Does featural description follow a universal or a language-specific pattern? This has been an overlooked question in the literature (Kremer & Baroni, Reference Kremer and Baroni2011). Comparative studies of English norms and other languages such as Italian and German (Kremer & Baroni, Reference Kremer and Baroni2011) seem to indicate no relationship between feature production and language-specific characteristics. However, given the recent publication of the Spanish norms (Vivas, Vivas, Comesaña, Garcia Coni, & Vorano, Reference Vivas, Vivas, Comesaña, García Coni and Vorano2017), there are no comparative studies of this language and English yet which could support the aforementioned findings that indicate no outstanding differences across languages in relation to the characterization of core features. The main purpose of the present article is to make a cross-linguistic comparison of the structural organization of semantic feature productions between Spanish and English so as to discuss empirical information about the relationship between semantic processing and different languages. In addition, focusing on bilingual semantic memory accounts that propose higher conceptual overlap for concrete and cognate words (de Groot, Reference de Groot1992; Van Hell & de Groot, Reference Van Hell and de Groot1998) compared to abstract and noncognate lexical items, the role of cognate status was assessed.
Recognizing which are the main features underlying the semantic representation of a concept is key to the understanding of models of semantic memory. Recent distributional and feature-based models of semantic memory have studied the properties and organization of the semantic features that are involved in the representation of concepts (Baroni, Murphy, Barbu, & Poesio, Reference Baroni, Murphy, Barbu and Poesio2010; Bolognesi, Reference Bolognesi2017; Cree, McNorgan, & McRae, Reference Cree, McNorgan and McRae2006; Sartori, Polezzi, Mameli, & Lombardi, Reference Sartori, Polezzi, Mameli and Lombardi2005; Taylor, Moss, & Tyler, Reference Taylor, Moss, Tyler, Hart and Kraut2007; Vigliocco, Meteyard, Andrews, & Kousta, Reference Vigliocco, Meteyard, Andrews and Kousta2009; Vigliocco, Vinson, Lewis, & Garrett, Reference Vigliocco, Vinson, Lewis and Garrett2004). The fact that semantic features are key to identify a given concept is widely accepted (Cree et al., Reference Cree, McNorgan and McRae2006; Taylor et al., Reference Taylor, Moss, Tyler, Hart and Kraut2007) and extensively acknowledged by different theoretical models (e.g., Cree & McRae, Reference Cree and McRae2003; Humphreys & Forde, Reference Humphreys and Forde2001; Mahon & Caramazza, Reference Mahon and Caramazza2009). Sartori et al. (Reference Sartori, Polezzi, Mameli and Lombardi2005) even assign an excluding role to this factor in the organization of conceptual knowledge.
Due to the growing interest in the study of the role of semantic features in the organization of concepts, numerous research groups of different countries and languages have analyzed and elaborated feature production norms (Buchanan, Holmes, Teasley, & Hutchinson, Reference Buchanan, Holmes, Teasley and Hutchison2012; Buchanan, Valentine, & Maxwell, Reference Buchanan, Valentine and Maxwell2019; De Deyne et al., Reference De Deyne, Verheyen, Ameel, Vanpaemel, Dry, Voorspoels and Storms2008; Devereux, Tyler, Geertzen, & Randall, Reference Devereux, Tyler, Geertzen and Randall2014; Lenci, Baroni, Cazzolli, & Marotta, Reference Lenci, Baroni, Cazzolli and Marotta2013; McRae, Cree, Seidenberg, & McNorgan, Reference McRae, Cree, Seidenberg and McNorgan2005; Moldovan, Ferré, Demestre, & Sánchez-Casas, Reference Moldovan, Ferré, Demestre and Sánchez-Casas2015; Montefinese, Ambrosini, Fairfield, & Mammarella, Reference Montefinese, Ambrosini, Fairfield and Mammarella2014; Ruts et al., Reference Ruts, De Deyne, Ameel, Vanpaemel, Verbeemen and Storms2004; Stein & de Azevedo Gomes, Reference Stein and de Azevedo Gomes2009; Vinson & Vigliocco, Reference Vinson and Vigliocco2008; Vivas et al., Reference Vivas, Vivas, Comesaña, García Coni and Vorano2017; Zannino et al., Reference Zannino, Perri, Pasqualetti, Di Paola, Caltagirone and Carlesimo2006). Typically, in the collection of semantic features, participants are presented with a set of concepts and are asked to produce the features that they believe best describe these concepts, a task known as a feature-listing task. This collection of features constitutes a semantic register of produced semantic features of a particular linguistic community. In addition, semantic feature production norms also provide several qualitative and quantitative measures (e.g., Wu & Barsalou, Reference Wu and Barsalou2009; taxonomy, imaginability, relevance, and significance) that are crucial for the design of controlled experimental stimuli. For example, in order to avoid cultural biases and construct stimuli lists matched in psycholinguistic variables, researchers may need normative values of all those variables regarding a particular linguistic community
In contrast, empirically derived semantic feature production norms play also a highly important role in the elaboration and testing of theories about semantic memory. Devereux et al. (Reference Devereux, Tyler, Geertzen and Randall2014) suggest that theories about the representation and processing of concepts have improved from models based on information derived from the semantic production norms. This information refers not only to the identity of the features produced in the norms but also to their statistical properties. In this framework, statistical data about the frequency of occurrence and the probability of feature co-occurrence are proposed as the fundamental organizational principles of cognitive models of semantic memory. This data allows for the development of precise quantitative theories about the organization of conceptual knowledge to be explored by means of computational models (Al Farsi, Reference Al Farsi2018; Baroni & Lenci, Reference Baroni and Lenci2010; Cree et al., Reference Cree, McNorgan and McRae2006; Lenci, Reference Lenci2018; Riordan & Jones, Reference Riordan and Jones2011). It is worth mentioning that we agree with Riordan and Jones (Reference Riordan and Jones2011) that featural and distributional models should not be conceptualized as competing theories; the focus should rather be on understanding the cognitive mechanisms human beings employ to integrate the two sources.
Gentner and Goldin-Meadow (Reference Gentner, Goldin-Meadow, Gentner and Goldin-Meadow2003) state that the dominant position within cognitive psychology in the last few decades has been that (a) conceptual structure is relatively constant in its core features across cultures, and (b) conceptual structure and semantic structure are closely coupled. Regarding the latter distinction, it is important to point out that “conceptual structure” refers to the mental representations of a given entity, while “semantic structure” is a wider term that encompasses the general organization of semantic memory, including various linguistic units and multimodal meanings assigned to words (Vigliocco & Filipovic, Reference Vigliocco and Filipovic2004). In this sense, the contribution of this study would be to provide a description of the different degrees of association between the paired productions of both languages in order to address the extensively discussed problem related to the effect of language on the structuring of concrete conceptual representations.
Against this background, we present a cross-linguistic comparison of Argentine Spanish and American English semantic norms for 219 concrete concepts (the intersection between both norms) in order to explore to what extent these norms reflect properties observed across languages (i.e., universal) and to what extent they are language specific. We expect concept descriptions to be generally stable across these language norms, providing some evidence that concrete words have conceptual cores.
Method
Stimuli
Stimuli were collected from the English (McRae et al., Reference McRae, Cree, Seidenberg and McNorgan2005) and Spanish (Vivas et al., Reference Vivas, Vivas, Comesaña, García Coni and Vorano2017) sets of semantic feature norms. The English norms comprised 540 concepts and the Spanish one 400. For this study we selected 219 concepts common to both languages from 19 different concept categories.
Procedure
The translation of Spanish concepts to English was key to allow the matching up of concepts between languages. The English translation was made by a native Spanish speaker who lived in the United States for 10 years and by a native American English speaker who specialized in literature.
A very important aspect to consider when creating a semantic feature norm is to guarantee that synonymous features are registered in the same way within and between concepts. Given that semantic feature production norms are generated from the contribution of a large number of participants, and that each of them uses different words to characterize the concepts included in the worksheets they have to complete, it is remarkable to notice the different words spontaneously produced by them in order to refer to the same feature. For example, in order to characterize the Spanish concept “sol” (sun), some participants wrote “amarillo” (yellow) while others “es amarillo” (it is yellow). Such cases, which are very frequent in the norms, are considered superficial variations of the same semantic content because there is no semantic difference among “amarillo” (yellow masculine gender), “amarillento” (yellowish), and “es de color amarillo” (it is yellow/it is yellow in color), but a difference in the way of referring to the same content. This variability should be reduced because of two fundamental reasons; first, the mass of data to process should become manageable, and second, the values of many of the variables that are crucial for semantic features (distinctiveness, relevance and production frequency, etc.) would be erroneously calculated if the spontaneous productions of semantic features remain unaltered. For example, let us consider how the variable frequency behaves for the Spanish concept “silla” (chair): 11 participants produced “tiene patas” (it has legs), 7 participants wrote “posee patas” (it possesses legs), and 6 simply responded “patas” (legs). If we consider these cases in isolation, these frequency values are not very high (taking into account that the highest value is 30, as each concept was characterized by 30 participants). However, if these responses are collapsed into a single feature (in this case, the resulting semantic feature is “tiene patas” [it has legs]), the frequency of production of the resulting semantic feature considerably increases, reaching a value of 24. In this study, we carried out a second interlinguistic coding (between the semantic features of both norms) for the same aforementioned reasons and under the same criteria. It is accepted a priori that the coding of responses may bring difficulties, as mentioned by Rogers et al. (Reference Rogers, Garrad, McClelland, Lambon Ralph, Bozeat, Hodges and Patterson2004), as researchers may vary in the way they code and analyze responses. However, as stated by these authors, this problem becomes less serious if it is assumed that verbally listed features do not constitute the conceptual representation per se. In this sense, the importance of the homogenization of data should not be underestimated because of the reasons already stated. What is very important then is to have a unified corpus with theoretically founded and empirically adequate coding criteria. These criteria have to be agreed on by the different groups of researchers devoted to the elaboration of semantic feature production norms. Once agreed upon, criteria should be rigorously applied to guarantee the replication of procedures.
Data analysis
The semantic production norms in English (McRae et al., Reference McRae, Cree, Seidenberg and McNorgan2005) and Spanish (Vivas et al., Reference Vivas, Vivas, Comesaña, García Coni and Vorano2017) were elaborated on a basis of 540 and 400 concrete concepts, respectively, using an intersection between both norms of 219 concepts. For the generation of verbal descriptors and the verbal semantic field for the 219 concepts, the production frequency of features for each concept was considered. Based on that, the 438.txt files generated contained the name of the concept alongside its most frequent featural descriptions. To calculate the semantic relationship between concepts of both languages, we used the cosine between vectors, which could be considered analogous to correlation coefficients (the more semantically related two words are, the higher is its cosine value). Specifically, we used the standard n-dimensional inner product for the Euclidean space to compare vectors using the angle they form with each other. Parallel vectors represent the greatest similarity (i.e., a cosine value of 1) while orthogonal vectors represent the greatest difference (i.e., a cosine value of 0; Kintsch, Reference Kintsch2001). This calculation was done with the software SynonymFinder (Beta version 1.1; http://iaai.fi.mdp.edu.ar:8080/sfweb). With the result of the calculation of the cosine between each concept pair, the software generates a square matrix mode1, which is the one in which columns and rows refer to the same set of entities (see Matrix 1, in the online-only supplementary material). Based on this matrix, we calculated how many concepts did or did not have their strongest link (in terms of cosine values) with their equivalent in the other language. Then, we calculated the mean of those values for both the concepts that did and did not have their strongest link with their equivalent in the other language, also looking for intralinguistic cases that showed similar values to those observed in interlinguistic examples. Specifically, for the concepts that did not coincide with their equivalent in the other language, we calculated the difference in terms of cosine values between each concept’s highest interlinguistic associate and its equivalent in the other language. Finally, Johnson’s method (Reference Johnson1967) was applied to do the hierarchical clustering by using UCINET software (Borgatti, Everett, & Freeman, Reference Borgatti, Everett and Freeman2002), taking as a starting point this matrix. We repeated this analysis for both cognate and noncognate words.
The results of the clustering analysis was plotted using the social network visualization software NetDraw (Borgatti et al., Reference Borgatti, Everett and Freeman2002). In addition, two procedures were followed: (a) an analysis of the association level between the English and the Spanish matrices by means of the quadratic assignment procedure (QAP), which is principally used to test the association between networks in Social Network Analysis (Borgatti et al., Reference Borgatti, Everett and Freeman2002), and (b) the calculation of the degree centrality of the concepts from both matrices so as to analyze the degree of association between concepts from both languages. The latter analysis was conducted in SPSS 19. The QAP procedure was used here to compute the correlation between two square matrices, English and Spanish in this case. The algorithm proceeds in two steps. In the first one, it computes Pearson’s correlation coefficient between corresponding cells of the two data matrices. In the second step, it randomly permutes rows and columns synchronously of one matrix and recomputes the correlation and other measures. This step is carried out thousands of times in order to compute the proportion of times that a random measure is larger than or equal to the observed measure calculated in Step 1. A low proportion (<.05) suggests a strong relationship between the matrices that is unlikely to have occurred randomly. The centrality degree calculates the degree and normalized degree centrality of each entity. The number of entities adjacent to a given entity in a symmetric matrix is the degree of that entity (Wasserman & Faust, Reference Wasserman and Faust1998).
Results
The vector comparison method showed that the average of the strongest link between a concept and its equivalent in the other language had a value of .7 in 87.21% of the cases (being 0 and 1 indicators of a null and a full relationship, respectively), while the remaining 12.79% had an average value of .5. In addition, and regarding concepts that did not coincide with its equivalent in the other language, differences between each concept’s highest associate and its equivalent in the other language were not very significant, reaching an average value of 0.11090, with a standard deviation of 0.094080 (Figure 1).
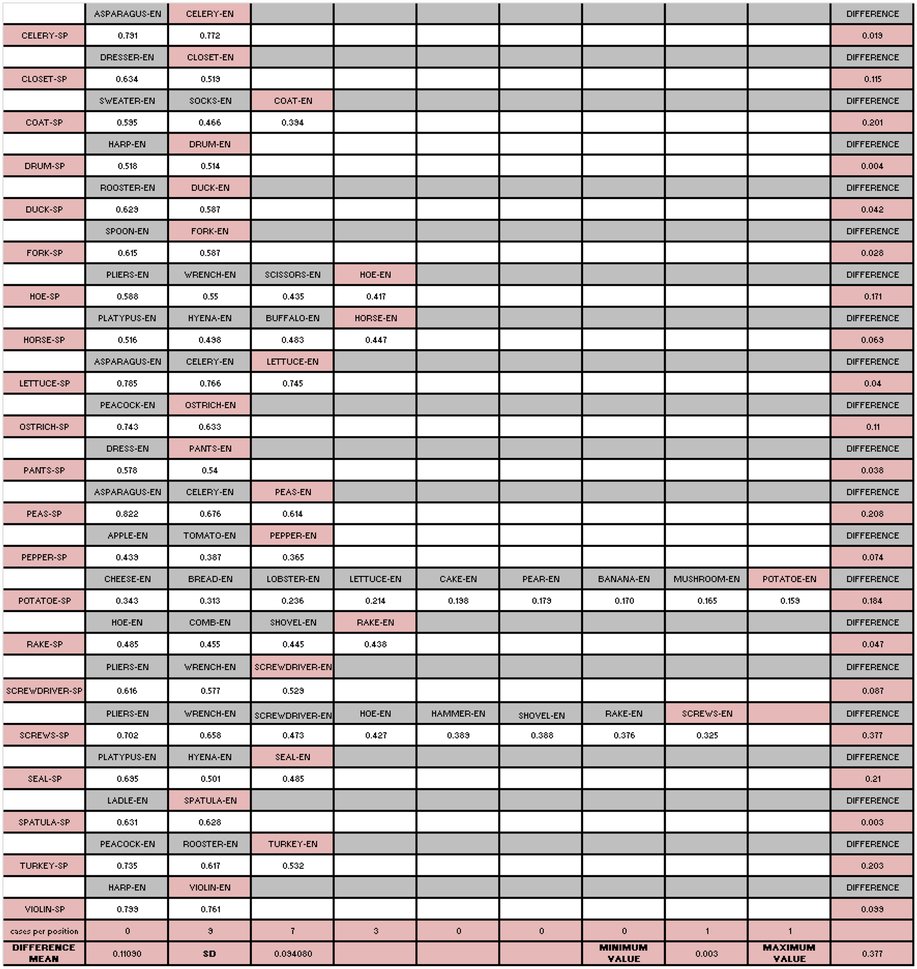
Figure 1. Extract of a transposed matrix of concepts that did not coincide with its equivalent in the other language, showing a continuum ranging from the value of its first associate to its equivalent in the other language.
Further, several cosine values of the interlinguistic relationships between different concepts are similar even intralinguistically. For example, the value for the words “ant” in English and “escarabajo” (beetle) in Spanish is .646, and the value for the words “hormiga” (ant) in Spanish and “beetle” in English is .444. Similarly, the intralinguistic value for the English and Spanish words “ant” and “escarabajo” (beetle) is .508 and .661, respectively.
The QAP analysis revealed a high level of association between concepts of both languages, showing a strong positive correlation between the two squared matrices (i.e., English and Spanish; r = .771, p = .001). The same pattern was observed for both cognates (r = .831, p = .001) and noncognates (r = .757, p = .001). A Fisher’s transformation test (Fisher, Reference Fisher1915) revealed that the difference between the correlation coefficients of cognates and noncognates was not significant (Z = 1.33, p = .0918). Typically, cognates are defined as translation equivalents that overlap in meaning and lexical form (Prior, MacWhinney, & Kroll, Reference Prior, MacWhinney and Kroll2007). In our study, the criterion used to classify words into cognates and noncognates was that they shared at least 40% of phonological overlap (e.g., barrel–barril, tomato–tomate). Furthermore, as can be seen in the hierarchical clustering (see Figure 2), concepts that share the same category are grouped together. Again, the same was found for both cognates (see Figure 3) and noncognates (see Figure 4).

Figure 2. Cluster diagram showing the links (in terms of cosine values) between concepts of the English (blue squares) and Spanish (red dots) semantic norms in our database. This diagram was plotted with NetDraw Software.

Figure 3. Cluster diagram showing the links (in terms of cosine values) between cognate concepts of the English (-EN) and Spanish (-SP) semantic norms in our database. This diagram was plotted with NetDraw Software.

Figure 4. Cluster diagram showing the links between noncognate concepts of the English (-EN) and Spanish (-SP) semantic norms in our database. This diagram was plotted with NetDraw Software.
Finally, due to the nonparametric distribution of cases, a Spearman correlation analysis of the degree of centrality of both networks was conducted. This represents an indirect measure of the amount of semantic features that each concept shares with the rest in the network. Results showed a strong positive correlation between the degree of centrality of both Spanish and English concepts that comprised the networks (r = .726, n = 219, p = .001).
Discussion
This work performed a cross-linguistic comparison of Spanish (Vivas et al., Reference Vivas, Vivas, Comesaña, García Coni and Vorano2017) and English (McRae et al., Reference McRae, Cree, Seidenberg and McNorgan2005) semantic feature production norms comprising 219 common concrete concepts. The geometric technique of vector comparison in the Euclidean n-dimensional space was used alongside the calculation of the network’s degree of centrality. We found that conceptual structure was similar in both languages independent of the cognate status of words, further suggesting the existence of some sort of core features across languages. Below we address these issues in turn.
Before discussing the results obtained in the present study, it is worth explaining what we mean by “coincidence with its equivalent.” Under this term, we include those cases in which a concept obtains its strongest link with its equivalent in the other language, that is to say, interlinguistic cases. Then, for example, “ashtray” from the English norm has its strongest interlinguistic link (value .7) with “cenicero” (ashtray) from the Spanish norm. In these cases, the strongest link, which never reaches a “value of 1” but averages values higher than .6, was found for the relationship with its equivalent in the other language in 87.21% of the cases.
Murphy (Reference Murphy2002, p.1) defines a concept as “a mental representation corresponding to that category (the class of objects in the world).” Moreover, he states that concepts “are a kind of mental glue” as they connect our previous experiences to our present and future interactions with objects. In this sense, we consider that the 87.21% of the interlinguistic coincidence found in our study gives support to the hypothesis we have put forward: as a concept-generation process, feature production norms would have at least some kind of universal core, independent of the language that uses it. The aforementioned hypothesis aligns with what Gentner and Goldin-Meadow (Reference Gentner, Goldin-Meadow, Gentner and Goldin-Meadow2003) have stated in relation to the dominant position within cognitive psychology in recent years, that (a) conceptual structure is relatively constant in its core features across cultures, and (b) conceptual structure and semantic structure are closely coupled.
We found a high percentage of coincidence between the cosine values of the English and Spanish concept equivalents. In addition, the QAP analysis showed a high correlation between both language networks even for both cognates and noncognates, further suggesting that this relationship is independent of the cognate status of words. Finally, though tentatively, we also observed some intralinguistic examples of concepts that exhibited similar values to the ones yielded in the interlinguistic analysis. For instance, the relationship between the cosine values of the words “ant” (hormiga) and “beetle” (escarabajo) is quite similar both inter- and intralinguistically. This information could be indicative of some sort of intra- and interlinguistic conceptual network (Lamas, Vivas, & Vorano, Reference Lamas, Vivas, Vorano and Vigaro2012; Vorano, Zapico, Corda, Vivas, & Vivas, Reference Vorano, Zapico, Corda, Vivas and Vivas2014), and could coincide with the proposal by Moss, Tyler, and Taylor (Reference Moss, Tyler, Taylor and Gaskell2009) when they state that in the framework of these models, concepts are represented as activation patterns of several nodes within a neural network; in other words, these nodes correspond to various semantic features.
As shown in the clusterization, concepts are grouped together by type of related category (musical instruments, things that fly, insects, fruits/vegetables, etc.), and this phenomenon is also evident with concepts that do not coincide with its equivalent in the other language. The same was found in the degree of centrality analysis that was carried out. Specifically, when degree of centrality values were organized in descending order, a clear distinction between living and nonliving things was observed, representing the highest and lowest values, respectively. Moreover, it is important to notice that within living things, the animal category was the one that shared the greatest number of attributes with the rest of the members in the network. This could also be considered another strong indicator not only for the existence of a conceptual core but also for the presence of another level of intra- and interlinguistic association that is not so specific but still constant.
These results are consistent with what Kremer and Baroni (Reference Kremer and Baroni2011) proposed, as they state that when considering language communities that share similar cultural foundations, research studies suggest that there are no remarkable differences across languages in relation to the semantic features used by native speakers to characterize concepts. In this sense, it is worth mentioning that these results may change dramatically if the languages compared entailed significant cultural differences. More interesting, the present study found few linguistic as well as cultural differences worth mentioning. For instance, one of these differences in our study is evident in the concept “pavo” (turkey) from the Spanish norm (Vivas et al., Reference Vivas, Vivas, Comesaña, García Coni and Vorano2017) that coincides in its higher value with the concept “peacock” from the English norm (McRae et al., Reference McRae, Cree, Seidenberg and McNorgan2005). The first cultural difference is evidenced by the fact that “turkey” is a typical everyday food in the United States, and a traditional Thanksgiving and Christmas dish. However, it is rarely eaten by Argentinians, from whom Spanish semantic features were collected. This difference generates variation in different characteristics of the conceptual structure, like “familiarity.” Second, both linguistic labels in English differ phonologically, “turkey” and “peacock,” which does not allow speakers to make associations at a phonological level. Conversely, the Spanish concepts “pavo” (turkey) and “pavo real” (peacock) are homonymous, with the exception that the latter concept is adjectivized, which would imply the access to a phonological level of association before other levels.
In contrast, there are 12.79% of concepts whose strongest link does not coincide with the equivalent in the other language. We believe, however, that this lack of coincidence does not contradict what we have claimed earlier about the existence of core features. As illustrated in Figure 1, for the Spanish concept “lechuga” (lettuce), the English equivalent, “lettuce,” occupies the third position, with a relationship value between each other of .745. This could be interpreted as saying that its semantic distance in relation to the Spanish concept “lechuga” (lettuce) is larger than the distance between “asparagus” in English and “celery” in English, with values of .785 and .766, respectively. Even though this result is correct, it is surprising that the difference between the highest value for “asparagus” and “lettuce,” which would be the corresponding one but occupies the third position, is only .04. If the column in Figure 1 labeled “difference” is analyzed, even though the maximum value for the concept “tornillo” (screws) is high (.377), in general, the differences were not very significant, reaching an average value of .11090, with a standard deviation of 0.094080, which would support our claim. Moreover, if the total values of cases per position are observed, it would be noted that the larger concentration is found in the second and third positions with 76% of the total, and that values decrease significantly and abruptly from the fourth column on. Once more, we consider this result to be indicative of the existence of strong interlinguistic relationships between concepts, even in cases where there is no coincidence. Finally, it is crucial to acknowledge that the present results may only apply to concrete concepts. As shown by some studies, abstract concepts might lack of conceptual core (Pulvermüller, Reference Pulvermüller2018) and exhibit remarkable processing differences (Bolognesi & Sten, Reference Bolognesi and Steen2018) compared to concrete concepts.
To sum up, and in line with the findings reported by Kremer and Baroni (Reference Kremer and Baroni2011) about the relationships between the semantic production norms in English, German, and Italian, the results of the present study show a general consistency in the distribution of patterns in English and Spanish. The 87.21% of the concepts analyzed in this study revealed the strongest interconceptual link with interlinguistic pairs with an average value of .7, being 0 and 1 indicators of a null and a full relationship, respectively.
Conclusion
A set of 219 concrete concepts common to both English and Spanish semantic feature norms was compared to assess whether concepts’ core features evidence universal or language-specific properties. Even though few linguistic as well as cultural differences were observed affecting the structuring of conceptual representation of concrete words, our results suggested that there are no remarkable differences across languages in terms of the semantic features used by native speakers to describe and characterize concrete concepts. In this sense, our findings are indicative of the existence of some sort of core features common to both languages.
Acknowledgment
This research was supported by National Agency for Scientific and Technological Promotion Grant PICT 2015-0983 (to J.V.).