Bilingualism is a complex and multifaceted life experience. The onset of bilingualism can occur at any stage in life, and it can extend into old age. Some bilinguals learn both languages at a young age in the home, and others learn their languages in adulthood when they immigrate to a new country. Language and neurocognitive processing are intimately related to the timing of bilingual language experience, such as age of second language (L2) acquisition (Berken, Chai, Chen, Gracco, & Klein, Reference Berken, Chai, Chen, Gracco and Klein2016; Berken, Gracco, Chen, & Klein, Reference Berken, Gracco, Chen and Klein2015; Flege, Munro, & Mackay, Reference Flege, Munro and Mackay1995; Gullifer et al., Reference Gullifer, Chai, Whitford, Pivneva, Baum, Klein and Titone2018; Johnson & Newport, Reference Johnson and Newport1989; Klein, Mok, Chen, & Watkins, Reference Klein, Mok, Chen and Watkins2014; Kousaie, Chai, Sander, & Klein, Reference Kousaie, Chai, Sander and Klein2017; Kousaie & Phillips, Reference Kousaie and Phillips2011). However, bilinguals also use their languages to various degrees and for a multitude of reasons, independent of the age at which they were acquired.
Sometimes, the communicative context or geographic location may dictate the use of one language or the other. For example, bilinguals living in English monolingual areas of the United States may use English primarily in the workplace. Bilinguals living in the French monolingual province of Quebec (Canada) are regulated to use French, at least initially, with customers in the workplace. At the same time, bilinguals in each of these areas may use both of their languages jointly in other settings, such as when speaking with bilingual friends or family. Theories, past and present, together with recent psycholinguistic research suggest that it is crucial to quantify these types of language experience and usage patterns in order to provide an adequate description of the core phenomena of interest, which include language processing, language learning, and language control (Abutalebi & Green, Reference Abutalebi and Green2016; Anderson, Hawrylewicz, & Bialystok, Reference Anderson, Hawrylewicz and Bialystok2018; Anderson, Mak, Keyvani Chahi, & Bialystok, Reference Anderson, Mak, Keyvani Chahi and Bialystok2018; Beatty-Martinez & Dussias, Reference Beatty-Martinez and Dussias2017; Beatty-Martinez et al., Reference Beatty-Martinez, Navarro-Torres, Dussias, Bajo, Guzzardo Tamargo and Kroll2020; Bice & Kroll, Reference Bice and Kroll2019; DeLuca, Rothman, Bialystok, & Pliatsikas, Reference DeLuca, Rothman, Bialystok and Pliatsikas2019; Green & Abutalebi, Reference Green and Abutalebi2013; Green & Wei, Reference Green and Wei2014; Grosjean, Reference Grosjean1985, Reference Grosjean2016; Gullifer et al., Reference Gullifer, Chai, Whitford, Pivneva, Baum, Klein and Titone2018; Gullifer & Titone, Reference Gullifer and Titone2020a, Reference Gullifer and Titone2020b; Sulpizio, Del Maschio, Del Mauro, Fedeli, & Abutalebi, Reference Sulpizio, Del Maschio, Del Mauro, Fedeli and Abutalebi2019).
It is now becoming clear that language experience is not a categorical phenomenon. Instead, it exists on a multidimensional spectrum, and the social factors surrounding language experience have consequences for language processing, cognitive processing, and brain organization (e.g., Anderson, Hawrylewicz, et al., Reference Anderson, Hawrylewicz and Bialystok2018; Baum & Titone, Reference Baum and Titone2014; Dash, Berroir, Joanette, & Ansaldo, Reference Dash, Berroir, Joanette and Ansaldo2019; DeLuca, Rothman, et al., Reference DeLuca, Rothman, Bialystok and Pliatsikas2019; DeLuca, Rothman, Bialystok, & Pliatsikas, Reference DeLuca, Rothman, Bialystok and Pliatsikas2020; Gullifer et al., Reference Gullifer, Chai, Whitford, Pivneva, Baum, Klein and Titone2018; Gullifer & Titone, Reference Gullifer and Titone2020a; Li, Legault, & Litcofsky, Reference Li, Legault and Litcofsky2014; Luk & Bialystok, Reference Luk and Bialystok2013; Sulpizio et al., Reference Sulpizio, Del Maschio, Del Mauro, Fedeli and Abutalebi2019; Tiv, Gullifer, Feng, & Titone, Reference Tiv, Gullifer, Feng and Titone2020). Given that these phenomena are studied in different locations around the world, a common quantification of these experiences is necessary to ensure comparability between studies.
Of relevance here, recent theoretical efforts to describe neurocognitive aspects of bi- and multilingualism invoke the idea of relative balance of use for each language (i.e., the distribution of first language [L1] vs. L2+ language experience), which may be assessed in addition to sheer language exposure. Thus, a key factor in assessing language experience would involve understanding whether people use some or all of the languages they know jointly in communicative contexts or separately. In line with this idea, various theoretical perspectives have converged upon similar ideas, but using different terminology. For example, the adaptive control hypothesis (Abutalebi & Green, Reference Abutalebi and Green2016; Green & Abutalebi, Reference Green and Abutalebi2013; Green & Wei, Reference Green and Wei2014) makes the distinction between single language contexts (where only one language is used), dual language contexts (where two languages are used, typically with different speakers), and dense code-switching contexts (where two languages are mixed within a single utterance). Dual language contexts are thought to involve greater competition between the two languages, requiring greater need for the application of control mechanisms. A related theory, Grosjean’s “language mode hypothesis” (Grosjean, Reference Grosjean1997, Reference Grosjean2001), conceptualized the idea of a bilingual mode and a monolingual mode, which, when entered, can up- or downregulate the activation of the unintended language. Grosjean states that these modes may not be completely independent and instead exist along a continuum.
Crucially, bilinguals differentially distribute their use of particular languages depending on the communicative context in question and even the topics of conversation that occur in that context (e.g., Grosjean, Reference Grosjean1998, Reference Grosjean2016; Schrauf, Reference Schrauf2002, Reference Schrauf2009; Tiv, Gullifer, et al., Reference Tiv, Gullifer, Feng and Titone2020; Vaid & Menon, Reference Vaid and Menon2000). Grosjean refers to this observation as the complementarity principle, and he notes that each individual may exhibit an entirely unique pattern of language distribution. For example, in Montréal, Quebec, a participant may report using only French in the home, and report using both French and English in social situations. In contrast, another participant may report using both languages throughout all of their communicative contexts.
In some cases, the communicative context may place specific demands on the speaker. Provincial regulations in Quebec stipulate that French is the prominent language of business and commerce, and thus most work environments in the bilingual city of Montréal require knowledge and use of French. In the Montréal context, we consistently find distinct patterns of language distribution between home and work contexts (Gullifer & Titone, Reference Gullifer and Titone2020a; Tiv, Gullifer, et al., Reference Tiv, Gullifer, Feng and Titone2020), though we note that sometimes work contexts are reported to be quite bilingual, counter to official regulations. In other cases, language choice may be under complete control of the speaker, such as the use of language for purposes of inner thought and mental computations (e.g., dreaming, thinking, counting, and arithmetic). Although bilinguals differ in the extent to which they report using each of their languages for various purposes, individual differences in language usage in various communicative contexts are differentially associated with several aspects of bilingual language experience, including length of residence in a country, L2 age of acquisition (AoA), self-reported language proficiency, objective language proficiency, and language dominance (e.g., Gullifer & Titone, Reference Gullifer and Titone2020a; Schrauf, Reference Schrauf2009; Tiv, Gullifer, et al., Reference Tiv, Gullifer, Feng and Titone2020; Vaid & Menon, Reference Vaid and Menon2000). Of importance, these observations have profound implications for assessing language proficiency.
Minimally, language proficiency reflects one’s ability to apply language knowledge for the purposes of comprehension and production. Although many measures of language proficiency probe various linguistic domains (e.g., lexical and syntactic domains), within-domain proficiency is often treated in a global sense, without regard for the communicative context in which the language is used. However, because bilinguals distribute their use of particular languages differentially throughout various social and communicative contexts, proficiency for a given language may develop uniquely in some contexts. As a simple example, consider a French–English bilingual who uses French but not English in the home. This individual may have greater difficulty accessing English vocabulary knowledge for concepts that typically occur in the home (e.g., terms for cooking appliances), which could manifest as slower reaction times on a picture naming test for those items or fewer exemplars produced in a verbal fluency task. Similar difficulties could extend to French vocabulary knowledge for concepts that occur outside the home. In other words, “people become bilingual by domain and not globally” (Schrauf, Reference Schrauf2009).
It thus follows from these theories that fluent language production, comprehension, and associated control processes may become adapted to the social context of language usage. In order to properly assess how bilingual (or multilingual) experiences contribute to these adaptive processes, it is necessary to assess multiple aspects of language experience, including age of exposure, amount of sheer language usage, and language balance, across several social and communicative contexts. Despite the existence of highly sophisticated methods for quantifying behavior and brain function, and detailed questionnaires that probe various experiences with language, there is a lack of validated analysis routines that allow researchers to precisely quantify the rich and diverse nature of people’s experiences using language.
To address these issues, which have led to persistent gaps in the literature, we proposed a method for assessing language balance in various communicative contexts among multilingual participants called language entropy (Gullifer et al., Reference Gullifer, Chai, Whitford, Pivneva, Baum, Klein and Titone2018; Gullifer & Titone, Reference Gullifer and Titone2018, Reference Gullifer and Titone2020a, Reference Gullifer and Titone2020b).Footnote 1 The general concept of entropy comes from physics and information theory, and it provides a measure of diversity and uncertainty when the relative proportion of occurrences for a set of “states” is known (e.g., Shannon, Reference Shannon1948). Here, we take “states” to be the usage of a particular language (e.g., English or French) within a communicative context (e.g., at home or at work). This information is frequently elicited directly by language background questionnaires, or it can be otherwise computed from questions probing language usage, and it can be used to compute an entropy score directly for each probed context. To reduce the number of variables and identify the core latent constructs that potentially drive language entropy variables, we use latent variable analysis.
We argue that language entropy can continuously estimate the extent to which individuals immerse themselves in dual versus single language contexts (or language mode; Gullifer et al., Reference Gullifer, Chai, Whitford, Pivneva, Baum, Klein and Titone2018; Gullifer & Titone, Reference Gullifer and Titone2018, Reference Gullifer and Titone2020a, Reference Gullifer and Titone2020b). To illustrate language entropy, imagine two hypothetical people, William and Zoe. William reports hearing only English in the home and never French (100% English, 0% French; a single language context that may induce a monolingual mode). William would receive a low language entropy score in the home (i.e., language entropy of 0), reflecting low language-related diversity and low language-related uncertainty in the home. Thus, at any given moment in the home, William is quite certain that he would hear English (and not other languages). In contrast, Zoe reports equal exposure to English and French in the home (50% French, 50% English; a dual language context that may induce a bilingual mode). Zoe would receive a higher score (i.e., language entropy of 1), reflecting higher language-related diversity and uncertainty in the home. In other words, at any given moment in the home, Zoe may be highly uncertain about whether French or English will be used next (in the absence of other information that could provide a cue about language, such as speaker identity) due to higher diversity in language exposure compared to William.
Our initial work shows that for a sample of over 400 Montréalers, language entropy is composed of two core latent constructs (Gullifer & Titone, Reference Gullifer and Titone2020a, Reference Gullifer and Titone2020b): general entropy (composed of variables related to reading, speaking, entropy at home, and entropy for social purposes) and work-related entropy (composed of variables related to entropy at work with some cross-loading from social entropy). We then show that individual scores on these constructs are associated with self-reported language proficiency (such as L2 abilities and L2 foreign accentedness; Gullifer & Titone, Reference Gullifer and Titone2020a) and with proactive executive control abilities and associated brain networks (Gullifer et al., Reference Gullifer, Chai, Whitford, Pivneva, Baum, Klein and Titone2018; Gullifer & Titone, Reference Gullifer and Titone2020b), in line with predictions of theoretical models outlined above. Work by others shows that language entropy is related to patterns of dual language use such as engagement in language mixing (Kałamała, Szewczyk, Chuderski, Senderecka, & Wodniecka, Reference Kałamała, Szewczyk, Chuderski, Senderecka and Wodniecka2020), the ability to mentalize (or engage in social–cognitive processing) in the first and second languages (Tiv, O’Regan, & Titone, Reference Tiv, O’Regan and Titone2020), and the organization of brain networks responsible for language and executive control (Sulpizio et al., Reference Sulpizio, Del Maschio, Del Mauro, Fedeli and Abutalebi2019).
Importantly, as raised earlier, bilingualism is a multifaceted language experience, and thus several other constructs must be assessed besides language entropy, such as historical language exposure (e.g., L2 AoA) and sheer exposure to the second language. The relative import of each of these variables may depend on the domain of inquiry (e.g., language proficiency vs. language/executive control), the bilingual or multilingual population in the sample, and the communicative context of language usage. Thus, in our view, these constructs should be investigated with continuous measures that are assessed jointly. This allows for the estimation of the unique explanatory power of each construct that indexes bilingual language experience. An emerging picture from recent work on joint contributions suggests that several factors (i.e., L2 AoA, sheer L2 exposure, and language entropy) predict self-reported language proficiency (Gullifer & Titone, Reference Gullifer and Titone2020a), but language entropy best predicts proactive executive control (Gullifer & Titone, Reference Gullifer and Titone2020b).
Critically, our prior work has limitations that we identify here, some of which we are able to directly address. First, Gullifer and Titone (Reference Gullifer and Titone2020a) relied primarily upon self-reported measures of language abilities as a proficiency estimate. Although there are some demonstrations that self-reported measures correlate well with objective measures of proficiency (e.g., Blanche & Merino, Reference Blanche and Merino1989; Jia, Aaronson, & Wu, Reference Jia, Aaronson and Wu2002; Schrauf, Reference Schrauf2009), there are concerns that self-ratings may be poor indicators of proficiency. Participants may not be able to accurately assess their own language abilities, or they may up- or down-weight their abilities to adhere to cultural norms and expectations (Tomoschuk, Ferreira, & Gollan, Reference Tomoschuk, Ferreira and Gollan2019; Zell & Krizan, Reference Zell and Krizan2014). Thus, there may be inherent issues using self-ratings as indicators of language proficiency when comparing across populations. However, as described earlier, objective proficiency measures may also be fundamental indicators of social and cultural patterns of language use. Similar critiques about validity can be made for other aspects of language experience that rely on self-report, including L2 AoA, sheer L2 exposure, and language entropy. In our view, in the absence of explicit validation of these constructs, the best approach is to continue assessing the interrelationships between these constructs, including objective measures.
Second, in our previous work, the assessment of the communicative contexts in which language is used was highly coarse. For instance, we assessed language entropy in a small number of communicative contexts (e.g., at home, at work, in social settings, for reading, and for speaking). Yet, individuals engage in many more contexts of language use throughout their day. This includes the use of language for mental processes and computations such as thinking, dreaming, arithmetic, and counting (e.g., Schrauf, Reference Schrauf2009; Vaid & Menon, Reference Vaid and Menon2000); language use in other communicative contexts, such as in the university environment; and language use for consumption of media (e.g., television, radio, and internet). The importance of assessing language usage across several communicative contexts, and in particular the ways bilinguals and multilinguals use their languages flexibly and integratively within these contexts (see e.g., translanguaging; García & Wei, Reference García and Wei2012), has long been known from work within and at the intersection of sociolinguistics, applied linguistics, and bilingualism (e.g., Commins, Reference Commins1989; García & Wei, Reference García and Wei2012; Schrauf, Reference Schrauf2009; Vaid & Menon, Reference Vaid and Menon2000). These approaches are gaining recognition in the cognitive sciences more generally (e.g., Anderson, Hawrylewicz, et al., Reference Anderson, Hawrylewicz and Bialystok2018; Anderson, Mak, et al., Reference Anderson, Mak, Keyvani Chahi and Bialystok2018; DeLuca et al., Reference DeLuca, Rothman, Bialystok and Pliatsikas2019; Grosjean, Reference Grosjean2016).
Thus, our purpose here is to further assess the structural properties of and interrelationships between several constructs related to bilingual language experience, including the timing of language exposure, language entropy, sheer amount of exposure to the L2, and language proficiency. We extracted this information from several measures on a health and language background questionnaire. To assess the timing of L2 exposure, we extracted information from an item that probed L2 AoA. To assess language entropy and sheer L2 exposure, we extracted information about language usage from multiple items that tap into different communicative contexts of language use. To assess language proficiency, we extracted verbal fluency production data (objective proficiency) and self-rated language abilities (subjective proficiency).
We then computed factor analyses and resultant factor scores for each of the constructs with multiple items: language entropy, sheer L2 exposure, and language proficiency. The factor analyses provide information about the latent structure of each facet of bilingual language experience. Next, we extract individual scores for each latent factor and model the extent to which the scores on these factors can jointly classify the timing of language exposure in a grouped manner (simultaneous acquisition, sequential L1 French, or sequential L1 English). Finally, we model whether combinations of factor scores for language entropy, sheer L2 exposure, and L2 AoA (as a continuous measure) relate to factor scores for language proficiency. This approach addresses some of the limitations of previous work; namely, we included objective measures of language proficiency together with subjective measures. Moreover, our questionnaire probed estimated language usage in 16 communicative contexts, including for internal purposes (for things like thinking and dreaming), use in various social settings, and use for media consumption.
To preview the findings reported below, we show that language entropy and sheer L2 exposure comprise similar latent structures, involving two core latent variables: (a) a factor that includes measures of internalized language use (such as thinking, dreaming, and counting) and social language use; and (b) a factor that includes measures related to externally directed or professional use. Language entropy (but not sheer L2 exposure) also includes a third factor that reflects measures of media usage. Crucially, we then showed that factor scores for sheer L2 exposure (but not language entropy) discriminate whether individuals acquired their languages simultaneously or sequentially.
Next, we assessed relationships with language proficiency. We show that language proficiency comprises three latent variables: (a) a factor that includes measures of objective and subjective L2 proficiency, (b) a factor that includes measures of subjective L1 proficiency, and (c) a factor that includes measures of objective L1 proficiency. Factor scores for both sheer L2 exposure and language entropy were important in predicting factor scores for L2 proficiency. In contrast, only factor scores for sheer L2 exposure predicted factor scores for subjective L1 proficiency. There were no significant predictors of factor scores for objective L1 proficiency. Together, these results demonstrate how bilingual language experience can be quantified jointly and continuously to predict other constructs of interest. Moreover, they also show the differential contributions of each construct related to bilingual language experience, depending on the outcome measure and also the communicative context in which language is used.
Method
Participants
We tested 87 bilingual speakers of French and English who completed a health and language background questionnaire as part of their participation in a larger project. Participants were young adults (M age = 23.8 years, SD age = 4.1 years) from the Montréal area, and many were university students. The majority of participants were born in Canada (n Canada = 75). Some of the participants were born outside of Canada and subsequently moved to Canada at an early age (n France = 3; n USA = 2; n China = 1; n Zimbabwe = 1; n Australia = 1; n unknown = 4). The speakers differed with respect to the languages that they first acquired: 27 acquired French and English simultaneously, 34 English followed by French sequentially, and 26 acquired French followed by English sequentially. On average, participants acquired their L2 early in life (M L2AoA = 3.8 years SD L2AoA = 3.2 years). Participants’ first language was determined through inspection of language history questions that probed age of language onset. Some participants (n = 15) reported current exposure to a third language (L3) besides English or French. On average, for conversations, participants reported using the L1 64% of the time and the L2 35% of the time. Among participants with and L3, they reported using it 5% of the time. Of note, average proportions do not sum to 100% because not all participants spoke an L3. Participants came from generally high socioeconomic backgrounds as measured by highest parental education. Among the available data on parental education, 73 participants had parents with at least some college education. See Table 1 for a summary of demographic and language history data. All participants gave informed consent, and the McGill Research Ethics Board approved data collection for any linked projects.
Table 1. Summary of participant characteristics, including demographic, language history, and verbal fluency data

Materials
Assessing self-reported language exposure
All participants included in this sample completed a health and language background questionnaire. This questionnaire has sections that probe basic demographic information (e.g., age, gender, and education), language background and experience (including language use and exposure for various purposes and in various communicative contexts, self-reported language abilities, etc.), and basic health information (e.g., data collected to assess eligibility for magnetic resonance imaging studies). This questionnaire was adapted from various questionnaires used in the field, including the Language Experience and Proficiency Questionnaire (Marian, Blumenfeld, & Kaushanskaya, Reference Marian, Blumenfeld and Kaushanskaya2007) and the Language History Questionnaire 2.0 (Li, Zhang, Tsai, & Puls, Reference Li, Zhang, Tsai and Puls2014), and questions were adapted to apply to the Montréal and Canadian contexts. We detail the extraction of these measures below.
Assessing self-reported age of language acquisition
Participants answered several questions about the AoA for all of the languages that they knew. Participants were asked: “At what age did you first start to learn each language in terms of speaking (at what age did you speak your first words?), reading, and writing, and the number of years you have spent learning each language.” They were also asked: “Please indicate the age at which you started to learn each language in the following situations—indicate the age in the boxes only for situations that are relevant: at home, at school, after immigrating to the country where spoken, informal settings (e.g., nannies or friends), software (e.g., Rosetta Stone), other.” Through visual inspection of these data, we determined AoA for each language based on the earliest age that the participant entered. If the AoA was the same for multiple languages, we determined that they acquired the languages simultaneously. In this case, L1/L2/L3 were determined on the basis of self-reported proficiency. If the AoA was different for multiple languages, then the L1/L2/L3 were determined on the basis of AoA.
Assessing self-reported language usage among communicative contexts
Participants reported the extent to which they used their languages in 16 different communicative contexts with one of two measurement types. The communicative contexts together with their measurement types are listed in Table 2. Measurement types included either a percentage of language use (0%–100%) or 7-point Likert scale where 1 indicated no use and 7 indicated use always. Language use related to inner thoughts and for the purposes of emotion or anger was elicited with the following prompt: “How often do you use your languages for the following activities? Use the following scale and fill in the number in the table. [1 (never)–7(always)].” Language use for a set of questions that generally probed passive language use (e.g., listening, reading, and writing) was elicited via the following prompt: “Please estimate the total number of hours each day that you spend engaged in the following activities, and indicate what percentage of that time you spend engaged in that activity in each of the languages that you know (please write down the languages). If you are not currently engaged in an activity using that language write ‘0’; the total percentage for each activity should equal 100%.” Language use related to conversations in different social contexts was elicited via the following prompt: “Please estimate the percent of conversations that take place in each of your languages, and what percentage of that is with the following people. The total across languages should equal 100% and the total within each language should equal 100%.”
Table 2. Communicative contexts and measurement types elicited by the language history questionnaire. Information was elicited for the L1, L2, and L3

The computation of language entropy requires the proportion of language usage within each communicative context to be known. Percentages are easily converted to proportions by dividing by 100. Likert scales were converted to proportions with the following procedure. We first subtracted 1 from each elicited score, so that the value for “never” would be adjusted to 0. We then divided a language’s score within a context by the sum total of the scores across languages within that communicative context. For example, a participant who reported (after adjustment) the following data for dreaming “L1: 6” and “L2: 5” would receive the following proportions for dreaming L1: “6/11” and “L2: 5/11.”
A careful reader will notice that the wording of questions about passive language use differed from the wording of questions about conversations in social contexts. Specifically, for passive language use, participants were told to sum language use for each activity to 100% (e.g., Reading for fun––French: 50%, English: 50%, total: 100%). This wording is ideal for the computation of language entropy for each activity. In contrast, for conversations in various social contexts, participants were asked to sum use with each interlocuter type to 100% within a language (e.g., English use––with family: 10%, with friends: 40%, with classmates: 20%, with coworkers: 30%, total: 100%). This wording is less ideal for the computation of language entropy for each interlocuter type (though it could, in principle, be used to compute a type of interlocuter entropy within language). Crucially, because participants reported the percentage of all conversations that occur in each language across interlocuter types (e.g., French: 80% and English: 20%), we could derive the percentage of language use within each interlocuter type.
To derive the percent of language use within interlocuter type, we conducted the following procedure. We multiplied the proportion of usage for each interlocuter type within a language (e.g., English use with family) by the proportion of overall usage of that language (e.g., English use overall), yielding proportions of use that sum to 1 across all languages and interlocuter types. Then, within each interlocuter type, we divided each proportion by the sum total proportion within the interlocuter type, yielding proportions of usage for each language that sum to 1 within an interlocuter type.
Once all of the data were in common proportions, tracking language use in each context, we computed language entropy for each communicative context/interlocuter type. We also retained proportions of language use specific to the L2 for each context, and we used these data to index sheer L2 exposure.
Computing language entropy
For each of the 16 communicative contexts, we computed language entropy (H) with the following equation
$H = - \mathop \sum \nolimits_{i = 1}^n {P_i}lo{g_2}\left( {{P_i}} \right)$
using the methods available in the languageEntropy R package (Gullifer & Titone, Reference Gullifer and Titone2018). In this equation, n represents the total possible languages within a context and P
i
is the proportion that language
i
is used within a context. Thus, if a bilingual reported using French 80% of the time and English 20% of the time within the work context, one computes language entropy by summing
$0.80 \times lo{g_2}\left( {0.80} \right)$
and
$0.20 \times l{o_2}\left( {0.20} \right)$
, and then multiplying by –1 to yield a positive language entropy value. Language entropy for this hypothetical individual’s work context is 0.72.
Theoretically, the entropy distribution has a minimum value of 0 when the proportion of usage for one language is 1.0 (and the other is 0), representing completely compartmentalized language usage within a communicative context. The distribution has a maximum value equal to log n (1 for two languages and approximately 1.585 for three languages) when the proportion of use for each language is equal, representing completely integrated language usage within a communicative context. This procedure resulted in 16 entropy scores for each participant that pertained to language entropy in each of the 16 communicative contexts.
Assessing objective language proficiency
Objective language proficiency was assessed through performance on verbal fluency tasks. Participants completed category and letter fluency tasks in French (category: fruits, letters: F, L, and P) and English (category: animals, letters: A, F, and S). In each portion of a task, they were asked to name as many exemplars as possible in 60 s, drawn from each category or letter. We computed the mean number of exemplars produced in each language across letter prompts, and because participants only completed one category in each language, we used the number of exemplars produced.
Assessing subjective language proficiency
Subjective language proficiency was extracted from the health and language background questionnaire (i.e., items probing language abilities). Participants reported their language abilities in the L1 and L2 for reading, writing, speaking, and listening. These data were elicited with the following prompt: “Please rate your current ability in reading, writing, speaking, and listening for all languages that you know according to the following scale [1-very poor—7-native-like].” They also reported the extent to which they believe they have an accent when speaking via the following prompt: “Do you have a foreign accent in the languages that you speak? Please rate how strong you think your accent is according to the following scale [1-none—7-extremely strong].” Of note, participants did not regularly report accentedness in the L1, and as such, we extracted only accentedness in the L2.
Results
After extracting and computing language entropy, sheer L2 exposure, and language proficiency (verbal fluency performance and self-rated abilities), we prepared each data set for factor analysis. A primary concern was how to handle missing observations. All of the 16 communicative contexts measuring language usage and exposure (used to compute language entropy and sheer L2 exposure) had some missing observations (there were only 17 complete cases). Table 3 illustrates the number of missing cases for each communicative context. Missing data could have been due to many reasons, such as participants not engaging in a particular context (e.g., not listening to radio or TV) or reporting exposure with a specific language in that context (e.g., only listening to the radio/TV in their first language). We could not omit missing values, as the sample size would not be adequate for analysis, and omitting outliers can introduce statistical bias. Language entropy can be successfully computed when particular languages are not used within a communicative context, as the missing language(s) will be assumed to have exposure of 0. However, for instances where participants did not engage in a certain context at all with any language, language entropy would be unknown. Thus, we imputed language entropy data by partial mean matching using the methods available in the mice package in R (van Buuren & Groothuis-Oudshoorn, Reference van Buuren and Groothuis-Oudshoorn2010). We did not impute values for sheer L2 exposure, and instead assumed that an unknown value was 0 in that context (similar to the assumptions of the language entropy method).
Table 3. The number of missing cases for each communicative context

After preprocessing the data, we conducted factor analyses separately for language entropy, sheer L2 exposure, and language outcome data sets using methods available in the psych package (Revelle, Reference Revelle2017). The number of factors was determined through visual inspection of a parallel plot (via fa.parallel). For each analysis, we computed two goodness of fit metrics: the Kaiser–Meyer–Olin measure (KMO) and Bartlett’s test. We assumed that factors were likely correlated, and thus we used oblique, oblimin, rotations.
What is the latent structure for language entropy?
We conducted a factor analysis on language entropy data for the 16 communicative contexts using the procedures outlined above. Goodness of fit metrics indicated that the language entropy data were factorable, Bartlett’s test: χ2 (120) = 607.785, p < .001; KMO: 0.70. Of note, three items dropped below the 0.50 KMO threshold for sampling accuracy (language entropy for radio/TV, language entropy for writing papers, language entropy with colleagues). We determined that a three-factor solution was ideal to model language entropy data, and we thus computed an oblique factor analysis with three factors that, together, explained 42% of the variance. Factors were correlated at |r| <= .18.
Factor loadings for language entropy are illustrated in Figure 1. The first factor (entropyF1: 24.3% of the variance) appears to be related to language entropy for speaker internal purposes (e.g., counting, inner speech, dreaming, and thinking) and when communicating with friends and family. The second factor (entropyF2: 9.7% of the variance) appears to be related to language entropy for externally directed or professional purposes. The third factor (entropyF3: 8.4% of the variance) appears to be related to language entropy for consumption of media.

Figure 1. Illustration of latent structure for language entropy. The vertical axis depicts each communicative context for which language entropy was computed. The horizontal axis depicts the factor loading. Each factor (entropy F1, entropy F2, and entropy F3) is displayed as a separate panel. The entropy F1 factor appears to index language entropy for internal purposes or with friends and family. The entropy F2 factor appears to index language entropy in professional settings or for externally directed purposes. The entropy F3 factor appears to index language entropy for media consumption.
What is the latent structure for sheer L2 exposure?
We next conducted a factor analysis on language history data that probed sheer exposure to the L2 in the 16 contexts above (percentage data and Likert data were both transformed to proportions of L2 usage), using the procedures outlined above. Tests indicated that the correlation matrix was factorable: KMO measure for the correlation matrix was 0.82, and no items dropped below the 0.50 threshold for sampling accuracy; Bartlett’s test χ2 (120) = 733.848, p < .001. We determined that a two-factor solution was ideal to model sheer L2 exposure data, and thus we computed an oblique factor analysis with oblimin rotation with two factors that explained 42% of the variance. The two factors were highly correlated at r = .48.
Factor loadings for L2 exposure are illustrated in Figure 2. The first factor (exposure F1: 21.7% of the variance) appears to be related to L2 use for speaker internal purposes (e.g., counting, inner speech, dreaming, and thinking). The second factor (exposure F2: 20.6% of the variance) appears to be related to L2 use for externally directed or professional purposes.

Figure 2. Illustration of latent structure for sheer L2 exposure. The vertical axis depicts each communicative context for which L2 exposure was extracted. The horizontal axis depicts the factor loading. Each factor (exposure F1 and exposure F2) is displayed as a separate panel. The exposure F1 factor appears to index L2 usage for internal purposes. The exposure F2 factor appears to index L2 usage in professional settings or for externally directed purposes.
What is the latent structure for language proficiency?
We then conducted a factor analysis on language proficiency data (objective: category and letter fluency in L1 and L2; subjective: accentedness in L2, writing ability in L1 and L2, reading ability in L1 and L2, speaking ability in L1 and L2, and listening ability in L1 and L2), using the procedures outlined above. Tests indicated that the correlation matrix was factorable: KMO measure for the correlation matrix was 0.71, and no items dropped below the 0.50 threshold for sampling accuracy; Bartlett’s test χ2 (120) = 607.785, p < .001. We determined that a three-factor solution was ideal to model proficiency data, and thus we computed an oblique factor analysis with oblimin rotation with three factors that explained 57% of the variance. Factors were correlated at |r| <= .13.
Factor loadings for objective and subjective proficiency are illustrated in Figure 3. The first factor (proficiency F1: 26.8% of the variance), appears to be related to a combination of objective and subjective proficiency in the L2. The second factor (proficiency F2: 18.2% of the variance) appears to be related to subjective proficiency in the L1. The third factor (proficiency F3: 12.1% of the variance) appears to be related primarily to objective proficiency in the L1 with cross-loading from L2 letter fluency.
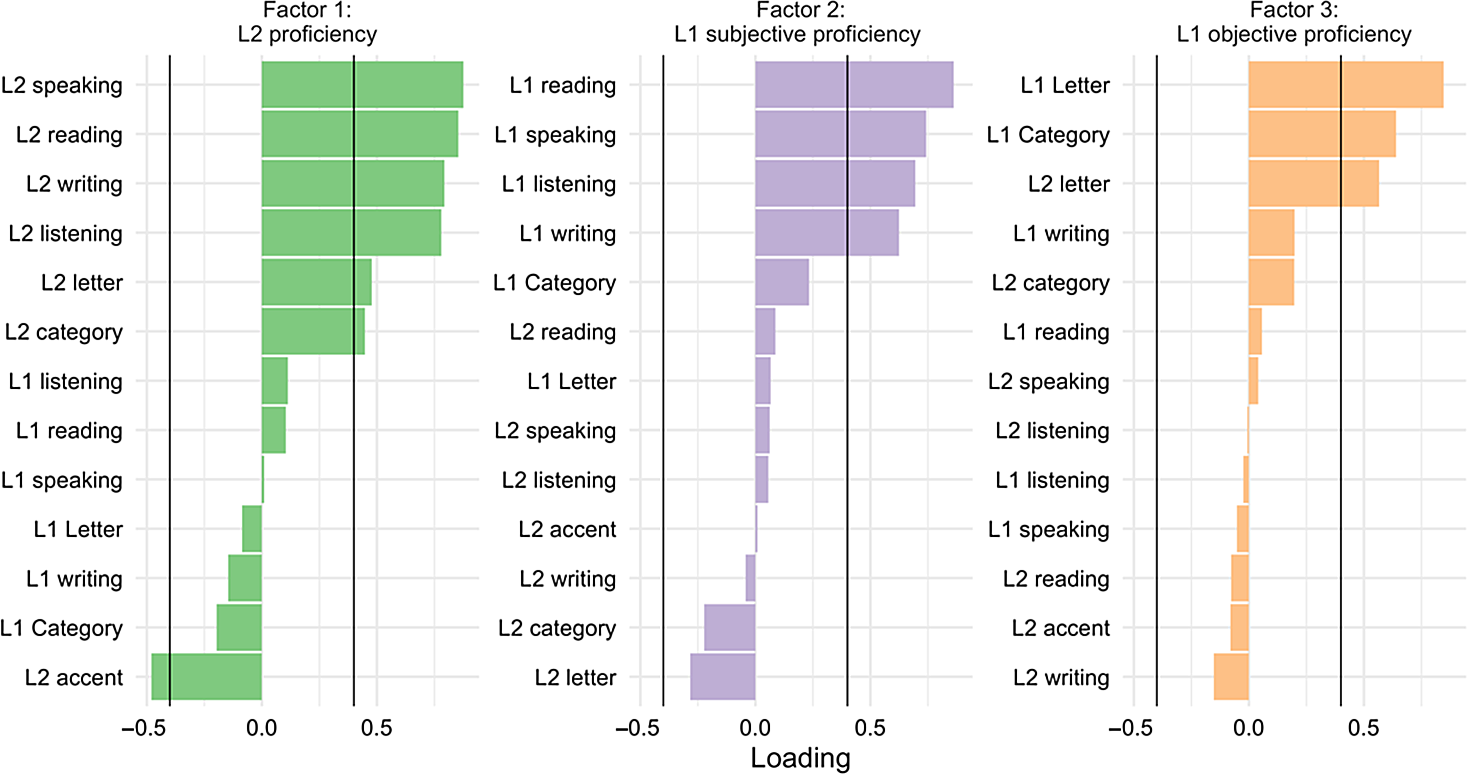
Figure 3. Illustration of latent structure for language outcomes. The vertical axis depicts each language outcome measure (e.g., letter fluency, category fluency, or self-rated ability item). The horizontal axis depicts the factor loading. Each factor (proficiency F1, proficiency F2, and proficiency F3) is displayed as a separate panel. The proficiency F1 factor appears to index subjective and objective L2 proficiency. The proficiency F2 factor appears to subjective L1 proficiency. The proficiency F3 factor appears to index objective L1 proficiency.
Does information about L2 exposure, language entropy, or language proficiency predict timing of language acquisition (simultaneous vs. sequential bilingualism)?
Once we had assessed the latent structures for sheer L2 exposure, language entropy, and language proficiency, our next goal was to determine whether individual scores on these factors jointly predict acquisition history (i.e., simultaneous, L1 English, or L1 French). Figures 4–6 illustrate the distributions of factor scores (Figure 4: L2 exposure, Figure 5: language entropy, and Figure 6: language proficiency) across the three acquisition groups (simultaneous, L1 English–L2 French, and L1 French–L2 English). To assess whether factor scores could discriminate acquisition group membership, we conducted multinomial regression (multinom function in the nnet package; Ripley, Reference Ripley2002). We constructed seven models to predict acquisition group (three levels: simultaneous, English L1, and French L1) as a function of various combinations of the continuous factor scores. We selected the model that minimized the Akaike information criterion (AIC) as the best fitting model (see Table 4).

Figure 4. Distribution of language entropy factor scores for each acquisition group (simultaneous bilinguals; L1 English–L2 French bilinguals; L1 French–L2 English bilinguals). Boxplots and violin plots are displayed; square points illustrate group means.

Figure 5. Distribution of sheer L2 exposure factor scores for each acquisition group (simultaneous bilinguals; L1 English–L2 French bilinguals; L1 French–L2 English bilinguals). Boxplots and violin plots are displayed; square points illustrate group means.

Figure 6. Distribution of language proficiency factor scores for each acquisition group (simultaneous bilinguals; L1 English–L2 French bilinguals; L1 French–L2 English bilinguals). Boxplots and violin plots are displayed; square points illustrate group means.
Table 4. Model comparisons for multinomial regressions predicting acquisition history (L1: simultaneous, L1 French, or L1 English) as a function of factor scores related to individual differences in sheer L2 exposure, language entropy, and language proficiency

Note: AIC is depicted for each model. The best model with the lowest AIC is bolded. The difference between AIC for each model and the AIC for the best model is also depicted.
The best model included factor scores for sheer L2 exposure and language proficiency but no scores for language entropy (AICbest = 145.3). However, comparing the AIC of the best model to the other models indicated that two other models may also be considered viable (see, e.g., Burnham & Anderson, Reference Burnham and Anderson2004): there was “substantial support” (i.e., AICdiff < 2) for the full model that included all factor scores (including language entropy; AICdiff = 1.7) and “strong support” (2 < AICdiff < 4) for the model that contained factor scores for sheer L2 exposure alone (AICdiff = 2.8). All other models exhibited considerably less support (i.e., AICdiff > 4).
We inspected the best model to determine the direction of the effects. We found that scores on the externally directed or professional factor (exposure F2) and the subjective L1 proficiency factor (proficiency F2) discriminated between the groups. Specifically, individuals with higher exposure F2 scores were more likely to have French as the L1 (b = 1.54, 95% confidence interval; CI [0.49, 2.58], z = 3.706, p < 0.01) and less likely to have English as the L1 (b = –1.26, 95% CI [–2.49, –0.04], z = –3.474, p < 0.05) relative to simultaneous bilinguals. Individuals with higher proficiency F2 scores were less likely to have French as the L1 (b = –1.08, 95% CI [–2.12, –0.04], z = –2.179, p < 0.05) relative to simultaneous bilinguals. See Table 5 for the full results of the best model.
Table 5. Summary table for the best model (Model 5)

*p < .05. **p < .01.
Does information about acquisition history, L2 exposure, or language entropy predict language proficiency?
Our next goal was to determine whether individual factor scores for sheer L2 exposure, language entropy, and acquisition history (continuous effect of L2 AoA) jointly predict language proficiency (composed of the set of three-factor scores representing: L2 proficiency, subjective L1 proficiency, and objective L1 proficiency). Thus, we conducted three sets of linear regressions for each language outcome component, with four models specified in each set. All regressions included an effect of L2 AoA. However, within each set of regressions, there were four parameterizations to assess the relative importance of entropy factor scores and exposure factor scores (separately and together), and to assess interactions between L2 AoA and the factor scores. For each of the three language proficiency factor scores, we selected the model that minimized AIC as the best fitting model. Model parameterizations and comparisons (with AIC) are illustrated in Table 6.
Table 6. Model comparisons for linear regressions predicting language proficiency factor scores as a function of factor scores related to individual differences in sheer L2 exposure, language entropy, and L2 AoA

Note: Separate models were run for each of the three factor scores: L2 proficiency (DV = proficiencyF1), L1 subjective proficiency (DV = proficiencyF2), and L1 objective proficiency (DV = proficiencyF3). AIC is depicted for the model of each fluency factor score. The best models (lowest AIC) are bolded.
For models predicting L2 proficiency (proficiency F1), the best model included additive effects of sheer L2 exposure and language entropy scores (AICbest = 190.7). Of note, another model with only sheer L2 exposure scores also received “strong support” (AICdiff = 2.5). See Table A.1 for the summary results of the four models. We inspected the best model to determine the direction of the effects. In this model, there was a significant effect related to factor scores for language entropy for externally directed or professional purposes (entropy F2; b = 0.193, 95% CI [0.011, 0.374], t = 3.706, p < .05). There was also a significant effect related to the sheer L2 exposure for internal purposes (exposure F1; b = 0.819, 95% CI [0.448, 1.190], t = 4.32, p < .001). These effects suggest that higher entropy F2 or exposure F1 scores were associated with higher L2 proficiency scores. No other effects were significant. Figure 7 provides an illustration of all effects in the model.

Figure 7. Illustration of model-predicted effects for proficiency F1 factor scores (i.e., L2 proficiency) as a function of L2 age of acquisition (AoA) and language exposure factor scores. Bolded outlines illustrate significant effects (p < .05). Increases in sheer L2 exposure for internal purposes were associated with increased L2 proficiency factor scores. Increases in professional language entropy were associated increased L2 proficiency factor scores. Confidence bands illustrate 95% confidence intervals.
For models predicting subjective L1 proficiency (proficiency F2), the best model included additive effects of sheer L2 exposure scores (AICbest = 213.5), and all other models exhibited considerably less support (i.e., AICdiff > 4). See Table A.2 for the summary results of the four models. We inspected the best model to determine the direction of the effects. In this model, there was a significant effect related to the sheer L2 exposure scores for externally directed or professional purposes (exposure F2; b = –0.514, 95% CI [–0.746, –0.281], t = 4.33, p < .001). This effect suggests that higher exposure F2 scores are associated with lower subjective L1 proficiency. No other effects were significant. Figure 8 provides an illustration of all effects in the model.

Figure 8. Illustration of model-predicted effects for proficiency F2 factor scores (i.e., subjective L1 proficiency) as a function of L2 area of acquisition (AoA) and language exposure factor scores. Bolded outlines illustrate significant effects (p < .05). Increases in sheer L2 exposure in professional settings were associated with reduced subjective L1 proficiency factor scores. Confidence bands illustrate 95% confidence intervals.
For models predicting objective L1 proficiency (proficiency F3), the best model included additive effects of sheer L2 exposure (AICbest = 214.5). Of note, two other models also received “strong support” (entropy alone, AICdiff = 3.4; entropy and exposure, AICdiff = 3). See Table A.3 for the summary results of the four models. We inspected the best model to determine the direction of the effects. There were no significant effects in this model (or any of the other “supported” models). Figure 9 provides an illustration of all effects in the model.

Figure 9. Illustration of model-predicted effects for proficiency F3 scores (i.e., objective L1 proficiency) as a function of L2 area of acquisition (AoA) and language exposure factor scores. Bolded outlines illustrate significant effects (p < .05). No effects were significant. Confidence bands illustrate 95% confidence intervals.
Discussion
Our goal was to assess the structural properties of and relationships between continuous constructs that jointly index the bilingual language experience, including the timing of language exposure, language entropy, sheer L2 exposure, and language proficiency (measured through objective and subjective measures).
As a marker of the timing of language exposure, we measured L2 AoA continuously. L2 AoA is a crucially important determinant of several aspects of linguistic, cognitive, and brain function (Berken et al., Reference Berken, Gracco, Chen and Klein2015, Reference Berken, Chai, Chen, Gracco and Klein2016; Flege et al., Reference Flege, Munro and Mackay1995; Gullifer et al., Reference Gullifer, Chai, Whitford, Pivneva, Baum, Klein and Titone2018; Johnson & Newport, Reference Johnson and Newport1989; Klein et al., Reference Klein, Mok, Chen and Watkins2014; Kousaie et al., Reference Kousaie, Chai, Sander and Klein2017; Kousaie & Phillips, Reference Kousaie and Phillips2011). Of note, participants are often dichotomized into groups on the basis of L2 AoA (e.g., simultaneous bilinguals, L1 French bilinguals, and L1 English bilinguals). This approach was also taken here to provide a means of comparison. Of note, we also included measures of current exposure including sheer L2 exposure and language entropy. Successfully acquiring an L2 requires exposure to that L2, and measures of sheer L2 exposure are associated with several core language processing phenomena, such as the magnitude of lexical frequency effects during L2 and in L1 reading (Whitford & Titone, Reference Whitford and Titone2012).
Finally, we included measures of language entropy. Language entropy provides an estimate of balance or diversity in language exposure. While language entropy covaries with sheer L2 exposure (measures of L2 exposure figure into the computation of entropy), it taps into a different theoretical construct as it provides an index of balanced exposure accounting for multiple languages.
We assessed each of these constructs continuously through data collected on a health and language background questionnaire with the additional inclusion of verbal fluency data to assess objective language proficiency. For constructs that tap into current language experience and exposure, we further assessed the variation that exists between and within communicative contexts providing granularity to the assessment. Specifically, for sheer L2 exposure and language entropy, we considered language usage for various purposes, including for internal thought (e.g., thinking and dreaming) and mental computations (e.g., counting and mental arithmetic); with friends and family, for school and work, and for the consumption of media. We also assessed L2 proficiency through both objective (verbal fluency) and subjective (self-report) measures.
We conducted factor analyses on these measures to assess the latent structures of these constructs: sheer L2 exposure, language entropy, and language proficiency. We showed that latent factor scores for sheer L2 exposure and language proficiency could discriminate between groups of bilinguals who differ in their acquisition history (i.e., simultaneous French–English bilinguals, L1 French–L2 English bilinguals, and L1 English–L2 French bilinguals). Latent factor scores for language entropy factor scores were relatively less important in discriminating the groups. We then showed that latent factor scores for sheer L2 exposure and language entropy were important in predicting language proficiency (L2 proficiency and subjective L1 proficiency but not objective L1 proficiency). We now discuss the core findings in turn.
Measuring current language exposure: Latent structure of sheer L2 exposure and language entropy
The factor analysis on measures of sheer L2 exposure revealed evidence for two latent factors indexing sheer L2 exposure (a) for internal-driven purposes (e.g., arithmetic and numerical operations, thinking talking to oneself, dreaming, and expressing emotion), and (b) for externally directed or professional purposes (e.g., reading online, reading for work, writing papers, and writing e-mails). The factor analysis on language entropy measures revealed a similar factor structure with the inclusion of an additional factor indexing language entropy for the consumption of media. Thus, there appears to be a fundamental distinction between language usage patterns for internally driven versus externally driven purposes.
These findings are compatible with other work that suggests a similar dichotomy between internal and external usage patterns (e.g., Schrauf, Reference Schrauf2009; Vaid & Menon, Reference Vaid and Menon2000). For example, Schrauf showed that among older adult bilinguals who moved from Puerto Rico to the mainland United States, English (L2) usage patterns resulted in three factors: amount of English used for internally driven purposes (e.g., thinking, dreaming, counting, expressing emotion, swearing, and talking to oneself), among coworkers and family (workmates, significant other, offspring, in-laws, and family), and in social settings (friends and neighbors). The importance and structure of the internally driven measures are generally consistent across studies and suggests that most of the variance in the patterns of reported language usage can be explained by internal motivations of the participants.
Moreover, an individual’s personal usage of language may be quite distinct from how that individual reports using language in the environment for communication. The loading of measures related to the social usage of language (e.g., patterns of usage among coworkers, or family and friends) tends to shift across studies conducted in different environments. Thus, these patterns of social language usage are fundamentally related to the broader environment in which language is used (e.g., French and English in Montréal vs. English and Spanish in New York).
Our findings extend past work on the latent structure of language entropy for French–English bilinguals living in Montréal (Gullifer & Titone, Reference Gullifer and Titone2020a). Past work shows that language entropy is composed of two principal factors related to general language entropy (reading, speaking, home, and social entropy) and language entropy for professional settings (at work, with some cross-loading from social language entropy). The structural separation between work contexts and other contexts is generally supported in the present data set because measures indexing use in the work environment (together with other externally driven factors) loaded separately from language use for internal purposes, with family members, and with friends. The results also support the existence of another distinct component related to distributions of language use for the purposes of media consumption. However, this factor was generally weak, explaining only a small portion of variance and included only one major measure (usage for TV and radio). Future questionnaires should probe more specific types of media in order to more accurately measure this component (e.g., movies, online videos, podcasts, etc.).
Crucially, our detailed assessment of current bilingual language experience, including sheer L2 exposure and language entropy, represents an expansion on past work. When prior studies assess sheer L2 exposure, they tend to extract responses on a single questionnaire item (e.g., self-reported L2 exposure globally). However, the use of single, “global” items can be problematic, as participants may respond to these items with different contexts in mind, or they may be unable to accurately compute a mental average of language usage. Global items ultimately ignore potential variation within and across communicative contexts. Here we assessed sheer L2 exposure or use within several communicative contexts, including for internal thoughts and computations, with friends and family, for school and work, and for the consumption of media. This approach mirrors that taken for our assessment of language entropy in several communicative contexts. Of note, here we have expanded the number of communicative contexts for which we assessed language entropy relative to prior work. In the past, we assessed language entropy for the purposes of reading, speaking, talking with friends, talking with coworkers, and talking with family. That approach either leaves out or collapses across several crucial dimensions of bilingual language usage.
Although this study extends past work on language entropy, there are notable divergences that we point out here concerning the first latent variable. In past work, the first language entropy component comprised a variety of entropy measures, including for reading (generally), for speaking (generally), with family, and with friends. We thus referred to that latent component as “general language entropy.” Here, the first latent factor is more precisely related to distributions of language usage for internal purposes and mental computations, for which we had multiple measures. Other measures, that indexed, for example, reading in various contexts, are distributed across other factors (i.e., factors for externally driven or work purposes and media consumption). Of note, we also assessed reading in a more fine-grained manner than before (i.e., reading for fun, reading for work, or reading online), allowing for greater specificity of this domain. This shift in the factor loadings, particular when measures are assessed in a granular, context-dependent, manner, is important to consider for future studies. It also suggests that when language usage is probed “generally” or “globally” (e.g., overall percentage of time spent reading), participants may respond with a particular domain in mind, such as usage for internal purposes or with family members, as opposed to how they tend to use language in the everyday environment. Thus, for maximally sensitive measurement of usage and exposure, it is important to assess context-specific language usage.
We also found that the distributions of language usage with family and friends loaded onto this first factor, though the loadings were muted compared to other, more important, measures. This further suggests aspects of language use that are driven primarily by the participant’s intentions and that these intentions are crucially shaped interactions with family and friends, which likely occur early in on life.
When comparing the factor structures for sheer L2 exposure and language entropy, there is considerable overlap. This is, perhaps, not entirely surprising because measures of L2 exposure (together with exposure for each other language reported by the participant) figured into the computation of language entropy. The overlapping results here suggest that the domains of language usage (i.e., the communicative contexts) that drive language use are common for language entropy and L2 exposure, at least for this population of primarily university students living in Montréal. However, for reasons we return to later, it is important not to conflate these two factors, as they tap into different theoretical constructs and may thus relate to different factors of bilingual language experience such as language control processes.
The factor analyses, and the questionnaire on which they are based, have several limitations that could be addressed in future studies. Specifically, future questionnaires should more systematically probe crucial contexts of language usage with a similar number of items. For example, here, the factor indexing media usage was dominated by a single item (listening to TV/radio), likely contributing to the poor sampling accuracy of this measure related to language entropy and to inconsistent loadings across language entropy and sheer exposure constructs. Moreover, it may be beneficial to include a similar number of items related to language production and language comprehension crossed by the written and spoken modalities to provide a fuller picture of bilingual language usage. This would give the most accurate assessment of language exposure and usage across communicative contexts. Finally, future questionnaires should require that participants respond to all questions related to the communicative contexts and explicitly specify if they do not use a language in a specific context to reduce missing data.
Measuring language proficiency: Latent structure of objective and subjective proficiency measures
The factor analysis on language proficiency measures revealed evidence for three latent factors indexing (a) overall L2 proficiency (objective and subjective), (b) subjective L1 proficiency, and (c) objective L1 proficiency. This suggests that participants in our sample were generally able to judge their objective L2 performance through subjective measures, at least in a relative manner between participants. In other words, people who score high on the L2 verbal fluency task, tended to rate themselves more highly on the self-reported L2 measures (i.e., higher L2 abilities and lower L2 accent). The observation of convergent patterns between objective and subjective L2 proficiency measures stands in contrast to previous work indicating that bilinguals are not able to accurately judge their performance in the L2 (e.g., Tomoschuk et al., Reference Tomoschuk, Ferreira and Gollan2019). At the same time, subjective measures did not always correlate well with objective measures, reflected in the two latent factors indexing L1 objective proficiency and L1 subjective proficiency. This finding is somewhat surprising, because where individuals’ thoughts about language ability diverged from objective performance was in the native language, which is frequently more dominant than the L2. Ultimately, these findings illustrate a key point made by Tomoschuk et al. that self-ratings (and by extension, the extent to which they line up with objective measures) may depend on aspects of the population being sampled, as some populations may tend to rate themselves higher or lower in line with cultural expectations and norms. Regarding the present participants, all participants were sampled from the Montréal area. This location is somewhat unique because bilingualism and multilingualism are the norm and there is contact between many languages, including mainly French and English. Thus, we echo calls to incorporate subjective and objective measures of proficiency (e.g., Surrain & Luk, Reference Surrain and Luk2019; Tomoschuk et al., Reference Tomoschuk, Ferreira and Gollan2019) so that the validity of each can be assessed.
Do individual differences in bilingual language experience discriminate acquisition history and predict language proficiency?
We then assessed whether individual differences along these continuous dimensions can be used to predict membership of participants among the three language acquisition groups (simultaneous, L1 English, and L1 French), and that individual differences in language entropy and sheer L2 exposure can be used to predict language proficiency. We generally found that the professional L2 exposure and the objective L1 proficiency factors discriminated between the groups. Individuals with higher professional L2 exposure scores were more likely to have French as the L1 and less likely to have English as the L1 relative to simultaneous bilinguals. Individuals with higher objective L1 proficiency scores were less likely to have French as the L1 relative to simultaneous bilinguals. This finding is likely related to characteristics of the sample. In particular, most of the participants were students at McGill University, an English-speaking campus. Thus, participants who speak French as a native language in this environment will inherently have greater exposure to the L2 in this setting. In contrast to the results for sheer L2 exposure, language entropy was not as important in discriminating the language groups. This finding is consistent with previous work showing only small relationships between language entropy and L2 AoA (Gullifer et al., Reference Gullifer, Chai, Whitford, Pivneva, Baum, Klein and Titone2018; Gullifer & Titone, Reference Gullifer and Titone2020a).
In terms of predicting language proficiency as a function of continuous individual differences in bilingual language experience, we found that high L2 proficiency (a component representing subjective and objective proficiency) was associated with high sheer L2 exposure for internal purposes and high entropy associated with externally directed or professional purposes. Subjective L1 proficiency decreased as a function of sheer L2 exposure in professional settings, but objective L1 proficiency was generally unrelated to these individual differences. In other words, individual differences in bilingual language experience were associated with L2 outcomes (self-reported and objective measures), but they were only associated with self-reported measures in the L1. These results confirm the idea that language proficiency is not a unitary construct and that people become proficient bilinguals by way of distributing usage of their languages differentially in different communicative contexts to achieve various communicative goals.
Regarding the distribution of language use, due to the fact that sheer L2 exposure and language entropy shared similar latent factor structures, one might argue that the two constructs are tracking the same underlying construct and that it would be difficult to effectively disentangle the two. Moreover, given that the introduction of language entropy results in yet another construct to measure in bilingualism, researchers may be tempted to opt for easier measures like global L2 exposure. However, we reiterate that a reliance on global measures may hold unintended consequences. Moreover, we have shown here sheer L2 exposure and language entropy exhibit different contributions in L2 proficiency depending on the communicative context.
Differential contributions of language entropy and sheer L2 exposure are most evident in our work in the domain of executive control and brain organization (Gullifer et al., Reference Gullifer, Chai, Whitford, Pivneva, Baum, Klein and Titone2018; Gullifer & Titone, Reference Gullifer and Titone2020b). In that work, language entropy is the best predictor for measures of executive control relative to other individual differences measures (including exposure, and L2 AoA). The specific import of language entropy for control processes is predicted by theoretical perspectives like the adaptive control hypothesis (Abutalebi & Green, Reference Abutalebi and Green2016; Green & Abutalebi, Reference Green and Abutalebi2013; Green & Wei, Reference Green and Wei2014; Grosjean, Reference Grosjean1997, Reference Grosjean2001) and recent empirical evidence (Gullifer et al., Reference Gullifer, Chai, Whitford, Pivneva, Baum, Klein and Titone2018; Gullifer & Titone, Reference Gullifer and Titone2020a; Sulpizio et al., Reference Sulpizio, Del Maschio, Del Mauro, Fedeli and Abutalebi2019) whereby people adapt their control processes as a function of the language demands of the communicative or interactional context, and in particular the distributions of language usage that occurs in that context (e.g., in single language contexts vs. dual language contexts). Of note, predictions of these theories cannot be easily addressed through global L2 exposure with standard statistical practices that assume linear relationships between predictors and outcome variables because single language contexts and monolingual modes occur at both ends of the L2 exposure spectrum. Thus, language entropy should be considered in future work (perhaps jointly with other measures) if there is substantive reason to assess the relative distributions of language usage (e.g., balance of L1 – L2+ usage).
Bilingualism as a multidimensional spectrum of experiences
In this study, we offer another demonstration of how bilingual language experience can be quantified as a multidimensional spectrum of experiences. This perspective is increasingly becoming the norm in the field of bilingualism (e.g., Anderson, Hawrylewicz, et al., Reference Anderson, Hawrylewicz and Bialystok2018; Baum & Titone, Reference Baum and Titone2014; Dash et al., Reference Dash, Berroir, Joanette and Ansaldo2019; DeLuca et al., Reference DeLuca, Rothman, Bialystok and Pliatsikas2019, Reference DeLuca, Rothman, Bialystok and Pliatsikas2020; Gullifer et al., Reference Gullifer, Chai, Whitford, Pivneva, Baum, Klein and Titone2018; Gullifer & Titone, Reference Gullifer and Titone2020a; Li, Legault, et al., Reference Li, Legault and Litcofsky2014; Sulpizio et al., Reference Sulpizio, Del Maschio, Del Mauro, Fedeli and Abutalebi2019; Tiv, Gullifer, et al., Reference Tiv, Gullifer, Feng and Titone2020). Previously, it was common to collapse bilinguals and monolinguals into groups. However, a dichotomous approach assumes that all participants are homogenous in their language experience, and it washes away important variance that is now known to influence language processing, language control, cognitive control, and brain structure and function. Here we jointly account for multiple sources of variation that include, on the one hand, static historical aspects of language exposure and, on the other hand, current language experience.
Of importance, there are several dimensions of bilingual experience we did not measure or assess here that are, nonetheless, part of the bilingual experience. We did not assess the code-switching behavior of the participants or their experiences with translanguaging. Yet engagement in these flexible language practices is thought to be another important determinant of how the language and control systems of bilinguals become adapted through interactional contexts (e.g., Adler, Valdes Kroff, & Novick, Reference Adler, Valdes Kroff and Novick2020; Green & Wei, Reference Green and Wei2014). Moreover, while our measures of sheer L2 exposure and language entropy tap into the extent of current language exposure within an immersion context, they do not account for the duration of exposure. Duration of exposure has been shown to contribute to adaptive modulations in neuroanatomical brain structure and language processing (e.g., DeLuca et al., Reference DeLuca, Rothman, Bialystok and Pliatsikas2019; Dussias & Sagarra, Reference Dussias and Sagarra2007). A final key issue in this domain is validity, reliability, and generalizability of the measures and latent constructs that index the bilingual language experience, including L2 AoA, sheer language exposure, language entropy, and language proficiency. Future work should assess the joint contribution of all of these factors and their applicability to other populations. Many of these constructs are based on self-report, and there are concerns about whether participants are able to reliably assess their experiences. To ensure reliable and valid measures, detailed questionnaires (with multiple questions estimating each factor of interest) should be used to discern the constructs of interest. Sample sizes should be sufficiently large to employ statistical approaches that allow for the assessment of construct validity (such as factor analysis or structural equation modeling). Even objective measures should be validated to ensure that they are assessing the construct of interest (e.g., language proficiency) and not potentially erroneous constructs.
Conclusions
Bilingualism is a multidimensional spectrum of experiences composed of several continuous constructs associated with language usage and exposure across multiple languages. To build a complete picture of bilingualism, it is crucial to account for as many of these factors to the greatest extent possible. A wealth of literature suggests that the timing of language exposure and the amount of language exposure are crucial constructs to consider when studying bilingual and multilingual populations. However, these constructs do not fully capture the full range of bilingual experience; in particular, they do not capture the diversity associated with language use in the social world or for internalized purposes.
A key challenge in obtaining a full picture of the bilingual experience is the requirement to engage with a high dimensional space. We proffer language entropy as a means to estimate a core construct related to social language usage and as a means to help reduce complexity of the space. Language entropy is a highly flexible measure of language balance: it can be applied to situations where more than two languages are in use, and it can be applied to many types of data that provide information about language usage. While we estimate language entropy from self-reported data, similar (and perhaps more precise) estimates could also be derived from naturalistic production. Moreover, we show how data reduction techniques such as factor analyses or principal component analysis can also be used to further reduce the dimensionality of a problem and to identify the latent structure for constructs of interest. A precise quantification of bilingualism in all of its complexity will better enable researchers to tackle broader problems and questions in bilingualism, language processing, language acquisition, and language control.
ACKNOWLEDGMENTS
We acknowledge funding from the following sources: Fonds de recherche du Québec– Société et culture; Fonds de Recherche du Québec–Nature et technologies; Social Sciences and Humanities Research Council of Canada; Natural Sciences and Engineering Research Council; and the Blema & Arnold Steinberg Family Foundation.
APPENDIX A
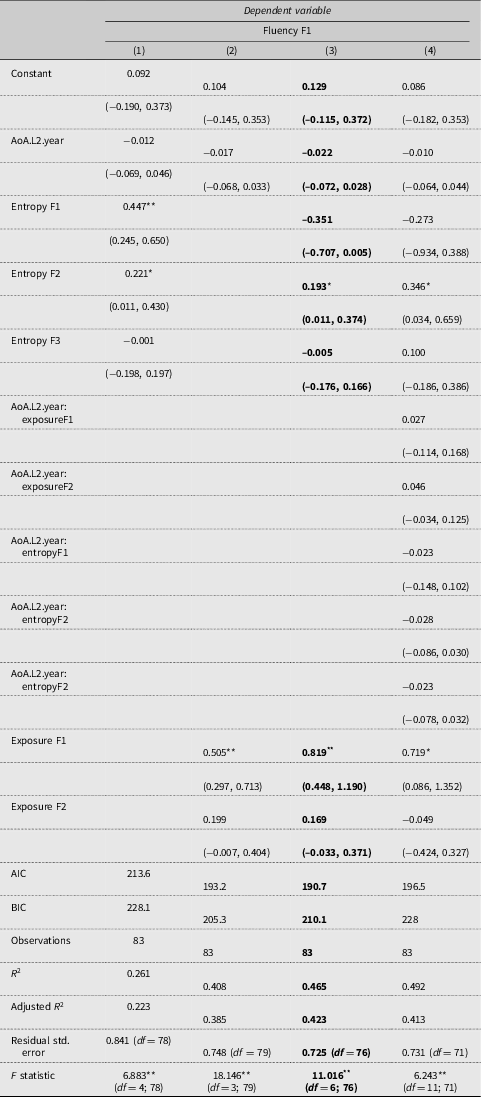
*p < .05. **p < .001.
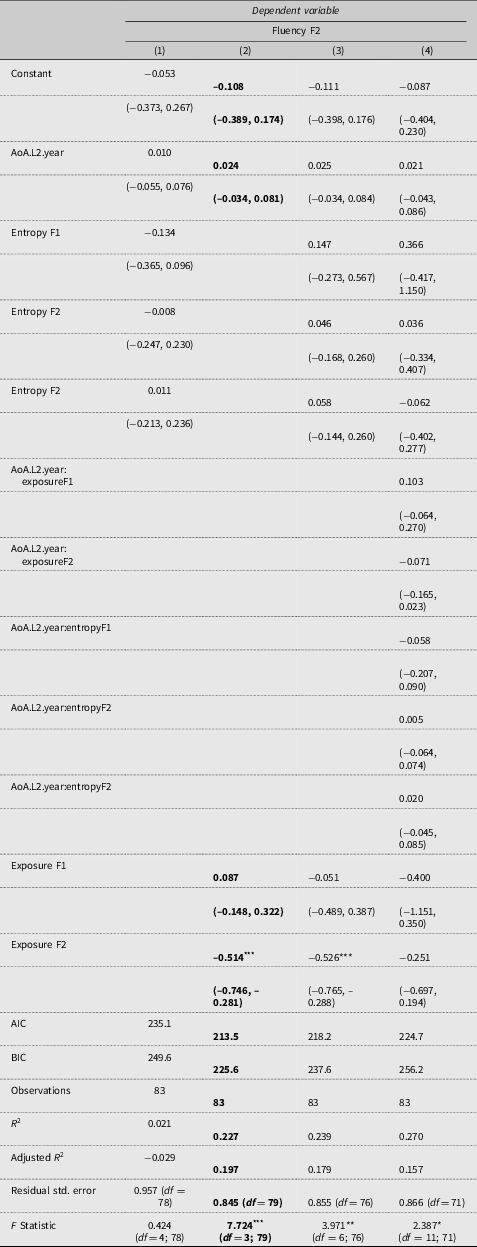
*p < .05. **p < .01. ***p < .001.
