a set of circumstances that define three general body conditions, optimal, intermediate, and poor (Figure 6),Reference Galloway, Birkby, Jones, Henry and Parks25 were considered in the development of the model.

Figure 6. During Postmortem Examination, the 33 Different Regions (βi) of the Victim's Body Are Analyzed and the Body Condition-Category Is Identified.
Third, in the final step of the comparison process, a fusion algorithm was defined to integrate the similarity findings from each attribute comparison between two records so that a consolidated overall matching value could be produced between each pair of confronting AM and PM records. The fusion algorithm is adapted from the general coefficient of similarity proposed by GowerReference Lumijärvi, Laurikkala and Juhola26 and is defined by the expression described in Figure 7. The Gower's coefficient is one of the best-known and widely used measures for this purpose.Reference Podani27 It allows handling data sets characterized by the simultaneous presence of different measurement units, as in this case, in which the computation of similarity is characterized by heterogeneous similarity measures, and has the advantage of tolerating missing values, which is very possible in actual mass fatality incident scenarios.

Figure 7. Fusion Algorithm.
The approach adopted in the study for addressing missing values was to exclude from the overall similarity computation score those attributes that have missing entries (as deducted from the g function in Figure 7). Consequently, a “corrective factor” η was introduced in the equation S(x,y) to account for how many and which types of attribute-values are missing. This coefficient η, called missing adjusting factor, has null effect on the score S when the record is complete (η = 1), whereas it has a negative effect on S when a record has missing attribute-values (η<1). In particular, the magnitude of the impact is determined by the number and the types of attribute-values that are missing in the records being compared.
The following examples can clarify the need for such a coefficient. If the equation S(x,y) did not include the coefficient η, then the overall similarity score computed between a pair of PM and AM records having 31 matching attributes would measure the same value as the similarity computed between two confronting records having just a single matching attribute, with the other 30 attribute-values missing. In both cases, the overall similarity score would be equal to one. Moreover, two AM records may each have one single missing attribute-value; for example, the first AM record is missing the data regarding eye color, while the second record is missing the information regarding preexisting amputation. If both records have all 30 attributes that match the corresponding attributes of a given PM record, then the overall similarity score in both cases is equal to one according to the equation S(x,y). However, even though the similarity score is achieved in both cases by the match of 30 attribute-values, the two results should differ, owing to the different reliability values associated with the missing attributes. That is, the preexisting amputation of a body part has much higher potential of being reliable than the eye color, and thus has a greater potential accuracy effect.
The System Simulation
System simulations were then conducted to assess the effectiveness of the developed DST and to investigate whether combinations of attributes used in conventional methods of body identification could decrease the level of uncertainty in mass fatality victim identification. A wide range of incident scenarios were designed, and their simulations were run to conduct this assessment. The simulation model was built employing the same framework used in the DST. The baseline simulation was developed by modeling the AM database, which is constructed during the AM data collection phase (from structured missing person reports), and the data comparison and reconciliation phase of the DVI process (from PM examination).
The description of the simulation model is beyond the scope of this article, but details on the simulation architecture are available.Reference De Cosmo15
RESULTS
The results obtained by running the simulation model are briefly summarized and presented here. A more detailed description of the system simulation and the conducted scenarios will be the subject of a separate article.
The output statistics generated by each simulation scenario demonstrated the effectiveness of the DST in decreasing the level of uncertainty related to identification accuracy using only conventional methods of victim identification. The simulation outcomes also suggested that if an unidentified body lacks any unusual physical characteristic (usually represented by the presence of one of the specific peculiarity attributes), then the differentiation between the exact AM match and the most similar individual in the AM database may be less clear and certain. In these situations, however, the prioritizing of AM records using similarity scores can still reduce the total number of the AM records that eventually are accessed for direct examination and comparison. If these strategies are ineffective at reducing to a “reasonable” number the potential AM matching records, then technological identification methods become essential for accurately establishing the victim's identity.
From the analysis of the simulation outputs, it was observed that the higher the percentage of missing values in the AM database, the lower the probability of finding the exact AM match in the group of AM records with the highest similarity score for a given PM record. This expected finding provided another indication for using technological methods as the primary evidence for establishing the identity of the human remains.
DISCUSSION
The results of this study demonstrated that carefully captured conventional elements of identification, when combined and considered as a data set, can be valuable in establishing identification of human remains within an acceptable level of uncertainty (acceptable level is defined by the authority charged with confirming the body's identity). Under the circumstances of a mass fatality incident, the further pursuit of identification through technological methods may be subordinated and the resources for technological methods directed toward cases in which conventional features are insufficiently unique or are unavailable. More importantly, this approach may effectively reduce the mismatch between the availability of overall fatality identification resources and the potential victim identification workload, as the priority for scarce technological resources is assigned to human remains whose conditions preclude use of conventional methods of body identification.
By relying on conventional evidence in all the situations in which conventional methods have the potential to be highly accurate, the need for more costly, time-consuming, and labor-intensive technological techniques of identification is reduced. This resource reduction improves the overall efficiency of the DVI process. Using these considerations, the DVI process flow inside the morgue facility in the current DVI model was modified to incorporate these DST process-improvement strategies. This next-generation DVI process varies from the single-step decision-making process depicted in Figure 1. As illustrated in Figure 8, the proposed DVI process increases the number of paths through which a body may pass during its PM examination. The next-generation DVI process incorporates two distinct decision points, thus becoming a sequential-decision process.

Figure 8. The Next-Generation Conceptual Disaster Victim Identification (DVI) Decision-Making: A Sequential Decision Process.
A major advantage provided by this model is that many sets of intact human remains can bypass the slowest throughput stations in the PM arm of the model, thus improving the efficiency of body identification in mass fatality incidents. When the PM evidence collected from the human remains meets the criteria for conventional stations only (at the initial decision point or first assessment in Figure 8), the morgue path for that body may skip the collection stations for technological PM evidence and continue directly to the data comparison and reconciliation phase. If a match is found that confidently demonstrates the body's identification (second decision point or second assessment), a final decision can be confirmed, avoiding unnecessary waiting time for technological AM records that are not critical for establishing the identity of that victim.
Another benefit is the redistribution of the workload on the different stations in the morgue. The overall effect is to decrease the need for scarce technological resources by reducing the number of bodies examined in those stations and consequently the amount of data processed. It also avoids the undesirably redundant PM examinations and, by promoting overall process efficiency, possibly increases the quality of the collected data. This redistribution of the workload also may relieve some of the personnel fatigue noted by forensic experts during mass fatality incidents.Reference Lain, Griffiths and Hilton8 Reducing this “burnout” for forensics personnel is important, as it is noted to be a prominent factor contributing to the error rate in PM data collection.Reference Kieser, Laing and Herbison28
Implementing this decision-making model may also have a positive effect on AM-PM data reconciliation. This phase is one of the most complex and time consuming in the DVI process.7 During this phase, PM and AM data are analyzed and compared to find possible matches. The selective reduction in the number of technological examinations may control the amount of data that has to be processed and compared to establish the identity of a victim.
In a well-designed and managed DVI system, any processing delay for technological data analysis may be justifiable only when the condition of the unknown human remains requires advanced methods for accurate identification: when the DVI's first assessment (see Figure 8) does not meet the designated criteria and “collection-phase II” is required. These considerations were used to develop the proposed next-generation DVI system (Figure 9), which incorporates the decision-making process shown in Figure 8. The order of sequence that the bodies transit in the morgue stations is redesigned from the model in Figure 2. The number of pathways that each body could follow during its PM examination is now increased; the actual path for each body is determined through the sequential decision steps.

Figure 9. The Proposed Next-Generation Disaster Victim Identification (DVI) System.
Human remains, after entering the morgue, are first examined through a defined series of conventional stations. This examination sequence starts with the photography of the remains and any personal effects. (Photography is included in the typology of conventional stations, because of its wide availability, ease of operation, and relatively low cost for basic equipment such as digital cameras.) The photographs are collected and cataloged, and the process is continued with the external examination of the victim's remains to gather the relevant external physical evidence; data are directly recorded into the PM record. After the conventional examination, the human remains may be returned to the holding area or may continue with analyses in the technological stations if the collected conventional evidence does not provide a strong basis for establishing the victim's identity within an acceptable level of uncertainty.
CONCLUSIONS
Our findings demonstrate that the developed DST, applied under controlled circumstances, can decrease the uncertainty associated with presumptive identification established using conventional evidence. Accuracy is accomplished through enhanced objectivity and reproducibility in collecting and recording the conventional evidence, and through the careful methodology followed in processing the data through the comparison and reconciliation phase of the DVI process. The findings also suggest that when conventional methods of body identification are as rigorously controlled, and findings are as objectively captured and processed as with technological methods, the uncertainty in identifying human remains from conventional methods may become comparable to the level associated with most technological methodologies.
Although this study did not address the use of the personal effects of deceased victims in the identification process, an approach similar to that presented for conventional physical features may be helpful for this forensic evidence. This methodology may be particularly valuable in the exhumation of mass graves, where clothing and personal effects become very important in expediting body identification.Reference Komar29Reference Djuric, Dunjic, Djonic and Skinner30
The proposed next-generation DVI system, as presented here, may markedly improve the speed and efficiency of the DVI process in selected mass fatality situations, without sacrificing accuracy. Field studies are indicated to further assess this approach.