Introduction
Translating a concept in both languages is fundamental to the bilingual experience. When a bilingual looks at an image, two names of that object in the bilingual's two different languages are said to be automatically activated. Much psycholinguistic research has focused on the magnitude, and the directionality of such translation activation (Hoshino & Kroll, Reference Hoshino and Kroll2008; Kroll & Ma, Reference Kroll, Ma, Fernández and Cairns2018; Kroll & Stewart, Reference Kroll and Stewart1994; Sunderman & Kroll, Reference Sunderman and Kroll2006; Van Hell & Tanner, Reference Van Hell and Tanner2012). More recent studies have shown that translation is spontaneous and unconscious even in bilinguals who have good proficiency in both their languages (Thierry & Wu, Reference Thierry and Wu2007). Studies using the visual world paradigm have revealed the spontaneous nature of both within- and cross-language activation at phonological and semantic level (Canseco-Gonzalez, Brehm, Brick, Brown-Schmidt, Fischer & Wagner, Reference Canseco-Gonzalez, Brehm, Brick, Brown-Schmidt, Fischer and Wagner2010; Ju & Luce, Reference Ju and Luce2004; Lagrou, Hartsuiker & Duyck, Reference Lagrou, Hartsuiker and Duyck2011; Marian, Spivey & Hirsch, Reference Marian, Spivey and Hirsch2003; Mercier, Pivneva & Titone, Reference Mercier, Pivneva and Titone2014; Mishra & Singh, Reference Mishra and Singh2014; Spivey & Marian, Reference Spivey and Marian1999; Weber & Cutler, Reference Weber and Cutler2004). Models such as BLINCS (Shook & Marian, Reference Shook and Marian2013) predict the nature of eye movements during bilingual audio-visual language processing and how these dependent variables reflect subtle aspects of phonological and semantic activation in bilinguals. Working memory as a critical resource plays a key role in many aspects of bilingual language processing (Hadar, Skrzypek, Wingfield & Ben-David, Reference Hadar, Skrzypek, Wingfield and Ben-David2016; Ito, Corley & Pickering, Reference Ito, Corley and Pickering2018). On several occasions, we encounter the need to perform cross-modal information processing under a concurrent working memory load. For instance, when we are trying to integrate some spoken and visual information while simultaneously rehearsing a telephone number for later retrieval. In this study, we ask if working memory load influences this so-called “automatic” or spontaneous nature of translation activation in bilinguals. The issue is whether, and if so, which cognitive systems constrain language non-selective activation as seen in multiple domains and modalities of bilingual language processing. Here, we do not explore if working memory capacity affects an individual's language non-selective activation but use working memory as a cognitive load to track its influence on audio-visual processing.
In the visual world paradigm, eye movements are tracked to visual objects as a function of simultaneous spoken language input (Huettig & Altmann, Reference Huettig and Altmann2005; Mishra, Reference Mishra2009; Tanenhaus, Spivey-Knowlton, Eberhard & Sedivy, Reference Tanenhaus, Spivey-Knowlton, Eberhard and Sedivy1995). As participants listen to fragments of spoken language, their eye movements are driven towards visual objects that are related to the spoken language in some manner. We chose this paradigm for two reasons: 1) It has been extensively used to examine bilingual parallel language activation during processing of spoken information in the co-presence of visual information and robust evidence for cross-linguistic activation in bilinguals has been observed; 2) the online nature of the eye movement data obtained with this paradigm allows tracking the time-course of cross-linguistic activation. For example, Mishra and Singh (Reference Mishra and Singh2014), in a visual world eye-tracking study found that Hindi–English bilinguals looked immediately at the Hindi word bandar/monkey among distractors as they listened to the English word gun/bandook. That is because bandar is phonologically related to the translation of gun (bandook). This activation was seen in both L1-L2 (i.e., L1 spoken word) and L2-L1 (i.e., L2 spoken word) directions suggesting that bilinguals activate cross-language orthographic information in either language direction. In a later study, the authors demonstrated such cross-linguistic translation activation using pictures as well (Mishra & Singh, Reference Mishra and Singh2016). Importantly, these studies show that unbalanced bilinguals who have acquired their second language much later in life but have reasonable proficiency in it activate cross-linguistic information with input in either of their languages.
Can such cross-linguistic activation as seen through eye movements be constrained by an external factor? Few recent studies have provided evidence in favour of this (Blumenfeld & Marian, Reference Blumenfeld and Marian2013; Mercier et al., Reference Mercier, Pivneva and Titone2014). For instance, Blumenfeld and Marian (Reference Blumenfeld and Marian2013) observed that Spanish–English bilinguals with better inhibitory control on a non-linguistic Stroop task showed lesser parallel activation in the later period (approximately 600–800 ms after word onset). Similarly, Merceir et al. (2014) found that bilinguals with better scores on oculomotor inhibitory control tasks showed smaller within-language and cross-language activation during the visual world task. These studies suggest that general-purpose executive control processes can help in the conflict resolution between two languages during spoken language processing and constrain the degree of parallel language activation.
Several researchers have advanced theories on the mechanism of language-mediated eye movements assigning a critical role to working memory (Huettig, Olivers & Hartsuiker, Reference Huettig, Olivers and Hartsuiker2011a). Huettig et al. (Reference Huettig, Olivers and Hartsuiker2011a) have suggested that working memory acts as the buffer which integrates the incoming visual information from the display and the linguistic information evoked due to the processing of the spoken words and directs eye movements to appropriate locations. Working memory has also been suggested to play a role in the retrieval of visual information during the integration of linguistic and visual information (Knoeferle & Crocker, Reference Knoeferle and Crocker2006).
In the only other study to examine the role of working memory load on cross-linguistic activation, Prasad, Viswambharan and Mishra (Reference Prasad, Viswambharan and Mishra2019) administered the visual world paradigm under a visual working memory load. Participants heard a spoken word and viewed a display containing the spoken word referent, the cohort of the translation equivalent and two unrelated distractors. Before the visual world display, these were asked to remember an array of five colours and were presented a test array after the visual world display. The task was to judge if the test array was the same or different compared to the earlier array. The study showed reduced cross-linguistic activation but only when the participants were asked to click on the spoken-word referent (Experiment 1). No such effects were observed when there was no task-demand (Experiment 2) suggesting that a visual working memory load can constrain irrelevant cross-linguistic activations under certain conditions. In another study, Huettig and Janse (Reference Huettig and Janse2016) examined anticipatory spoken language processing using the visual world paradigm where participants listened to spoken sentences and looked at four line drawings. Their interest was to see if participants looked at the context-appropriate objects anticipatorily and whether their working memory capacity modulated the looks to the objects. It was found that working memory capacity positively correlated with the extent of language-mediated eye movements during prediction. These findings suggest that cognitive resources, specifically working memory, are required for language-mediated eye movements during spoken word comprehension.
Considering the paucity of research on the role of working memory on language-mediated eye movements in bilinguals, we examined it using the visual world paradigm on Hindi–English bilinguals. Participants were asked to maintain a set of digits in memory which was followed by a visual world display of written word along with spoken word input. One of the written words in the display was a phonological competitor of the translation of the spoken word (TE cohort), and others were unrelated distractors. We adapted the design and the stimuli of Mishra and Singh (Reference Mishra and Singh2014) who had found activation of TE cohorts when the spoken words were presented in both L1 or L2 using similar bilinguals. To make the participants attentive to the visual world segment of the study, they were asked to report the identity of the words presented on the display on some trials. Previous visual world studies have used written words in place of pictures and have found similar effects for language-mediated eye movements to targets (Huettig & McQueen, Reference Huettig and McQueen2007; Salverda & Tananhaus, Reference Salverda and Tanenhaus2010) as well as competitors (Veivo, Järvikivi, Porretta & Hyönä, Reference Veivo, Järvikivi, Porretta and Hyönä2016; Veivo, Porretta, Hyönä & Järvikivi, Reference Veivo, Porretta, Hyönä and Järvikivi2018).
An important variable in our study was the direction of language input. Since we were expecting listeners to spontaneously translate the spoken word into the other language which was then expected to activate the phonological cohort, it is important to know how such a mechanism is affected by input in L1 and L2. The revised hierarchical model (RHM, Kroll & Stewart, Reference Kroll and Stewart1994) is a developmental model for bilingual language processing which assumes that early learners of L2 have weaker conceptual links between L2 words and their meaning. So, when the input is in L2, they access the meaning via the L1 translation of the word. Thus, backward translation (L2 to L1) is mediated through lexical links between L2 and L1 whereas forward translation (L1 to L2) can proceed through the strong conceptual links of L1 words. The model, thus, predicts faster backward translation through the direct lexical route which has been backed up by several studies using translation recognition/production tasks (Kroll, Michael, Tokowicz & Dufour, Reference Kroll, Michael, Tokowicz and Dufour2002; Poarch, Van Hell & Kroll, Reference Poarch, Van Hell and Kroll2015; Talamas, Kroll & Dufour, Reference Talamas, Kroll and Dufour1999). However, the opposite pattern of results has also been found with faster translation in the forward direction (Christoffels, De Groot & Kroll, Reference Christoffels, De Groot and Kroll2006; Sunderman & Priya, Reference Sunderman and Priya2012). For instance, Sunderman and Priya (Reference Sunderman and Priya2012) found that Hindi–English bilinguals were faster in translating from L1 to L2 in a translation recognition task. The authors suggested that this reversed pattern of results could be attributed to the higher L2 proficiency of their participants. This suggests that as the proficiency in L2 increases, the reliance on L1 translation to access the meaning of L2 words decreases which weakens the direct lexical activation of L1 upon hearing/reading L2. Thus, the pattern of faster translation from L2 to L1 observed in L2 learners becomes reversed with increasing L2 proficiency.
Although it is to be noted that several researchers have criticised the basic tenets of RHM on the grounds of lack of evidence for some of its key predictions (Brysbaert & Duyck, Reference Brysbaert and Duyck2010). One of the fundamental assumptions of RHM is the existence of separate lexicons for the two languages and as a consequence selective access to either of the languages. But, cross-linguistic activation seen in the visual world paradigm indicates non-selective access (Spivey & Marian, Reference Spivey and Marian1999). Studies using word recognition tasks have shown that the other language is active even when the entire task is run in one language (Thierry & Wu, Reference Thierry and Wu2007). Also, the translation asymmetry (faster translation in L2 to L1 direction) predicted by RHM in early L2 learners is not always observed. As described above, this asymmetry is expected to even out with increasing L2 proficiency, but equivalent translation effects are observed even for L2 learners (e.g., Duyck & Brysbaert, Reference Duyck and Brysbaert2004). This suggests that the assumption of conceptual mediation only through L1 in L2 learners, as assumed by RHM, is not necessarily true. L2 words also seem to have conceptual links. In contrast, the BIA+ model (Dijkstra & van Heuven, Reference Dijkstra and Van Heuven2002) assumes an integrated lexicon and predicts cross-linguistic activation given any language input. Importantly, BIA+ architecture proposes that non-linguistic factors such as WM and executive control cannot directly influence lexical activations and are slower in exerting their influence. Thus, according to BIA+ cross-linguistic activations are more bottom-up and automatic in the early stages of word recognition, whereas later activations are more susceptible to control (Blumenfeld & Marian, Reference Blumenfeld and Marian2013). Thus, we would expect the WM load to influence cross-linguistic activations only in the later stages of comprehension.
Our participants in this study were Hindi (L1) – English (L2) bilingual university students who predominantly used L2 in their daily communication and were expected to be highly proficient in L2. In previous studies on the same population, similar sample of Hindi–English bilinguals have been found to be more proficient in their L2 than L1 (Bhatia, Prasad, Sake & Mishra, Reference Bhatia, Prasad, Sake and Mishra2017; Prasad, Viswambharan & Mishra, Reference Prasad, Viswambharan and Mishra2019). Thus, we expected the translation from L2 to L1 to not be mediated by the lexical route and thus be more effortful and slow. If this is indeed the case, any additional working memory load should affect language-mediated search in the L2-L1 direction more so than the L1-L2 direction. Since eye movements are an outcome of the translation process itself, the load should affect this very process.
We chose the proportion of fixations as our main dependent measure as eye movements are considered to be a direct correlate of linguistic processing. Researchers have shown that a greater number of saccades are made to an object which has an enhanced representation in the mind (Altmann & Kamide, Reference Altmann and Kamide2007). This occurs because the spoken language input increases the activation of the referred objects. As discussed in the previous section, bilinguals also routinely activate the translations of the words they encounter and their related words. Thus, listening to a word triggers activations of neighbouring words in that language, the translation word and the words related to the translation. Objects in the display whose representations match the spoken-word triggered activations then attract eye movements towards them. Different aspects of eye movements are used to indicate language-mediated behaviour. Typically, the proportion of fixations – the likelihood of fixating an object in a given time window relative to overall looks in that time window – is said to index the level of attention deployed at the location of the object. Some researchers have also measured the proportion of time participants look at an object (dwell time) or the latency of the first saccade to an object, although the proportion of fixations remains the most commonly used measure (Huettig, Rommers & Meyer, Reference Huettig, Rommers and Meyer2011b).
It is to be noted that in spite of many visual word eye-tracking studies on such issues with bilinguals, many things related to the core mechanisms remain unclear. It is very difficult to say with confidence what exactly these looks towards visual objects mean – linguistic activation or ocular responses related to search? Nevertheless, we assume here that eye movements towards various objects indicate the actual psycholinguistic process of translation triggered by the spoken word input. Therefore, we expect the working memory load to reduce the competitor activations to a greater extent in the direction in which translation activation is more effortful, which in this case is the L2 – L1 direction.
The other very important point is the effect of load on the time course of activation. Since eye movements measured with this paradigm provide online data, we can map the degree of activation or deactivation over a period and compare them across objects. Based on the predicted translation asymmetry in this set of bilinguals, it is likely that the translation will be fast in the L1 – L2 direction and, therefore, corresponding eye movements towards the competitors should show a peak in the early time windows (200–600 ms). Further, the effect of load might be seen more in the later period since previous studies have shown that only later activation is under cognitive control whereas early parallel activation is more automatic (Blumenfeld & Marian, Reference Blumenfeld and Marian2013, although there is no definite consensus regarding what exactly constitutes “late” and “early”). This is also in line with the precautions of BIA+ model. Therefore, we will explore if working memory load affects activation of TE cohorts on different time scales depending on the language of the input spoken word. In the absence of previous background research, we do not have exact predictions regarding the specific time course effects and its interaction with language direction.
The first experiment was administered without any load to replicate the basic effect of cross-linguistic activation observed in Mishra and Singh (Reference Mishra and Singh2014). The effect of the load was examined in Experiment 2 (load: 2 and 4 letters) and Experiment 3 (load: 2, 6 and 8 letters). Thus, we aimed to examine the effect of increasing load on cross-linguistic activation. If working memory load constrains the processing of spoken words, we predicted that the load should lead to either delayed or reduced looks towards the TE cohorts. However, if language-mediated eye movements are automatic and independent of working memory, we predicted that there would be no difference in the time course and magnitude of cross-linguistic activation as a function of load.
Experiment 1
Methods
Participants
Nineteen Hindi–English bilinguals (7 females, mean age = 23.4 years, SD = 0.6) participated in the main experiment (Table 1). All participants belonged to the students’ community of the University of Hyderabad. Hindi (L1) was their native language and they had learnt English (L2) early in life (at 4.8 ± 1.9 years) through formal schooling. Participants completed a series of control tasks which included tasks measuring their proficiency in L1 and L2. A language questionnaire (Bhatia et al., Reference Bhatia, Prasad, Sake and Mishra2017; Kapiley & Mishra, Reference Kapiley and Mishra2018, Reference Kapiley and Mishra2019; Roychoudhuri, Prasad & Mishra, Reference Roychoudhuri, Prasad and Mishra2016) was also administered to each participant which contained questions that required self-rating (on a scale of 1–10, 10 being the highest) on their level of proficiency in reading, speaking and auditory comprehension. It is apparent from the data that participants had acquired English at an early age and used English throughout their education in college and university. Therefore, these were early bilinguals. Informed written consent was taken from all participants for their participation in the study. The study was approved by the institutional ethics committee of University of Hyderabad.
Table 1. Language proficiency and Demographic data

10 point scale. 1 = poor, 10 = excellent.
Note: t-tests were performed between L1 and L2 self-rating scores. The significance values are mentioned in the table.** p < 0.01, * p < 0.05
Vocabulary test
All the participants completed an online vocabulary test (WordORnot, Center for Reading research, Ghent University) which was administered to test their proficiency in L2. The test consisted of categorising the displayed string of letters as a “word” or a “non word”. The total score was the difference in the percentage of trials in which a word was categorised correctly and the percentage of trials a non-word was judged as a word (Table 1). Based on their research so far, the researchers who developed this test estimate that a second language speaker of English with high proficiency would judge approximately 33% of the words correctly (Brysbaert, Stevens, Mandera & Keuleers, Reference Brysbaert, Stevens, Mandera and Keuleers2016).
Verbal fluency tests
Verbal fluency tests were administered in both L1 and L2. The participants were given three letters (F, A, S in L2 and क (Ka), प(Pa), म(Ma) in L1) and asked to produce as many words as possible for each letter in one minute. FAS was chosen for English as it is one of the commonly used tasks, especially in clinical settings, to examine language impairment (Spreen & Strauss, Reference Spreen, Strauss, Spreen and Strauss1998). We similarly chose the Hindi letters based on a battery of neuropsychological tests adapted for the Indian context (Hariprasad, Koparde, Sivakumar, Varambally, Thirthalli, Varghese, Basavaraddi, & Gangadhar, Reference Hariprasad, Koparde, Sivakumar, Varambally, Thirthalli, Varghese, Basavaraddi and Gangadhar2013; Rao, Subbakrishna & Gopukumar, Reference Rao, Subbakrishna and Gopukumar2004). The number of unique words spoken were recorded and noted down for each letter. A cumulative score for each language was calculated by averaging the scores of the three letters. There was no difference in verbal fluency scores(Table 1) between L1 (M = 11.65) and L2 (M = 11.25), p = .72.
Material & stimuli
Eighty common L1 nouns with their L2 translations and eighty L2 nouns with their L1 translations were selected. Out of these, forty L1 nouns and forty L2 nouns were taken from a previous study of one of the authors (Mishra & Singh, Reference Mishra and Singh2014). A total of 160 sets of word stimuli along with 160 spoken words were created (Appendix 1). Each set of word stimuli consisted of four written words where one of the words was a phonological competitor of the translation equivalent of the spoken word (TE cohort) and others were unrelated distractors. For example, if the spoken word was सड़क/SaDak (Road), the display contained the word Rope which is a phonological cohort of the translation word Road and 3 unrelated ditsractors. In the “L1-L2” language direction, the spoken words were in L1 and the display consisted of L2 written words. Similarly, for the “L2 – L1” direction, L2 spoken words were coupled with the display containing L1 written words. All the words were displayed at the center of equal sized quadrants of size 256 * 192 pixels. The L1 words were written in Krutidev font and the L2 words in Times New Roman, font size 26.
Stimuli rating
Ten Hindi–English bilinguals who did not take part in the eye tracking study performed the translation agreement task on the 160 set of stimuli. Participants were asked to rate the translation pairs for their accuracy in both the language directions. Agreement on the translation of the spoken word was rated on a 5-point scale (5 indicating highest agreement). The ratings showed that the agreement was high, both for L2 spoken words (M = 4.99, SD = 0.03) and L1 spoken words (M = 4.95, SD = 0.06). The participants also rated the extent of phonological and semantic similarity between the translation word and the four written words on the display. For the L1 – L2 direction, the phonological similarity of translation words to the TE cohorts (M = 4.71, SD = 0.20) was significantly higher (p < .001) compared to the distractors (M = 1.00, SD = 0.002). We also made sure that the TE cohort and the distractors were semantically different from the translation word, (M = 1.05, SD = 0.11 and M = 1.02, SD = .05 respectively). Similarly, for the L2 – L1 direction, the TE cohort was significantly more similar sounding (M = 4.44, SD = 0.62) to the translation word compared to the distractors (M = 1.15, SD = 0.31), p < .001. The TE cohort and the distractors were also compared semantically with the translation word (M = 1.22, SD = .34 and M = 1.18, SD = .28) which showed that they belonged to different semantic categories. We also calculated the Levenshtein distance for an objective measure of the similarity between the words. Levenshtein distance is the number of insertions, deletions, substitutions required to transform one string to the other. We found that the levenshtein distance between the translation of a spoken word and it is cohort (M = 2.75, SD = 1.18) was significantly lower (p < .001) than the distance between the spoken word and unrelated distracters (M = 5.14, SD = 1.09).
The spoken words were recorded using the software “Audacity” by a native speaker of L1 and saved as .wav files. The mean duration of the spoken words in L1 and L2 was 413.13 ms (SD = 86.3 ms) and 495.33 (SD = 103.8) ms, respectively.
Procedure
Participants sat at a distance of 60 cm from a 19 inch LCD monitor with a screen resolution of 1024 × 768 pixels and a refresh rate of 60 Hz. Stimuli were prepared using SR Research Experiment Builder software (SR Research Ltd, Ontario, Canada) and eye movement data was controlled by a computer running Eyelink 1000 eye tracking system. The experiment began after a successful 13 point calibration (see Figure 1 for sample trials). Every trial started with a black fixation cross (1° by 1°) presented for 1000 ms against a white background at the center. Then, a display containing four written words and a spoken word were presented simultaneously for 3000 ms through Creative Labs SBS A300 speakers. In order to make sure that participants paid attention during the visual world task, they were asked a question regarding one of the words on 10% of the trials (total 16 trials). On these 10% of the trials, the question was “did you see the word X?”, where X referred to one of the distractor words present in the display on half of the trials. On the other half, X referred to a word which was not present in the display. Participants were expected to press Yes (left shift key) or No (right shift key) on the keyboard in response to this question. The key-to-response mapping was counterbalanced across participants. Participants were given 20 practice trials before the start of the main experiment. A self-paced break was given halfway through the experiment after which drift correction was performed to ensure proper calibration.

Fig. 1. Sequence of events in Experiment 1 and 2. In Experiment 1, visual world display was presented for 3000 sm along with a spoken word. In this example, spoken word is in L1: Mor (Peacock). The display consists of a phonological cohort of Peacock: Peanut and three unrelated distractors. In Experiment 2, the visual world display was preceded by the presentation of letters to be remembered (2 or 4 letters). The memory test question was presented just after the visual world display on every trial. Participants were asked to recognise if the set of letters (“HF”) was the correct backward sequence of the letters presented before. In this example, the correct answer is Yes. On 10% of the trials, a question was asked on the visual world display. Participants had to judge if the given word (Tree) was present in the display.
There were a total of 160 trials divided into two blocks. Trials were divided equally and blocked between language-directions (L1 – L2, L2 – L1). The order of presentation of the blocks as well as the trials within the blocks were randomised.
Data analysis
Eye movements
Fixations to the TE cohort and distractor words were extracted using the Data viewer software (SR research, Ontario). Each quadrant containing the written word was considered as an area of interest (AOI). The fixations falling in these AOIs from the onset of the spoken word till 1000 ms were extracted and considered for analysis. This time range was divided into 20 ms bins and fixations to each AOI for each bin was calculated (Figure 2). The proportion of fixations to an object was calculated as the number of fixations to that object in the given time bin divided by the total number of fixations in that time bin. The proportion of fixations to the three distractors were averaged (see Table 2 for proportion of fixation values for all conditions).

Fig. 2. Comparing TE cohort activation observed in Mishra and Singh (Reference Mishra and Singh2014) with the current study results (Experiment 1). Parallel activation is indicated by the significantly higher proportion of looks to TE cohorts compared to distractors. Significant parallel activation was observed in Experiment 1 (without load) for both language directions replicating findings from Mishra and Singh (Reference Mishra and Singh2014).
Note: “L2 – L1” – L2 spoken words. “L1 – L2” – L1 spoken words. Proportion of fixations, denoted by P(fix), were only used for plotting for ease of visualisation. Statistical analysis was performed on logit transformed data. Error bands denote ± 1 SE
Table 2. Proportion of fixations under no-load (Experiment 1)

Note: Bold values indicate significant differences between looks to TE cohorts (TEC) and distractors (D) for that condition.
For statistical analysis, the proportion data was logit transformed (Barr, Reference Barr2008) and mixed effects logistic regression analysis was performed with package “lme4” in R (Bates, Maechler, Bolker & Walker, Reference Bates, Maechler, Bolker and Walker2015, version 1.1–10). Glmer function was used with family specified as binomial and link logit. Language of the spoken word (L1, L2; henceforth referred to as “spoken word language”) and object type (TE cohort, distractor) were considered as fixed effects. L2 spoken word and TE cohorts were considered as baseline. To examine how cross-linguistic activation unfolds over time, the time period was divided into 200 ms time windows consistent with previous visual world studies (Huettig et al., Reference Huettig, Rommers and Meyer2011b, Mishra & Singh, Reference Mishra and Singh2014; Prasad et al., Reference Prasad, Viswambharan and Mishra2019). It is to be noted that although the trial period was divided into 20 ms bins for the sake of visualisation, statistical analysis was done on 200 ms time periods which we will henceforth refer to as “time-windows”. Separate models were then created for each time-window (TW1: 0–200 ms, TW2: 200–400 ms, TW3: 400–600 ms, TW4: 600–800 ms, TW5: 800–1000 ms) with language direction and object type as fixed effect. In the eye movements analysis, “cross-linguistic activation” is used to denote a significant difference between proportion of looks/fixation duration for TE cohorts compared to distractors.
Manual responses
Accuracy analysis was performed on the responses in the visual world task. Trials were dummy coded as 1 (“Correct trials”) and 0 (“Incorrect trials”). Mixed effects regression models with family specified as binomial and link logit were fitted for Accuracy data. Spoken word language (L1, L2) was considered as the fixed effect. Response time data was not analysed as the visual world task was not a speeded task.
In all the models, two-way and 3-way interactions were included in the mixed effects models only when they significantly improved the model fit as determined by forward model comparison. For all the models, Participants and Items were considered as random effects. Random slopes were not included in the lmer model on overall fixations with Time as a factor as the model failed to converge. Subject-wise random slopes were included for each of the fixed effects. A random slope was kept in the random effect structure if including it improved the model fit. We did not include item-wise random slopes as the model often failed to converge and also because item-wise variation is usually lesser than subject-wise variation. Statistical significance was determined using the p values obtained through the default output of the glmer function in R based on asymptotic Wald tests (Luke, Reference Luke2017).“multcomp” package in R was used to perform pairwise comparisons by constructing contrast matrices (Hothorn, Bretz & Westfall, Reference Hothorn, Bretz and Westfall2008).
Word frequency
Word frequency is an important predictor in psycholinguistic experiments. Since we did not collect frequency ratings from the participants, we used the Worldlex database (Gimenes & New, Reference Gimenes and New2016) to calculate the average frequency of the words presented on the visual world display. We extracted the frequency per million values based on blogs and newspapers for both L1 and L2 for TE cohorts and each of the distractors. The database also has data from twitter but it was not available for L1: thus, data from newspapers and blogs was used for both the languages for the sake of consistency. The log transform of frequency of TE cohorts (logTE) and the log transform of the average frequency of the three distractors (logD) was then entered as a fixed effect in all the analyses (eye movements and manual responses). They were retained in the model only when it improved the model fit as compared to a model without them. These model comparisons were made using the ANOVA function in R which yielded p values based on chi square tests.
Results
Fixations
logTE and logD were excluded as fixed effects as they did not significantly improve the model fit. The analysis of the logit transformed proportion of fixations between 0–1000 ms after word onset showed a main effect of time (β = 0.0001, t = −4.68, p < .001). All the two-way and three-way interactions involving time were also significant (|t| > 3) indicating that the participants' looks towards TE cohorts and distractors varied as a function of time. It is difficult to examine complex 3-way or 4-way interactions through pairwise comparisons. Thus, separate models were created for each 200 ms time window in line with previous studies (e.g., Prasad et al., Reference Prasad, Viswambharan and Mishra2019). Looks to TE cohorts were significantly greater than looks to distractors in the first three time windows (0 ms – 600 ms), as indicated by a main effect of object type (TW1: β = −0.1, t = −3.88, p < .001; TW2: β = −0.14, t = −3.74, p < .001; TW3: β = −0.16, t = −3.63, p < .001 respectively). The language of the spoken word influenced cross-linguistic activation from TW3 (400 ms) onwards as indicated by a significant interaction between spoken word and object type for TW 3, 4 and 5 (TW3: β = 0.18, t = 3.16, p = .001; TW4: β = -0.11, t = -2.97, p = .003; TW5: β = -0.16, t = −4.13, p < .001 respectively). Pairwise comparisons for TW3 showed higher cross-linguistic activation when spoken word was in L2 (β = 0.16, z = 3.63, p < .001) compared to when the spoken word was in L1 (β = 0.04, z = 0.99, p = .481). Pairwise comparisons for TW 4 revealed significant cross-linguistic activation only when the spoken word was in L1 (β = 0.12, z = 2.42, p = .027), but not when spoken word was in L2 (β = 0.01, z = 0.21, p = .9). A similar pattern was observed in TW5 with significant cross-linguistic activation observed for L1 spoken words (β = 0.15, z = 3.41, p = .001) but not for L2 (β = −0.004, z = −0.09, p = .99) spoken words.
Visual world task
Spoken word language did not modulate the accuracy during the visual world task (β = −0.35, z = −0.91, p = .362, L1: M = 81.58%, SD = 25.47; L2: M = 84.96%, SD = 23.56). There was a marginally significant effect of distractor frequency (β = 0.49, z = 1.76, p = .08). Accuracy increased with greater frequency of the distractor words.
Discussion
The objective of this experiment was first to establish cross-linguistic activation in this set of bilinguals using the design of Mishra and Singh (Reference Mishra and Singh2014). Participants looked at the TE cohort of the spoken word more compared to the distractors indicating activation of the non-target language. Looks towards TE cohorts were significantly fewer and diminished faster when participants heard spoken words in L2. These results mostly replicate the findings of Mishra and Singh (Reference Mishra and Singh2014) and other similar studies in this set of Hindi–English bilinguals. Most of the visual world studies on bilinguals conducted to examine translation activation have used pictures in the display. While some of the interactive models of bilingual language processing (such as BIA) predict both orthographic and phonological activation of the competitor language while processing words in one language, experimental evidence for such activation during cross-modal processing is sparse (but see, Mishra & Singh, Reference Mishra and Singh2014; Veivo et al., Reference Veivo, Järvikivi, Porretta and Hyönä2016, Reference Veivo, Porretta, Hyönä and Järvikivi2018). Thus, the results of Experiment 1 once again show that bilinguals activate orthographic forms of the translations and their competitors while listening to a spoken word.
Experiment 2
Methods
Participants
Twenty-two Hindi–English bilinguals (four females, mean age = 22.5 years, SD = 0.87) participated in the experiment. None of the them had taken part in Experiment 1.
Material and stimuli
Stimuli used in the visual world component were the same as Experiment 1.
Procedure
In addition to the visual world component, each trial had a working memory component. A string of 2 or 4 English alphabet letters (Times New Roman, Bold, 26 pt size) were displayed one after the other at the center of the screen before the onset of the visual world display. These letters were randomly selected (without replacement) by the software on each trial from a set of nine English alphabet letters: B, F, H, J, L, M, Q, R and X (Marty, Chemla & Spector, Reference Marty, Chemla and Spector2013). Each letter stayed for 1000 ms with an interval of 500 ms in between during which a blank screen was displayed.
The visual world display was then presented followed by the working memory task (test phase) in which the same set of letters were shown together. On 50% of the trials, the exact backward sequence of the letters was shown. On the other half of the trials, some other combination of letters randomly chosen by the software was shown. For example, if the letters B, H, M, L were initially shown sequentially in that order, the test phase display could be LMHB (correct backward sequence) or some other combination of the letters. The participants had to judge if the order of letters in the test phase was the correct backward sequence of the previously shown letters. Participants indicated their response by pressing Yes (left shift key) or No (right shift key) on the keyboard. The key-to-response mapping was counterbalanced across participants. Our working memory task involved recognition and not recall (e.g., asking participants to recall the letters shown earlier and enter them through keyboard). Recall tasks are generally assumed to be more effortful, utilise greater working memory resources and require self-initiated processes than recognition tasks (Arenberg, Reference Arenberg, Eisdorfer and Lawton1973; Burke & Light, Reference Burke and Light1981; Schonfield & Robertson, Reference Schonfield and Robertson1966). It is possible that a recall task would have led to ceiling effects such that no effect would be seen on the visual world component of the task. As the participants were asked to pay attention to both the working memory task (presented on every trial) and the visual world task (presented on 10% of the trials), it was important not to make the working memory task too difficult. Many such dual-task studies that have examined the role of working memory load – for example, on attentional selection – have similarly used recognition tasks (e.g., Soto, Heinke, Humphreys & Blanco, Reference Soto, Heinke, Humphreys and Blanco2005; Woodman & Luck, Reference Woodman and Luck2004).
A total of 160 trials were divided equally between the language directions and type of load into 4 blocks. Language direction was the first level of blocking and type of load was the second level. That is, all trials with L1 (or L2) spoken word were presented together. Within that, trials with the two types of load were presented in blocks (where either of them could appear first). The ordering of the blocks was randomised at each blocking level. The trial order within each block was also randomised.
Data analysis
Eye movements
The analysis procedure was the same as that of Experiment 1. Load (4 letters, 2 letters) was additionally included as a fixed effect in the analysis of logit transformed fixations with 4-letter load as the reference
Manual responses
The accuracy data from the visual world task data was analysed using mixed effects (as described for Experiment 1) with load as an additional fixed effect. The accuracy on the working memory task was also analysed using the same procedure.
Results
Fixations
There was a main effect of Time (β = 0.0002, t = −4.84, p < .001). A significant interaction between object type, load, time and spoken word language was also observed (β = 0.0006, t = 6.03, p < .001 Table 3, Figure 3). Separate analysis was performed for each 200 ms time window. No significant cross-linguistic activation was observed in the first time window (β = −0.03, t = −0.55, p = .583). In the second time window (200 ms – 400 ms), a significant interaction between spoken word language, load and object type was observed (β = −0.17, t = −2.46, p = .014). Separate models were created for each language direction to probe this 3-way interaction. There was a significant interaction between load and object-type for L1 (β = −0.11, t = −2.31, p = .021) but not L2 spoken words (β = 0.05, t = 1.18, p = .238). Pairwise comparisons showed significant cross-linguistic activation (greater amount of looks to TE cohorts compared to distractors) when the spoken words were in L1 only for the 2-letter load condition (β = 0.1, z = 3.01, p = .005) but not for the 4-letter load condition (β = −0.008, z = −0.25, p = .96). No difference in the looks was observed for English spoken words in both the load conditions (t < 1.2). Interestingly, looks to TE cohorts were significantly reduced (TW3: β = 0.09, t = 2.02, p = 0.048) or equivalent (TW4: β = 0.05, t = 0.88, p = .384; TW5: β = 0.1, t = 1.62, p = .113) compared to distractors in the subsequent time windows, indicating that the cross-linguistic activation was completely inhibited 400 ms onwards. There were marginally or fully significant interactions between language direction, object type and load in these time windows (TW3: β = 0.1, t = −1.66, p = .097; TW4: β = 0.12, t = 1.74, p = .081; TW4: β = 0.44, t = 6.35, p < .001) but they were driven by greater looks to distractors compared to TE cohorts (see Appendix for full model outputs).
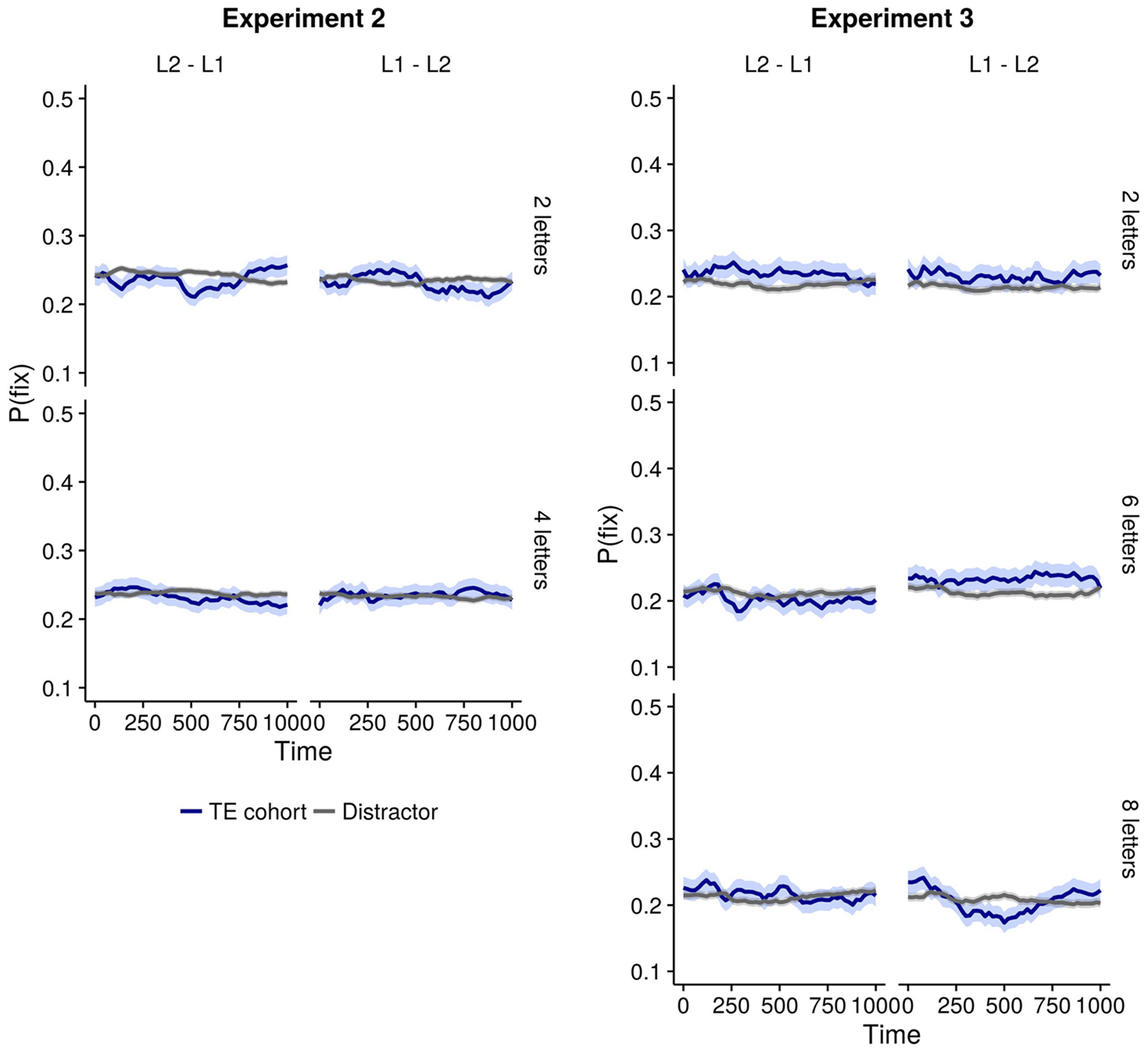
Fig. 3. Plots showing parallel activation in Experiment 2 and 3. Early activation (200–400 ms) was observed only for L1 spoken words (“L1 – L2”) under 2-letter load (Experiment 2 and 3) and 6-letter load (Experiment 3) condition. In both Experiment 2 and 3, no activation was observed when spoken words were in L2 (“L2 – L1”).
Note: Proportion of fixations, denoted by P(fix), were only used for plotting for ease of visualisation. Statistical analysis was performed on logit transformed data. Error bands denote ± 1 SE
Table 3. Proportion of fixations under two and four -letter load conditions (Experiment 2)

Note: Bold values indicate significant differences between looks to TE cohorts and distractors for that condition.
Visual world task
The accuracy on the visual world task was higher in the 2-letter load condition compared to the 4-letter load condition (β = 1.19, z = 3.18, p = .001). There was also a significant interaction between load and spoken word language (β = −1.19, z = −2.459, p = .014). Pairwise comparisons showed that the difference in accuracy between 2-letter and 4-letter load conditions was significant only when spoken words were in L2 (β = −1.19, z = −3.18, p = .003, 2-letters: M = 71.59%, SD = 32.08; 4-letters, M = 50%, SD = 31.81) compared to L1 (β = 0.00006, z = 0, p = 1, 2-letters: M = 51.14%, SD = 23.75; 4-letters, M = 51.14%, SD = 24.97). Thus, the working memory load interfered with the visual world task more when the spoken words were in L2.
Working memory task
The accuracy was higher on trials with 2-letter load (M = 94.26%, SD = 3.6) compared to the 4-letter load condition (β = 0.53, z = 3.62, p < .001, M = 90.96%, SD = 13.41).
Discussion
In Experiment 2, the visual world display was presented concurrently with a verbal working memory load of 2 or 4 letters. Participants maintained the verbal load while attending to the visual world task. Two important results emerged from the analysis of the proportion data. First, the proportion of looks towards TE cohorts did not differ significantly from the looks towards distractors when the input was in L2 for both the load conditions. This means that the TE cohort activation that we observed in Experiment 1 disappeared when participants listened to spoken words in the second language. When the input was in L1, participants looked at the TE cohorts more than the distractors only in the initial period (200–400 ms) and only in the 2-letter load condition. Thus, the presence of the load completely inhibited L2 – L1 activation, whereas L1 – L2 activation was preserved under a low load (2 letters) in the initial period and completely inhibited under a high load (4 letters). This indicates that the effect of the load was greater when the spoken word was in L2. Second, accuracy was higher under the 2-letter load condition compared to the 4-letter load condition, but only when the spoken words were in L2. The load did not affect the accuracy of the responses when the spoken words were in L1. It additionally showed that the load manipulation worked and had differential effects on the processing involved during the presentation of the visual world display.
In summary, we observed that looks towards competitors were inhibited under a high working memory load (4 letters). Since this claim is essentially based on a null result (no differences in looks to TE cohorts and distractors), we aimed to replicate this effect in the next experiment using three different types of load: 2, 6 and 8 letters.
Experiment 3
Methods
Participants
Twenty-four Hindi English bilinguals (Mean age = 24.42 years, SD = 1.38, 18 female) participated in the main experiment. None of the participants had taken part in either Experiment 1 or 2.
Material and stimuli
Stimuli used in the visual world component and the working memory component were similar to that used in Experiment 1.
Procedure
A procedure similar to Experiment 2 was used here with a minor change in the load manipulation. Three types of loads were used: 2, 6 and 8 letters.
Data analysis & Results
The analyses procedure was exactly the same as that of Experiment 2.
Fixations
There was a significant effect of Time on the looks to the visual world display (β = 0.0001, t = −2.59, p = .009. Separate models for each time-window were created to examine the time-course of these effects (Table 4, Figure 3). No significant cross-linguistic activation was observed in the first time-window (β = −0.09, t = −1.57, p = .121). In the second time window, a significant three-way interaction between spoken word language, load and object type was observed (β = −0.33, t = −4.11, p < .001). Separate models were created for each language direction to examine this 3-way interaction which showed a 2-way significant interaction between load and object type (β = 0.2, t = 3.73, p < .001). Pairwise comparisons on this model showed that when spoken words were in L1, the looks to TE cohorts were greater compared to distractors under 2 and 6 letter load (β = 0.11, z = 2.12, p = .09 and β = 0.15, z = 2.78, p = .015 respectively). No such differences were observed for eight-letter load (β = −0.09, z = −1.73, p = .204). No cross-linguistic activation was observed for English spoken words under any of the load conditions (t < 1). In all the subsequent time windows (400 ms onwards), there was no significant cross-linguistic activation in any of the conditions (t < 1)
Table 4. Proportion of fixations as a function of load (Experiment 3)

Note: Bold values indicate significant differences between looks to TE cohorts (TEC) and distractors (D) for that condition.
Visual world task
The accuracy analysis showed a marginally significant interaction between spoken word language and load (β = −1.51, z = −1.65, p = .099). Pairwise analysis indicated that the accuracy was higher under 6-letter load when compared to 8-letter load (β = −1.86, z = −2.82, p = .026, 2-letters: M = 62%, SD = 43.97; 6-letters: M = 82.67%, SD = 21.77; 8-letters: M = 46%, SD = 37.97), only when the spoken word was in L2. Load did not modulate the level of accuracy for L1 spoken words (p > .3, 2-letters: M = 65.33%, SD = 22.52; 6-letters: M = 58%, SD = 23.62; 8-letters: M = 69.44%, SD = 27.66).
Working memory task
The accuracy on the working memory task did not differ between any of the three load conditions (t < 1, 2-letters: M = 86.32%, SD = 14.17; 6-letters: M = 88.85%, SD = 11.31; 8-letters: M = 89.44%, SD = 12.86)
Discussion
Once again, we found that the working memory load reduced the proportion of looks to competitors. Specifically, when spoken words were in L1, higher looks to TE cohorts were observed for 2 and 6 letter load conditions in 200–400 ms time window. Subsequently, there was no difference between looks to competitors and distractors. When spoken words were in L2, the looks to TE cohorts did not differ significantly from looks to distractors in any of the load conditions. Surprisingly, TE cohort activation was observed for 6-letter load condition (Experiment 3) but not for the 4-letter condition (Experiment 2) in the 200–400 ms time window. Based on the results of Experiment 2, we expected cross-linguistic activation to be completely inhibited for the 6-letter load condition in both language directions even in the early time-window. We currently do not have any explanation as to why significant early activation was observed with such a relatively high working memory load. It is necessary to do more experiments with different levels of load to establish if there's a gradient effect of the load on cross-linguistic activation or if there is a threshold point beyond which no activations are seen. We are only suggesting that cross-linguistic activations are indeed susceptible to external constraints such as working memory resources. Future research should focus on the boundary conditions of this mechanism in more detail.
We also observed that the working memory load modulated the accuracy on the visual world task, but only when spoken words were in L2. Participants were more accurate in the 6 letters condition than in the 8 letters condition. Accuracy was not modulated by load for L1 spoken words. To summarise, Experiment 3 replicated the findings of Experiment 2 and showed that maintaining a very high working memory load while viewing the visual world display constrains cross-linguistic activation as seen through language-mediated eye movements. Overall, the constraining effect of the load was higher in the L2 – L1 direction in both the experiments.
General discussion
In three experiments, we examined the effect of a verbal working memory load on cross-linguistic translation activation in Hindi–English bilinguals. Participants saw four written words on the screen along with the audio input of a spoken word while holding 2,4, 6 or 8 letters in memory (Experiment 2 and 3). The baseline experiment without any load (Experiment 1) established the robust activation of TE cohorts in both language directions thereby replicating previous findings (Mishra & Singh, Reference Mishra and Singh2014). With the load (2 and 6 letters), TE cohorts were activated only in the initial time period (200–400 ms) and only when the spoken word was in L1. There was no activation of the TE cohorts when spoken was in L2 under any of the load conditions. The accuracy on the visual world task was also modulated by the load, but only when the spoken words were in L2. Thus, concurrent working memory load (of 2,4 and 6 letters) suppressed later activation in the L1 – L2 direction but completely inhibited L2 – L1 activation. To our knowledge, this is the first study to show the constraining effect of a concurrent working memory load on language nonselective activation during audio-visual spoken language processing. Whether such activations are automatic or subject to any form of control has been a matter of debate (Mishra, Olivers & Huettig, Reference Mishra, Olivers and Huettig2013). Our results clearly show that working memory load can significantly affect such non-selective activations.
The critical question we wanted to answer is should a WM load influence the forward or the backward direction more? Given that our bilinguals were unbalanced with higher proficiency in L2, we expected them to translate words with less ease from L2 to L1 than in the reverse direction. Assuming that the WM load consumes cognitive resources, any process that requires such a resource more – such as activating the translations and related words – will also suffer more under the load. With concurrent load, we found almost no evidence of TE cohort activation when spoken words were in L2. This pattern of results can be understood if we assume that for such bilinguals who have acquired higher proficiency in the second language, translation of lexical items from L2 to L1 does not proceed via the direct lexical route (as it does in early L2 learners) which probably makes it more resource consuming than the activation in the opposite direction. Similar results were obtained on the same population by Prasad et al. (Reference Prasad, Viswambharan and Mishra2019) where the effect of a visual working memory load on cross-linguistic activation was greater when the spoken words were in L2. Although, Prasad et al. (Reference Prasad, Viswambharan and Mishra2019) used line drawings in their display which also consisted of the spoken-word referent (unlike the current study which only had TE cohort in the display). Nonetheless, both the studies lead us to tentatively conclude that L2 – L1 activation requires more verbal working memory resources than L1 – L2 activation in bilinguals with high L2 proficiency. More such studies directly comparing two groups of bilinguals differing in their L2 proficiency are required to firmly establish the role of L2 proficiency on the automatic nature (or the lack of it) of cross-linguistic activation.
Proportions of looks towards visual referents in a visual world design are time sensitive. Early or late activations indicate the nature of attentional demands. Early activations have been assumed to be more automatic and late activations to be more strategic (Blumenfeld & Marian, Reference Blumenfeld and Marian2013). We observed early (200–400 ms after word onset) L1 – L2 activation in the 2 and 6 letter load conditions (Experiment 2 and 3). This activation was suppressed in the later time windows. This pattern of data suggests that working memory load constrains the strategic and resource demanding (late) activations and not the more robust initial automatic activations. Blumenfeld and Marian (Reference Blumenfeld and Marian2013) observed that when English-Spanish bilinguals listened to spoken words in Spanish, cross-linguistic activation in the early time periods (300–500 ms after word onset) was not inhibited by executive control processes. Executive control measures correlated with cross-linguistic activations only for the later time windows (633–767 ms). Participants with better performance on the Stroop task also inhibited cross-linguistic activations more effectively. This led the authors to conclude that early parallel language activation is automatic while later activation is under strategic control. Our results are in line with these findings.
In Experiment 1, we mostly replicated the findings of Mishra and Singh (Reference Mishra and Singh2014). But, it is important to note a few key differences in the results of the two studies. No cross-linguistic activation was observed for L2 spoken words 600 ms onwards in the current study whereas Mishra and Singh (Reference Mishra and Singh2014) found robust activations in both the language directions till 1000 ms after word-onset. Interestingly, in the current study, TE cohort activation was also enhanced in the 400–600 ms time window for L2 spoken words compared to L1 spoken words. A possible reason for this discrepancy could be that the participants in this study were highly proficient in L2 compared to the Hindi–English bilinguals in Allahabad who participated in Mishra and Singh (Reference Mishra and Singh2014). University of Allahabad is a state university in the northern part of India where Hindi is the native language of most students, as a result of which most students dominantly use Hindi for their communication. In contrast, University of Hyderabad attracts students from all over the country and English is the predominant language of communication on campus. As discussed previously, high proficient bilinguals no longer have the need to translate L2 words into L1 which could have led to the reduced TE cohort activation in the current study for L2 spoken words at later stages. It is not possible to quantify these differences as neither we nor Mishra and Singh (Reference Mishra and Singh2014) collected any data on the percentage of use and exposure to L2. At the moment, we can only speculate that contextual differences between the two locations could have led to these observed differences in the results.
Our findings have consequences for various bilingual language processing models. As discussed in the introduction, RHM explains translation effects as a function of developing second language proficiency in unbalanced bilinguals. We cannot directly compare our results with the predictions of RHM because the model was proposed to account for language production data. However, our task essentially required people to translate and activate cohorts of translation simultaneously with audiovisual input. Even if not directly related, Pickering and Garrod (Reference Pickering and Garrod2013) have proposed an integrated model of language processing that links the mechanisms of production and comprehensions together. Therefore, RHM should predict active bidirectional translation effects in our bilinguals who were unbalanced; in our other studies we have shown translation effects in these types of bilinguals in the Indian context. However, it remains to be examined to what extent RHM predicts translation in bilinguals who use languages that have no cognates such as our bilinguals. It is to be noted that later criticisms of the model on the grounds that relate to not finding evidence of asymmetry in naming tasks in bilinguals may not be directly relevant to our conclusions drawn from a visual world experiment (Brysbaert & Duyck, Reference Brysbaert and Duyck2010). It has not always been straight forward to predict the directionality and magnitude of translation effects in balanced and unbalanced bilinguals who use different languages. Our data assumes a wide-ranging connectionist framework that has widespread activations and cross-linkages across phonological and orthographic levels. This is precisely the working assumption of BIA+ which predicts co-activations in multiple modalities across languages. Although BIA+ does not include any cognitive constraints such as WM and attention, it certainly helps us in theorising about the nature of bottom-up co-activations both within and between languages. BIA + predicts unconstrained language non-selective activation. However, it is not always clear if BIA + would predict activation of translations of words across languages that involve semantics. It certainly predicts activation of cohorts both within and across languages.
More recently Dijkstra, Wahl, Buytenhuijs, Van Halem, Al-Jibouri, De Korte and Rekké (Reference Dijkstra, Wahl, Buytenhuijs, Van Halem, Al-Jibouri, De Korte and Rekké2019) proposed the Multilink model, which integrates some of the assumptions of both RHM and BIA + . The model is comprehensive and attempts to explain data from various language tasks, but as Mishra (Reference Mishra2019) pointed out, the model has little relevance for languages such as Hindi and English that do not share orthography or phonology. Further, the model also does not account for cross-modal activations derived from the visual world paradigm. However, the model does refer to the nonlinguistic control mechanisms that could constrain language activation in the bilingual mind. Our use of working memory load was cognitively constraining from a limited resource point of view. This kind of data should further help to modify the predictions of Multilink model which attempts to synthesise both linguistic and cognitive variables in bilingual language activation.
The Bilingual Language Interaction Network for Comprehension of speech (BLINCS) model was developed specifically to explain audio-visual integration and cross-linguistic activation as seen in visual world eye-tracking paradigm (Shook & Marian, Reference Shook and Marian2013). We have shown non-cognate cross-linguistic activation, which BLINCS explains at the level of semantics. In addition to supporting BLINCS’ core predictions regarding parallel activation in the audio-visual domain, we have further shown the dynamic influence of WM load on such activations. Currently, there are no other models that explain such multimodal interactions, and therefore, the BLINCS model can be further extended by considering the various other cognitive variables that influence parallel language activation. However, it is to be noted that all the above-mentioned languages have been built based on European languages. But there are major differences in reading, writing, speaking and listening practices between different languages making it difficult to directly borrow their predictions and apply it to the Indian context. Further, cognitive constraints on bilingual language processing depend on individual differences in executive control (Mercier et al., Reference Mercier, Pivneva and Titone2014), which in turn depend on broader social and cultural context. Thus, there is an urgent need to incorporate diverse languages, especially belonging to different language families, in the existing bilingual language processing models.
Surprisingly, there was no effect of frequency in all the experiments. This was unexpected considering robust frequency effects are often observed during word recognition (Brysbaert, Buchmeier, Conrad, Jacobs, Bölte & Böhl, Reference Brysbaert, Buchmeier, Conrad, Jacobs, Bölte and Böhl2011, Brysbaert et al., Reference Brysbaert, Stevens, Mandera and Keuleers2016; Monsell, Doyle & Haggard, Reference Monsell, Doyle and Haggard1989). Frequency effects are generally observed in lexical decisions tasks or word recognition tasks. The nature of frequency effects in cross-modal language processing as seen in the visual world paradigm has not been examined thoroughly. Although we expect that frequencies to play some role in the language mediated eye movements seen in this paradigm, we are not in a position to clearly explain the lack of frequency effects in our study. One possible reason could be that our participants were highly proficient users of both the languages. The Hindi–English bilinguals lived in a dynamic language context in the University where both L1 and L2 are used actively. It is known that high language exposure leads to reduced frequency effects (Monaghan, Chang, Welbourne & Brysbaert, Reference Monaghan, Chang, Welbourne and Brysbaert2017), presumably because such individuals also get exposed to low-frequency words more often. But this is just speculation at this point. More studies directed at examining the interaction between word frequency and spoken word recognition in visual world tasks are necessary to throw more light on this issue.
There are a few limitations in the current design that might have influenced our results and interpretations. Our sample size was low in all the experiments (< 25). Visual-world studies typically have more number of participants to lend more power to the findings. But it is to be noted that, we had a high number of experimental trials (80 for each language direction) which is double the number of trials used in Mishra and Singh (Reference Mishra and Singh2014). Further, we replicated the main pattern of results across two experiments with load making our findings moderately robust. However, we acknowledge that more experiments with large number of participants are required to develop a comprehensive model of cross-linguistic activation as seen through eye movements and the nature of their dependence on capacity-limited resources. Additionally, our design created a dual-task scenario on some trials. In such a context, participants have to strategise and prioritise their division of attention between the two tasks. Since the working memory task preceded the visual world task and was present on every trial, we assume that the participants considered this to be the primary task. Therefore, we cannot rule out the influence of such factors on the eye movements. We also acknowledge that it is still unclear what exactly such eye movements refer to. The linguistic activation of the TE cohort and programming an eye movement to a visual referent are different things and involve different mechanisms. While we measure the eye movements as a dependent measure, we take them to indicate linguistic activation itself. However, our results do not clarify if working memory load influenced the linguistic activation itself or the oculomotor aspects of the activation.
In sum, our study can contribute towards constraining the current models of cross-linguistic activation in bilinguals. In spite of the limitations, we have provided some initial results to show that cross-linguistic activation seen during bilingual language processing is not entirely spontaneous and uncontrolled when selective attention is channelised elsewhere.
Acknowledgements
We thank Divya Bhatia and Kesaban Roychoudhuri for help in data collection. This work was supported by a Department of Science and Technology grant sanctioned to RKM from the Indian Government.
Data availability statement
The data and materials for all experiments are available at https://osf.io/psy3x/?view_only=b876874da97a4587a94f56424dba4581
Appendix 1.

Appendix B
Full model output from linear mixed effects analysis exported through “stargazer” package in R (Hlavac, 2014). Treatment coding was used for the variables spoken-word language (Hindi, English) and object (“TE”, “D”). The models assumed TE as baseline. Time windows are denoted as “TW1”, “TW2”, “TW3”, “TW4”, “TW5”. “logD” and “logTE” refer to log transformed average frequencies for distractors and TE cohorts respectively. In Experiment 2, load had two levels (“high”, “low” with high as baseline). In Experiment 3, load had three levels: “load1”, “load2”, “load3” referring to 2-letter, 6-letter- and 8-letter condition respectively. “Load1” was the baseline. The full structure of the model is specified on top of the output table.
Experiment 1
1.1 Overall fixation
Looks ~ language * IA * Time + (1 | Subject) + (1 | Item)

1.2 Time-window analysis
TW1: Looks ~ language * IA + (1 + language | Subject) + (1 | Item)
TW2,3,4,5: Looks ~ language * IA + (1 + language + IA | Subject) + (1 | Item)

Pairwise (TW3)
Fit: lmer(formula = Looks ~ language * IA + (1 + language +
IA | Subject) + (1 | Item), data =
control_noload_logit_long_filtered_TW3)
Linear Hypotheses:
Estimate Std. Error z value Pr(>|z|)
Eng = = 0 0.16236 0.04472 3.630 0.000544 ***
Hin = = 0 0.04433 0.04472 0.991 0.481642
–––
Signif. codes: 0 ‘***’ 0.001 ‘**’ 0.01 ‘*’ 0.05 ‘.’ 0.1 ‘ ’ 1
(Adjusted p values reported -- single-step method)
Pairwise (TW4)
Fit: lmer(formula = Looks ~ language * IA + (1 + language +
IA | Subject) + (1 | Item), data =
control_noload_logit_long_filtered_TW4)
Linear Hypotheses:
Estimate Std. Error z value Pr(>|z|)
Eng = = 0 0.01098 0.05128 0.214 0.9590
Hin = = 0 0.12418 0.05128 2.422 0.0267 *
–––
Signif. codes: 0 ‘***’ 0.001 ‘**’ 0.01 ‘*’ 0.05 ‘.’ 0.1 ‘ ’ 1
(Adjusted p values reported -- single-step method)
Pairwise (TW5)
Fit: lmer(formula = Looks ~ language * IA + (1 + language +
IA | Subject) + (1 | Item), data =
control_noload_logit_long_filtered_TW5)
Linear Hypotheses:
Estimate Std. Error z value Pr(>|z|)
Eng = = 0 −0.004171 0.045108 −0.092 0.99294
Hin = = 0 0.153654 0.045108 3.406 0.00126 **
–––
Signif. codes: 0 ‘***’ 0.001 ‘**’ 0.01 ‘*’ 0.05 ‘.’ 0.1 ‘ ’ 1
(Adjusted p values reported -- single-step method)
1.3 Accuracy on visual world task
TASK_ACCURACY ~ language + logD + (1 + logD | RECORDING_SESSION_LABEL) + (1 | serial)

Experiment 2
2.1 Overall fixations
Looks ~ language * IA * load * Time + (1 | Subject) + (1 | Item)

2.2 Time-window analysis
TW1
Looks ~ language * IA * load + (1 + language + IA + load | Subject) + (1 | Item)

TW2
Looks ~ language * IA * load + (1 + language + IA | Subject) + (1 | Item)
L1: Looks ~ IA * load + (1 + load | Subject) + (1 | Item)
L2: Looks ~ IA * load + (1 + load + IA | Subject) + (1 | Item)

Pairwise (L1)
Fit: lmer(formula = Looks ~ IA * load + (1 + load | Subject) + (1 |
Item), data = expt2_logit_long_filtered_TW2_Hindi)
Linear Hypotheses:
Estimate Std. Error z value Pr(>|z|)
low(TE-D) = = 0 0.101869 0.033767 3.017 0.0051 **
high(TE – D) = = 0 −0.008433 0.033767 −0.250 0.9611
–––
Signif. codes: 0 ‘***’ 0.001 ‘**’ 0.01 ‘*’ 0.05 ‘.’ 0.1 ‘ ’ 1
(Adjusted p values reported -- single-step method)
TW3
Looks ~ language * IA * load + (1 + language + IA + load | Subject) + (1 | Item)
L1: Looks ~ IA * load + (1 + IA + load | Subject) + (1 | Item)
L2: Looks ~ IA * load + (1 + IA + load | Subject) + (1 | Item)

TW4
Looks ~ language * IA * load + (1 + language + IA + load | Subject) + (1 | Item)
L1: Looks ~ IA * load + (1 + IA + load | Subject) + (1 | Item)
L2: Looks ~ IA * load + (1 + IA + load | Subject) + (1 | Item)

TW5
Looks ~ language * IA * load + (1 + language + IA + load | Subject) + (1 | Item)
L1: Looks ~ IA * load + (1 + IA + load | Subject) + (1 | Item)
L2: Looks ~ IA * load + (1 + IA + load | Subject) + (1 | Item)

2.3 Accuracy on visual world task and memory task
TASK_ACCURACY ~ language * load + (1 + language |
RECORDING_SESSION_LABEL) + (1 | serial)
Memory_Accuracy ~ load + (1 | RECORDING_SESSION_LABEL) + (1 | serial)

Pairwise (visual world task)
Fit: glmer(formula = TASK_ACCURACY ~ language * load +
(1 + language | RECORDING_SESSION_LABEL) + (1 |
serial), data = expt2_vwRT, family = binomial(link = “logit”))
Linear Hypotheses:
Estimate Std. Error z value Pr(>|z|)
eng(high-low) = = 0 −1.194e+00 3.748e-01 −3.184 0.0029 **
hin(high-low) = = 0 1.751e-05 3.117e-01 0.000 1.0000
–––
Signif. codes: 0 ‘***’ 0.001 ‘**’ 0.01 ‘*’ 0.05 ‘.’ 0.1 ‘ ’ 1
(Adjusted p values reported -- single-step method)
Experiment 3
3.1 Overall fixations

3.2 Time-window analysis
TW1
Looks ~ language * IA * load_new + (1 + language +
load_new + IA | Subject) + (1 | Item)

TW2
Looks ~ language * IA * load_new + (1 + language +
load_new + IA | Subject) + (1 | Item)
L1: Looks ~ IA * load_new + (1 + IA + load_new | Subject) + (1 | Item)
L2: Looks ~ IA * load_new + (1 + IA + load_new | Subject) + (1 | Item)

Pairwise (L1)
Fit: lmer(formula = Looks ~ IA * load_new + (1 + IA + load_new | Subject) +
(1 | Item), data = expt3_logit_long_filtered_TW2_Hindi)
Linear Hypotheses:
Estimate Std. Error z value Pr(>|z|)
load1(TE-D = = 0 0.11557 0.05472 2.112 0.0903.
load2(TE-D) = = 0 0.15242 0.05475 2.784 0.0150 *
load3(TE-D) = = 0 −0.09609 0.05550 −1.731 0.2037
–––
Signif. codes: 0 ‘***’ 0.001 ‘**’ 0.01 ‘*’ 0.05 ‘.’ 0.1 ‘ ’ 1
(Adjusted p values reported -- single-step method)
TW3, TW4 and TW5
Looks ~ language * IA * load_new + (1 + language +
load_new + IA | Subject) + (1 | Item)

3.3 Accuracy on visual world and memory task
Visual world task: TASK_ACCURACY ~ condition_spoken_word * load_new + (1 | RECORDING_SESSION_LABEL) + (1 | serial)
WM task:

Pairwise (Visual world task)
Fit: glmer(formula = TASK_ACCURACY ~ language * load_new +
(1 | RECORDING_SESSION_LABEL) + (1 | serial), data = expt3_vwRT,
family = binomial(link = “logit”))
Linear Hypotheses:
Estimate Std. Error z value Pr(>|z|)
eng(6-2) = = 0 1.1716 0.6587 1.779 0.322
eng(8-2) = = 0 −0.6853 0.6825 −1.004 0.818
eng(8-6) = = 0 −1.8568 0.6572 −2.825 0.026 *
hin(6-2) = = 0 −0.3351 0.6341 −0.529 0.980
hin(8-2) = = 0 0.1937 0.5757 0.337 0.996
hin(8-6) = = 0 0.5289 0.6387 0.828 0.901
–––