I. INTRODUCTION: WHAT IS AI/ML SAMD?
Artificial intelligence (“AI”) has the power to revolutionize the health care industry. It can detect diseases earlier, give more accurate diagnoses, and significantly improve personalized medicine.Footnote 1 In 2018, for example, the U.S. Food & Drug Administration (“FDA”) authorized a diabetic retinopathy detecting device.Footnote 2 The software, IDx-DR, uses an AI algorithm to examine eye images for early signs of this progressive condition.Footnote 3 The FDA, under the De Novo premarket review pathway, prioritized review of the software as a breakthrough device, meaning that despite its low-to-moderate risk, the FDA provided intensive guidance to facilitate rapid development.Footnote 4 Also in 2018, the FDA permitted marketing for another device brought through De Novo premarket review: the Viz.AI Contact application.Footnote 5 This revolutionary software delivers clinical decision support based on computed tomography (“CT”) results to notify providers of potential strokes.Footnote 6 Similar products may now go through the 510(k) process, a simpler premarket review process, by demonstrating substantial equivalence to the initial device.Footnote 7 Both of these devices use AI technology to make faster and more accurate diagnoses.Footnote 8 Unlike some other forms of AI, these software programs have locked algorithms that do not change with use.Footnote 9 The manufacturer must manually verify and validate any updates to the software and submit a new 510(k) for significant updates.Footnote 10
The FDA has proposed a regulatory framework to review AI software that is not locked but continuously learning.Footnote 11 Continuously learning software responds to real-world data and can make modifications without manufacturer intervention.Footnote 12 This Article discusses FDA’s proposed regulatory framework for AI medical devices. It evaluates the proposed regulatory framework against the backdrop of current technology, as well as industry professionals’ desired trajectory, to determine whether the proposed regulatory framework can ensure safe and reliable medical devices without stifling innovation.
The proposed regulatory framework places effective limits on continuously learning algorithms.Footnote 13 These limits give manufacturers freedom to allow their devices to adapt to real-world data without fundamentally changing the nature of the device post-market. The proposed framework, however, does not give adequate attention to protecting patient data, monitoring cybersecurity, or ensuring safety and efficacy. The FDA, or other relevant agencies, should increase regulatory oversight of these areas to protect patients and providers relying on this new technology.
A. Key Definitions
AI technology uses statistical analysis and if-then statements to learn from real-world data and improve its performance.Footnote 14 Machine learning (“ML”) is one type of technique through which AI learns.Footnote 15 The Food, Drug & Cosmetic Act (“FD&C Act”) classifies AI/ML-based software that is intended to “treat, diagnose, cure, mitigate, or prevent disease or other conditions” as a medical device.Footnote 16
Adaptive AI/ML technology uses either “locked” algorithms or “continuously learning” algorithms.Footnote 17 In software, algorithms are the processes, or rules, a program follows to solve problems.Footnote 18 Locked algorithms yield the same results every time the same input is applied.Footnote 19 As the name suggests, continuously learning algorithms adapt over time. These algorithms acquire knowledge from real-world experiences and can change even after the manufacturer distributes the software for use.Footnote 20 Continuously learning algorithms can yield different outputs given the same set of inputs because the algorithm changes with real-world data.Footnote 21 This technology is especially beneficial for optimizing performance based on the way users implement the device.Footnote 22
The FDA adopted the term “Software as a Medical Device” (“SaMD”) from the International Medical Device Regulators Forum (“IMDRF”) for any software used for a medical purpose that does not rely on a hardware medical device.Footnote 23 The other two types of medical device software include: (1) Software in a Medical Device, which is “software that is integral to a medical device,” and (2) “software used in the manufacture or maintenance of a medical device.”Footnote 24 SaMD stands out from these other two forms of medical device software because SaMD may interface with hardware medical devices but cannot “drive” the device or be necessary for the device to achieve its intended medical purpose.Footnote 25 For example, the FDA would classify a mobile medical application that helps a dermatologist diagnose skin lesions as SaMD while classifying software imbedded in a cochlear implant that can calibrate or change the implant’s settings as software in a medical device.Footnote 26 In determining the classification of medical device software, it can be helpful to consider (1) to what extent the software controls the operation or function of a medical device and (2) whether the software could run on a non-medical computing platform, such as a mobile device or general-purpose computing platform.Footnote 27 AI/ML SaMD is a subset of SaMD that relies on an AI/ML algorithm, either locked or continuously learning, to function.Footnote 28
B. Four Categories of AI/ML SaMD
Currently, the four main types of AI/ML SaMD cover (1) the management of chronic diseases, (2) medical imaging, (3) the Internet of Things (“IoT”), and (4) surgical robots.Footnote 29
1. Managing Chronic Diseases
AI/ML technology can be especially beneficial for people with chronic illnesses because of its ability to give personalized care recommendations and monitor patients in real-time. For example, AI/ML SaMD offers a potentially life-changing solution for many people living with diabetes, a chronic illness afflicting four hundred and twenty-five million people worldwideFootnote 30 and accounting for 12% of the world’s healthcare costs.Footnote 31 AI products can transform diabetes management through automated retinal screening, clinical decision-making support, predictive population risk, and patient self-management tools.Footnote 32 Medtronic’s Sugar.IQ diabetes assistant, for example, can predict hypoglycemic events and help patients make strategic treatment decisions.Footnote 33 Sugar.IQ works with Medtronic’s Guardian Connect, a smartphone-connected continuous glucose monitoring system.Footnote 34 Medtronic reported major success with the Sugar.IQ product, boasting that patients stayed in the optimal glycemic range for an hour more each day than when just using Guardian Connect.Footnote 35
Despite the company’s success, Medtronic’s products have already illustrated the potential dangers of using SaMD that rely on the internet.Footnote 36 Medical devices that interact with smartphones and the internet carry potential cybersecurity risks.Footnote 37 Medtronic recently recalled their MiniMed insulin pumps after discovering they were vulnerable to hacking; an unauthorized person could connect to the pump and operate it remotely.Footnote 38 These MiniMed pumps use a closed-loop automated insulin delivery system, often called an “artificial pancreas,” that was originally cleared by the FDA in 2016.Footnote 39 The FDA and manufacturers will need to work together to address these cybersecurity vulnerabilities as AI software will increasingly depend on internet-connected devices.
2. Medical Imaging
AI SaMD in medical imaging speeds up image processing and interpretation, improving accuracy and consolidating data for reporting, follow-up planning, and data mining.Footnote 40 Because of its ability to examine wide ranges of images, AI algorithms have been found to identify patterns and spot abnormal findings with greater speed and accuracy than a radiologist in several studies.Footnote 41 Additionally, AI technology has been shown to improve the quality of images and reduce the amount of time a patient needs to be in a MRI scanner, lowering the overall radiation dose and optimizing staffing and MRI scanner use.Footnote 42
In September 2019, the FDA cleared GE Healthcare’s Critical Care Suite, a collection of AI algorithms on a mobile X-ray device, via the 510(k) pathway.Footnote 43 The approved algorithms improve the diagnosing time of a suspected pneumothorax, a type of collapsed lung.Footnote 44 It often takes up to eight hours for a radiologist to review an x-ray image due to significant backlog time, but the Critical Care Suite’s algorithm can detect pneumothorax and alert both the nurse and the radiologist to prioritize the case for review.Footnote 45 When detected early, clinicians simply insert a tube to release the trapped air.Footnote 46 If detected too late, the patient will have difficulty breathing and possibly die.Footnote 47 AI technology in medical imaging can save lives by improving the accuracy and speed of diagnosis. Diagnosing SaMDs need to give accurate and reliable output, however, or false positives will further backlog a physician’s time, and false negatives will delay needed care. The efficacy of AI SaMD should be a key concern for regulators and manufacturers.
3. Internet of Things (“IoT”)
IoT comprises the cohort of connected devices, namely SaMD, that communicate with one another, like mobile health apps and wearable technology.Footnote 48 IoT products represent “a segment of the medical device market valued at over $40 billion and expected to rise to over $155 billion by 2022.”Footnote 49 Wearable technology like FitBits and smart watches track health data and are capable of complex analytics to diagnose health problems at early stages and monitor chronic conditions.Footnote 50 The Apple Watch electrocardiogram (“ECG”) app recently received a Class II De Novo designation, allowing users to monitor their heart rate and heart rhythm to detect atrial fibrillation (“AFib”).Footnote 51 While a clinical study showed the ECG app correctly diagnosed AFib with 98.3% sensitivity and 99.6% specificity, Apple must still advertise their app as intended for informational use only, rather than diagnostic use, because of its over-the-counter nature.Footnote 52
Even these lower-risk devices, however, may raise data privacy, efficacy, and safety concerns because they collect health data and may be used to make decisions affecting user health.Footnote 53 IoT has the potential to connect clinics, hospitals, homes, offices, and transportation to create seamless transitions of interconnectivity and thus transform patient care, but it needs proper regulatory guidance to ensure safety and efficacy.Footnote 54
4. Surgical Robots
Potentially, the most innovative and concerning use of AI in health care is that of surgical robots. Although surgeons have used machines like the da Vinci Surgical Robot for many years,Footnote 55 researchers are developing AI technology to automate suturing and improve surgical robots’ skills affecting completion time, path length, depth perception, speed, smoothness, curvature, and workflow.Footnote 56 ML is especially helpful in surgery because it can automate routine tasks by extracting data, predicting problems, and making decisions without human intervention.Footnote 57 With enough data, ML robots could use complex algorithms to spot problem areas and make strategic decisions much faster and with more accuracy than any surgeon.Footnote 58 Surgical robots introduce major FDA-approval safety and efficacy concerns based on the high-risk nature of surgery. Proper training and implementation of this SaMD technology will be key to ensuring continued safety and efficacy post-market. The industry and policymakers should develop consistent processes and regulations that monitor these robots from design to implementation.
C. FDA Response
AI/ML technology in health care varies significantly between the levels of risk it imposes on the patient and the potential liability it opens for the hospital and provider. Because AI/ML SaMD modifies its own algorithms and can potentially change the nature and intended use of the device in question,Footnote 59 the FDA must design a new regulatory framework that can adapt to continuously learning software. The FDA requested feedback from industry professionals on a discussion paper (“AI/ML discussion paper”) containing a proposed regulatory framework for modifications to AI/ML SaMD in April 2019 and opened public comments until June 2019.Footnote 60 In the AI/ML discussion paper, the FDA proposed a Total Product Lifecycle (“TPLC”) Approach to regulating AI/ML SaMD and put forth Good Machine Learning Practices (“GMLPs”).Footnote 61 In January 2021, the FDA released a five-part action plan responding to the AI/ML discussion paper’s comments and outlining issues to address in future iterations of the proposed framework.Footnote 62
II. BACKGROUND: SaMD REGULATION
Software can be difficult to regulate. Software reacts inconsistently on different hardware, users control installation of updates, and users may duplicate and distribute the software themselves, potentially imputing liability beyond the manufacturer.Footnote 63 The FDA will regulate software as a medical device when its intended use is for a medical purpose like diagnosing, preventing, monitoring, or treating.Footnote 64 The FDA views SaMD within a controlled lifecycle approach and provides guidance from design and development to post-market surveillance.Footnote 65 Within this lifecycle approach, “manufacturers of SaMD are expected to have an appropriate level of control to manage changes,” and manufacturers should perform risk assessments for each change to determine whether it affects core functionality and risk categorization before releasing the change.Footnote 66
The traditional SaMD regulatory framework assumes the manufacturer has significant control over the software’s individual functions. Manufacturers must incorporate the ecosystem in which the SaMD resides into any risk assessment and consider the connections to other systems, the information presented to users, hardware platforms, operating platforms, and changes to integration.Footnote 67 Ultimately, SaMDs are categorized by risk stemming from the state of the health care situation (critical, serious, non-serious) to the significance of the health care decision (treat/diagnose, drive clinical management, inform clinical management).Footnote 68 The current regulatory framework does not assume that software modification will change the categorization, but it still requires the risk assessment to confirm that the change does not place any undue risk on the user.Footnote 69
D. How is AI/ML Different?
The FDA could initially approve a device, but what happens if the device changes post-market through machine learning, expands its scope, or does not work as intended?
Up until the AI/ML discussion paper’s release (April 2019), the FDA had only cleared or approved a few AI/ML SaMDs, all of which had locked algorithms prior to marketing and distribution.Footnote 70 Locked algorithm SaMDs are most like non-AI SaMDs and can follow the same traditional regulatory framework with few modifications.Footnote 71 Current medical device or SaMD regulations, however, are not well suited for continuously learning technology. AI/ML technology requires a vast amount of real-world data to learn (“training”) and improve performance (“adaptation”).Footnote 72 ML relies on predictable environments to generate patterns,Footnote 73 but human bodies are not always predictable. If an algorithm is not locked, but continuously learning, manufacturers will not always be able to predict how a software is going to react in real-time based on new data. Unlike regular SaMD, a manufacturer might not be able to stop and approve every AI/ML algorithm adaptation before its use on patients. The ability to adapt in real-time makes continuously learning technology valuable, but this feature is difficult to regulate because of the concerns rising from a software that can change on its own without additional oversight.
A new regulatory approach for this adaptive technology needs to be agile enough to keep up with rapid product adaptations while maintaining safety and efficacy. Continuously learning technology is “highly iterative, autonomous, and adaptive” requiring a new, TPLC approach and new GMLPs.Footnote 74 AI/ML SaMD poses significant challenges in establishing a regulatory framework given the large variation of risk between medical devices. Diagnosing based on AI interpretations of x-ray images is very different from AI use in surgical robots in terms of the risk imposed on the patient. Any SaMD regulatory framework will need to continue to factor levels of risk into the level of scrutiny given each device. Additionally, the new regulatory framework will need to address privacy concerns regarding the vast amount of patient data the algorithms need to function well.
III. RELEVANT RULES: THE FDA’S 2019 PROPOSED REGULATORY FRAMEWORK
Current SaMD policy requires manufacturers to submit a marketing application to the FDA prior to distribution.Footnote 75 Based on the categorized risk of the device, the FDA then generally requires a 510(k) notification (for lower risk or substantially equivalent devices), De Novo request (to re-classify new devices automatically labeled as Class III or high risk, as Class I or II or lower risk),Footnote 76 or premarket approval application (requires proof of efficacy for high risk devices).Footnote 77 Manufacturers must then submit modifications for premarket review to FDA, and if the modification affects risk, risk controls, functionality, or performance, they must implement updates to the software.Footnote 78 Whether locked or continuously learning, modifications to AI/ML SaMD are usually categorized as performance modifications, input modifications, or intended use modifications.Footnote 79 Modifying intended use may change a device’s risk categorization.Footnote 80 For locked algorithms, 510(k) guidance requires a premarket submission for modifications that introduce a new risk, change risk controls, or significantly affect clinical functionality.Footnote 81 However, since continuously learning modifications can be implemented and validated through “well-defined and possibly fully automated processes” based on new data, it would be difficult, and potentially impossible, for a manufacturer to sufficiently stop and submit a premarket review for any changes.Footnote 82 New regulations need to address this difficulty.
E. Total Product Lifecycle Approach
FDA built on the TPLC approach initially developed for the Software Pre-Certification Program when designing its proposed regulatory format.Footnote 83 The FDA designed the TPLC framework to require that “ongoing algorithm changes follow pre-specified performance objectives[,] … change control plans,” and keep up with a validation process to ensure improvements to performance, maintain safety and efficacy of the software, and monitor in real-time once the device is on the market.Footnote 84 The TPLC approach would allow the FDA to shift the focus from just approving final, finished software products and instead take a more holistic approach in evaluating the manufacturer itself in charge of development, testing, and performance to speed up the approval process.Footnote 85
1. Manufacturer Review
In the AI/ML discussion paper, the FDA advocates for a “culture of quality and organizational excellence” in the manufacturers making and developing SaMD.Footnote 86 Following the Pre-Certification TPLC approach, the FDA would conduct reviews of manufacturers for reasonable assurance that they are producing high quality software, testing, and performance.Footnote 87 The Pre-Certification TPLC approach recommends a streamlined premarket review for manufacturers who demonstrate “excellence in developing, testing, maintaining, and improving software products.”Footnote 88 This streamlined review, however, is notably absent in the AI/ML discussion paper’s TPLC approach, and instead, GMLPs would govern the standards for organizational excellence without offering a streamlined path to review.Footnote 89 GMLPs cover data management, feature extraction, training, and evaluation.Footnote 90 Demonstrating GMLPs will likely be the first step to approval.
2. Premarket Safety and Efficacy Review
Under the FDA’s AI/ML discussion paper, manufacturers would have the option to demonstrate the safety and efficacy of their products by submitting a “predetermined change control plan” (“Change Control Plan”) while in the initial premarket review to signal to the FDA that it has thought about its capacity to manage and control future risk related to software changes.Footnote 91 The Change Control Plan will include both the types of predicted modifications (“SaMD Pre-Specification[s]”) and the strategy used to implement those modifications (“Algorithm Change Protocol”).Footnote 92
a. SaMD Pre-Specifications and Algorithm Change Protocol
To provide SaMD Pre-Specifications, the manufacturer must envision the direction in which the device will go as it learns and develops. The predicted modifications should be related to the performance, inputs, and intended use of the AI/ML SaMD.Footnote 93 The Algorithm Change Protocol serves as the strategy the manufacturer will use to manage and control modifications. The goal of the Algorithm Change Protocol is for the manufacturer to constrain future risks to users. Components of a proper protocol would include data management, re-training, performance evaluation, and update procedures.Footnote 94 In theory, the SaMD Pre-Specifications and Change Control Plan place limits on the algorithm’s ability to learn and adapt automatically, so the device remains safe and effective after the FDA approves it.
The FDA’s AI/ML discussion paper provides examples of boundaries set by the Change Control Plan.Footnote 95 Changes that merely improve on performance and input are reasonable, but changes that alter the intended use from low risk to medium risk, as would be the case where the device shifted from identifying disease to driving clinical management, must be anticipated, documented, and prepared for in the Algorithm Change Protocol.Footnote 96 Currently, if a manufacturer modifies its SaMD, it usually must submit a new 510(k).Footnote 97 Under the AI/ML discussion paper’s proposed framework, AI/ML SaMD with an approved SaMD Pre-Specification and Algorithm Change Protocol can make the changes specified in the plan without having to submit a new 510(k).Footnote 98
3. Real-World Monitoring
The most important aspect of the TPLC approach concerns real-world performance monitoring. Because continuously learning algorithms can adapt while in use, manufacturers must monitor changes and quickly react to safety concerns. The FDA will require a manufacturer to commit to “transparency” by submitting periodic reports with performance metrics and updates on any SaMD Pre-Specification and Algorithm Change Protocol modifications that it implements.Footnote 99 The FDA suggests that reporting and monitoring can be tailored for different devices based on risk, types of modifications, and “maturity of the algorithm.”Footnote 100
See Appendix A for a visual of the FDA’s TPLC approach for AI/ML SaMD. After initially requiring a “culture of quality and organizational excellence,” the FDA would allow the manufacturer significant latitude in envisioning which direction the device will go.Footnote 101 Through the Pre-Specifications and Algorithm Change Protocol, the manufacturer would assume responsibility of preparing for the device’s eventual changes. The FDA would then keep an eye on these algorithms in real-world monitoring by requiring manufacturers to remain vigilant and file frequent reports.Footnote 102
IV. APPLYING THE LEGAL RULES: DOES THE PROPOSED FRAMEWORK WORK?
An algorithm in the operating room would be revolutionary. AI/ML SaMD could help spot blood vessels or tumors, support surgical decision-making, deliver patient information in real time, increase accuracy, and improve patient outcomes.Footnote 103 Even if AI/ML assisted surgery becomes feasible, the surgeon would likely still remain in full control, while making better and faster decisions using the “augmented reality” of AI/ML technology.Footnote 104 However, surgery is messy and complicated. We are still a long way from designing an AI/ML algorithm that could interpret soft tissue in real-time through a camera.Footnote 105 Even though we may be a long way off from AI/ML-assisted surgery, regulatory systems must be able to support our aspirations.Footnote 106
Before manufacturers can venture into “fully robotic surgery,” the FDA needs to master the AI/ML concerns of lower-risk technologies. By focusing on technologies like diagnosis and pathology we can better understand how AI/ML software works in patient care. As of Fall 2021, the FDA has cleared seventy-nine algorithms through 510(k) premarket reviews and De Novo pathways.Footnote 107 Radiology and cardiology make up the majority of those cleared algorithms, and they do everything from detecting atrial fibrillations to diagnosing lung cancer.Footnote 108 In reviewing the authorizations, manufacturers do not warn users that patient data can fundamentally change the potential outputs, but rather manufacturers emphasize that patient data can inform potential outputs and develop personalized care.Footnote 109 Although manufacturers of approved algorithms do not publicly state whether their algorithms are locked or continuously learning, it seems that the FDA has still only approved locked algorithms.Footnote 110
Troubleshooting diagnosis and pathology AI/ML gives the FDA time to figure out the correct approaches to regulating other AI/ML SaMD. Diagnosis and pathology AI/ML has the potential to change how we screen diseases so patients can focus on prevention and staying healthy rather than on recovering from manifested disease.Footnote 111 Industry professionals hypothesize that cost-effective, minimally invasive devices could factor in biometrics, environmental factors, and behavioral factors to predict life-threatening conditions through AI algorithms.Footnote 112 Health care would become integrated into home settings through digital assistants and smartphones to manage symptoms, educate patients, and monitor medication use.Footnote 113
A. Breast Cancer Screening: Trial, Error, and Possibility
Breast cancer screenings and diagnosis provide a relevant case study into the challenges and possibilities of regulating AI/ML radiology SaMD.
1. CAD: A Very Expensive Failure
Over six hundred thousand individuals worldwide died from breast cancer in 2020.Footnote 114 To address this widespread issue, computer-aided detection (“CAD”) promised to increase cancer detection rates by 20%.Footnote 115 CAD works as a “second reader” of mammograms, identifying abnormalities in breast tissue and flagging areas of concern.Footnote 116 Manufacturers vigorously lobbied Congress for the FDA to approve CAD mammograms and subsequently for Medicare to pay for them.Footnote 117 The FDA approved CAD in 1998 based on very limited studies, which is common for most medical device approvals.Footnote 118 CAD obtained Medicare reimbursement in 2002, and by 2010, 74% of mammograms were interpreted by CAD.Footnote 119 As of 2015, CAD mammograms added up to $400 million dollars per year in health care spending, accounting for $1 of every $10,000 spent on health care.Footnote 120
Fairly soon after CAD was widely implemented in hospitals across the country, it was obvious that CAD was not as successful as intended. A 2015 study found that CAD was not associated with improved “sensitivity, specificity, positive predictive value, cancer detection rates, or other proximal screening outcomes.”Footnote 121 Sensitivity was actually worse with CAD, according to radiologists who interpreted mammograms both with and without the software.Footnote 122 Developers of the software did not factor in the way radiologists would respond to the product.Footnote 123 Because no large population studies or randomized trials evaluated the software, developers failed to see that doctors might change their behavior when using it.Footnote 124 Due to the excess of false positives, radiologists either overreacted to the CAD output, greatly increasing the services provided to patients, or they eventually ignored the constant flagging.Footnote 125 CAD’s failure illustrates the problem of wide-scale product implementation before a showing of effectiveness or knowing how the technology will be used in practice.Footnote 126 Despite its clinical failure, CAD is still widely used today because most insurance companies continue to cover its cost.Footnote 127 In 2019, iCAD (a medical device company) advertised that its CAD devices offer “improve[d] cancer sensitivity” through artificial intelligence, image processing, pattern recognition, and statistical/mathematical formulas, citing a 2008 study reviewing 231,221 mammograms.Footnote 128
While we are arguably still in an “era of choosing wisely” and being “cautious before implementing and paying for medical technology,”Footnote 129 we are also in an era of profound innovation. Should the FDA’s role be to make sure authorized devices are legitimately efficacious to justify insurance coverage, or is the agency’s primary concern the safety of the devices? One of CAD’s biggest problems proved to be the lack of premarket, large-scale testing to evaluate how effectively the devices operate in real life. Still, as diagnostic tools, the devices are relatively safe and low risk, despite being ineffective.
Unlike with pharmaceuticals, where the Centers for Medicare and Medicaid (“CMS”) is required to cover any FDA-approved drug, medical device companies must apply to insurance companies and CMS, to cover their newly approved devices.Footnote 130 This bifurcated system presumably creates a barrier in which medical devices must still prove their efficaciousness to justify insurance coverage even when a device is safe.Footnote 131 Even with the bifurcated system in place, however, coverage is not always centered around efficaciousness. For example, CMS originally covered CAD primarily because members of Congress lobbied heavily for both its approval and reimbursement.Footnote 132 New streamlined coverage opportunities continue to break down the bifurcated system. For example, the recent Medicare Coverage of Innovative Technology in which CMS will cover new breakthrough devices “as early as the same day as” FDA market authorization.Footnote 133 This new path to coverage could be game—Footnote 134 but it could also welcome an influx of expensive devices like CAD that are technically safe but not efficacious. If policymakers continue this trend toward streamlining coverage, the FDA’s role in determining insurance coverage for medical devices will only increase.
2. QuantX: Still Prioritizing Innovation
The FDA has erred on the side of innovation when it comes to radiology devices. In 2018, the agency proposed an order to reclassify certain radiology devices from Class III (highest risk, requiring premarket approval) to Class II (medium risk, requiring a 510(k) premarket notification).Footnote 135 This order will make it easier for a manufacturer of these products to get approval because, under most circumstances, it will not have to conduct safety and efficacy trials to gain clearance.Footnote 136 The FDA acknowledges that these types of radiology devices, like medical image analyzers (e.g., CAD mammograms) carry risks, including false positives (potentially increasing the number of additional services, like biopsies), false negatives (delaying treatment), device misuse on unintended populations or hardware (lower performance), misuse of protocol (lower sensitivity), and device failure (incorrect assessment).Footnote 137 The FDA proposes “special controls” to mitigate these risks, including reader studies, detailed labeling requirements, design verification, and limiting use to providers.Footnote 138
Recently, the FDA approved a De Novo application for a new CAD software called QuantX, granting reclassification from Class III to Class II.Footnote 139 QuantX, now called Qlarity Imaging, holds itself out as the “first FDA-cleared computer-aided diagnosis AI” software for radiology.Footnote 140 Qlarity software uses an AI algorithm.Footnote 141 The algorithm pulls from a database of reference mammogram images to generate a “QI score” indicating breast abnormalities.Footnote 142 The De Novo clearance proscribes general and special controls to manage potential risks,Footnote 143 but these controls are largely grounded in concerns of false positives, false negatives, incompatible hardware, or device failure. The clearance raises some cybersecurity concerns but maintains that the software follows the FDA’s cybersecurity guidance, so risks are minimal.Footnote 144 Qlarity claims their software results in a 39% reduction in missed breast cancers and a 20% overall diagnostic improvement.Footnote 145 Even with De Novo clearance, however, are we likely to see another expensive CAD disaster?
For the first FDA-cleared AI radiology device, the FDA evaluated the Qlarity software with regular SaMD restrictions and did not require any specific restrictions on the algorithm itself.Footnote 146 The De Novo clearance did specify, however, that for premarket notification submissions for future similar devices cleared through the 510(k) pathway, the special controls include “a detailed description of the device inputs and outputs.”Footnote 147 The FDA assumes that the inputs and outputs are stable and can be clearly identified and predictedFootnote 148—like in a locked algorithm. Qlarity Imaging has already stated that it plans to expand the diagnostic scope of its AI software to even more medical conditions.Footnote 149 The FDA might require more rigorous standards with future iterations of this product considering the proposed regulatory framework for AI/ML SaMD, or it might mimic the same approach as the original QuantX.
3. Regina Barzilay: Forays into Continuously Learning Technology
The traditional approach to regulating SaMD worked with Qlarity’s locked algorithm. But new advances are on the horizon for which the FDA will need to be prepared. Regina Barzilay, a computer science professor at the Massachusetts Institute of Technology, has developed a new AI/ML tool for detecting breast cancer up to five years before a physician would typically detect any abnormalities.Footnote 150 Barzilay wanted to create a program that would consolidate the experiences of as many women as possible to make diagnosis and treatment more efficient.Footnote 151 She first realized that hospitals have large amounts of inaccessible data when she herself was a breast cancer patient.Footnote 152 Despite many other patients in the hospital in a similar position, the doctors could not tell her how those other patients responded to certain treatments and surgeries.Footnote 153 She discovered that, despite the sheer amount of hospital data recorded, most of the data was written in “free-text” and thus not accessible for a computer to process.Footnote 154
Working from three decades of pathology reports from more than 100,000 patients, Barzilay has developed a machine that can analyze a mammogram more closely than any human thus far.Footnote 155 The software can detect very subtle changes in tissue that humans cannot see, including changes “influenced by genetics, hormones, lactation, [and] weight changes.”Footnote 156 The developers have conducted studies showing that patients labeled high risk were 3.8 times more likely than those not labeled high risk to develop breast cancer within five years.Footnote 157 This software program could solve problems of over/under testing by personalizing the frequency of screenings and biopsies by risk factors rather than by age.Footnote 158
The TPLC approach of the FDA’s proposed AI/ML framework could be beneficial in regulating Barzilay’s software. Under this framework, Barzilay would be required to follow GMLPs and only use relevant data, acquired in consistent and generalized ways, and maintain algorithm transparency.Footnote 159 Moreover, Barzilay could submit a predetermined change control plan to show the FDA the direction in which the software is likely to go and how she will facilitate any necessary changes.Footnote 160 A program like this one has the potential to change its intended use. With enough data the software could become helpful in diagnosing breast cancer, monitoring breast cancer, or possibly screening for additional types of cancer. Barzilay would then need to submit a new 510(k) to make changes of that nature.Footnote 161 The real-world monitoring would be the most significant aspect of the regulatory process. The FDA would require Barzilay to submit periodic reports of any changes or safety concerns; but the reporting would probably be flexible because of the lower risk (Class II) nature of the device.Footnote 162 Even with lower risk devices, however, risks can rise when computers begin doing things that humans cannot do.Footnote 163 The FDA proposed regulations might not be enough to control for these increased risks—especially if the risks are difficult to promptly detect, such as an algorithm bias.Footnote 164
While Barzilay’s software could change how doctors screen for breast cancer by constantly incorporating experiences of new women with a continuously learning algorithm, it raises some key questions. When an algorithm makes a mistake, who is liable? Will patients trust computers to do what doctors used to do? How do we monitor physician use of the program to avoid problems like those experienced with the original CAD software? How do we access the great amount of data tucked away in hospitals to generate tools that consider a wide range of experiences? Are there ethical issues in using patient data? Could insurance companies and employers misuse cancer data predictions? Are there cybersecurity concerns? Should there be limits on how deeply the software can learn?
The FDA needs to address these privacy, cybersecurity, and safety and efficacy issues resulting from AI/ML software. The FDA has released some guidance on cybersecurity, but this guidance needs to be incorporated into the actual regulations. It should not be left up to manufacturers to self-regulate.Footnote 165 To date, the FDA has not released guidance regarding the protection of patient data in SaMD.Footnote 166
Tracking the evolution of breast cancer screening technology illustrates the range of FDA responses to new SaMD innovations. Initial approval of CAD software added additional financial strains to our health care system, while providing minimal, if any, benefits.Footnote 167 The FDA followed the same regulation procedure in the face of new AI radiology technology with QuantX.Footnote 168 So far, no issues have surfaced with QuantX. But with continuously learning algorithms on the horizon, like Barzilay’s new software, the FDA will need a more appropriate response to the innovative nature of this new technology.
V. NEW WAYS TO REGULATE THE ISSUE: WHAT WILL ACTUALLY WORK?
The AI/ML discussion paper assumes that every software change can be intentional.Footnote 169 The AI/ML discussion paper supposes that the manufacturer can pause and wait for approval before modifying its software.Footnote 170 This assumption runs counter to the nature of AI/ML technology, which is inherently fluid. The AI/ML discussion paper does allow for learning based on real-world data,Footnote 171 and the software is expected to improve over time. But problems could arise if the software’s capabilities expand beyond its intended use, which could happen in the process of using the software. The designation of certain radiology technology as a Class II device is thus problematic in this context because the FDA does not require many safety and efficacy trials to determine how the device might possibly change with use, or to reexamine the safety and efficacy after changes have already occurred.Footnote 172 Instead, the FDA maintains a faster De Novo or 510(k) review process to prioritize innovation and ease administrative burdens.Footnote 173 While this potential regulatory framework offers significant flexibility to manufacturers in allowing them to create Predetermined Change Protocols so that their devices can update autonomously,Footnote 174 the proposed regulatory framework does not do enough to address cybersecurity, privacy, or safety and efficacy concerns that arise when devices develop over time based on user data.
For example, IoT devices illustrate the pressing need to address cybersecurity, privacy, and safety and efficacy concerns in AI SaMD. IoT devices produce a significant amount of data.Footnote 175 Placing this level of health data in a patient’s hands could result in negative side effects for both the physician and patient.Footnote 176 Manufacturers must strike a balance between giving patients direct access to their health information, and not overburdening physicians with an “avalanche of inconsequential data.”Footnote 177 The efficacy of these devices should be an important consideration given their ability to directly affect the doctor-patient relationship and thus the level of care that the doctor can provide. Additionally, protecting the privacy of this data needs to be a main concern of regulators.Footnote 178 Congress made regulating some IoTs very difficult when it revised the FD&C Act to remove certain general wellness software from the definition of a medical device.Footnote 179 This revision means that the FDA does not have the authority to regulate fitness trackers, coaches, and general wellness apps that do not diagnose or treat medical conditions.Footnote 180 The FDA’s hands are tied when it comes to regulating this particular type of AI SaMD, so other policymakers will need to intervene to make sure these devices remain safe for users. Regulatory and legislative bodies will need to work together to address these concerns in all types of AI SaMD because the current proposed regulatory framework combined with the current legislative authority to regulate certain devices will not provide adequate protection.
A. Monitoring Cybersecurity
The proposed framework generates concern about the outstanding cybersecurity and privacy risks of AI/ML technology, an issue left unaddressed in the January 2021 Action Plan.Footnote 181 The greatest risk rests in IoT devices because they are interconnected via networks. This risk was demonstrated when the FDA discovered that Medtronic’s insulin pumps could be hacked and remotely controlled.Footnote 182 Over 4,000 patients’ continuous glucose monitoring systems could be maliciously accessed and modified to over or under deliver insulin, causing hypoglycemia (low blood sugar) or diabetic ketoacidosis (high blood sugar).Footnote 183 In response to these cybersecurity risks, the FDA suggests that manufacturers monitor their vulnerabilities and devise mitigations to address them.Footnote 184 The FDA has provided extensive advice regarding cybersecurity in general, but has not given much advice regarding the explicit issues arising from AI/ML technology.Footnote 185
One way of monitoring vulnerabilities would be for manufacturers to hire outside consultants to test the companies’ firewalls. These consultants essentially try to hack the device as many ways as possible to detect flaws and develop solutions before an actual hacker does.Footnote 186 The Combination Products Coalition has suggested that the FDA support training for hospitals regarding good practices for data integrity.Footnote 187 However, the FDA does not, and cannot, regulate hospital and physician training.Footnote 188 Instead, the FDA should require manufacturers to conduct vulnerability tests during premarket review and periodically while the product is on the market. Manufacturers should be required to submit cybersecurity reports to the FDA as a part of their commitment to Real-World Monitoring. Cybersecurity monitoring should be a requirement, not a suggestion.
Beyond patient consent and post-market monitoring, patients must be protected in the event of data security breaches. The ISO 14971 sets the international standard for risk management in medical devices.Footnote 189 The latest revision to ISO 14971 redefines “harm” to include the loss of medical and personally identifiable information due to data security breaches.Footnote 190 However, ISO 14971 compliance is not explicitly required by the FDA, but only serves as a best practice for manufacturers to follow.Footnote 191 Legislators or regulators should codify the ISO 14971’s medical device standard to give the FDA, or another government agency, the authority to require explicit compliance now that medical device software has the capability of storing and analyzing patient data.
B. Protecting Patient Privacy
AI/ML use in SaMD raises significant patient privacy concerns because of the ethical impact of generating data and optimizing algorithms based on data from real people.Footnote 192 Facebook’s suicide detection algorithm demonstrates one of the clearest examples of this concern.Footnote 193 Facebook uses algorithms to search posts and messages for suicidal signs and contacts police about ten times per day for “wellness checks.”Footnote 194 The algorithms are kept private as “trade secrets,” but they are unregulated and threaten people’s “privacy, safety, and autonomy.”Footnote 195 Facebook can easily share this data with third parties and other large technology companies. Additionally, police are ill-equipped to handle suicidal and mentally ill people; moreover, sending police to someone’s home without a warrant opens that person up to additional searches and seizures.Footnote 196 Should the FDA be regulating these algorithms like SaMD? Detecting suicidal ideation could be considered a form of medical screening and diagnosis, placing these algorithms in the category of SaMD. Even if the FDA were to regulate this algorithm as SaMD, however, the FDA would provide little protection for patient privacy.
Another problem is that many new algorithms rely on real-world data to function.Footnote 197 They do not use old archives of data, but instead collect data based on interactions with real patients.Footnote 198 There are different types of data collection: organizational data collection (applicant level data), patient data collection (patient reported outcomes data), FDA data and archives, and passive data collection (publicly available, like social media feeds).Footnote 199 For many AI SaMDs, the software delves into very private aspects of people’s lives and identifies patterns of decision-making of which an individual might not even be aware.Footnote 200 For this reason, the FDA has encouraged manufacturers to share personal patient data with the patients themselves.Footnote 201 This call for increased access to patient data illustrates the level of transparency the proposed framework calls for in their real-world monitoring, but again this call is merely a suggestion and not a requirement. The FDA needs to facilitate patient access as algorithms become more advanced. Elon Musk, the CEO of SpaceX and Tesla, has warned that “AI is a rare case where we need to be proactive in regulation instead of reactive because if we’re reactive in AI regulation it’s too late.”Footnote 202
Many mobile health apps that collect large amounts of health data are not subject to HIPAA regulations because they are not “covered entities” under the law and do not diagnose or treat their users.Footnote 203 Unregulated “big data” poses a threat beyond just targeted advertising and can lead to health scoring and discrimination.Footnote 204 When it comes to patient privacy, the FDA often defers to the Department of Health and Human Services or the Federal Trade Commission.Footnote 205 The FDA, however, has the most oversight over medical devices and should take on a more active role to protect patient privacy. The European Union addresses patient privacy in their General Data Protection Regulation (“GDPR”) by focusing on informed consent.Footnote 206 The GDPR states that a person has the right “not to be subject to a decision based solely on automated processing, including profiling, which produces legal effects concerning him or her or similarly significantly affects him or her” without the subject’s “explicit consent” or in “some other limited circumstances.”Footnote 207 To adequately obtain consent, individuals must have access to meaningful information about the significance of their data and how the AI algorithm works.Footnote 208 The FDA needs to mandate this level of rigorous consent in their premarket review and monitor software developers post-market.
The new European Medical Device Regulation, EU Regulation No. 745 (“Regulation 745”) of 2017, expands on the GDPR’s patient privacy concerns and supports more rigorous post-market protections.Footnote 209 Annex I to Regulation 745 requires that SaMDs develop and manufacturer software “in accordance with the state of the art, taking into account the principles of development life cycle and risk management, including information security, verification, and validation.”Footnote 210 Additionally, the regulation sets minimum requirements regarding network and security measures, such as “protection against unauthorized access,” especially unauthorized access that could affect the functioning of the device.Footnote 211
In furtherance of the Regulation 745 requirement, the European Union Agency for Cybersecurity has encouraged health care organizations to comply with security measures by using in-house “cloud services” to avoid security threats caused by third party suppliers.Footnote 212 The Medical Device Coordination Group of the European Commission published additional guidance on how to meet the Regulation 745 requirements and included importers and distributors of medical devices in the list of entities required to meet these requirements illustrating a commitment to post-market surveillance.Footnote 213 In a way, the Regulation 745 and subsequent guidance mirrors a TPLC regulatory approach in terms of monitoring SaMD use over time; however, Regulation 745 makes cybersecurity and privacy a critical benchmark in determining the safety and efficacy of a device by providing concrete requirements for manufacturers to follow.Footnote 214
C. Ensuring Safety and Efficacy
Premarket regulations are often not strong enough to foresee potential harm to patients. A study of medical software from 2011 to 2015 found that 627 medical devices were subject to recall based on software defects.Footnote 215 The FDA’s Pre-Certification Program ignores an individual device’s potential defects by placing the focus on the manufacturer’s “commitment to organizational excellence.”Footnote 216
The European Union addresses safety concerns by requiring more rigorous premarket clinical evidence and more in-depth post-market product monitoring than the FDA.Footnote 217 The GDPR requires strict pre-clinical and clinical data.Footnote 218 Like the FDA’s 510(k) requirements, the GDPR requires a new unique device identification number (“UDI-DI”), for software modifications that change their intended use, but the GDPR takes it a step further and also requires a new UDI-DI for changes to original performance, safety, or interpretation of data.Footnote 219 Minor software revisions, like bug fixes or security patches, still require approval but with a software update identification number (“UDI-PI”).Footnote 220 These strict regulations seem to prohibit continuously learning software while allowing manufacturers to create locked algorithm software if they are willing to take on the major compliance burden.
1. Computational Modeling in Algorithm Change Protocols
The FDA can increase the safety of AI/ML SaMD without the European Union’s extreme measures. The FDA could require software manufacturers to use computational models to conduct safety trials and develop in depth algorithm change protocols. Computational models help manufacturers assess the device’s potential to change over time and supplement clinical trials.Footnote 221 Some manufacturers already use computational modeling and simulation for medical devices like stents, inferior vena cava (“IVC”) filters, and stent-grafts to supplement bench testing and assess potential adaptations or failures.Footnote 222 Computational modeling helps predict post-market failures, but it can also help assess and fix unforeseen failures if they do happen.Footnote 223 Upfront costs of computer models are high, but these costs are much less than of clinical trials.Footnote 224 The FDA has already issued guidance on how to report computational modeling studies and the FDA’s Center for Devices and Radiological Health (“CDRH”) advocates for their use with medical devices.Footnote 225 Computational modeling, however, raises concern over the source, ownership, and use of model data.Footnote 226 The FDA will need to provide further guidance as to how manufacturers should protect the privacy of modeling and training data, as well as control for potential algorithmic bias in the selection of training data.Footnote 227
Increasing the prevalence of computational modeling for AI/ML medical devices could help ensure post-market safety and efficacy by simulating real-world algorithm changes in the development stage to help manufacturers create more realistic Algorithm Change Protocols and address concerns as early as possible.
2. Post-Market Safety Concerns Outside of the FDA’s Scope
Some post-market safety concerns of AI/ML SaMD fall outside of the FDA’s regulatory scope because the issue lies with the device’s implementation. The FDA monitors adverse health outcomes, post-market performance, and manufacturing facilities, but does not have statutory authority to “regulate the practice of medicine and therefore [does] not supervise or provide accreditation for physician training nor [does it] oversee training and education related to legally marketed medical devices.”Footnote 228 This policy can make it difficult to implement uniform safe and effective training practices for AI medical devices, and thus some safety and efficacy concerns cannot be addressed in premarket approvals.Footnote 229
For example, without the direct control of a surgeon, autonomous robots raise serious ethical and liability concerns that need to be proactively addressed before the FDA begins to approve these devices.Footnote 230 For instance, who should be found liable if a surgical error due to AI/ML SaMD harms a patient? If AI/ML SaMD devices are on the market, patients should have opportunities for remedies in the case of harm. Under current U.S. law, a robot cannot be held liable for its actions, and any damage is imputed to the manufacturer, operator, or maintenance personnel.Footnote 231 Researchers have looked to autonomous driving for guidance in assigning liability. The Society of Automotive Engineers (“SAE”), a U.S.-based engineering professional organization, outlined various levels of autonomous cars that could carry different levels of liability, where Level 0 represents full driver control and Level 5 represents full automation with no human intervention.Footnote 232 Under this framework, humans would be fully responsible for the “driver assistance” in Levels 0 through 2, but there seems to be no obvious consensus as to the liability concerns posed by Levels 3 through 5.Footnote 233 The United States has not yet set standards of liability for autonomous cars based on these, or any, levels.Footnote 234
In the case of robotic surgery, medical malpractice suits will largely determine future liability and help answer these questions. However, policymakers and device-manufacturers can be proactive in creating standards of liability, developing consistent training and implementation guidelines to reduce the risk of harm to patients, and providing post-market opportunities to review safety concerns as the AI/ML SaMD adapts over time. It will be up to the industry and agencies to develop consistent processes and regulations that monitor these robots, and other AI/ML SaMD, from design to implementation, because FDA-approval mechanisms can only go so far.
D. Maintaining a Balance Between Innovation and Regulation
AI/ML medical devices generate unique concerns because many SaMD creators are software developers new to the health care industry and the FDA regulatory process. The FDA may need to clarify and simplify the regulatory process to encourage innovation for these new creators.Footnote 235 ML remains a rapidly growing field on which the health care industry needs to capitalize. Analysts expect the deep learning market, a subsection of machine learning, to surpass $18 billion by 2024.Footnote 236 The FDA must be agile in devising new processes, guidance, or regulations that allow these technology companies to move quickly and efficiently so the health care industry can reap the benefits of AI/ML technology.
The FDA’s proposed framework appears flexible in that the FDA remains aware that a continuously learning device can and will change.Footnote 237 The FDA has tried to set up a solution for this adaptive technology, however, the FDA still assumes manufacturers can maintain control of the device to the point where modifications can wait for approval before being updated.Footnote 238 For even bigger changes, the FDA still requires a whole new 510(k) submission.Footnote 239 These submissions are burdensome, but they protect patients from devices working beyond their approved scope, which may be beneficial in protecting patients’ safety.
The TPLC process, however, could create a huge administrative burden for both the FDA and SaMD manufacturers.Footnote 240 Monitoring development and maintenance before, during, and after approval generates a great deal of information for an already overburdened agency to handle. In its AI/ML discussion paper, the FDA has indicated that it expects periodic reporting of updates and performance metrics.Footnote 241 Depending on the risk categorization of the device, these reports could be in the form of a general annual, or otherwise periodic, report, but they may need to be unique for each manufacturer depending on the modifications, risk, and maturity of the algorithm.Footnote 242 The FDA recognizes that each manufacturer will have unique mechanisms for how they update software, how the software impacts the compatibility of supporting devices or accessories, and how they notify users of updates; thus, each manufacturer will have different reporting needs and abilities.Footnote 243 In its comment on the proposed regulations, the Combination Products Coalition recommended piloting a “Real World Data Collection Program” to assess whether the benefits of real-world monitoring for 510(k) devices outweigh the administrative burden to both the FDA and manufacturer.Footnote 244 The proposed regulatory framework will increase administrative burdens on the FDA by requiring additional information from 510(k) devices.Footnote 245 Ultimately though, increased regulation of 510(k) devices would be beneficial considering the FDA’s recent proposal to shift more Class III devices to Class II status.Footnote 246
The FDA will need to be proactive in enforcing its regulations despite the administrative burden. Innovative technology companies are a welcome addition to the health care industry, but concerns remain as to whether they will conform to the strict regulatory burdens of the industry. Silicon Valley has a tendency to “release something new and deal with the consequences later.”Footnote 247 Facebook violated nearly every boundary of privacy long before the Federal Trade Commission intervened; Amazon evaded sales taxes for years to undercut retailers and dominate the market share; Uber and Lyft launched ride-sharing services in cities across the country without obtaining any transportation licenses; and Airbnb avoided all hotel regulations to become a $30 billion international company.Footnote 248 These companies developed cheap and efficient services, banking on quickly building up intense popularity (i.e., consumer buy-in) to pressure officials into allowing them to stay on the market. Technology companies are now eager to shake up the health care industry. They specialize in data and about one third of the world’s data is health care information.Footnote 249 Technology companies are developing medical-grade consumer technology and using their consumer expertise to “enhance and simplify the patient experience.”Footnote 250 If technology companies can disrupt the health care industry and establish consumer by-in before regulators get involved, these companies could harness public pressure to create their own regulations.Footnote 251
New approaches to health care from technology companies that center the patient experience and ease burdens on providers would be beneficial. But these new companies need to know the rules before they jump in, and the health care industry needs to know how to respond to these new players. These two groups do not need to be in opposition to each other but should instead work together and combine their separate expertise. The FDA can facilitate this innovation by clarifying existing regulations, proactively enforcing regulations, relying on industry expertise in the design of regulations, and encouraging open communication and information sharing.
VI. CONCLUSION
AI/ML SaMD manufacturers face an uphill battle as they work hand-in-hand with the FDA to develop effective regulations. The current proposed framework seems sufficient for locked algorithms, where manufacturers can stop and wait for approval before making modifications. The rules, however, might not be well-suited for continuously learning algorithms where changes can be more fluid. By putting up safeguards to stop too many untested algorithm changes, the FDA has succeeded in slowing down and preemptively regulating this new technology. The FDA, health care industry, and relevant policymakers should focus on preemptively ensuring good cybersecurity and privacy practices. Medical device products need to be safe and effective before manufacturers place them on the market. The FDA cannot let Silicon Valley engage in their usual practice of ‘do first, ask for forgiveness later’ when it comes to health care products that directly impact patient safety and privacy. The FDA needs to use preemptive regulations and active enforcement to ensure that software developers do not end up crafting their own regulations. AI technology is rapidly advancing, and we do not want to wait to enforce proactive regulations before it is too late.
VII. APPENDIX
A. Figure 2. Overlay of FDA’s TPLC approach on AI/ML workflow
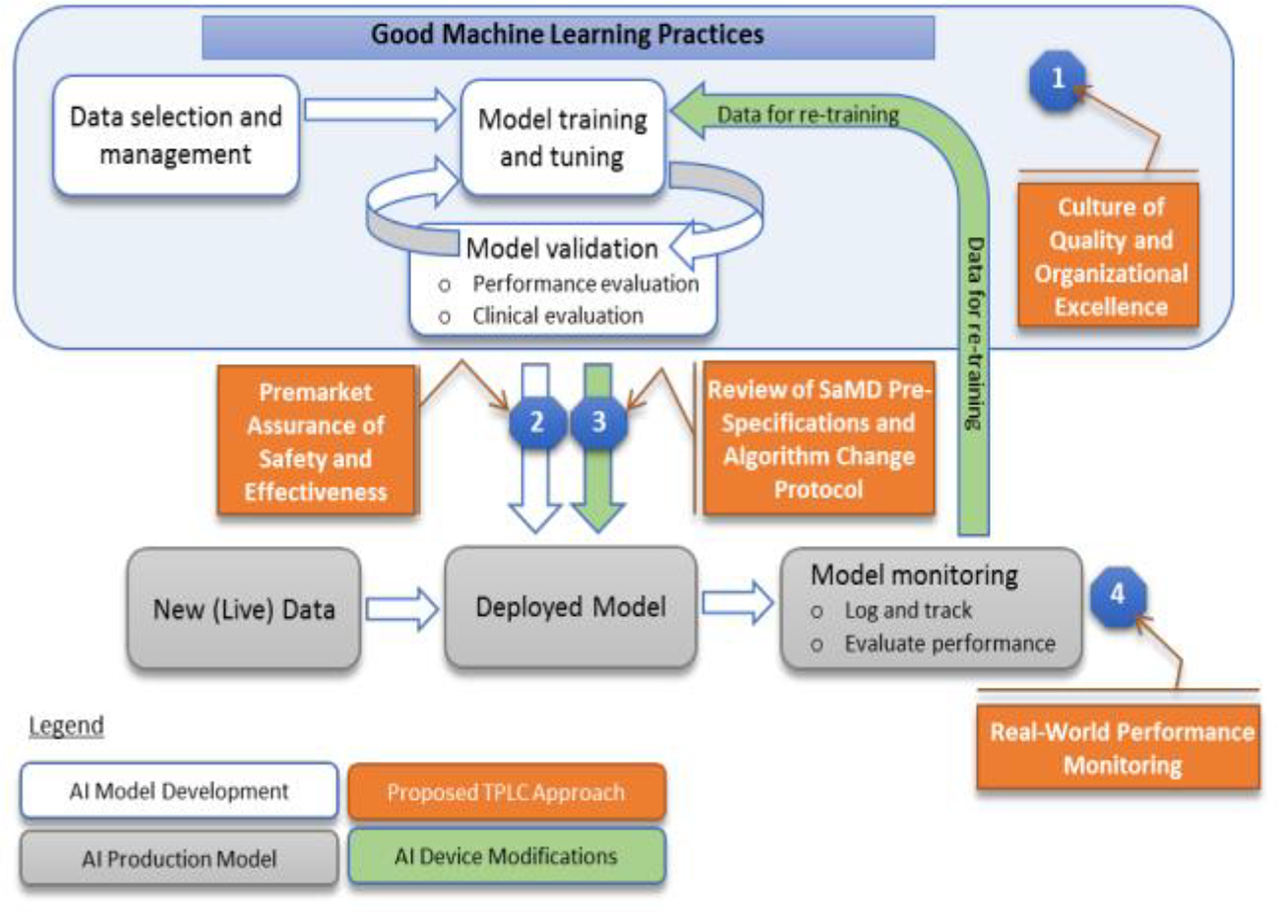
⍏U.S. Food & Drug Admin., Proposed Regulatory Framework for Modification to Artificial Intelligence/Machine Learning (AI/ML)-Based Software as a Medical Device (SaMD) 8 (2019), https://www.fda.gov/media/122535/download [https://perma.cc/4RFZ-QKSS].