Autism spectrum disorder (ASD) is behaviorally defined by the presence of social communication deficits and restricted and repetitive behaviors. While these core behavioral domains provide a loose structure to the behavioral phenotype of ASD, the constellation and severity of behavioral symptoms and associated features exhibited by affected individuals varies greatly (Kim, Macari, Koller, & Chawarska, Reference Kim, Macari, Koller and Chawarska2016; Veenstra-VanderWeele & Blakely, Reference Veenstra-VanderWeele and Blakely2012). This phenotypic heterogeneity is reflected in the increasingly complex genetics associated with autism, an evolving landscape that includes de novo and inherited risk factors and multiple rare and common variants (Geschwind & State, Reference Geschwind and State2015). Amid the complexity and variation in the outward manifestation of ASD, as well as in its genetic architecture, is the acknowledgment that fundamentally, it is a disorder of neurodevelopment. Although diagnosed by virtue of the presence or absences of set behavioral indicators, autism is biologically based and arises from an altered trajectory of brain development that begins very early in ontogeny.
The developmental window in which ASD unfolds is a relatively narrow one, affording researchers the opportunity to isolate developmental events leading to its onset. Until recently, however, the emergence of autism eluded direct study (Landa & Garrett-Mayer, Reference Landa and Garrett-Mayer2006; Zwaigenbaum et al., Reference Zwaigenbaum, Bryson, Rogers, Roberts, Brian and Szatmari2005). Approaches to understanding how the disorder developed early in life predominantly relied on retrospective methods, or inferences based on studies of newly diagnosed toddlers and preschoolers, and even conjecture based on findings from older children or adults. These approaches advanced knowledge but were ultimately limited in their ability to capture the unique and often subtle events that preceded a diagnosis. With regard to the brain, retrospective methods were confined in large part to records of head circumference (Lainhart et al., Reference Lainhart, Piven, Wzorek, Landa, Santangelo, Coon and Folstein1997; Woodhouse et al., Reference Woodhouse, Bailey, Rutter, Bolton, Baird and Le Couteur1996). This left the possibility of generalizing brain findings from older individuals to inform pathogenesis, an inferential leap fraught with error given that brain development is inherently dynamic and nonlinear (e.g., Raznahan et al., Reference Raznahan, Shaw, Lalonde, Stockman, Wallace, Greenstein and Giedd2011). For example, studying brain features in young adults with ASD may speak to cumulative effects specific to that period of life, but offers little insight into the etiology. While the relevance of age effects on the neurodevelopment of ASD have been long appreciated (Courchesne et al., Reference Courchesne, Karns, Davis, Ziccardi, Carper, Tigue and Courchesne2001; Hoshino, Manome, Kaneko, Yashima, & Kumashiro, Reference Hoshino, Manome, Kaneko, Yashima and Kumashiro1984), the events of the first years of life were, until relatively recently, largely matter for speculation.
The past 15 years have seen significant gains in the number of magnetic resonance imaging (MRI) studies focused on ASD in infancy and toddlerhood. This growth in the published literature is due in part to improved methods for acquiring MRI data from young children (Dean et al., Reference Dean, Dirks, O'Muircheartaigh, Walker, Jerskey, Lehman and Deoni2014; Nordahl et al., Reference Nordahl, Simon, Zierhut, Solomon, Rogers and Amaral2008) as well as technological advances in image processing (Goodlet, Fletcher, Gilmore, & Gerig, Reference Goodlett, Fletcher, Gilmore and Gerig2009). Another source of growth comes from the prospective study of infant siblings of older children with ASD, or so-called baby sibs design. Approximately 10%–20% of younger siblings of children with ASD will themselves develop the disorder (Messinger et al., Reference Messinger, Young, Webb, Ozonoff, Bryson, Carter and Zwaigenbaum2015; Ozonoff et al., Reference Ozonoff, Young, Carter, Messinger, Yirmiya, Zwaigenbaum and Stone2011; Sandin et al., Reference Sandin, Lichtenstein, Kuja-Halkola, Larsson, Hultman and Reichenberg2014), providing researchers with a practical means of charting its development over the first years of life by following infants at high familial risk over time. Studies employing this approach have provided critical insights into the behavioral development of ASD, including data suggesting that core social communicative features emerge in the second year of life (Hudry et al., Reference Hudry, Chandler, Bedford, Pasco, Gliga, Elsabbagh and Charman2014; Ozonoff et al., Reference Ozonoff, Iosif, Baguio, Cook, Hill, Hutman and Young2010; Zwaigenbaum et al., Reference Zwaigenbaum, Bryson, Rogers, Roberts, Brian and Szatmari2005) and may be preceded by more subtle associated features during infancy (Elison et al., Reference Elison, Paterson, Wolff, Reznick, Sasson, Gu and Piven2013; Elsabbagh et al., Reference Elsabbagh, Mercure, Hudry, Chandler, Pasco and Charman2012; Flanagan, Landa, Bhat, & Bauman, Reference Flanagan, Landa, Bhat and Bauman2012; Jones & Klin, Reference Jones and Klin2013; Shic, Macari, & Chawarska, Reference Shic, Macari and Chawarska2014). The disorder is not yet discernable at birth or even in later infancy, nor does it appear abruptly as with the flip of a switch when a child reaches a certain age. Instead, it entails a dynamic and likely constructive process, a true journey wherein multiple facets of vulnerability aggregate into the phenotype of ASD over time. The developing brain is integral to this pathogenic process, and elucidating its role prior to the consolidation of behavioral symptoms may be the key to discovering how the disorder might be detected prior to consolidation and ameliorated through early intervention or strategic prevention.
What follows is a review and discussion of recent neuroimaging studies of autism focused on the first years of life. Our purpose in so doing was to identify and synthesize themes within the published literature that inform issues of timing related to proximal mechanisms of the symptom profiles that define autism. The corpus of studies reviewed herein was identified through systematic searches of PubMed and Google Scholar using variations related to autism (autism OR autistic), infancy or toddlerhood (AND infants OR infancy OR toddlers OR early), and variations related to brain imaging (AND magnetic resonance OR MRI OR brain imaging OR resting state OR diffusion OR DTI [diffusion tensor imaging] OR fMRI [functional MRI]). To supplement, we also reviewed the reference sections of recently published reports to ensure adequate coverage of the literature. Studies identified through this process are presented and briefly described in Table 1. We acknowledge that electrophysiology and other imaging methods (e.g., near infrared spectroscopy) implemented during this developmental period contribute to our understanding of neural function, but are beyond the scope of this review.
Table 1. Neuroimaging studies of ASD during infancy and toddlerhood
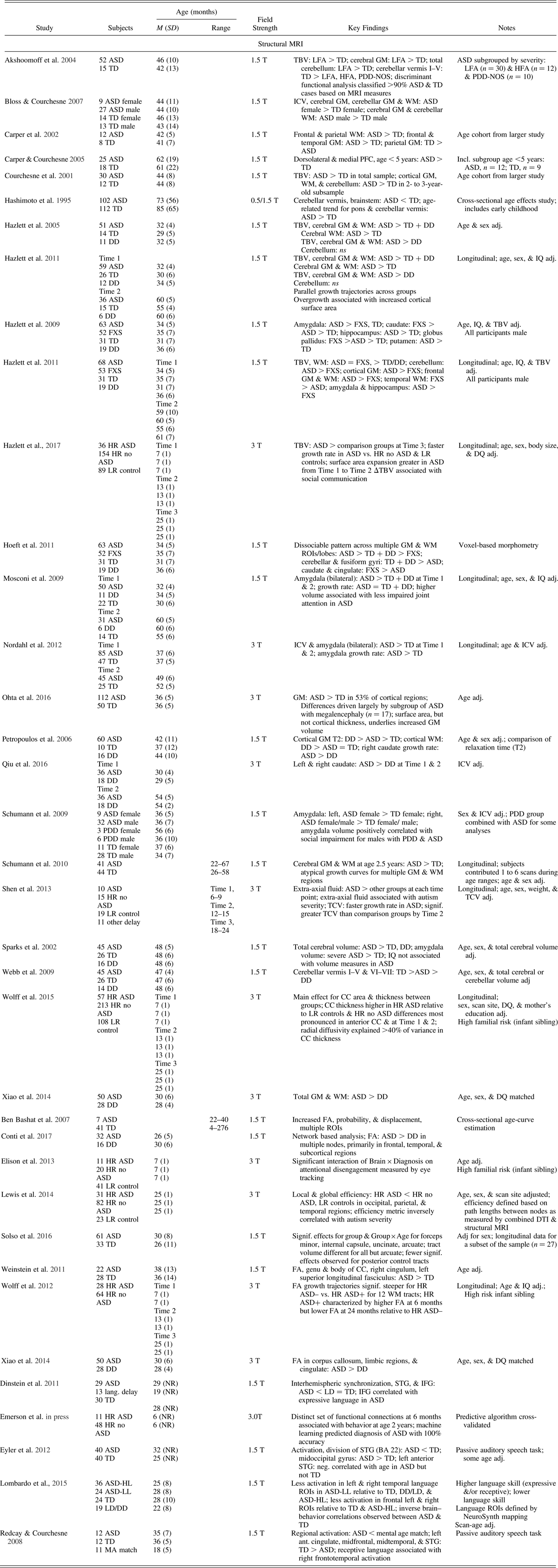
Note: Adapted from “On the Emergence of Autism: Neuroimaging Findings From Birth to Pre-School,” by J. J. Wolff and J. Piven, Reference Wolff and Piven2013, Neuropsychiatry, 3, 209–222. Copyright 2013 by Open Access Journals. Adapted with permission. ASD, autism spectrum disorder; BA, Broadmann area; DD, developmentally delayed; DQ, developmental quotient; FA, fractional anisotropy; FXS, fragile X syndrome; GM, grey matter; HFA, high functioning autism; HL, higher language; HR, high risk; ICV, intercranial volume; IFG, inferior frontal gyrus; LFA, low functioning autism; LL, lower language; LR, low risk; MRI, magnetic resonance imaging; PDD-NOS, pervasive developmental disorder, not otherwise specified; PFC, prefrontal cortex; ROI, region of interest; STG, superior temporal gyrus; TBV, total brain volume; TD, typically developing control; WM, white matter.
Brain Structure
Cerebral volume
The observation that brain structure may be grossly altered in ASD harkens back to Leo Kanner's initial work describing 11 cases, wherein he noted simply that “five had relatively large heads” (Reference Kanner1943, p. 248). Over 50 years later, Kanner's succinct but certainly prescient observation was substantiated through several independent studies of head circumference in children and adults with autism (Davidovitch, Patterson, & Gartside, Reference Davidovitch, Patterson and Gartside1996; Lainhardt et al., Reference Lainhart, Piven, Wzorek, Landa, Santangelo, Coon and Folstein1997; Woodhouse et al., Reference Woodhouse, Bailey, Rutter, Bolton, Baird and Le Couteur1996). In the first MRI studies to identify generalized brain overgrowth in ASD, Piven et al. observed increased total midsagittal brain area (Reference Piven, Nehme, Simon, Barta, Pearlson and Folstein1992) and total brain volume (Reference Piven, Arndt, Bailey, Havercamp, Andreasen and Palmer1995) in adult males with autistic disorder. Although these studies of adults, along with several contemporaries focused on substructures, provided evidence suggesting atypical brain development associated with autism, they were significantly limited in their ability to speak to pathogenesis.
In the first imaging study of early brain development in ASD, Courchesne et al. (Reference Courchesne, Karns, Davis, Ziccardi, Carper, Tigue and Courchesne2001) identified increased whole-brain volume in a small subsample of 2- to 4-year old children with autism relative to controls. Total brain volume differences extended to both cerebral white and gray matter, with both regions showing cross-sectional age effects whereby increased volumes were evident in autism prior to school age but not thereafter. This prompted the authors to suggest that autism might be characterized by early brain overgrowth followed by slowed growth into later childhood. Follow-up work suggested that this pattern was evident across frontal, parietal, and temporal lobes (Carper, Moses, Tigue, & Courchesne, Reference Carper, Moses, Tigue and Courchesne2002), with the frontal lobe showing the strongest effects by preschool and early school age, particularly in the dorsolateral prefrontal cortex (Carper & Courchesne, Reference Carper and Courchesne2005). Although tempered by relatively small sample sizes and reliance on cross-sectional versus longitudinal data, this series of findings indicated that brain overgrowth could be an early neural signature of ASD. This raised interest in the study of head circumference as a surrogate marker of brain growth and early risk for the disorder (e.g., Courchesne, Carper, & Akshoomoff, Reference Courchesne, Carper and Akshoomoff2003). Recent work comparing children with ASD to community controls, as opposed to national norms, has demonstrated that head circumference may not be suitable for these purposes (Raznahan et al., Reference Raznahan, Wallace, Antezana, Greenstein, Lenroot, Thurm and Giedd2013; Zwaigenbaum et al., Reference Zwaigenbaum, Young, Stone, Dobkins, Ozonoff, Brian and Messinger2014).
The MRI finding of early brain overgrowth associated with ASD has been reported by several independent research groups. For example, Sparks et al. (Reference Sparks, Friedman, Shaw, Aylward, Echelard, Artru and Dager2002) found that cerebral volumes were significantly increased in 3- to 4-year-old children with ASD relative to both typically developing children and children with developmental delay. The finding that children with ASD differed from those with developmental delay is notable as it provided some evidence that brain overgrowth in ASD may be disorder specific and not a function of intellectual disability, a position supported by follow-up work examining differences in T2 relaxation times (Petropoulos et al., Reference Petropoulos, Friedman, Shaw, Artru, Dawson and Dager2006). Bloss and Courchesne (Reference Bloss and Courchesne2007) examined sex differences in 3-year-olds with ASD relative to controls, identifying that both girls and boys with ASD showed increased total brain and gray matter volumes. In a combined brain imaging and head circumference study, Hazlett et al. (Reference Hazlett, Poe, Gerig, Smith, Provenzale, Ross and Piven2005) downward extended previous work to toddlers, finding that those with ASD were characterized by significantly greater cortical white and gray matter volumes relative to typically developing children as well as children with developmental delay. Retrospective head circumference data suggested that children with ASD followed a head growth trajectory similar to that of typically developing community controls through infancy, showing divergence and overgrowth starting around age 12 months. In a study of toddler and preschool age boys, Hoeft et al. (Reference Hoeft, Walter, Lightbody, Hazlett, Chang, Piven and Reiss2011) found that children with idiopathic autism showed volume increases in frontal, temporal, and subcortical regions relative to typically developing and developmentally delayed controls, as well as children with fragile X syndrome. In a study of toddlers with ASD and an age, sex, and developmental quotient matched control group, Xiao et al. (Reference Xiao, Qiu, Ke, Xiao, Xiao, Liang and Liu2014) also found significantly elevated gray and white matter volumes in those with ASD. As expected, there are also findings that diverge from this pattern. Nordahl et al. (Reference Nordahl, Scholz, Yang, Buonocore, Simon, Rogers and Amaral2012) reported increased brain volume in preschool-age boys with ASD who had experienced a regression according to parent report. However, boys who did not experience regression showed similar brain volume to typically developing controls. It is highly unlikely that the phenomenon of regression would be limited only to simplex cases, and it is unclear whether brain development differs among infant siblings who experience regression. That said, prospective longitudinal studies of high-risk infants (multiplex cases) have not provided conclusive evidence of observed acute skill loss (Brian et al., Reference Brian, Bryson, Smith, Roberts, Roncadin, Szatmari and Zwaigenbaum2016).
Several longitudinal imaging studies have provided important details on trajectories of brain growth in children with ASD over the first years of life. In a report on brain volume changes in children with and without ASD from toddlerhood through age 5, Schumann et al. (Reference Schumann, Bloss, Barnes, Wideman, Carper, Akshoomoff and Courchesne2010) noted significant differences in growth trajectories for total cerebral volume as well as several cortical regions, including the parietal and cingulate cortices. For the majority of gray and white matter regions reported, growth trajectories for children with ASD were elevated across the age range relative to controls. Hazlett et al. (Reference Hazlett, Poe, Gerig, Styner, Chappell, Smith and Piven2011) identified a similar pattern, with total gray and white matter volumes elevated and stable across ages 2 to 4 in children with ASD relative to typically developing and developmentally delayed controls. Volumetric differences observed between groups were driven by surface area and not cortical thickness, a finding recently replicated by Ohta et al. (Reference Ohta, Nordahl, Iosif, Lee, Rogers and Amaral2016). With two longitudinal imaging studies suggesting that brain overgrowth was already in place by age 2 (Hazlett et al., Reference Hazlett, Poe, Gerig, Styner, Chappell, Smith and Piven2011; Schumann et al., Reference Schumann, Bloss, Barnes, Wideman, Carper, Akshoomoff and Courchesne2010) and head circumference data suggesting that those with ASD might first diverge at age 1 (Hazlett et al., Reference Hazlett, Poe, Gerig, Smith, Provenzale, Ross and Piven2005), a critical next step was to downward extend the study of brain growth in ASD to infancy.
Shen et al. (Reference Shen, Nordahl, Young, Wootton-Gorges, Lee, Liston and Amaral2013) reported data from a prospective, longitudinal study of infants at high familial risk for ASD, who are designated as such by virtue of having an older sibling with the disorder (baby sibs design). High-risk participants, along with a low-risk control group, were followed from age 6 months to age 24 and 36 months, at which times they received a diagnostic assessment. The authors found that total cerebral volume in high-risk infants who developed ASD (HR-ASD; n = 10) did not differ from high-risk infants who did not or from typically developing controls at age 6 months. However, by age 12 months, group differences emerged, with HR-ASD showing increased volume relative to comparison groups. The finding that brain volume differences were not present at age 6 months but emerged by age 12 months was also recently reported by Hazlett et al. (Reference Hazlett, Gu, Munsell, Kim, Styner, Wolff and Piven2017), who tracked development in 435 infants at 6, 12, and 24 months. Of these, 148 contributed data at each time point (HR-ASD = 15, high risk without an ASD diagnosis = 91, low-risk controls = 42). The authors identified increased rates of surface area expansion from age 6 to 12 months, followed by increased rate of total brain volume from 12 to 24 months. These findings directly support the hypotheses generated from previous work (Hazlett et al., Reference Hazlett, Poe, Gerig, Styner, Chappell, Smith and Piven2011) suggesting that surface area expansion underlies brain overgrowth in ASD. A machine learning approach to diagnostic classification, applied to features of surface area growth from 6 to 12 months, predicted diagnostic outcome at age 24 months with 88% sensitivity and 95% specificity (Hazlett et al., Reference Hazlett, Gu, Munsell, Kim, Styner, Wolff and Piven2017).
Cerebellum
While findings pertaining to cerebral cortical volumes have generally converged across studies, results for the cerebellum have been less consistent. In a study of the cerebellum and brain stem in individuals with autism ages 6 months to 20 years, Hashimoto et al. (Reference Hashimoto, Tayama, Murakawa, Yoshimoto, Miyazaki, Harada and Kuroda1995) identified that these structures were significantly smaller in comparison to typically developing controls. This result was consistent for the subgroup of participants who were under the age of 4 (n = 53). The authors also noted differences in cross-sectional age effects for the pons and most cerebellar lobules. In a study of 3- to 4-year-olds, Webb et al. (Reference Webb, Sparks, Friedman, Shaw, Giedd, Dawson and Dager2009) found that, when adjusted for total cerebral volume, children with ASD were intermediate to typically developing (TD) controls and children with developmental delay for vermis I–X, such that TD > ASD > developmental delay. Bloss and Courchesne (Reference Bloss and Courchesne2007) identified significantly elevated cerebellar white matter volume in girls and boys with ASD relative to TD controls, as well as lower cerebellar gray matter volume in girls with ASD only. Akshoomoff et al. (Reference Akshoomoff, Lord, Lincoln, Courchesne, Carper, Townsend and Courchesne2004) also reported increased cerebellar white matter as well as enlargement of the anterior lobe in 2- to 5-year-old children with ASD relative to TD controls. Several others, however, have reported no differences in cerebellum volumes when total brain or cerebral volume is accounted for (Hazlett et al., Reference Hazlett, Poe, Gerig, Smith, Provenzale, Ross and Piven2005, Reference Hazlett, Poe, Gerig, Styner, Chappell, Smith and Piven2011; Sparks et al., Reference Sparks, Friedman, Shaw, Aylward, Echelard, Artru and Dager2002), suggesting that the cerebellum may not be uniquely enlarged in young children with ASD.
Subcortical structures
Relatively fewer brain imaging studies of young children with ASD have focused on subcortical structures. This may be due in part to the unique methodological challenges associated with tissue segmentation in the early developing brain, such as indeterminate white-gray boundaries or the lack of off-the-shelf processing tools specific to pediatric scan data. In a study of 3- to 4-year-olds with ASD, Sparks et al. (Reference Sparks, Friedman, Shaw, Aylward, Echelard, Artru and Dager2002) identified enlargement of both the bilateral hippocampus and the amygdala. When the authors modeled the data accounting for total cerebral volume, only the amygdala remained enlarged, and only in the subgroup of children with more severe autism. In a longitudinal follow-up of these children, increased right amygdala volume at 3–4 was associated with poorer social and communicative outcome at age 6 (Munson et al., Reference Munson, Dawson, Abbott, Faja, Webb, Friedman and Dager2006). No predictive associations were reported for the hippocampus. Schumann, Barnes, Lord, and Courchesne (Reference Schumann, Barnes, Lord and Courchesne2009) also reported evidence of amygdala enlargement in ASD, further noting that this increase was most pronounced in girls with ASD relative to controls. The authors also reported that increased amygdala volume was associated with greater severity of social and communication deficits. In a longitudinal study of toddlers with ASD, Mosconi et al. (Reference Mosconi, Cody-Hazlett, Poe, Gerig, Gimpel-Smith and Piven2009) identified that amygdala enlargement was present and stable from ages 2 to 4 relative to TD controls. In contrast to brain–behavior associations reported by Schuman et al. (Reference Schumann, Barnes, Lord and Courchesne2009), Mosconi et al. found that amygdala volume was positively associated with better joint attention outcomes among children with ASD. In a study of several subcortical structures, Hazlett et al. (Reference Hazlett, Poe, Lightbody, Gerig, Macfall, Ross and Piven2009) also reported that the amygdala was 20% larger in toddlers with ASD relative to TD controls. The authors further identified that volume was increased in the hippocampus as well as components of the basal ganglia, including the caudate nucleus, globus pallidus, and putamen. Qiu et al. (Reference Qiu, Chang, Li, Qian, Xiao, Xiao and Ke2016) recently reported findings from a longitudinal study showing that both the left and right caudate were enlarged in ASD relative to a developmentally delayed control group from ages 2 to 4 years, with the right caudate showing a significantly faster growth rate in ASD.
Other structural segmentations
In their prospective, longitudinal study of infants at risk for ASD, Shen et al. (Reference Shen, Nordahl, Young, Wootton-Gorges, Lee, Liston and Amaral2013) measured volumes of extra-axial fluid, defined as cerebral spinal fluid occupying the subarachnoid space, at ages 6, 12, and 24 months. The results indicated that extra-axial fluid volume was significantly elevated in HR-ASD across ages 6, 12, and 24 months of age relative to high-risk infants without ASD and low-risk controls. Using automated methods in a sample size five times larger than the initial report, Shen et al. (Reference Shen, Kim, McKinstry, Gu, Hazlett, Nordahl and Piven2017) replicated this result. They also showed that extra-axial fluid was disproportionately increased in more severely affected children. In another prospective, longitudinal study of high-risk infants, Wolff et al. (Reference Wolff, Gerig, Lewis, Soda, Styner, Vachet and Piven2015) reported on trajectories of midsagittal corpus callosum morphometry across ages 6, 12, and 24 months of age. They found that corpus callosum area and thickness were significantly higher in infants who received a diagnosis of ASD at age 2 years. Observed increases in thickness were most prominent in the anterior region of the corpus callosum, and effects were strongest at ages 6 and 12 months. The authors also noted that measures of area and thickness were significantly associated with degree of restricted and repetitive behavior in participants with ASD.
Discussion of structural findings
The most consistent finding to emerge from structural MRI studies is that total brain or cerebral volume is significantly elevated in toddlers who develop ASD (Courchesne et al., Reference Courchesne, Karns, Davis, Ziccardi, Carper, Tigue and Courchesne2001; Hazlett et al., Reference Hazlett, Poe, Gerig, Smith, Provenzale, Ross and Piven2005, Reference Hazlett, Poe, Gerig, Styner, Chappell, Smith and Piven2011, Reference Hazlett, Gu, Munsell, Kim, Styner, Wolff and Piven2017; Schumann et al., Reference Schumann, Bloss, Barnes, Wideman, Carper, Akshoomoff and Courchesne2010; Sparks et al., Reference Sparks, Friedman, Shaw, Aylward, Echelard, Artru and Dager2002). Volume differences have been reported for both white and gray matter, though more frequently for the latter. Recent findings that include data on infants suggest that brain growth in children who develop autism begins to diverge from a typical trajectory between 6 and 12 months of age, and that this process may be initially driven by rate of cortical surface area expansion (Hazlett et al., Reference Hazlett, Gu, Munsell, Kim, Styner, Wolff and Piven2017). The specific connection to surface area (Lui, Hansen, & Kriegstein, Reference Lui, Hansen and Kriegstein2011) suggests that an altered process of neuronal progenitor cell proliferation, differentiation, and migration may underlay macrostructural effects (Piven, Elison, & Zylka, Reference Piven, Elison and Zylkain press). There is evidence implicating progenitor cells from studies of human iPS cells derived from individuals with idiopathic ASD (Marchetto et al., Reference Marchetto, Belinson, Tian, Freitas, Fu, Vadodaria and Muotri2016) as well as mouse models of genetic conditions linked to autism (Pucilowska et al., Reference Pucilowska, Vithayathil, Tavares, Kelly, Karlo and Landreth2015). While cerebral overgrowth may not be a uniform feature of ASD, findings to date have converged in describing the timing and potentially the mechanism underlying one specific pathogenic process culminating in ASD. This work has provided new inroads for the presymptomatic detection of ASD (Hazlett et al., Reference Hazlett, Gu, Munsell, Kim, Styner, Wolff and Piven2017) as well as the possibility of reversing its effects on neural architecture (Marchetto et al., Reference Marchetto, Belinson, Tian, Freitas, Fu, Vadodaria and Muotri2016).
Several studies have also identified early differences in subcortical and other noncerebral structures. The most commonly reported subcortical finding is that of amygdala overgrowth in toddlers with ASD. It has been suggested that overgrowth of this structure may be unique to the early development of the disorder (Schumann & Amaral, Reference Schumann and Amaral2006), contributing to the atypical scaffolding of fundamental social and emotional skills (Sparks et al., Reference Sparks, Friedman, Shaw, Aylward, Echelard, Artru and Dager2002). This interpretation is in line with studies finding no differences or even reduced amygdala volumes in adults with ASD (Nacewicz et al., Reference Nacewicz, Dalton, Johnstone, Long, McAuliff and Oakes2006; Schumann & Amaral, Reference Schumann and Amaral2006) and is supported by work highlighting differential age effects (Nordahl et al., Reference Nordahl, Scholz, Yang, Buonocore, Simon, Rogers and Amaral2012; Schumann et al., Reference Schumann, Hamstra, Goodlin-Jones, Lotspeich, Kwon, Buonocore and Amaral2004). There are also data suggesting that overgrowth extends to other subcortical structures, such as the caudate nucleus (Hazlett et al., Reference Hazlett, Poe, Lightbody, Gerig, Macfall, Ross and Piven2009; Petropoulos et al., Reference Petropoulos, Friedman, Shaw, Artru, Dawson and Dager2006; Qiu et al., Reference Qiu, Chang, Li, Qian, Xiao, Xiao and Ke2016). This structure, along with others comprising the basal ganglia, have been associated with restricted and repetitive behaviors among preschool-age children with ASD (Estes et al., Reference Estes, Shaw, Sparks, Friedman, Giedd, Dawson and Dager2011; Qiu et al., Reference Qiu, Chang, Li, Qian, Xiao, Xiao and Ke2016; Wolff, Hazlett, Lightbody, Reiss, & Piven, Reference Wolff, Hazlett, Lightbody, Reiss and Piven2013). One report suggests that the corpus callosum may also be associated with repetitive behaviors in children with ASD during infancy and toddlerhood, and that this structure is significantly thicker across the 6- to 24-month age interval (Wolff et al., Reference Wolff, Gerig, Lewis, Soda, Styner, Vachet and Piven2015). There are also now two reports on separate study samples indicating that extra-axial cerebral spinal fluid is elevated during infancy and toddlerhood in children who develop ASD (Shen et al., Reference Shen, Nordahl, Young, Wootton-Gorges, Lee, Liston and Amaral2013, Reference Shen, Kim, McKinstry, Gu, Hazlett, Nordahl and Piven2017). This intriguing finding suggests that the transport of waste or cytokines, and possibly the interaction of cerebrospinal fluid with the lymphatic system (Louveau et al., Reference Louveau, Smirnov, Keyes, Eccles, Rouhani, Peske and Kipnis2015), may be disrupted. While atypical cerebellar features have been consistently identified in studies of older individuals with ASD (Fatemi et al., Reference Fatemi, Aldinger, Ashwood, Bauman, Blaha, Blatt and Welsh2012), whether and how it is associated with the disorder early in life is less clear (Bloss & Courchesne, Reference Bloss and Courchesne2007; Hazlett et al., Reference Hazlett, Poe, Gerig, Smith, Provenzale, Ross and Piven2005, Reference Hazlett, Poe, Gerig, Styner, Chappell, Smith and Piven2011).
Diffusion MRI
Diffusion-weighted MRI (DW-MRI) is a specialized form of imaging sensitive to the displacement of water molecules through body tissue (Mori & Zhang, Reference Mori and Zhang2006). Based on the magnitude with which these molecules diffuse along multiple field gradients, the microstructural properties of tissue at the voxel level may be estimated given that diffusion rate is influenced by tissue structure. DTI is a DW-MRI method that accounts for directionality within each three-dimensional voxel. In the brain, this form of imaging is typically applied to the study of white matter architecture to characterize the structural properties of local or long-range connectivity. Common measures derived from DTI data include axial and radial diffusivity, representing diffusion along and orthogonal to the principle eigenvector, respectively; mean diffusivity; and fractional anisotropy (FA), which reflects degree of diffusion along the principle eigenvector relative to other directions. Rather than characterizing any one aspect of underlying structure, these measures are influenced by multiple facets of tissue composition, including but not limited to axon size, packing density, fiber cohesion, and myelination (Jones, Knösche, & Turner, Reference Jones, Knösche and Turner2013).
There has been sustained interest in the potential role that disrupted or atypical neural connectivity may play in the development of ASD, and diffusion MRI has offered a means to interrogate this issue. To date, however, only a small fraction of published DTI studies of ASD have focused on the first years of life (Travers et al., Reference Travers, Adluru, Ennis, Tromp, Destiche, Doran and Alexander2012). In a cross-sectional study of 7 toddlers with ASD, Ben Bashat et al. (Reference Ben Bashat, Kronfeld-Duenias, Zachor, Ekstein, Hendler, Tarrasch and Ben Sira2007) identified higher FA in those with ASD relative to typically developing children. Effects were observed across multiple fiber pathways including several subdivisions of the corpus callosum, the corticospinal tract, and both the external and internal capsule. Increased FA in children with ASD was also reported by Weinstein et al. (Reference Weinstein, Ben-Sira, Levy, Zachor, Ben Itzhak, Artzi and Ben Bashat2011) in a study of 21 toddler and preschool-age children with ASD relative to typically developing controls. Using tract-based spatial statistics, they identified significantly increased FA in the area of the cingulum, corpus callosum, and superior longitudinal fasciculus. In a study of toddlers with ASD and an age, sex, and developmental quotient matched control group, Xiao et al. (Reference Xiao, Qiu, Ke, Xiao, Xiao, Liang and Liu2014) reported significantly increased FA in the several regions using voxel-based analysis including the area of the corpus callosum, limbic system, and cingulate cortex. Recently, Conti et al. (Reference Conti, Mitra, Calderoni, Pannek, Shen, Pagnozzi and Guzzetta2017) applied a network-based analysis to diffusion data in a study of 32 toddlers with ASD and a comparison group of 16 toddlers with broadly defined developmental delay. The authors identified significantly increased FA in the ASD group, particularly in voxels linking frontal, temporal, and subcortical regions. The authors also reported differences in number of streamlines between regions; however, there is some question as to the validity of such data (e.g., Jones et al., Reference Jones, Knösche and Turner2013).
Two longitudinal DTI studies have elucidated the finding of increased FA associated with ASD, identifying both increased FA and slower development of white matter pathways in ASD. In a study of 92 infants at high familial risk for ASD across ages 6, 12, and 24 months, Wolff et al. (Reference Wolff, Gu, Gerig, Elison, Styner, Gouttard and Piven2012) found significant differences in growth trajectories for 12 of 15 fiber pathways between infants who did and did not receive a diagnosis of ASD at age 2. Trajectories for the group who developed ASD were nearly uniformly characterized by a pattern of increased FA at age 6 months followed by slower growth thereafter. In a study of 1- to 4-year-olds with and without ASD, Solso et al. (Reference Solso, Xu, Proudfoot, Hagler, Campbell, Venkatraman and Courchesne2016) identified significant group and Group × Age effects for FA in several white matter pathways, including the corpus callosum, uncinate fasciculus, and arcuate fasciculus. Overall, differences were most evident in frontal versus posterior white matter. The authors also reported significant differences in fiber pathway volumes based on voxel count. For both FA and volume, the developmental pattern was similar to that reported by Wolff et al. (Reference Wolff, Gu, Gerig, Elison, Styner, Gouttard and Piven2012), with the ASD group higher initially but showing slower growth over time.
Several studies have utilized diffusion MRI data to examine other aspects structural connectivity, including its relation to behavior. Lewis et al. (Reference Lewis, Evans, Pruett, Botteron, Zwaigenbaum, Estes and Piven2014) used DW-MRI data to estimate properties of network efficiency in a study of 2-year olds at high and low familial risk for ASD. They found evidence of decreased local and global efficiency among high-risk children with ASD relative to high-risk children without ASD and low-risk controls. Differences were most evident in the occipital and temporal regions, and to a lesser extent in the parietal lobe. The authors also reported an inverse relationship between measures of efficiency and autism symptom severity as indexed by the Autism Diagnostic Observation Schedule. In another study of children at low and high familial risk for ASD using both DTI and eye tracking, Elison et al. (Reference Elison, Paterson, Wolff, Reznick, Sasson, Gu and Piven2013) found that functional coupling between visual orienting and the splenium of the corpus callosum apparent among low-risk controls was not observed in 7-month-old infants who later developed ASD. Wolff et al. (Reference Wolff, Swanson, Elison, Gerig, Pruett and Styner2017) recently reported that FA in cerebellar and corpus callosum white matter pathways from 6 to 24 months was positively associated with restricted and repetitive behaviors, as well as responsiveness to sensory stimuli, in toddlers with ASD. The brain–behavior relations identified by Wolff et al. were relatively specific, and did not extend to other fiber pathways or to social communication.
Discussion of diffusion MRI findings
Relative to structural MRI, diffusion MRI is a newer and thereby less established approach to brain imaging. Nonetheless, there are converging findings across studies suggestive of effects specific to the early development of autism. Foremost among these findings is that of increased FA in infants and toddlers who either have or are later diagnosed with ASD (Ben Bashat et al., Reference Ben Bashat, Kronfeld-Duenias, Zachor, Ekstein, Hendler, Tarrasch and Ben Sira2007; Conti et al., Reference Conti, Mitra, Calderoni, Pannek, Shen, Pagnozzi and Guzzetta2017; Solso et al., Reference Solso, Xu, Proudfoot, Hagler, Campbell, Venkatraman and Courchesne2016; Weinstein et al., Reference Weinstein, Ben-Sira, Levy, Zachor, Ben Itzhak, Artzi and Ben Bashat2011; Wolff et al., Reference Wolff, Gu, Gerig, Elison, Styner, Gouttard and Piven2012; Xiao et al., Reference Xiao, Qiu, Ke, Xiao, Xiao, Liang and Liu2014). The finding of increased FA, consistent across six DTI studies of ASD, is in distinct contrast with what has been commonly reported among older children and adults with the disorder, wherein FA is relatively lower in individuals with a diagnosis (Travers et al., Reference Travers, Adluru, Ennis, Tromp, Destiche, Doran and Alexander2012). Based on findings from two studies reporting developmental trajectories, it appears that FA may be initially higher, but eventually lower, in children with ASD relative to those without the disorder (Solso et al., Reference Solso, Xu, Proudfoot, Hagler, Campbell, Venkatraman and Courchesne2016; Wolff et al., Reference Wolff, Gu, Gerig, Elison, Styner, Gouttard and Piven2012). If FA is elevated in children with ASD during the first years of life, but not thereafter, it implicates one or more of the rapid and dynamic neurodevelopmental events unique to this period.
It may seem initially counterintuitive that FA would be increased in children with ASD during early childhood. One might interpret this as evidence of precocious neural development or hyperconnectivity. For example, higher FA resulting from precocious development could plausibly result from atypical timing of sensitive or critical period plasticity (LeBlanc & Fagiolini, Reference LeBlanc and Fagiolini2011). However, a more parsimonious explanation is that FA does not reflect a unitary and invariant aspect of white matter structure. Based on the relative magnitude of diffusion along the major eigenvector, FA is generally regarded to index the strength and coherence of a given fiber pathway (i.e., “more is better”). This interpretation may be relatively accurate in describing structural connectivity in the mature brain. However, it may be less so among young children for whom the nature and pace of neurodevelopment differs. For example, white matter pathways undergo dramatic change during early childhood, a process that includes significant pruning in addition to axon growth and myelination (Deoni et al., Reference Deoni, Mercure, Blasi, Gasston, Thomson, Johnson and Murphy2011; Gao et al., Reference Gao, Lin, Chen, Gerig, Smith, Jewells and Gilmore2009; LaMantia & Rakic, Reference LaMantia and Rakic1990). While reports of relatively lower FA in older individuals with ASD may be driven by factors such as myelin content and axon caliber, higher FA in infants and toddlers may reflect altered axon growth, retraction, and experience-dependent refinement (McFadden & Minshew, Reference McFadden and Minshew2013). There is evidence of dampened axonal pruning and growth in ASD (Zikopoulos & Barbas, Reference Zikopoulos and Barbas2010) that may also explain volumetric increases reported in white matter during infancy and toddlerhood (Wolff et al., Reference Wolff, Gerig, Lewis, Soda, Styner, Vachet and Piven2015). Resolution as to the meaning of higher FA in ASD may ultimately demand further study using higher resolution imaging as well as histological studies of nonhuman animal models.
Auditory-Evoked and Resting-State fMRI
FMRI is a relatively common method for deriving estimates of brain activation based on task-evoked or spontaneous fluctuations in blood oxygen across space and time. Task-evoked brain activation is implemented to localize a given psychological/motor phenomenon, whereas the study of spontaneous fluctuations of blood oxygenation provides insight into the intrinsic architecture of neural functional connectivity. Both of these methods are highly susceptible to motion artifacts, thereby precluding implementation in very young children while awake. However, auditory-evoked fMRI and resting-state fMRI can be acquired during natural sleep, and there are now six published studies reporting results from auditory-evoked fMRI or resting-state fMRI in infants and toddlers with ASD.
In the first study to use auditory-evoked fMRI during natural sleep with young children with autism, Redcay and Courchesne (Reference Redcay and Courchesne2008) identified significant differences in regional activation patterns in response to forward and backward speech sounds in a small sample of toddlers with autism (n = 12) relative to two control groups. They also reported data suggesting lateralization favoring the right hemisphere in the ASD group. Using the same paradigm, Eyler, Pierce, and Courchesne (Reference Eyler, Pierce and Courchesne2012) examined neural responses to speech sounds in 40 children ages 12–48 months with ASD relative to typically developing controls. They reported significantly less activation in the left superior temporal gyrus in children with ASD, as well as evidence for right lateralization in response to speech sounds (in contrast to left lateralization observed among the control group). Building on this work (Eyler et al., Reference Eyler, Pierce and Courchesne2012; Redcay & Courchesne, Reference Redcay and Courchesne2008), Lombardo et al. (Reference Lombardo, Pierce, Eyler, Carter Barnes, Ahrens-Barbeau, Solso and Courchesne2015) examined activation patterns in regions associated with language function in 60 toddlers with ASD relative to two control groups. The ASD group was subdivided on the basis of expressive and receptive communication scores. The authors reported that toddlers with ASD who had poorer language a year later showed less activation in bilateral temporal and frontal regions of interest relative to comparison groups, including children with ASD who had relatively age-typical language scores. The authors also reported an inverse pattern of brain–behavior correlations between the ASD groups and typically developing controls. While language function was positively correlated with activation strength in putative language regions of interest for the TD group, there were significant negative correlations for those with ASD. Conversely, those with ASD showed positive correlations between language measures and nonlanguage regions, such as the cerebellum, thalamus, and motor cortex.
A study from the same research group regressed auditory-evoked activations out of the model to examine correlations between spontaneous fluctuations in blood flow between anatomically defined regions of interest (Dinstein et al., Reference Dinstein, Pierce, Eyler, Solso, Malach, Behrmann and Courchesne2011). Decreased coactivation of bilateral superior temporal and inferior frontal gyri (IFG) was observed in children with ASD relative to children with language delay and TD controls. The authors noted a significant positive association between expressive language and an index of interhemispheric synchrony in the IFG among the group of children with ASD. They also reported that social and communication scores derived from the Autism Diagnostic Observation Schedule were negatively associated with IFG synchrony. While compelling, these results should be interpreted with caution as methods for identifying and controlling for motion artifacts in resting-state connectivity data emerged subsequent to this publication (see Power, Barnes, Snyder, Schlaggar, & Petersen, Reference Power, Barnes, Snyder, Schlaggar and Petersen2012; Satterthwaite et al., Reference Satterthwaite, Wolf, Loughead, Ruparel, Elliott, Hakonarson and Gur2012; Van Dijk, Sabuncu, & Buckner, Reference Van Dijk, Sabuncu and Buckner2012). A recent study of preschool-aged children (mean age 3.5) that adopted rigorous motion-rejection/correction strategies showed weaker functional connectivity between the amygdala and bilateral medial prefrontal cortex, the striatum, and various regions within the temporal lobe (Shen et al., Reference Shen, Li, Keown, Lee, Johnson, Angkustsiri and Nordahl2016). The authors also reported weaker functional connectivity between V1 and somatosensory regions in children with ASD as compared to age-matched controls.
Patterns of whole-brain functional connectivity may represent a potential biomarker of ASD during infancy. In a prospective study of infants at high familial risk for ASD, Emerson et al. (Reference Emerson, Adams, Nishino, Hazlett, Wolff, Zwaigenbaum and Pivenin press) utilized resting-state fMRI collected at age 6 months to predict diagnostic outcomes at age 2 years. The authors reported that a cross-validated machine-learning algorithm applied to the imaging data yielded a positive predictive value of 100% and a negative predictive value of 96%. Functional connections contributing to the predictive algorithm were derived on the basis of their association with cognitive and behavioral features, such as social communication and repetitive behavior, at age 2 years. Although based on a relatively modest sample (n = 59 high-risk infants, 11 of whom were later diagnosed with ASD), the results suggest the possibility of a presymptomatic test for ASD that could be used to make clinical decisions for infants who are already at elevated risk due to family history.
Discussion of auditory-evoked and resting state fMRI
Functional neuroimaging during natural sleep offers a potentially powerful approach for characterizing emerging specialization (via auditory-evoked paradigms) as well as the architecture of functional connectivity during the first years of life. To date, however, there have been relatively few published studies applying these methods to infants and toddlers who have or later develop ASD. In our survey of the literature, we identified only six such studies. The majority of this work has been produced by one research group and has largely focused on brain regions previously associated with language processing. These four studies by Courchesne et al. have identified atypical response patterns to speech sounds, evidence of right lateralization, as well as diminished synchronous activation of putative language regions in children with ASD (Dinstein et al., Reference Dinstein, Pierce, Eyler, Solso, Malach, Behrmann and Courchesne2011; Eyler et al., Reference Eyler, Pierce and Courchesne2012; Lombardo et al., Reference Lombardo, Pierce, Eyler, Carter Barnes, Ahrens-Barbeau, Solso and Courchesne2015; Redcay & Courchesne, Reference Redcay and Courchesne2008).
Findings from Shen et al. (Reference Shen, Li, Keown, Lee, Johnson, Angkustsiri and Nordahl2016) concerning diminished cortical and subcortical amygdala connectivity compliment neuroimaging reports of atypical amygdala structure among young preschool-aged children (Mosconi et al., Reference Mosconi, Cody-Hazlett, Poe, Gerig, Gimpel-Smith and Piven2009; Munson et al., Reference Munson, Dawson, Abbott, Faja, Webb, Friedman and Dager2006; Schumann et al., Reference Schumann, Barnes, Lord and Courchesne2009). Evidence that whole-brain functional connectivity patterns could serve as a sensitive and specific presymptomatic biomarker of autism in high-risk sibs will very likely catalyze future work. Based on recent advances in this imaging modality, it is our view that there is considerable potential for functional connectivity MRI to inform (a) the underlying neurobiological substrates of autism and (b) clinical prediction. Nevertheless, a word of caution is warranted as this field is continually refining analytic methods (e.g., Power, Schlaggar, & Petersen, Reference Power, Schlaggar and Petersen2015). Nonetheless, work on functional connectivity collected during the infant/toddler period is steadily accelerating (Gao, Lin, Grewen, & Gilmore, Reference Gao, Lin, Grewen and Gilmore2016), and it is our expectation that the number of such studies focused on ASD will significantly increase in the coming decade.
General Discussion
Based on the clustering of clinically significant behavioral features, a diagnosis of ASD is the result of a cascade of effects likely beginning in utero. While this cascade of effects may occur over a broader temporal period (Brian et al., Reference Brian, Bryson, Smith, Roberts, Roncadin, Szatmari and Zwaigenbaum2016; Davidovitch, Levit-Binnun, Golan, & Manning-Courtney, Reference Davidovitch, Levit-Binnun, Golan and Manning-Courtney2015), ASD emerges during a unique window of brain development characterized by changes that are extraordinary in both rate and scope. It is during this short but crucial period of development wherein a child transitions from appearing typical across multiple cognitive and behavioral domains to manifesting impairment and developmental delays. Again drawing on the analogy of the journey to autism, it is not the diagnostic outcome, perhaps viewed as the “destination,” that is of foremost interest. Instead, the primary source of intrigue and the largely untapped opportunity are the events of the 1,000 or so days from birth to the eventual consolidation of autistic symptoms. Along with prospective behavioral studies of infants and toddlers, brain-imaging research has significantly advanced our knowledge of the early development of autism and potential sources of vulnerability for the disorder.
Several consistent themes have emerged from neuroimaging studies of infants and toddlers who have or later develop ASD. First and foremost among these is that of increased cerebral volume involving both gray and white matter (Courchesne et al., Reference Courchesne, Karns, Davis, Ziccardi, Carper, Tigue and Courchesne2001; Hazlett et al., Reference Hazlett, Poe, Gerig, Smith, Provenzale, Ross and Piven2005, Reference Hazlett, Poe, Gerig, Styner, Chappell, Smith and Piven2011, Reference Hazlett, Gu, Munsell, Kim, Styner, Wolff and Piven2017; Nordahl et al., Reference Nordahl, Scholz, Yang, Buonocore, Simon, Rogers and Amaral2012; Ohta et al., Reference Ohta, Nordahl, Iosif, Lee, Rogers and Amaral2016; Schumann et al., Reference Schumann, Bloss, Barnes, Wideman, Carper, Akshoomoff and Courchesne2010; Shen et al. Reference Shen, Nordahl, Young, Wootton-Gorges, Lee, Liston and Amaral2013; Sparks et al., Reference Sparks, Friedman, Shaw, Aylward, Echelard, Artru and Dager2002; Xiao et al., Reference Xiao, Qiu, Ke, Xiao, Xiao, Liang and Liu2014). The process of tissue overgrowth appears to begin in middle infancy, is established by toddlerhood, and remains stable through early school age. By adulthood, overgrowth may no longer be apparent, and the effects may even be reversed (Courchesne et al., Reference Courchesne, Karns, Davis, Ziccardi, Carper, Tigue and Courchesne2001; Zielinski et al., Reference Zielinski, Prigge, Nielsen, Froehlich, Abildskov, Anderson and Lainhart2014). Increased brain tissue volume in toddlers with ASD may be explained, and temporally preceded, by hyperexpansion of cortical surface area during the first year of life (Hazlett et al., Reference Hazlett, Gu, Munsell, Kim, Styner, Wolff and Piven2017). There is also initial evidence that the rate of surface area expansion during infancy may accurately predict a later diagnosis of ASD in children at familial risk. As with the cerebrum, overgrowth of subcortical structures, such as the amygdala and caudate nucleus, have also been reported (Hazlett et al., Reference Hazlett, Poe, Lightbody, Gerig, Macfall, Ross and Piven2009; Hoeft et al., Reference Hoeft, Walter, Lightbody, Hazlett, Chang, Piven and Reiss2011; Mosconi et al., Reference Mosconi, Cody-Hazlett, Poe, Gerig, Gimpel-Smith and Piven2009; Nordahl et al., Reference Nordahl, Scholz, Yang, Buonocore, Simon, Rogers and Amaral2012; Schumann et al., Reference Schumann, Barnes, Lord and Courchesne2009; Sparks et al., Reference Sparks, Friedman, Shaw, Aylward, Echelard, Artru and Dager2002), and in some cases linked to specific behavioral features of ASD (Estes et al., Reference Estes, Shaw, Sparks, Friedman, Giedd, Dawson and Dager2011; Mosconi et al., Reference Mosconi, Cody-Hazlett, Poe, Gerig, Gimpel-Smith and Piven2009; Munson et al., Reference Munson, Dawson, Abbott, Faja, Webb, Friedman and Dager2006; Schumann et al., Reference Schumann, Barnes, Lord and Courchesne2009; Wolff et al., Reference Wolff, Hazlett, Lightbody, Reiss and Piven2013).
There is also converging evidence of atypical structural and functional connectivity in the development of ASD. Seed-based, resting-state functional connectivity between the amygdala and canonical targets is reduced in preschool-aged children with ASD (Shen et al., Reference Shen, Li, Keown, Lee, Johnson, Angkustsiri and Nordahl2016), consistent with what might be expected given increased amygdala volumes and atypical FA in limbic regions such as the uncinate and the inferior longitudinal fasciculi (Conti et al., Reference Conti, Mitra, Calderoni, Pannek, Shen, Pagnozzi and Guzzetta2017; Solso et al., Reference Solso, Xu, Proudfoot, Hagler, Campbell, Venkatraman and Courchesne2016; Wolff et al., Reference Wolff, Gu, Gerig, Elison, Styner, Gouttard and Piven2012; Xiao et al., Reference Xiao, Qiu, Ke, Xiao, Xiao, Liang and Liu2014). A series of studies from Courchesne et al. also indicates atypical connectivity in language areas, patterns of which have been associated with measures of receptive and expressive language function (Dinstein et al., Reference Dinstein, Pierce, Eyler, Solso, Malach, Behrmann and Courchesne2011; Eyler et al., Reference Eyler, Pierce and Courchesne2012; Lombardo et al., Reference Lombardo, Pierce, Eyler, Carter Barnes, Ahrens-Barbeau, Solso and Courchesne2015; Redcay & Courchesne, Reference Redcay and Courchesne2008). A proof-of-principle study from the IBIS group demonstrated that 6-month-old functional networks revealed associations with later behavioral outcomes and could be exploited to accurately classify whether or not a high-risk child would be diagnosed with ASD at 24 months (Emerson et al., in press). The prognostic value of information gleaned from functional connectivity data was likewise demonstrated recently by Lombardo et al. (Reference Lombardo, Pierce, Eyler, Carter Barnes, Ahrens-Barbeau, Solso and Courchesne2015). To date, there are no longitudinal descriptions of whole-brain functional connectivity patterns across the first years of life, but longitudinal studies of structural connectivity suggest atypical growth patterns in infants/toddlers with ASD (Solso et al., Reference Solso, Xu, Proudfoot, Hagler, Campbell, Venkatraman and Courchesne2016; Wolff et al., Reference Wolff, Gu, Gerig, Elison, Styner, Gouttard and Piven2012, 2015). There is further evidence that the structural properties of white matter circuits may be fundamentally altered in young children who develop ASD in a manner unique to the first years of life (Ben Bashat et al., Reference Ben Bashat, Kronfeld-Duenias, Zachor, Ekstein, Hendler, Tarrasch and Ben Sira2007; Conti et al., Reference Conti, Mitra, Calderoni, Pannek, Shen, Pagnozzi and Guzzetta2017; Solso et al., Reference Solso, Xu, Proudfoot, Hagler, Campbell, Venkatraman and Courchesne2016; Weinstein et al., Reference Weinstein, Ben-Sira, Levy, Zachor, Ben Itzhak, Artzi and Ben Bashat2011; Wolff et al., Reference Wolff, Gu, Gerig, Elison, Styner, Gouttard and Piven2012; Xiao et al., Reference Xiao, Qiu, Ke, Xiao, Xiao, Liang and Liu2014). While associations between aspects of structural and functional connectivity to the emergence of ASD warrant further study, especially during early childhood, leveraging both modalities to inform models of pathogenesis and clinical prediction may prove especially fruitful.
Infant and toddler imaging studies have implicated, with some consistency, the structure and circuitry of multiple cortical and subcortical regions and tissue types in the development of ASD. Considered together, these observations suggest that autism may arise from a broad disturbance in central nervous system development that begins very early and has downstream effects on neural plasticity and specialization (Johnson, Jones, & Gliga, Reference Johnson, Jones and Gliga2015). Generalized brain overgrowth along with altered structural and functional connectivity evident by infancy or toddlerhood may be by-products of a common pathogenic process that begins in utero. Cortical neurons are almost exclusively generated prenatally, with peak proliferation occurring between 10 and 20 weeks of gestation in typical development (Samuelsen et al., Reference Samuelsen, Larsen, Bogdanovic, Laursen, Graem, Larsen and Pakkenberg2003). Following this period of exuberant overproliferation, the third trimester is marked by the elimination of approximately 50% of neurons through apoptosis (Rabinowicz, de Courten-Myers, Petetot, Xi, & de los Reyes, Reference Rabinowicz, de Courten-Myers, Petetot, Xi and de los Reyes1996). This regressive process is selective, and likely favors less established and weakly connected neurons for elimination (Buss, Sun, & Oppenheim, Reference Buss, Sun and Oppenheim2006). There is recent evidence that alterations in prenatal neuronal development involving progenitor cell division and differentiation, along with subsequent growth and refinement of neurites, may lay the foundation for ASD (Marchetto et al., Reference Marchetto, Belinson, Tian, Freitas, Fu, Vadodaria and Muotri2016). Abnormal processes related to neuronal proliferation in the second trimester or subsequent developmental elimination in the third could account for a pathological excess of neurons and give rise to the autistic phenotype during early childhood (Casanova et al., Reference Casanova, van Kooten, Switala, van Engeland, Heinsen, Steinbusch and Schmitz2006; Courchesne et al., Reference Courchesne, Mouton, Calhoun, Semendeferi, Ahrens-Barbeau, Hallet and Pierce2011; Fang et al., Reference Fang, Chen, Jiang, Liu, Yung, Fu and Ip2014). Even a relatively minor irregularity in neuronal development could have far-reaching cumulative effects on postnatal cortical plasticity (Kanold, Reference Kanold2009), connectivity (Simon et al., Reference Simon, Pitts, Hertz, Yang, Yamagishi, Olsen and Lu2016), and synaptic function (Fang et al., Reference Fang, Chen, Jiang, Liu, Yung, Fu and Ip2014; Johnson et al., Reference Johnson, Jones and Gliga2015) that impede a child's ability to acquire sophisticated social communication and produce an unusual excess of restricted and repetitive behaviors. Moreover, an altered process of neuronal development, which includes cell proliferation and apoptosis, could plausibly explain brain growth differences observed during the first years of life in children who develop autism (Hazlett et al., Reference Hazlett, Gu, Munsell, Kim, Styner, Wolff and Piven2017).
Inextricably linked to neurodevelopment occurring prenatally, postnatal developmental processes are likewise integral to the emergence of ASD. Infancy and toddlerhood are characterized by unique and rapid changes in brain structure and function, which at the cellular level includes peak synaptic growth and arborization (Huttenlocher, Reference Huttenlocher1979) as well as axon growth, pruning, and myelination (Cowan, Fawcett, O'Leary, & Stanfield, Reference Cowan, Fawcett, O'Leary and Stanfield1984; LaMantia & Rakic, Reference LaMantia and Rakic1990). Considering the neuroimaging studies reviewed herein, it appears highly likely that ASD arises from a confluence of multiple developmental events not limited to any single point of vulnerability. There is evidence implicating both synaptic and axonal plasticity in the development of the disorder. Postmortem studies of individuals with ASD indicate increased dendritic spine density (Hutsler & Zhang, Reference Hutsler and Zhang2010; Tang et al., Reference Tang, Gudsnuk, Kuo, Cotrina, Rosoklija, Sosunov and Sulzer2014) possibly resulting from deficient or less responsive pruning during early sensitive periods (Tang et al., Reference Tang, Gudsnuk, Kuo, Cotrina, Rosoklija, Sosunov and Sulzer2014). Postmortem studies and studies of nonhuman animal models of ASD have likewise identified evidence of atypical axon growth and myelination (Huang et al., Reference Huang, Chuang, Chou, Chen, Wang, Chou and Hsueh2014; Pacey et al., Reference Pacey, Xuan, Guan, Sussman, Henkelman, Chen and Hampson2013; Zikopoulos & Barbas, Reference Zikopoulos and Barbas2010) suggesting that axonal plasticity during early development may be altered in its timing or responsiveness. These and other histological findings complement findings from neuroimaging studies on the emergence of ASD, pointing to potentially multiple pre- and postnatal pathogenic processes involving neurogenesis, migration, regionalization, synaptogenesis, pruning, and development of short- and long-range connectivity. It is worth noting that the neurodevelopmental processes underlying ASD are not discrete, and each plays a critical yet mutually dependent role in early development. How these processes interact over time in determination of risk or protection, as well as to what extent they arise from a common mechanism or set of mechanisms, remain important targets for further study.
The Road Ahead
A necessary next step for studies into the development of ASD will be to account for individual variability. While there may be common features shared by many children diagnosed with ASD, it has been well established that no single neurobiological or behavioral feature is uniformly associated with the disorder. On the one hand, this reflects the etiologic and phenotypic heterogeneity of ASD and presents the opportunity to refine conceptualization of the disorder beyond a unitary construct. On the other hand, it presents a meaningful challenge to translating research into clinical practice, particularly for very young children for whom the disorder may not be clearly manifest. Although certain aspects of infant brain development, such as rate of surface area expansion or functional connectivity signatures, have shown promise in accurately predicting a diagnosis (Emerson et al., in press; Hazlett et al., Reference Hazlett, Gu, Munsell, Kim, Styner, Wolff and Piven2017) and informing models of pathogenesis (Piven et al., in press), extrapolating these findings beyond the ages at which these measurements were acquired or to other subgroups is rife with difficulty. One recent compelling hypothesis, anchored in long-term longitudinal data, suggests that trajectories of change/stability in a given domain (e.g., patterns of network connectivity, language functioning) may be particularly useful for parsing heterogeneity in a manner that could bolster clinical efforts (Lord, Bishop, & Anderson, Reference Lord, Bishop and Anderson2015). Autism is ultimately not immune to the developmental psychopathology principles of equifinality and multifinality (Cicchetti & Rogosch, Reference Cicchetti and Rogosch1996). Unfortunately, analytic approaches that embrace the developmental complexities inherent to ASD are the exception rather than the rule, and attention to such issues is in many cases limited to perfunctory discussions of heterogeneity. More long-term longitudinal studies incorporating multimodal neuroimaging data coupled with data from multiple levels of analysis are needed to fully characterize the heterogeneity of ASD and better capture how points of vulnerability vary across individuals and time. That said, the emerging body of results on brain development from studies of infant siblings with autism, which by design reduce etiologic heterogeneity by examining multiplex families, suggests some common starting points.
One important motivation for better characterizing the early neurodevelopment of ASD is to propel efforts to deliver targeted intervention or prevention. Early intervention can be highly effective in ameliorating the impact of ASD by improving long-term cognitive and behavioral outcomes (Dawson et al., Reference Dawson, Rogers, Munson, Smith, Winter, Greenson and Varley2009; Estes et al., Reference Estes, Munson, Rogers, Greenson, Winter and Dawson2015; MacDonald, Parry-Cruwys, Dupere, & Ahearn, Reference MacDonald, Parry-Cruwys, Dupere and Ahearn2014) and significantly decreasing lifetime costs associated with care (Chasson, Harris, & Neely, Reference Chasson, Harris and Neely2007). However, only a minority of young children with ASD achieve significant gains through such intervention, and many show little or no improvement in core deficit areas (Howlin, Magiati, & Charman, Reference Howlin, Magiati and Charman2009; Magiati, Wei Tay, & Howlin, Reference Magiati, Wei Tay and Howlin2012). This is in part due to a lack of precision in how interventions are tailored to individual needs. Because early childhood represents a finite window of significant brain and behavioral plasticity, the conventional trial-and-error approach to identifying an effective intervention risks the expenditure of valuable time and resources. The solution may lay in intervention strategies that take heterogeneity into account through individualization (Sherer & Schreibman, Reference Sherer and Schreibman2005). Cognitive and behavioral factors such as pretreatment cognitive function or adaptive communication ability can provide some guidance as to type, duration, and intensity of intervention, but the prescriptive power of these factors alone is limited (Kovshoff, Hastings, & Remington, Reference Kovshoff, Hastings and Remington2011; Magiati, Charman, & Howlin, Reference Magiati, Charman and Howlin2007). Accounting for variability in the neural phenotype of ASD has the potential to further improve links between the specific needs of an individual child and the content, intensity, and timing of intervention (Dawson, Reference Dawson2008; Ecker, Reference Ecker2017; Wolff, Reference Wolff, Reichow, Boyd, Barton and Odom2016). Accomplishing this will require novel and developmentally informed strategies that leverage behavioral and neurobiological variability to inform determination of risk and individualization of treatment.
The past two decades have been marked by a dramatic increase in knowledge pertaining to the neurodevelopment of ASD. This has included new insights into changes in brain structure and connectivity that take place prior to consolidation of clinical symptoms. Taken together, this work highlights an important and relatively narrow window of vulnerability for the development of autism. This work also provides opportunities to better detect risk for the disorder and intervene prior to an age when a diagnosis of autism is conventionally made. We would be remiss in failing to acknowledge, however, that there is no single, well-worn path to autism. Instead, there are multiple developmental pathways culminating in a common diagnosis, and clarifying these trajectories will likely increase the precision with which children are identified and provided treatment.